Introduction
In 1836 Elisha Bartlett, the editor of the American Journal of Medical Sciences, heralded a study as “one of the most important medical works of the present century, marking the start of a new era in science.”1 What evoked such praise and suggested a paradigm shift was Dr Pierre Louis’ systematic collection and numerical presentation of data on blood letting. Louis adopted a Baconian approach of collecting vast amounts of data on a large number of patients (by the standards of the early 1800s), which allowed him to systematically evaluate the efficacy of blood letting. Louis argued that large numbers of patients and enumeration were necessary to equalize differences between treatment groups since “by so doing, the errors (which are inevitable), being the same in two groups of patients subjected to different treatment, mutually compensate each other, and they may be disregarded without sensibly affecting the exactness of the results.”2 Louis subsequently went on to state: “a therapeutic agent cannot be employed with any discrimination or probability of success in a given case, unless its general efficacy, in analogous cases, has been previously ascertained” and thus, “without the aid of statistics nothing like real medicine is possible.”3
The prevailing concept of illness, at the time, was that the sick were contaminated, whether by some toxin or contagion, or an excess of one humor or another. This understanding of illness contained within it the idea that these states were improved by opening a vein and letting the sickness run out. Louis’ finding that blood letting hastened the death of the ill was a bombshell. George Washington had 2.4 liters of blood drained from him in the 15 hours prior to his death; he had been suffering from a fever, sore throat, and respiratory difficulties for 24 hours.4 Some have suggested that Washington was murdered.5–7
While this is a relatively recent example, the plea for comparative evaluation is mentioned as early as the Old Testament. Throughout history there have been repeated exhortations to quantify medical or health problems and to compare outcomes in patient groups managed differently, with the goal of setting state policy or assisting individual physicians.
In this chapter we will consider what evidence-based medicine is and then discuss an approach to evidence -based decision making. We will use a clinical case to highlight the components of this approach which include: clinical state and circumstances, patient preferences and actions, research evidence, and clinical expertise. At the end of the chapter we will review the application of these components of evidence-based decision making as they apply to our patient and provide a decision aid that clinicians can use in such a case. This chapter is an overview of core concepts and other chapters in this book (e.g. clinical trials and meta-analysis) provide more in-depth coverage of specific topics.
What is evidence-based medicine?
Although the foundations for evidence-based medicine were laid over several centuries, an explicit philosophy with its attendant concepts, definitions, and models has been largely developed as a formal doctrine only during the last few decades. Evidence-based medicine is about solving clinical problems. Initially, the focus of evidence-based medicine was largely on finding the best objective quantifiable research evidence relevant to the particular problem, and applying that evidence in resolving the particular issue.8 This early focus de-emphasized “intuition, unsystematic clinical experience, and pathophysiologic rationale as sufficient grounds for clinical decision making” and stressed “the examination of evidence from clinical research.”9 Subsequent versions of evidence-based decision making have emphasized that research evidence alone is rarely sufficient to make a clinical decision.10 Research evidence by itself seldom tells us what to do in individual situations, but rather it provides useful information that allows us to make more informed decisions. Clinicians must always view evidence in the context of the individual patient and then weigh the potential benefits versus the risks, costs, and inconveniences of each action. Ideally the patient’s values and preferences affect these issues10
An initial description of evidence-based medicine from an editorial in 1996 provided the following definition: “Evidence-based medicine is the conscientious and judicious use of current best evidence from clinical care research in the management of individual patients.”10 The editorial also included the caveat that the definition of evidence -based medicine will evolve as new types of information emerge and therefore will be continuously refined. The concepts of evidence-based medicine have evolved considerably and the current model is outlined in Figure 1.1.8 In the next section we use this model of evidence -based clinical decision making to help resolve a common clinical scenario.
Approach to evidence-based clinical decision making
Clinical scenario
A family physician refers a patient who has just moved cities to live with his daughter. The physician is requesting our input on the appropriateness of antithrombotic therapy. The patient is an 80-year-old male with a history of hypertension who 10 months ago, on routine exam, was diagnosed with atrial fibrillation. The patient suffered a major gastrointestinal bleed, requiring hospitalization, urgent endoscopy, and a transfusion 1 month prior to his diagnosis of atrial fibrillation. At the time of his bleed, the patient on endoscopy was diagnosed with a duodenal ulcer and Helicobacter pylori. The patient has been free of any gastrointestinal symptoms since his bleed 11 months ago and he received appropriate antibiotic and acid suppression therapy. Within 2 weeks of the patient’s diagnosis of atrial fibrillation he underwent a transesophageal echocardiogram in his former city of residence and this demonstrated normal valvular and left ventricular function and a left atrium measurement of 6.5 cm without evidence of thrombus. An attempt at cardioversion was unsuccessful. The patient is very worried about having a stroke as his wife was left dependent on him for 2 years prior to her death, following a major stroke. The referring physician, who recently had a patient who suffered a serious gastrointestinal bleed while on warfarin, is very concerned about the risk of bleeding given this patient’s age and history of gastrointestinal bleeding.
Model for evidence-based clinical decisions
Figure 1.1 depicts a model for evidence-based clinical decisions,8 which has more recently been redefined as “the integration of best research evidence with clinical expertise and patient values.”11 This model represents a desirable approach to how clinical decisions should be made. However, we acknowledge that, at present, many clinical decisions are not made this way. For instance, at present, clinicians’ individual preferences (as distinct from clinical expertise) often play a large role in their actions, leading to large “practice variations” in managing similar cases. For example, when faced with critically ill patients with identical circumstances, different clinicians may, according to their preferences, institute aggressive life-prolonging interventions or withdraw life support.12 Our model acknowledges that patients’ preferences should be considered first and foremost, rather than clinicians’ preferences, whenever it is possible and appropriate to do so (i.e. the patient wants to be involved in the decision making and they have the capacity to understand the outcomes and their consequences when explained by their physician). Although this model may look static, clinical decision making commonly requires an iterative approach whereby decisions are re -evaluated to ensure that they are still appropriate as evolving information comes to light. Integrating clinical state and circumstances, patient preferences and actions, and research evidence requires judgment and clinical expertise, thus constituting an overarching element. We will describe each of the components of the model, and the role of clinical expertise in integrating them.
Clinical state and circumstances
A patient’s clinical state and circumstances often play a dominant role in clinical decisions. Clinical trials provide us with results reflective of the average patient within the treatment groups of the trial. Rarely is a patient in clinical practice the same as the average patient from a clinical trial. Individual patients have unique characteristics that typically put them at lower or higher risk of the outcome or treatment side effect than the average patient in the trial. As such, optimal clinical decisions should be individualized to the patient’s clinical state. If a patient is at very high risk of a future vascular event but at low risk of any complication from a drug (e.g. a patient with a low-density lipoprotein value of 8.0 mmol/L post myocardial infarction and no contraindication to statin therapy), or conversely at low risk of the outcome and high risk of a treatment’s complications (e.g. a 40-year-old man with atrial fibrillation without any associated stroke risk factors who has experienced a major gastrointestinal bleed 2 weeks ago), the clinical state of the patient may dominate the clinical decision-making process.
It is notable that the circles of clinical state and circumstances and research evidence overlap. Frequently research evidence can inform us about the influence of the clinical state and circumstances. Considering our patient, the pooled data from five randomized controlled trials (RCTs) evaluating the efficacy of warfarin in patients with non-valvular atrial fibrillation (NVAF) demonstrated an average annual stroke rate of 4.5% and major bleeding rate of 1% in patients not receiving antithrombotic therapy.13 The investigators who combined the five RCTs used the control patient data to develop a clinical prediction tool to estimate the annual risk of stroke. Independent risk factors that predicted stroke in control patients were increasing age, a history of hypertension, diabetes, and prior stroke or transient ischemic attack (TIA).13 These trials likely exclude patients with a history of bleeding 11 months prior to enrollment and as a result we would not want to rely upon these trials to estimate our patient’s risk of severe bleeding; however, we believe, and research suggests,14 that the estimate of stroke from the clinical prediction tool (which takes into account age) is relevant to our patient even though he is older than the average patient in the trials. Using this model, our patient’s annual risk of stroke is predicted to be about 8%, which is higher than that of the average control patient in the five RCTs whose annual stroke rate was 4.5%.13
A clinical prediction tool has also been developed for predicting the risk of major bleeding (defined as the loss of 2 units of blood within 7 days or life-threatening bleeding) while taking warfarin therapy.15 Independent risk factors that predict major bleeding in patients taking warfarin include age > 65, history of stroke, history of gastrointestinal bleeding, recent myocardial infarction, anemia, renal failure, and diabetes (note that many of the factors that predict a higher risk of stroke also increase the risk of bleeding). Our patient’s annual risk of major bleeding of 8% also differs from that of the average patient receiving warfarin in the five RCTs whose annual risk of major bleeding was 1.3%. We are unaware of any clinical prediction tool for predicting major bleeding while taking aspirin and the atrial fibrillation trials had inadequate power to estimate this risk. The results of the meta-analysis by the antithrombotic trialists’ collaboration suggest that aspirin increases the risk of major bleeding from 1% to at least 1.3%.16 This likely is an underestimation in our patient who is older than the average patient who participated in the atrial fibrillation trials, and in this setting of suboptimal information we estimate our patient’s annual risk of major bleeding is approximately 2% with aspirin therapy.
The clinical circumstances we find ourselves in (e.g. our ability to administer and monitor a treatment) may be very different from that of an RCT. For example, the patient may not be able to obtain frequent tests of the intensity of anticoagulation. However, for a patient with the same clinical characteristics, we can frequently optimize clinical circumstances to decrease the risk of an outcome or treatment side effect. For example, we can decrease the risk of bleeding due to warfarin therapy by more intensive monitoring to ensure that the international normalized ratio (INR) is maintained in the range of 2–3. Thus, an “evidence-based” decision about anticoagulation for a patient with atrial fibrillation is not only determined by the demonstrated efficacy of anticoagulation and its potential adverse effects,17 but will vary based on the patient’s clinical state and according to individual clinical circumstances.
Patients’ preferences and actions
Patients may have no views or, alternatively, unshakable views on their treatment options, depending on their condition, personal values and experiences, degree of aversion to risk, healthcare insurance and resources, family, willingness to take medicines, accurate or misleading information at hand, and so on.8 Accordingly, individuals with very similar clinical states and circumstances may choose very different courses of action despite being presented with the same information about the benefits, risks, inconveniences, and costs of an intervention.
For our patient with NVAF, research evidence informs us about the differing preferences of patients and their physicians for antithrombotic therapy in atrial fibrillation when they weigh the competing risks of stroke and bleeding.18 In this study,18 participants (i.e. both physicians and patients) reviewed flipcharts describing in detail the acute and long-term consequences of a major and minor stroke and a major bleeding event. Participants were instructed that the likelihood of a minor or major stroke was equal. The participants then underwent a probability trade-off technique which determined the minimum number of strokes participants needed to be prevented before they felt antithrombotic therapy was justified (this value was determined for both warfarin and aspirin), given the associated increased risk of bleeding, costs and inconveniences. The same technique was also used to determine the maximum number of excess bleeds the participant considered acceptable with antithrombotic therapy (determined both for warfarin and aspirin), given the benefits in terms of stroke reduction with this therapy. This study demonstrates significant variability between physicians and patients in their weighing of the potential outcomes associated with atrial fibrillation and its treatment. Patients required less stroke reduction and were more tolerant of the risk of bleeding than physicians. For example, on average, patients were willing to accept the risk of 17 extra major bleeding events in 100 patients over a 2-year period if warfarin prevented eight strokes among these 100 patients. Physicians, however, were only willing to accept 10 major bleeding events for the same level of benefit. Furthermore, physicians varied significantly among themselves in how much bleeding risk they thought was acceptable for a given stroke reduction associated with an antithrombotic agent. Hence different physicians would make very different recommendations to the same patient with identical risks of bleeding and stroke. This underscores the importance of having patient values and preferences drive clinical decision making. It is the patient who is at risk of the outcome and hence, when willing and able, they should be the one to weigh the potential benefits versus the risks, costs, and inconveniences.
There is debate regarding the optimal way to elicit and incorporate patient preferences into clinical decision making. One method is to discuss the potential benefits and risks with a patient and then qualitatively incorporate your impression of the patient’s preferences into the clinical decision. Alternatively, at least two quantitative approaches exist: decision analytic modeling and probability trade-off technique. In a decision analytic model, a standard gamble, time trade-off or visual analog scale technique is used to determine the utility (patient value/preference) for the various outcomes. This information is then fed into a decision tree that includes the probabilities of the outcomes for all clinical decisions being considered. Using the decision tree, calculations are undertaken to determine what course of action optimally fits the patient’s preferences. Probability trade-off technique presents patients with the probabilities for the various interventions being considered and then asks them to make a decision based on this information. This allows a direct and quantitative incorporation of the patient’s preferences. The only study we are aware of that has directly compared these two quantitative approaches demonstrated that over twice as many patients stated they would base their preferences on the results of the probability trade-off as opposed to the decision analysis.19
Regardless of what their preferences may be, patients’ actions may differ from both their preferences and their clinicians’ advice.20 For example, a patient may prefer to lose weight, quit smoking and take their medications as prescribed, but their actions may fall short of achieving any of these objectives. Alternatively, they may follow the treatment as prescribed, even if they resent its imposition, adverse effects, and costs. Unfortunately, clinicians’ estimates of their patients’ adherence to prescribed treatments have no better than chance accuracy.21 Thus, physicians’ decisions for care will better meet the model’s specifications if they are able to assess whether their patients will follow, or are following, the agreed-upon decision.21
We recognize that at present formal incorporation of patients’ preferences is rarely done in clinical practice. This may be related to lack of training of physicians in these approaches, a reluctance to tread unfamiliar ground, and also in many circumstances the lack of accurate quantitative information on risk and benefits as well as clinical risk prediction tools. However, this is likely to change as clinical models can be derived from large databases and hand-held computers can be utilized to quantitate risks and benefits at the bedside.
Research evidence
We support a very broad definition of research evidence, namely “any empirical observation about the apparent relation between events.”22
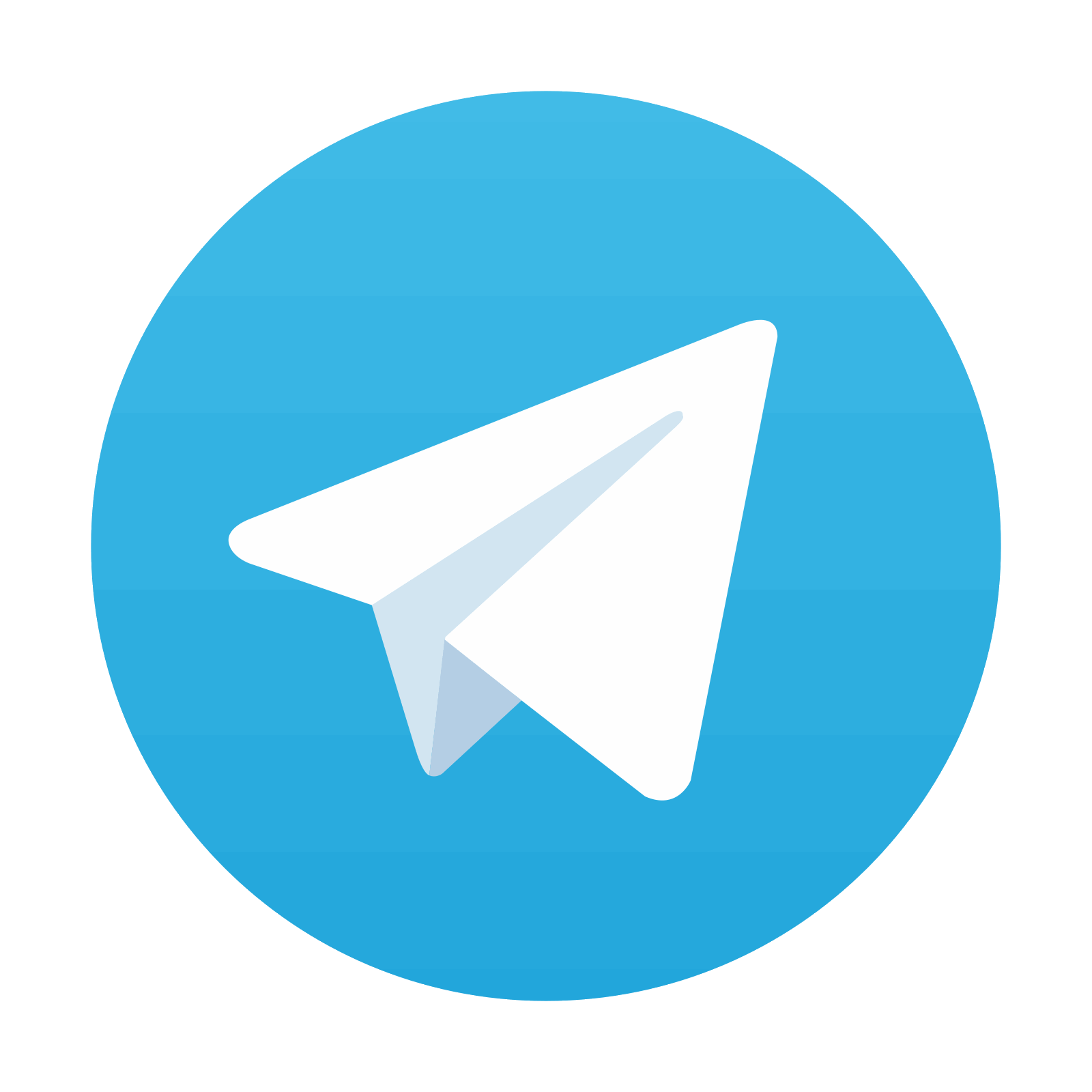
Stay updated, free articles. Join our Telegram channel

Full access? Get Clinical Tree
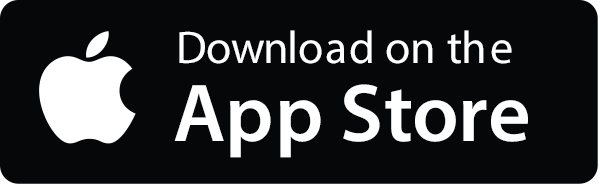
