Chapter 10 Outcomes data from medical procedures are commonly used to compare treatments or providers. Early databases were originally used to assess outcomes from cardiac surgical procedures, most commonly coronary artery bypass grafting (CABG). These registries were first constructed in the United States from administrative data from the U.S. Health Care Financing Administration (HCFA), the precursor to the current Center for Medicare and Medicaid Services (CMS). Although the purpose of publishing these database registries was to assess outcomes in various clinical programs, providers correctly argued that such data did not account for various patient-specific factors that may impact outcomes.1,2 This objection led directly to the development of a number of high-quality clinical databases and risk models to account for these patient-specific factors, especially in cardiac surgery.3,4 Because patient outcomes may be influenced by severity of illness, treatment effectiveness, and chance, such comparisons must account for differences in the prevalence of patient risk factors, a concept termed “case mix.”5–9 It is possible to reduce or eliminate outcome variability due to case mix through a number of methods, including randomization, which should balance both known and unknown risk factors. However, this is possible only in randomized controlled trials, which have their own shortcomings, such as the “generalizability” of the outcomes. Therefore, we have come to rely on registries when comparing outcomes among various treatments or providers. The use of covariate matching or propensity score matching techniques, which balance known risk factors, can be used to balance patient variables. However, in the majority of observational studies, risk adjustment has been used to account for case mix.10,11 With the use of statistical modeling techniques, most commonly multivariable regression analysis, the association between individual risk factors, known as predictor variables or covariates, and outcomes can be determined. 12 Once the impact of each risk factor is determined from a given population sample, it becomes possible to estimate the probability of the outcome for patients having particular combinations of these risk factors. Although risk models have been utilized for many years, their broader applicability and critical importance became more fully appreciated after the aforementioned 1986 release of unadjusted hospital outcome data by HCFA. Soon after this release, The Society of Thoracic Surgeons (STS) established an Ad Hoc Committee on Risk Factors for Coronary Artery Bypass Surgery. 13 Subsequently, a committee began work on the development of The STS National Cardiac Database, which was formally established in 1989. 14 Subsequently, risk models have been utilized to adjust cardiac surgery outcomes for preoperative patient characteristics and disease severity. 15 Many other clinical databases have been established to assess outcomes not only in cardiac surgery but also in cardiology and other fields. Many of them have developed risk models based on those registries. There is significant variability among these models, which are accounted for by the population studied, the period of the data collection, and the statistical techniques used to develop the model. This chapter examines the intricacies of how these factors bear on the assessment of risk in patients with valvular heart disease. Risk scores are predicted probabilities calculated from a multivariable logistic regression model calibrated on data from within a fixed time. It is, of course, a given that no risk adjustment model is better than the data on which it is based. Administrative databases, such as the CMS Medicare Provider Analysis and Review (MEDPAR) file, provide a readily available source of data on millions of patients. However, the file is based on claims data used for administrative billing purposes and does not capture key clinical data fields, such as ejection fraction, that may play a significant role in clinical outcomes. Hence, the first key component in constructing a robust risk model is the use of a clinical database with as complete and accurate data as possible. The second key factor is to use statisticians with sufficient experience in risk modeling because different multivariable equations can be developed from the same data. 15 Three techniques have been used for construction of cardiac surgery risk models. Bayesian models were initially used for the STS database to account for the significant amount of missing data. As data completeness improved, logistic regression models were used; The most common statistical technique used currently, logistic regression is employed not only by the STS but also by the models constructed by New York State, 16 the Veterans Administration, 17 and the Northern New England Cardiovascular Disease Study Group. 18 Other models, such as the Parsonnet score 19 and the European System for Cardiac Operative Risk Evaluation (EuroSCORE), 20 use simple additive scores with weights derived from logistic regression models. There is some evidence that logistic regression models perform better. 21 For development of a risk model, the study population is usually divided into a development or training sample and a validation or test sample. For the STS Isolated Valve Risk model, the study population was randomly divided into a 60% development sample and a 40% validation sample. The development sample was then used to identify predictor variables and estimate model coefficients. Data from the validation sample were used to assess model fit, discrimination, and calibration. 22 Discrimination refers to the model’s ability to separate two groups studied, for example, survivors and nonsurvivors. An area under the receiver operating characteristic curve (AUROC) is calculated using the c-index, with ranges between 0.5 and 1.0. The higher the value of the c-index, the better the discrimination, whereas values closer to 0.5 indicate that the model’s ability to discriminate is no better than random chance or the “flip of a coin.” 23 In most risk prediction models used for cardiac surgery, the AUROC ranges between 0.75 and 0.80. In the employment of risk adjustment, important limitations have to be taken into account in order that valid information and not “misinformation” is obtained from the “correction.” 24 First, risk algorithms are accurate only for the population and in the time frame in which they are developed and validated. Second, risk adjustment loses accuracy at the extremes of the population studied, where there are too few patients upon which to build a statistically valid model. This “tail of the bell-shaped curve” is where high-risk patients with aortic stenosis “reside,” accounting for some of the overestimation of risk seen with many models.25,26 Third, risk algorithms cannot reliably be applied directly to populations and treatments other than those in which they were developed. The implication is that although both surgical aortic valve replacement (AVR) and transcatheter aortic valve implantation (TAVI) are used in treating patients with aortic stenosis, AVR risk algorithms are based on “surgical” AVR outcomes and therefore may not to be directly applicable to TAVI. Fourth, risk algorithms cannot account for variables not collected or analyzed. This lack of accounting has one of two causes: (1) the occurrence of the factor or condition is so infrequent that its impact cannot be measured (e.g., porcelain aorta) or (2) the factor either may have not been previously known to be a factor that was causal or cannot be accurately measured or quantified. The role of frailty and its impact on outcomes of treatment is a case in point. Fifth, all risk predictors fall prey to the phenomenon “garbage in equals garbage out.” Unless the factors upon which the algorithm is formulated are based on complete and accurate data, an inaccurate predictor will result. A corollary is that the risk predictor must be “user friendly.” The greater number of variables collected in formulation of the risk algorithm, the more accurate the prediction of risk; however, the more burdensome the collection of data required, the less complete and accurate will be the information. So there must be a balance between including all information that is likely to be a factor in causing risk and “user-friendliness” in order to facilitate complete and accurate collection and ensure that the tool is routinely employed in decision making. Indeed, one risk algorithm for aortic stenosis, the Age, Creatinine, Ejection Fraction (ACEF) score, provides reasonable prediction using only the three factors in its name: age, serum creatinine level, and ejection fraction. 27 Profiling risk in patients undergoing medical procedures serves many purposes. 28 First, it allows outcomes prediction for individual patients so that both patient and caregiver can be better informed in making decisions regarding the advisability and risks of a specific medical procedure. Second, patients undergoing medical procedures frequently have comorbidities that cause varying levels of risk and therefore may adversely affect the outcomes of a procedure. When different modalities of treatment or different caregivers are compared, risk adjustment allows for a balanced analysis of outcomes by accounting for the risk factor variation among different patient cohorts. This correction allows for a “more level playing field” of outcomes assessment; also, this achievement of an “apples-to-apples” comparison is one of the advantages of clinical outcomes databases over administrative databases, which have limited ability to adjust risk. The earliest and most common use of risk prediction was for evaluating early mortality after isolated CABG. Because the procedure was performed commonly and outcomes were publicly reported, risk prediction for an “apples-to-apples” comparison among surgical centers performing CABG became common. “Early mortality,” as defined by the STS, includes all deaths occurring before 30 days whether in or out of the hospital and any death occurring in the hospital at any time. Other risk prediction models for early mortality include only in-hospital mortality, which misses approximately 10% of the early deaths. The advantage of reporting in-hospital mortality is that the data are more easily collected and probably more accurate. The disadvantage, however, is that very ill postoperative patients who are quite likely to die are frequently discharged to long-term acute care or skilled nursing facilities less than 30 days after surgery and are therefore not counted. In a 2012 analysis of EuroSCORE II outcome data, for example, in which hospital mortality is around 4%, adding 30-day mortality increases the reported mortality by about 0.6%, and adding 90-day mortality increases it further by about 0.9%. 29 When one is comparing various risk predictors, it is important to be sure that the same data definitions are being used by each model. Risk prediction models for early mortality after cardiac surgery have been expanded to use for other procedures. In addition isolated CABG (c-index 0.78), risk prediction is available for isolated surgical AVR, isolated mitral valve repair or replacement, CABG combined with AVR, and CABG with mitral valve repair or replacement. The weighting of the various risk factors is recalibrated with each new version of the STS Adult Cardiac Database according to the most recent data uploaded by the 1,005 cardiac surgery programs in the United States that participate in the database. Rankin et al 30 have published a risk prediction for multiple valve operations, including aortic and mitral valve operation, mitral and tricuspid and aortic valve operation, and mitral and tricuspid operation, that has acceptable discrimination (c-index 0.711 to 0.727). 30 In addition to early mortality, the STS risk prediction algorithm has been demonstrated to be predictive of long-term survival for isolated CABG, with survival at 1,3,5 and 10 years having similar AUROC values to the value of 30-day survival (0.794). 31 A composite score of mortality and major morbidity after surgical AVR has also been published. 32 The STS AVR composite score is based solely on outcomes, including risk-standardized mortality and any-or-none risk-standardized morbidity (occurrence of sternal infection, reoperation, stroke, renal failure, or prolonged ventilation). The STS online risk calculator is capable of calculating major morbidity in addition to mortality after surgical AVR. 33
Risk Assessment for Valvular Heart Disease
Background
How Risk Algorithms Are Constructed
Limitations of Risk Algorithms
Uses of Risk Algorithms
What Can Be Predicted?
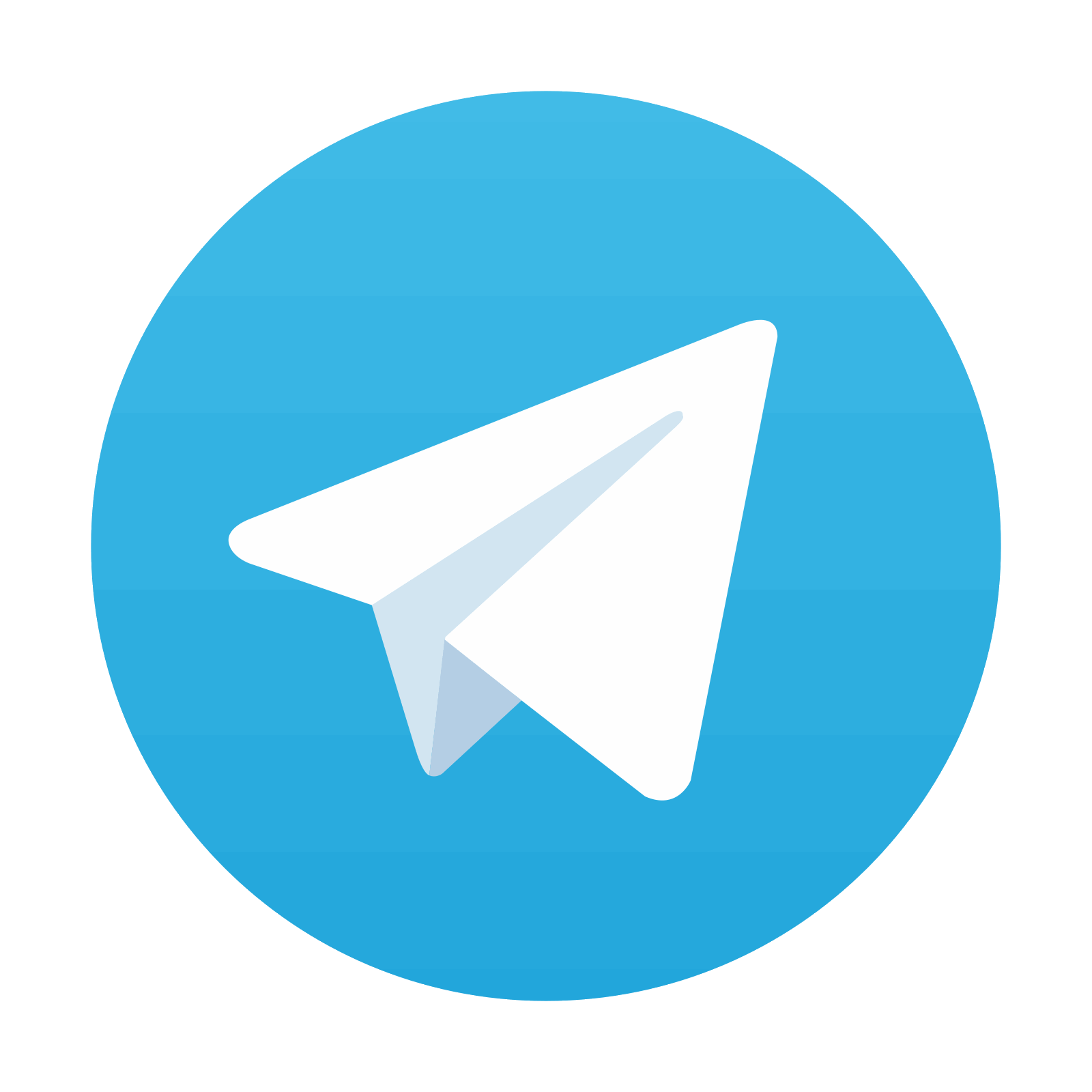