Database
Ashok N. Babu
A. Laurie Shroyer
Frederick L. Grover
Quality improvement endeavors within the field of cardiac surgical care have made substantial progress over the last 40 years. A key aspect that has promoted improvement in the processes, structures, and outcomes in cardiac surgical care is the development of multi-institutional databases to monitor clinical risk-adjusted outcomes following cardiothoracic surgery. Quality improvement database reports offer several inherent advantages to a cardiothoracic surgeon in clinical practice. Using statistical prediction models, reports generated from complete and high-quality databases offer a unique assessment of the interactive effects of multiple risk factors on outcome.
The majority of these cardiac surgery database initiatives began by focusing reports upon relatively crude measures of unadjusted operative (30 days) mortality as their primary outcome criteria. Since the mid-1990s, there has been a progressive increase in the level of sophistication in the databases. For example, risk models can be constructed from a list of preoperative variables, and these models can be used to predict the likelihood of adverse outcomes. Whereas database outcomes initially focused on mortality, more recent efforts expand the focus for risk-adjusted outcomes to also include major morbidity as well as risk-adjusted efficiency outcomes such as length of stay and cost. Databases offer the opportunity for both feedback and information exchange; facilitating the comparison of institutions, providing data to local clinical care teams to self-assess performance, and coordinating future improvement strategies. This exchange offers, in turn, the potential to further improve the processes, structures, and outcomes of care in cardiac surgery.
HISTORICAL CONSIDERATIONS
Local databases have been used by many institutions for more than 40 years. For example, hospital-based databases have been used by Duke University, the Cleveland Clinic, the Mayo Clinic, and others to monitor volume and mortality statistics within their institutions. The first large, multi-institutional monitoring of cardiac surgery outcomes began in the Department of Veterans Affairs (VA) with the establishment of the Cardiac Surgery Consultants Committee (CSCC) in 1972. Under a congressional mandate, the VA semiannual reports were combined with ongoing surveillance work toward the goal of assuring the quality of cardiac surgical care in the VA system. Until 1988, the CSCC used unadjusted mortality and volume as the main parameters to judge outcomes. Takaro and colleagues analyzed the VA experience from 1975 to 1984 and noted that the annual volume of cardiopulmonary bypass surgery rose from 3,000 to more than 6,400 procedures. Correspondingly, the observed mortality rate for coronary artery bypass procedures dropped from 4.7% to 3.6%. They noted that patient-related comorbidities accounted for most of the operative mortality, but advances in surgical and medical techniques also may have played an important role in this mortality rate reduction.
On March 12, 1986, the Department of Health and Human Services Health Care Financing Administration (HCFA) released to the public raw mortality data for coronary artery bypass grafting (CABG). This report actually listed hospitals that had mortality rates for Medicare patients undergoing CABG that were in excess of predicted mortality rates. Although these data were to be used originally for state peer-review organizations as a quality improvement tool, HCFA was forced to release these data through the Freedom of Information Act. These mortality rates were unfortunately not risk adjusted for clinical patient risk factors. In addition, diagnostic categories were grouped together without comprehensive clinical patient-specific risk adjustments related to severity of disease and comorbidities. Furthermore, the public release of these data prompted widespread concern that nonrisk-adjusted mortality could not offer an accurate reflection of the caseload mix, and relative outcomes within an individual program. Without appropriate patient-specific clinical risk stratification, accurate comparison of cardiac surgery outcomes between programs was widely perceived not to be useful for patient care, program management, or policy decisions. This action by HCFA served as a strong stimulus for the Society of Thoracic Surgeons (STS) to initiate its own cardiac surgical database with risk-adjusted methods.
In 1987, the VA Cardiac Surgery Advisory Group, then called the VA Cardiac Surgery Consultants Committee, was also concerned with the use of unadjusted operative mortality and volume as major quality indicators for the approximately 45 VA cardiac surgery programs. Using the logistic regression method approach developed by the Collaborative Study in Coronary Artery Surgery, Drs. Hammermeister and Grover developed a multivariable risk model using clinical and angiographic predictors of 30-day operative mortality separately for coronary artery bypass procedures and valve plus other operations. The method included capturing data on all cardiac surgery procedures performed using a single-page data sheet (originally of 52 data elements) including patient-specific clinical risk factors, cardiac catheterization assessment data, operative details, and a set of both mortality and major morbidity outcome variables. These initial efforts proved successful in the attempt to “level the playing field” by accounting for the individual patient risk factors that could play a role in determining outcomes after cardiac surgical procedures (according to the first VA report). Based on these early events, two large national databases, the STS National Adult Cardiac Surgery Database and the VA Cardiac Surgery Database, emerged in a complementary and synergistic manner to provide routine reports of cardiac surgical practices for their use in their own local quality improvement endeavors. Similarly,
large regional databases also evolved, including (but not limited to) the Northern New England Cardiovascular Study Group (NNE), the New York State databases, and more recently state databases in California, Massachusetts, and Pennsylvania.
large regional databases also evolved, including (but not limited to) the Northern New England Cardiovascular Study Group (NNE), the New York State databases, and more recently state databases in California, Massachusetts, and Pennsylvania.
DATABASE CONSTRUCTION
The factors that may influence the differences in outcomes include variations in patient risk factors, in the processes and/or structures for the clinical care provided, as well as random chance. Conceptually, there are different categories of patient risk characteristics that may influence cardiac surgical outcomes including patient demographic factors, socioeconomic factors, severity of cardiac disease, comorbidities, and patient lifestyle/health behaviors. As a general rule, patient risk factors should be assessed as close to the starting time frame for the cardiac surgical procedure as possible to most accurately and reliably evaluate the patient’s inherent risk. Using statistical modeling approaches, risk stratification for a given procedure holds constant the patient-specific characteristics that may influence the likelihood of specific patient outcomes. Risk-adjusted outcomes provide indirect measures to begin exploring the potential differences for quality of care.
Virtually all of the national, regional, and local cardiac surgical databases provide predictive risk stratification—that is, an estimation for a patient with a prespecified risk profile what is the risk of having an adverse cardiac surgical event occur. Surgeons must, however, be aware of the limitations and factors pertaining to the design, construction, and management of a particular database if it is to be optimally utilized. Of primary importance are the completeness, accuracy, reliability, timeliness, sensitivity to change, and integrity of the data entered. “Garbage in = garbage out” is operative in considering any analysis of a database. Ideally, all patients receiving a cardiac surgical procedure should be entered for analysis and the adverse outcomes (such as 30-day operative mortality) appropriately validated. For any given patient record, the data submitted must also be internally consistent. For example, patients entered into the database as needing emergent operations should have firm clinical data supporting the need for such a classification. Both intrafield and interfield edit checks may be used to evaluate data quality discrepancies. Finally, standardized definitions (with training for the data capture team) are important for all data elements. Common inconsistencies in application of definitions have been demonstrated for patient-related outcomes, such as perioperative morbidities, where the use of different diagnostic test assessments is not consistently performed across all settings. Surgeons should be aware of the important aspects of the management/operation of any given database, including the following:
Which outcomes are examined, and are definitions for the measured variables standardized? Mortality, for example, is the most widely used outcome because of its importance and it is readily available from hospital records. Such a dichotomous variable would seem relatively standard. However, some databases measure in-hospital mortality, whereas others track 30-day mortality after CABG (including both in-hospital deaths and deaths that occur within 30 days postsurgery). Other important outcome variables to monitor commonly include the occurrence of major complications (infection, stroke, prolonged ventilation, renal failure, etc.), cost, and length of hospital stay. These are more difficult to measure because complications may not be uniformly recorded across sites. Standardized definitions for these major morbid events are rigorously defined by both the STS and VA cardiac databases.
What is the intent of the data analysis? The database may be designed to provide a predicted outcome for a given patient or to compare surgeon-specific or program-specific outcomes. In addition, outcomes analysis may be used to drive national policy decisions or managed care accreditation. Discussions have been initiated also about using risk-adjusted outcomes for physician profiling and credentialing.
How are the data collected? Input for databases may be gathered in a retrospective, concurrent, or prospective manner. Depending on the database, data acquisition may be mandatory (VA database) or voluntary (STS database). Data acquisition and entry may be collected by members of the patient care team or by independent data collectors. For example, in the VA database, data are collected by nurse investigators who are independent of the surgical team. It is believed that independent collection of such data may result in more thorough and unbiased reporting (e.g., self-reporting for perioperative complications may not be consistently assessed and/or uniformly recorded across surgeons).
How are the missing data treated? Any large database will have incomplete data elements. Missing data happens more commonly in databases where the data are gathered and/or entered retrospectively. If the outcome of interest is missing, then the records with missing outcome data must be discarded because no reasonable method exists that is well accepted to impute outcomes. For all other nonoutcome variables, a variety of techniques may be used to estimate the missing data. Occasionally, there are enough missing data elements within any specific record to justify dropping this specific case. Common imputation techniques derive a value to be inserted for the missing variable based on values for other data fields for that same patient, in context of the data gathered for other patients. As the completeness rates for cardiac surgery databases have increased, however, the need for complex imputation techniques has begun to diminish.
How did the predictive model perform that was generated from the database? Construction of a predictive model typically involves several steps. First, a conceptual model is generated that identifies the clinically relevant variables (e.g., risk factors) that are thought to be associated with a particular outcome (e.g., mortality). In context of the previous literature published within the field, these clinical models are constructed by a panel of experts. After these risk factors have been identified, rigorous definitions must be used to standardize the data collection. After all database completeness, accuracy, and reliability verifications (including any required data record updates), both univariate and multivariate analyses may be performed for a procedure, for a selected population of patients, and for prespecified outcomes to determine which of these risk factors are statistically associated with adverse patient outcomes. As part of the univariate screening, the outcome rates for patients with a given risk factor are compared with the outcome rates for patients without the risk factor. The number of patients required for such an analysis varies based on the nature and frequency of the outcome studied, the number of patient risk variables planned for evaluation, and the statistical approach planned. Based on the rule that in logistic regression analysis, there should be no more than 1 risk factor assessed per 10 to 15 adverse
events, if ˜10 risk variables are used, >5,000 cases are required to develop a logistic regression model for CABG-only procedure, assuming an average mortality rate of <3%.
After univariate screening analyses have been performed, a multivariable predictive model is next generated. In the majority of the cardiac surgical literature, the endpoints analyzed for risk models have included risk-adjusted mortality or presence/absence of major perioperative morbidity. For any dichotomous modeling endpoint, the analytical technique originally used by the STS to generate a predictive equation was initially based on Bayesian theory to address the inherent challenges in a voluntary database related to missing data. However, the STS switched to multivariate logistic regression in 1997 when the data record completeness and quality improved such that the performance of the logistic regression approach was determined to be more robust. For dichotomous endpoints (e.g., 30-day operative mortality), the Northern New England Cardiovascular Consortium, VA, New York State, California State, and Massachusetts State databases have also used multivariate logistic regression as the most common risk modeling approach.
Multivariate logistic regression is a statistical technique that can be used for either explanation (to understand associations between risk factors and outcomes) or prediction (to estimate risk for new and different populations). To apply the logistic regression equation for prediction purposes, risk factors are normally entered into the mathematical equation for a given patient. Mathematically, application of the logistic regression equation to a specific patient’s data captured can yield the statistical probability for the adverse outcome of interest.
Before being actively used for quality assessment activities, the performance for any statistical modeling approach(es) employed should be thoroughly evaluated. For logistic regression models, the “predictive power” (or c-index) of the model describes the concordance of the outcome predictions in comparison to the actual outcomes observed. The c-index describes the area under the receiver-operating characteristic curve related to the true-positive rate (sensitivity) and the false-positive rate (1—specificity). For example, a c-statistic of 0.5 would indicate that the risk model has no better chance than flipping a coin of identifying “at risk” patients for adverse outcome events. Ideally, a predictive risk model will have a c-index of >0.70, meaning that on average 70% of the time that the outcome predicted by the model is accurate, leaving the remaining 30% subject to the unmeasured risk factors, the nature of the processes and structures of care rendered, as well as random chance.
Statistical risk models are best for predictions performed on large volumes of patient records, where the confidence intervals around the predictions may be relatively small, as compared with predictions made for a single patient where the confidence interval around the point estimate for risk prediction may be large. Given that it is virtually impossible for a single risk model to incorporate comprehensively all of the risk factors that are relevant to all patients, there commonly may be unmeasured risk variables (specifically, rare patient risk factors) that may predispose a given patient to an adverse event. The final predictive variables included in a logistic regression model, therefore, are generally those factors that have the largest impact and occur reasonably frequently. For example, a patient may have had chest radiation in the past, which can increase their risk of adverse perioperative outcomes. As chest radiation occurs infrequently, however, this risk factor may not be commonly placed upon the list of prediction variables that determine estimated operative morbidity and mortality. For this reason, statistical risk models may either underpredict or overpredict the risk for an individual patient, so that physicians need to take this into account when diagnosing unusual patient risk factors when deciding on appropriate therapy and discussing this with a given patient.
For logistic regression models, another key performance metric to be evaluated is the model’s calibration. Model calibration describes how well the model performs over the full range of risk estimates, as compared with the observed outcome rates. Models may work well for high-risk or for low-risk situations but not uniformly work well across all ranges of risk. A common metric used to assess logistic regression model performance related to calibration is the Hosmer-Lemeshow [H-L] test for “goodness of fit.” The H-L test is adapted from the Chi-square test, evaluating to what degree there is “no difference” in the predicted versus observed outcome rates across ordered risk categories (such as ordered deciles of risk). To be able to state that there is not a calibration challenge, therefore, the H-L test statistic’s P-value should be ideally >0.05—stating that there was no difference in calibration across the risk categories that were observed.
In addition to multivariable logistic regression for single endpoints, more complex regression approaches for multiple endpoints have recently been implemented by the STS. One notable example is the development of a comprehensive composite metric that assesses multiple aspects of quality rather than focusing only on risk-adjusted mortality. This acknowledges the fact that not all patients who survive CABG surgery have had equivalent quality procedures. The STS Database team and its Quality Measurement Task Force under the guidance of Dr. Shahian has developed a meaningful, NQF endorsed CABG Composite Score to assess the overall quality of care rendered. The results of this methodology are presented as both numerical scores and, for ease of consumer interpretation, as a “star” rating system. In the latter, programs whose results are estimated to have at least a 99% Bayesian probability of being superior to the STS average are awarded three stars (about 10% to 15% of all STS participant programs), whereas those with a similar probability of being worse than the STS average (again, about 10% to 15%) are awarded one star. The remaining or average quality programs are classified as two stars.
To calculate the STS CABG Composite Score for each hospital, the following four metrics were combined:
freedom from 30-day operative mortality (both any in-hospital death regardless of timing and any death within 30 days regardless of venue; risk-adjusted);
freedom from major morbidity (defined as the risk-adjusted occurrence of any one or more of the following endpoints: renal failure, reoperation, stroke, sternal wound infection, prolonged ventilation);
use of at least one internal thoracic arterial bypass graft conduit (considered as a “best surgical practice”); and
use of all recommended perioperative medications (preoperative beta blockade; discharge antiplatelet drugs, lipidlowering agents, and beta blockade) (an “all-or-none” endpoint; considered as a “best medical practice”).
The above metrics were analyzed together in a hierarchical model in order to estimate success rates for each of the four metrics by a participant. A participant’s final composite score was obtained by averaging their four success rates. Albeit more complex analytically, this innovative
quality metric is multidimensional (evaluating simultaneously mortality, morbidity, and reflects compliance with what is considered the optimal CABG surgical and ischemic heart disease medical therapy practice) and fully risk adjusted. This intellectually elegant and clinically relevant composite metric provides an assessment of the quality metrics that hospitals can control (specifically their hospital’s compliance with best practices) while adjusting for the factors that hospitals cannot control (specifically, the risk characteristics of their patient population).
quality metric is multidimensional (evaluating simultaneously mortality, morbidity, and reflects compliance with what is considered the optimal CABG surgical and ischemic heart disease medical therapy practice) and fully risk adjusted. This intellectually elegant and clinically relevant composite metric provides an assessment of the quality metrics that hospitals can control (specifically their hospital’s compliance with best practices) while adjusting for the factors that hospitals cannot control (specifically, the risk characteristics of their patient population).
CURRENT ADULT CARDIAC SURGERY DATABASES
New York State Database
In response to the HCFA release of cardiac surgery data and as much as fivefold variations in mortality between hospitals, New York State organized a Cardiac Surgery Advisory Committee, which was charged with investigating issues related to quality assurance for cardiovascular disease. A clinical registry for cardiac surgery began collecting data in 1989 and one for percutaneous coronary intervention (PCI) began in 1992. This group developed a patient-specific cardiac surgical report form. This form reported demographic data, surgical procedures, admission and discharge dates, and information on risk factors, complications, and discharge status. Data forms are completed at each surgical program and forwarded to the Department of Health for analysis. Data reporting is mandatory.
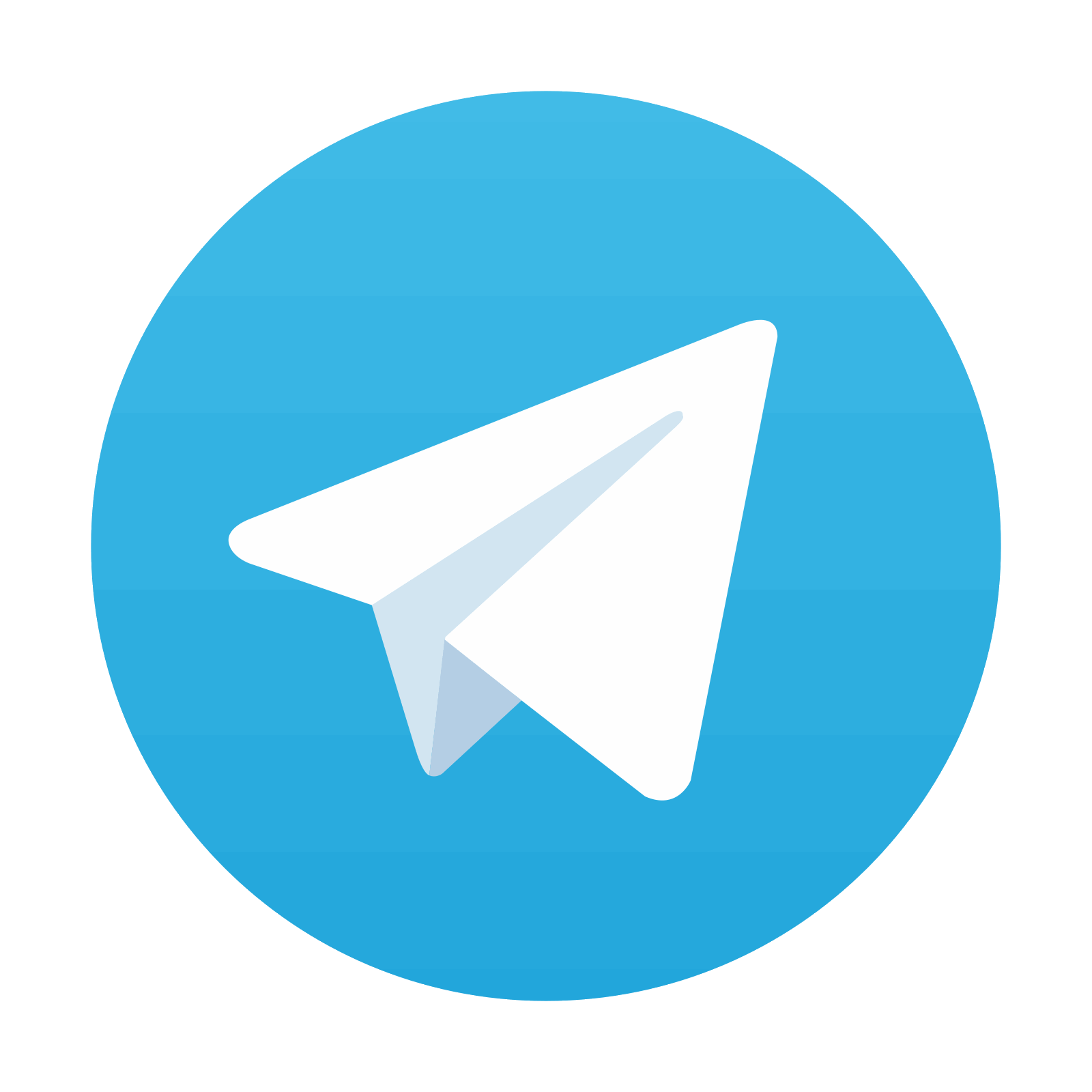
Stay updated, free articles. Join our Telegram channel

Full access? Get Clinical Tree
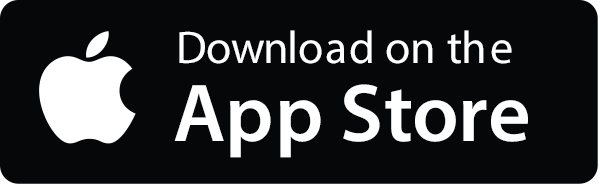
