Key Points
- •
Risk estimation usually originates with observational studies of the incidence of coronary heart disease events over time.
- •
Prediction of risk is dependent on accurate and precise baseline measurements in persons without coronary disease at the time of measurement.
- •
Follow-up of 10 years is a typical interval of interest for the prediction of coronary disease events in adults who are asymptomatic at baseline.
- •
Performance criteria for risk estimation include discrimination, calibration, and reclassification.
- •
Newer risk factors and biomarkers for heart disease can be evaluated in the context of existing risk estimation approaches.
Prediction of heart disease has become possible because of the long-term experience in observational studies that included detailed information on elements of risk before the development of clinical disease. Storage of information, computerization, and exportability of risk prediction tools have facilitated this process. The origins of coronary heart disease (CHD) risk estimation, the role of baseline measurements, determination of outcomes, statistical programming, algorithm development, and performance evaluation are the key concepts that underlie this discipline.
Many factors contribute to the risk for CHD and to the risk for cardiovascular disease (CVD) in general. The primary focus of this chapter is estimation of risk for CHD over a 10-year interval. There is considerable agreement about the key factors that are effective predictors of initial CHD events. Although there are differences between the predictions of CVD and of its constituent events (peripheral arterial disease, stroke, and heart failure ), there are many similarities, and information on the prediction of CVD is also provided.
Origins of Estimation of Risk for Coronary Heart Disease
The prediction of CVD outcomes has evolved considerably over recent years. Initial efforts were related to the development of logistic regression data analysis and its adaptation to the prediction of CHD events. The Framingham Heart Study began in 1948, and the researchers initially evaluated the role of factors such as age, sex, high blood pressure, high blood levels of cholesterol, diabetes mellitus, and smoking as risk factors for the onset of first CHD events. Logistic regression methods became available on large-frame computers in the 1950s and 1960s. This process involved assembling data for a population sample that had been monitored prospectively for the occurrence of a dichotomous event such as clinical CHD.
The initial approach involved identifying persons free of the vascular event of interest, obtaining baseline data on factors that might affect risk for the outcome, and monitoring the participants prospectively for the development of the clinical outcome under investigation. The original participants in the Framingham study returned for new examinations and assessment of new cardiovascular events every 2 years, and the researchers, using logistic regression in the data from the original Framingham cohort, developed cross-sectional pooling methods to assess risk over time.
Baseline Measurements as Predictors of Risk for Coronary Heart Disease
To develop reliable estimations of CHD risk, it is important to have a longitudinal study, standardized measurements at baseline, and adjudicated outcomes that are consistent over the follow-up interval. It is possible to undertake multivariate analyses of factors that might be associated with a vascular disease outcome in a cross-sectional study, but it is preferable to have a prospective design to fully understand the role of factors that might increase risk for developing a vascular disease event.
A prospective design is necessary because critical risk factors may change after the occurrence of CHD, and such a design allows the inclusion of fatal events as outcomes. The literature related to tobacco use and risk of CHD is informative with regard to this issue. After experiencing a myocardial infarction, a person may stop smoking or may underreport the amount of smoking that occurred before the occurrence of a myocardial infarction, which could lead to analyses in which the effect of smoking on risk for myocardial infarction would be underestimated.
Standardized measurements are important to use in assessing the role of factors that might increase risk for vascular disease outcomes. For example, blood pressure levels are typically measured in the arm with a cuff that is of appropriate size and is inflated and deflated according to a protocol; the level of the arm is maintained near the level of heart; measurements are taken in patients who have been sitting in a room at ambient temperature for a specified number of minutes; a sphygmomanometer that has been standardized is used; and determinations are made by properly trained personnel. Blood pressure can be measured inaccurately for many reasons, including inconsistent positioning of the patient, varying the time the subject is at rest before measurement, varying credentials of the examiner (e.g., nurses vs. doctors), and rounding errors when the measurements are recorded.
Lipid standardization has been helpful in ensuring accuracy and precision of lipid measurements, which are used to help assess risk for cardiovascular events, and measurements are typically obtained in the fasting state. The Lipid Research Clinics Program, initiated in the 1970s, led to the development of a Lipid Standardization Program at the Centers for Disease Control and Prevention, with monitoring of research laboratories that measure cholesterol, high-density lipoprotein (HDL) cholesterol, and triglyceride levels. This program updated the laboratory methods and techniques over time to accommodate newer methods of measurement.
Laboratory determinations have several potential sources of variability, including preanalytic, analytic, and biologic sources. Preanalytic sources of error include fasting status, appropriate use of tourniquets during phlebotomy, room temperature, and sample transport conditions. Laboratory variability is minimized through the use of high-quality instruments, use of reliable assays, performance of replicate assays, and use of algorithms to repeat assays if the difference between results of replicate assays exceeds specified thresholds. Other methods to ensure accuracy and precision with laboratory determinations include the use of external standards, using batching samples, and minimizing the number of lots for calibration. Sources of biologic variability include fasting status, time of day, season of the year, and intervening illnesses.
Another key risk factor is diabetes status. In many of the older studies, subjects did not fast for each clinical visit, and an expert-derived diagnosis of diabetes mellitus was used on the basis of available glucose information, medication use, and chart reviews. The American Diabetes Association has changed the criteria for diabetes since the 1970s. For example, diabetes was considered present in 1979 if fasting glucose level was 140 mg/dL or higher or if a nonfasting glucose level was higher than 200 mg/dL. These criteria were revised in 1997 so that a fasting glucose level of 126 mg/dL or higher was considered to be diagnostic for diabetes mellitus.
Coronary Heart Disease Outcomes
Total CHD (angina pectoris, myocardial infarction, and death from CHD) and “hard” CHD (myocardial infarction and death from CHD) are the outcomes that have been studied most frequently, but other investigators have reported on the risk of “hard” CHD; their studies included persons with a baseline history of angina pectoris, and the European CHD risk estimates have focused on the occurrence of death from CHD.
History of Estimation of Risk for Coronary Heart Disease
In the early 1970s, CHD risk was estimated with the use of logistic regression methods and cross-sectional pooling with the variables age, sex, blood pressure, cholesterol level, smoking, and diabetes. In initial research on CHD prediction, investigators used logistic regression analyses, and the relative risk effects for each of the predictor variables were provided. Time-dependent regression methods and the addition of HDL cholesterol levels as an important predictor led to improved prediction models for CHD, in which score sheets and regression equation information with intercepts were used to estimate absolute risk for CHD over an interval that typically spanned 8 to 12 years of follow-up.
Score sheets to estimate CHD risk were highlighted in a 1991 Framingham study–related publication about CHD risk in which total CHD was predicted, as were various first cardiovascular events. The outcome of interest was prediction of a first CHD event on the basis of the independent variables age, sex, high blood pressure, high blood cholesterol, diabetes mellitus, smoking, and left ventricular hypertrophy detected on the electrocardiogram (ECG-LVH). Risk equations with coefficients were provided to allow estimation of CHD risk by means of score sheets, pocket calculators, and computer programs.
A 1998 Framingham study–related article on CHD risk estimation showed little difference in the overall predictive capability for total CHD when total cholesterol level was replaced in the calculations by low-density lipoprotein (LDL) cholesterol, which suggested that an initial lipid screening with total cholesterol, HDL cholesterol, age, sex, systolic blood pressure, diabetes mellitus, and smoking had good overall predictive capabilities without lipid subgroup measurements. The 1998 CHD risk analyses did not include information on ECG-LVH as a risk predictor because the Joint National Committee on Prevention, Detection, Evaluation, and Treatment of High Blood Pressure had not recommended that electrocardiography be performed on asymptomatic middle-aged persons. Also, the prevalence of ECG-LVH was very low (a small percentage) in middle-aged white populations. In contrast, among African Americans, ECG-LVH has been much more common. It is thought that including electrocardiography might be particularly helpful for estimating CHD risk in African Americans and in other racial and ethnic groups in which ECG-LVH is more common and in which the population burden of hypertension is greater.
A workshop was convened by the National Heart, Lung and Blood Institute in 2001 to assess the ability to estimate risk of first CHD events in middle-aged Americans. In summaries of the workshop proceedings, D’Agostino and colleagues and Grundy and associates compared the predictive results for CHD in several studies by using equations used in the Framingham study or equations in which the variables were the same as those in the Framingham risk-estimation equations but with study-specific predictions. Participants in the workshop evaluated the role of calibration and used statistical adjustments for differences in risk factor levels and incidence rates. The summary findings included the following: (1) Relative risks for the individual variables were similar to those in the Framingham experience; (2) the Framingham equations predicted CHD quite well when applied to other populations, and the C-statistic for the Framingham prediction was usually very similar to the C-statistic from the study-specific predictor equation; and (3) in African Americans and Japanese American men from the Honolulu Heart Study, the Framingham equation had much less capability for discrimination.
Coronary Heart Disease Risk Algorithm Development
It is helpful to understand how CHD risk algorithms are currently developed and how performance criteria are used to evaluate prediction algorithms. The key starting point is the experience of a well-characterized prospective study cohort that is generally representative of a larger population group. That initial stipulation can help to ensure the generalizability of the results. Only data from subjects with complete outcome and covariate information for a given endpoint are used in the analyses.
Risk estimates for CHD are usually derived from proportional hazards regression models according to methods developed by Cox. The variables that are significant in the individual analyses are then considered for inclusion in multivariable prediction models according to a fixed design or a stepwise model in which an iterative approach is used to select the variables for inclusion. Pairwise interactions can be considered for inclusion in the model, but it may be difficult to interpret those results, and interactions may be less generalizable when tested in other population groups.
Traditional candidate variables considered for these analyses in American and European formulations have typically included systolic or diastolic blood pressure, blood pressure treatment, cholesterol level, diabetes mellitus, current smoking, and body mass index. Information related to treatment, such as blood pressure medication, should be included with caution in this situation because the risk algorithm is typically being developed from an observational study with a prospective design, not from a clinical trial in which treatments are randomly assigned. Some prediction equations have included data from persons with diabetes mellitus, but the Adult Treatment Panel guidelines reflected the opinion that persons with diabetes mellitus were already at high risk for CHD and that risk assessment was therefore not needed for these individuals. Reports and reviews published since 2001 have called into question whether diabetes mellitus is a “CHD risk equivalent,” and data have shown that the risk of a subsequent CHD event is approximately twofold for persons known to have diabetes mellitus and fourfold for those who have already experienced CHD.
A validation group is used to test the usefulness of the risk prediction algorithm. One approach is to use an internal validation sample within the study. By this method, a fraction of the data are used for model development, and the other fraction of the data are used for validation. An alternative to this approach is to take a very large fraction of the persons in the study and successively develop models from near-complete data sets. External validation of a risk prediction model—testing the use of the model in other population samples—is especially useful and provides the first indication of whether it is possible to generalize the risk prediction model to other scenarios.
Performance Criteria for Coronary Heart Disease Risk Algorithms
A variety of statistical evaluations are now available to evaluate the usefulness of CHD risk prediction and they are discussed successively as follows.
Relative Risk
For each risk factor, proportional hazards modeling yields regression coefficients for a study cohort. The relative risk of a variable is computed by exponentiating the regression coefficient in the multivariate regression models. This measure estimates the difference in risk between someone with a given risk factor such as cigarette smoking and someone who does not smoke. An analogous approach can be undertaken to estimate effects for continuous variables by showing effects for a specific number of units for the variable or by identifying differences in risk that are associated with a difference in the number of units that, in turn, are associated with a standard deviation for the factor.
Discrimination
Discrimination is the ability of a statistical model to distinguish patients who experience clinical CHD events from those who do not. The C-statistic is the typical performance measure used, which is analogous to the area under a receiver operator characteristic curve; it is a composite of the overall sensitivity and specificity of the prediction equation ( Figure 3-1 ). The C-statistic represents an estimate of the probability that a model will assign a higher risk to patients who develop CHD within a specified follow-up period than to patients who do not. The error associated with C-statistic estimates can itself be estimated.

Values for the C-statistic range from 0.00 to 1.00, and a value of 0.50 reflects discrimination by chance. Higher values generally indicate agreement between observed and predicted risks. The average C-statistic for the prediction of CHD is approximately 0.70. Using a large number of independent predictor variables can lead to better discrimination but can also “overfit” the model, whereby the statistical model can work very well for the derivation data set but have much lower discriminatory capability and limited accuracy in predicting the occurrence of outcomes with other data.
Calibration
Calibration is a measure of how closely predicted estimates correspond with actual outcomes. To present calibration analyses, the data are separated into deciles of risk, and observed rates are tested for differences from the expected rates across the deciles; they are tested with a version of the Hosmer-Lemeshow chi-square statistic. Smaller chi-square values indicate good calibration, and values higher than 20 generally indicate significant lack of calibration.
Recalibration
An existing CHD prediction model can be recalibrated if it provides relatively useful ranking of risk for the population being studied, but the model systematically overestimates or underestimates CHD risk in the new population. For example, recalibrating the Framingham risk-prediction equation would involve inserting the mean risk factor values and average incidence rate for the new population into the equation. Kaplan-Meier estimates can be used to determine average incidence rates. This approach was undertaken for Framingham risk-prediction equations that were applied to the CHD experience of Japanese-American men in the Honolulu Heart Study and for Chinese men and women. In each of these scenarios, the Framingham risk-prediction equation provided relatively good discrimination but did not provide reliable estimates of absolute risk. A schematic of such an approach is shown in Figure 3-2 , where the left panel shows CHD risk is systematically overestimated when the Framingham equation is applied to another population. After calibration, the estimation fits the observed experience much more closely, and the Hosmer-Lemeshow chi-square value is much lower.

Reclassification
Specialized testing in subgroups has been used to reclassify risk for vascular disease. An example of such an approach is the use of exercise testing to upgrade, downgrade, or confirm estimates of vascular disease risk in patients being evaluated for angina pectoris. CHD algorithms may do a reasonably good job in prediction of CHD risk, and the inclusion of a new variable may have minimal effects on C-statistic estimates. Methods developed to assess this approach have used a multivariate estimation procedure and tested the utility of a new test to increase, decrease, or confirm risk estimates. Pencina and coworkers published an updated method to assess reclassification that takes into account the potential reclassification of both cases and noncases.
Reclassification has practical applications, as shown in Figure 3-3 , in which an initial probability of CHD is estimated from a multivariate prediction equation, and additional information then provides an updated estimation of risk, which is commonly called the posterior estimate. If the new information did not provide any added value, the risk estimate would be the same as for the initial calculation, and the risk estimate would lie close to the identity line. The schematic shows the hypothetical effects for a small number of patients. For some individuals, the test result was positive, increasing the posterior risk estimates. On the other hand, negative tests moved the risk estimates downward for some individuals.

The magnitude of effects can be shown graphically by the length of the vertical lines and how they differ from the identity line. It is important to evaluate a posterior risk estimate that would reclassify the individual to a lower or higher risk category. For example, Figure 3-3 shows seven persons with an initial probability of developing disease in the 10% to 20% range. At the intermediate level the risk was increased in three persons and decreased in four persons with new variable information, but some of the risk differences did not differ appreciably from the initial estimates. Risk was reclassified into a higher category for only one person and to a lower category for two persons. Some authors have used performance measures such as the Bayes Information Criteria as another method to interpret potential effects of reclassification.
Current Estimation of Risk for Coronary Heart Disease
The current starting point for using a CHD risk-prediction equation in a person being screened for CHD is a medical history and a clinical examination with standardized collection of key predictor (independent) risk factors: age, sex, fasting lipids (total, LDL, and HDL cholesterol; ratio of total cholesterol to HDL cholesterol), systolic blood pressure, history of diabetes mellitus treatment, fasting or postprandial glucose levels, and use of tobacco and other substances ( Table 3-1 ). This information can be used to estimate risk of CHD over a 10-year interval through the use of score sheets or computer programs, as described at the website for the Framingham Heart Study ( http://www.framinghamheartstudy.org ). Risk estimation over 10 years with a score sheet based on the Framingham experience was used by the National Cholesterol Education Program in the Adult Treatment Panel III Guidelines ( Figure 3-4 ), and an interactive calculator is also available on the Internet ( http://hp2010.nhlbihin.net/atpiii/calculator.asp?usertype=prof ).
Variable | Reference | |||||||
---|---|---|---|---|---|---|---|---|
Wilson et al (1998) | ATP III (“Executive Summary,” 2001) | Assmann et al (2002) | euroSCORE (Conroy et al, 2003) | Wolf et al (1991) | Murabito et al (1997) | Butler et al (2008) | D’Agostino et al (2008) | |
Source | Framingham study | Framingham study | PROCAM | Europe | Framingham study | Framingham study | Health ABC study | Framingham study |
Outcome * | Total CHD | Hard CHD | Hard CHD | CHD mortality | Stroke | Intermittent claudication | Cardiac failure | Total CVD |
Age interval | 5 years | 5 years | 5 years | 5 years | Intervals vary | 5 years | 5 years | 5 years |
Inclusion criteria | No CHD | No CHD | Possible CHD | No CHD | Possible CHD | Possible CHD | Possible CHD | No CVD |
Sex | Men, women | Men, women | Men | Men, women | Men, women | Men, women | Men, women | Men, women |
BP levels | JNC category | Systolic BP | Systolic BP | Systolic BP | Systolic BP | JNC category | Systolic BP | Systolic BP |
BP therapy | No | Yes | No | No | Yes | No | No | Yes |
Cholesterol | Yes | Yes | No | Yes | No | Yes | No | Yes |
HDL cholesterol | Yes | Yes | Yes | No | No | No | No | Yes |
LDL cholesterol | Optional | No | Yes | No | No | No | No | No |
Cigarette smokers | Yes | Yes | Yes | Yes | Yes | Yes, number/day | No | Yes |
Glycemia | Patients with DM included | Patients with DM excluded | Diabetes status | Diabetes status | Diabetes status | Diabetes status | Glucose level | Diabetes status |
Other factors | — | — | — | — | ECG-LVH, atrial fibrillation | — | Heart rate ECG-LVH, serum albumin, creatinine | — |
Baseline CVD included | ECG-LVH | No | MI history | No | CHD | No | CHD | No |
* “Total CHD” refers to angina pectoris, myocardial infarction, and death from CHD; “hard CHD” refers to myocardial infarction and death from CHD.

Specialized models have been developed for persons with type 2 diabetes in which additional potential predictor variables are considered. The experience of diabetic patients who participated in the United Kingdom Prospective Diabetes Study has been used to develop this prediction algorithm, which can be accessed on the Internet ( www.dtu.ox.ac.uk/riskengine ). Stevens and colleagues, the authors of the algorithm, reported that the key predictor variables for initial CHD events were age, diabetes duration, presence of atrial fibrillation, glycosylated hemoglobin level, systolic blood pressure level, total cholesterol concentration, HDL cholesterol concentration, race, and smoking status.
European groups have developed strategies to estimate risk of CHD with European data. Investigators from the Prospective Cardiovascular Munster (PROCAM) in Germany monitored a cohort for the development of CHD, and their results were generally similar to what has been estimated from Framingham data (see Table 3-1 ). Their analyses were restricted to men. The factors significantly associated with the development of a next CHD event included age, LDL cholesterol concentration, smoking, HDL cholesterol concentration, systolic blood pressure, family history of premature myocardial infarction, diabetes mellitus, and triglyceride levels. The investigators in the Operative Urban Centers for Economic Requalification (CUORE) cohort study in Italy undertook prediction analyses in middle-aged men who were monitored for 10 years for CHD events. They found that age, total cholesterol concentration, systolic blood pressure, cigarette smoking, HDL cholesterol concentration, diabetes mellitus, hypertension drug treatment, and family history of CHD were associated with initial CHD events.
The CUORE investigators also tested the utility of Framingham and PROCAM estimating equations in Italy. They found that, in general, both Framingham and PROCAM overestimated CHD risk in Italian men, and after calibration of the Framingham equations, it was possible to reliably predict CHD events in their study cohort. Risk scores have also been developed in the United Kingdom (the QRISK calculator) and Scotland (the ASSIGN calculator) with consideration of the effects of social deprivation. The QRISK algorithm predicts total CVD according to age, sex, smoking status, systolic blood pressure, ratio of total serum cholesterol to high-density lipoprotein level, body mass index, family history of CHD (in a first-degree relative younger than 60), area measure of deprivation, and existing treatment with antihypertensive agent.
The European System for Cardiac Operative Risk Evaluation (euroSCORE) algorithm is currently the most popular CHD prediction algorithm in Europe (see Table 3-1 ). It predicts CHD mortality and includes data from a large number of studies across Europe to generate the risk-prediction algorithms. The factors used in the prediction included age, sex, smoking, systolic blood pressure, and the ratio of total cholesterol concentration to HDL cholesterol concentration. Slightly different versions of the risk-scoring algorithm are used in regions of higher risk (generally more Northern latitudes) than in regions of lower risk (more Southern regions of Europe). Unfortunately, not enough of the participating centers had data on CHD morbidity, and a prediction algorithm for total CHD that is based on experience across Europe is still in development.
Prediction of First Cardiovascular Disease Events
Approximately two thirds of CVD events represent CHD (myocardial infarction, angina pectoris, CHD death). There is considerable interest in the prediction of CVD in general and in the vascular disease events that do not represent CHD, such as intermittent claudication, stroke, and cardiac failure. For example, the determinants of intermittent claudication in the Framingham study were shown to be age, male sex, blood pressure, diabetes mellitus, cigarette smoking, cholesterol level, and HDL cholesterol level ( Figure 3-5 ; see also Table 3-1 ). A slightly different approach was undertaken in the prediction of first stroke events, and data from persons with heart disease at baseline were included in the analyses undertaken by Framingham investigators. They reported that age, male sex, blood pressure level, diabetes mellitus, and CHD were predictive of the incidence of stroke during follow-up ( Figures 3-6 and 3-7 ; see also Table 3-1 ). Similarly, the prediction of cardiac failure has often included data from persons known to have experienced CHD as at-risk individuals. For example, predictors of cardiac failure in the Health, Aging, and Body Composition (Health ABC) cohort included age, sex, coronary artery disease at baseline, systolic blood pressure, heart rate, left ventricular hypertrophy, cigarette smoking, fasting glucose level, serum creatinine concentration, and serum albumin concentration (see Table 3-1 and Figure 3-8 ).
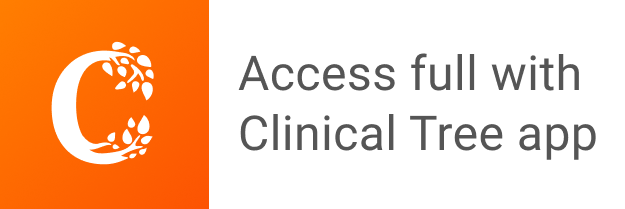