4
Seizure Signal Analysis
An original impression and the fundamental concepts of epilepsy were refined and developed in ancient Indian medicine during the Vedic period of 4500–1500BC. In the Ayurvedic literature of Charaka Samhita (around 400BC, and the oldest existing description of the complete Ayurvedic medical system), epilepsy is described as ‘apasmara’, which means ‘loss of consciousness’. The word ‘epilepsy’ is derived from the Greek word epilambanein, which means ‘to seize or attack’. It is now known, however, that seizures are the result of sudden, usually brief, excessive electrical discharges in a group of brain cells (neurons) and that different parts of the brain can be the site of such discharges. The clinical manifestations of seizures therefore vary and depend on where in the brain the disturbance first starts and how far it spreads. Transient symptoms can occur, such as loss of awareness or consciousness and disturbances of movement, sensation (including vision, hearing, and taste), mood, or mental function.
The literature of Charaka Samhita contains abundant references to all aspects of epilepsy including symptomatology, etiology, diagnosis, and treatment. Another ancient and detailed account of epilepsy is on a Babylonian tablet in the British Museum in London. This is a chapter from a Babylonian textbook of medicine comprising 40 tablets dating as far back as 2000BC. The tablet accurately records many of the different seizure types recognized today. In contrast to the Ayurvedic medicine of Charaka Samhita, however, it emphasizes the supernatural nature of epilepsy, with each seizure type associated with the name of a spirit or god – usually evil. Treatment was, therefore, largely a spiritual matter. The Babylonian view was the forerunner of the Greek concept of ‘the sacred disease’, as described in the famous treatise by Hippocrates (dated to the 5th century BC). The term ‘seleniazetai’ was also often used to describe people with epilepsy because they were thought to be affected by the phases of the moon or by the moon god (Selene), and hence the notion of ‘moonstruck’ or ‘lunatic’ (the Latinized version) arose. Hippocrates, however, believed that epilepsy was not sacred, but a disorder of the brain. He recommended physical treatments and stated that if the disease became chronic, it was incurable. However, the perception that epilepsy was a brain disorder did not begin to take root until the 18th and 19th centuries AD. The intervening 2000 years were dominated by more supernatural views. In Europe, for example, St. Valentine has been the patron saint of people with epilepsy since mediaeval times. During this time people with epilepsy were viewed with fear, suspicion, and misunderstanding and were subjected to enormous social stigma. People with epilepsy were treated as outcasts and punished. Some, however, succeeded and became famous the world over. Among these people were Julius Caesar, Czar Peter the Great of Russia, Pope Pius IX, the writer Fedor Dostoevsky, and the poet Lord Byron.
During the 19th century, as neurology emerged as a new discipline distinct from psychiatry, the concept of epilepsy as a brain disorder became more widely accepted, especially in Europe and the United States of America (USA). This helped to reduce the stigma associated with the disorder. Bromide, introduced in 1857 as the world’s first effective antiepileptic drug, became widely used in Europe and the USA during the second half of the last century. The first hospital centre for the ‘paralysed and epileptic’ was established in London in 1857. At the same time a more humanitarian approach to the social problems of epilepsy resulted in the establishment of epilepsy ‘colonies’ for care and employment.
The new understanding of epilepsy (pathophysiology) was also established in the 19th century with the work of neurologist Hughlings Jackson in 1873, who proposed that seizures were the result of sudden brief electrochemical discharges in the brain. He also suggested that the character of the seizures depended on the location and function of the site of the discharges. Soon afterwards the electrical excitability of the brain in animals and man was discovered by David Ferrier in London and Gustav Theodor Fritsch and Eduard Hitzig in Germany.
Hans Berger, a psychiatrist, developed the human EEG. Its important application from the 1930s onwards was in the field of epilepsy. The EEG revealed the presence of electrical discharges in the brain. It also showed different patterns of brainwave discharges associated with different seizure types. The EEG also helped to locate the site of seizure discharges and expanded the possibilities of neurosurgical treatments, which became much more widely available from the 1950s onwards in London, Montreal, and Paris.
During the first half of the 20th century the main drugs for treatment were phenobarbitone (first used in 1912) and phenytoin (first used in 1938). Since the 1960s, there has been an accelerating process of drug discovery, based in part on a much greater understanding of the electrochemical activities of the brain, especially the excitatory and inhibitory neurotransmitters. In developed countries in recent years, several new drugs have come into the market and seizures can now be controlled in 70–80% of newly diagnosed children and adults.
Neuroimaging techniques such as fMRI and position emission tomography (PET) boost the success in diagnosis of epilepsy. Such technology has revealed many of the more subtle brain lesions responsible for epilepsy. Several brain lesions such as trauma, congenital, developmental, infection, vascular, and tumour might lead to epilepsy in some people. Of the 50 million people in the world with epilepsy, some 35 million have no access to appropriate treatment. This is either because services are nonexistent or because epilepsy is not viewed as a medical problem or a treatable brain disorder. As a major campaign for the treatment of epilepsy, the International League Against Epilepsy (ILAE) and the International Bureau for Epilepsy (IBE) joined forces with the World Health Organization in 1997 to establish the Global Campaign Against Epilepsy to address these issues.
Epilepsy is a sudden and recurrent brain malfunction and is a disease that reflects an excessive and hypersynchronous activity of the neurons within the brain. It is probably the most prevalent brain disorder among adults and children, second only to stroke. Over 50 million people worldwide are diagnosed with epilepsy, whose hallmark is recurrent seizures [1]. The prevalence of epileptic seizures changes from one geographic area to another [2].
The seizures occur at random to impair the normal function of the brain. Epilepsy can be treated in many cases and the most important treatment today is pharmacological. The patient takes anticonvulsant drugs on a daily basis, trying to achieve a steady-state concentration in the blood, which are chosen to provide the most effective seizure control. Surgical intervention is an alternative for carefully selected cases that are refractory to medical therapy. However, in almost 25% of the total number of patients diagnosed with epilepsy, seizures cannot be controlled by any available therapy. Furthermore, side effects from both pharmacological and surgical treatments have been reported.
An epileptic seizure can be characterized by paroxysmal occurrence of synchronous oscillations. Such seizures can be classified into two main categories depending on the extent of involvement of various brain regions: focal (or partial) and generalized. Generalized seizures involve most areas of the brain whereas focal seizures originate from a circumscribed region of the brain, often called epileptic foci [3]. Figure 4.1 shows two segments of EEG signals involving generalized and focal seizures respectively.
Successful surgical treatment of focal epilepsies requires exact localization of the epileptic focus and its delineation from functionally relevant areas [4]. The physiological aspects of seizure generation and the treatment and monitoring of a seizure, including presurgical examinations, have been well established [3] and medical literature provided.
EEG, MEG, and recently fMRI are the major neuroimaging modalities used for seizure detection. The blood-oxygenation-level-dependent (BOLD) regions in fMRI of the head clearly show the epileptic foci. However, the number of fMRI machines is limited in each area, they are costly, and a full body scan is time consuming. Therefore, using fMRI for all patients at all times is not feasible. MEG, on the other hand, is noisy and since the patient under care has to be steady during the recording, it is hard to achieve clear data for moderate and severe cases using current MEG machines.
Therefore, EEG remains the most useful and cost effective modality for the study of epilepsy. Although for generalized seizure the duration of seizure can be easily detected using a naked eye, for most focal epilepsies such intervals are difficult to recognize.
From the pathological point of view, there are clear classification schemes for seizures. ‘Partial’ is used to describe isolated phenomena that reflect focal cortical activity, either evident clinically or by EEG. The term ‘simple’ indicates that consciousness is not impaired. For example, a seizure visible as a momentarily twitching upper extremity, which subsides, would be termed a simple partial seizure with motor activity. Partial seizures may have motor, somatosensory, psychic, or autonomic symptoms [5].
The term ‘complex’ defines an alteration of consciousness associated with the seizure. ‘Generalization’ is a term used to denote the spread from a focal area of the cortex, which could be evident clinically by EEG, and involves all areas of the cortex with resulting generalized motor convulsion. It is known that in adults the most common seizure type is that of initial activation of one area of the cortex with subsequent spread to all areas of the cortex; frequently this occurs too quickly to be appreciated by bedside observation.
The other major grouping of seizure types is for generalized seizures, which may be termed convulsive or nonconvulsive. For this type, all areas of the cortex are activated at once. This, for example, is seen with absence seizures and myoclonic seizures [6].
Figure 4.1 Two segments of EEG signals each from a patient suffering (a) generalized seizure and (b) focal seizure onset
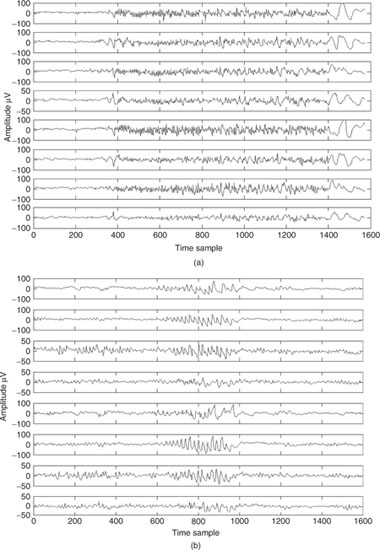
Tonic-clonic (grand mal) seizures are more common and because of that other types of seizures may escape detection. They were diagnosed for the first time in 1827 by Bravais [7]. The recurrent behaviour of the spike waveforms in the EEG signals triggers investigation for a possible seizure disorder. Seizures of frontal or temporal cortical origin with nonclassical motor movements are fairly commonly encountered. The patient may show some seemingly organized motor activity without the usually in-phase jerking movements more typical of generalized seizures. Also complicating the problem is that clouding or alteration of consciousness may occur without complete loss of consciousness.
The term ‘aura’ is used to represent ongoing seizure activity limited to a focal area of the cortex. In this case the abnormal electrical activity associated with the seizure does not spread or generalize to the entire cerebral cortex but remains localized and persists in one abnormal focus.
One seizure type may evolve into another seizure type. For example, a simple motor seizure may evolve into a complex partial seizure with altered consciousness; the terminology for this would be ‘partial complex status epilepticus’ [6].
Absence seizures (also known as petit mal) are a primarily generalized seizure type involving all cortical areas at once; this is typically a seizure disorder of childhood with a characteristic EEG pattern [6]. At times, absence seizures may persist with minimal motor movements and altered consciousness for hours or days. Absence epileptic seizure and complex partial epileptic seizure are often grouped under the term ‘nonconvulsive status epilepticus’ and are referred to at times as twilight or fugue states.
The term ‘subtle status epilepticus’ is more correctly used to indicate patients that have evolved from generalized convulsive epileptic seizure or are in a comatose state with epileptiform activity.
The character of an epileptic seizure is determined based on the region of the brain involved and the underlying basic epileptic condition, which are mostly age-determined. As a conclusion, clinical classification of epileptic seizures is summarized as:
- simple partial onset followed by alteration of consciousness;
- partial evolving to secondarily generalized.
Moreover, epileptic seizures may be chronic or acute. However, so far no automatic classification of these seizures based on the EEG waveforms has been reported.
Most traditional epilepsy analysis methods, based on the EEG, are focused on the detection and classification of epileptic seizures, among which the best method of analysis is still the visual inspection of the EEG by a highly skilled electroencephalographer or neurophysiologist. However, with the advent of new signal processing methodologies several computerized techniques have been proposed to detect and localize epileptic seizures. In addition, based on the mathematical theory of nonlinear dynamics [8], there has been an increased interest in the analysis of the EEG for the prediction of epileptic seizures.
Seizure detection and classification using signal processing methods has been an important issue of research for the last two decades [9–12]. Researchers have tried to highlight different signal characteristics within various domains and classify the signal segments based on the measured features. Adult seizure is more robust than neonate (newborn) seizure. Therefore, its detection, labelling, and classification is not very difficult. On the other hand, neonate seizure is more chaotic and although some methods have been suggested for the detection of such events the problem still remains open. Therefore, various automated spike detection approaches have been developed [13].
Recently, predictability of seizure from long EEG recordings has attracted many researchers. It has been shown that epileptic sources gradually tend to be less chaotic from a few minutes before the seizure onset. This finding is clinically very important since the patients do not need to be under anticonvulsant administration permanently, but from just a few minutes before seizure. In the following sections the major research topics in the areas of epileptic seizure detection and prediction are discussed.
4.1 Seizure Detection
4.1.1 Adult Seizure Detection
In clinics, for patients with medically intractable partial epilepsies, time-consuming video-EEG monitoring of spontaneous seizures is often necessary [11]. Visual analysis of interictal EEG is, however, time intensive. Application of invasive methods for monitoring the seizure signals and identification of an epileptic zone is hazardous and involves risk for the patient.
Before designing any automated seizure detection system the characteristics of the EEG signals before, during, and after the seizure have to be determined and evaluated. Several features have been identified to describe the features better. These may represent the static behaviour of the signals within a short time interval, such as signal energy, or the dynamic behaviour of the signals, such as chaoticity and the change in frequency during the seizure onset.
Automatic seizure detection quantification and recognition has been an area of interest and research within clinical, physics, and engineering communities since the 1970s [14–27]. In some early works in spike detection [16,21–23] a number of parameters such as relative amplitude, sharpness, and duration of EEG waves were measured from the EEG signals and evaluated. The method is sensitive to various artefacts. In these attempts different states such as active wakefulness or desynchronized EEG were defined, in which typical nonepileptic transients were supposed to occur [22,23]. A multistage system to detect the epileptiform activities from the EEGs was developed by Dingle et al. [18]. They combined a mimetic approach with a rule-based expert system, and thereby considered and exploited both the spatial and temporal systems. In another approach [19] multichannel EEGs were used and a correlation-based algorithm was attempted to reduce the muscle artefacts. Following this method, approximately 67% of the spikes can be detected. By incorporating both multichannel temporal and spatial information, and including the electrocardiogram, electromyogram, and electrooculogram information into a rule-based system [20], a higher detection rate was achieved. A two-stage automatic system was developed by Davey et al. [17]. In the first stage a feature extractor and in the second stage a classifier were introduced. A 70% sensitivity was claimed for this system.
Artificial neural networks (ANNs) have been used for seizure detection by many researchers [26,28]. The Kohonen self-organizing feature map ANN [29,30] was used for spike detection by Kurth et al. [28]. In this work, for each patient three differentsized neural networks (NNs) have been examined. The training vector included a number of signals with typical spikes, a number of eye-blinking artefact signals, some signals of muscle artefacts, and also background EEG signals. The major problem with these methods is that the epileptic seizure signals do not follow similar patterns. Presenting all types of seizure patterns to the ANN, on the other hand, reduces the sensitivity of the overall detection system. Therefore, a clever feature detection followed by a robust classifier often provides an improved result.
Among recent works, time–frequency (TF) approaches effectively use the fact that the seizure sources are localized in the time–frequency domain. Most of these methods are mainly for detection of neural spikes [31] of different types. Different TF methods following different classification strategies have been proposed by many researchers [32,33] in this area. The methods are especially useful since the EEG signals are statistically nonstationary. The discrete wavelet transform (DWT) obtains a better TF representation than the TF based on the short-term Fourier transform due to its multiscale (multilevel) characteristics; i.e. it can model the signal according to its coarseness. The DWT analyses the signal over different frequency bands, with different resolutions, by decomposing the signal into a coarse approximation and detail information. In a recent approach by Subasi [33], a DWT-based TF method followed by an ANN has been suggested. The ANN classifies the energy of various resolution (detail) levels. Using this technique, it is possible to detect more than 80% of adult epileptic seizures. Other TF distributions such as the pseudo-Wigner–Ville can also be used for the same purpose [34].
In an established work by Osorio et al. [35,36] a digital seizure detection algorithm has been proposed and implemented. The system is capable of accurate real-time detection, quantitative analysis, and very short-term prediction of the clinical onset of seizure. This system computes a measure, namely ‘foreground’, of the median signal energy in the frequencies between 8 and 42 Hz in a short window of specific length (e.g. two seconds). The foreground is calculated through the following steps: (1) decomposing the signals into epileptiform (containing epileptic seizures) and nonepileptiform (without any seizure) components using a 22-coefficient wavelet filter (DAUB4, level 3), which separates the frequency subbands from 8 to 40 Hz; (2) the epileptiform component is squared, and 3) the squared components are median filtered. On the other hand, a ‘background’ reference signal is obtained as an estimate of the median energy of a longer time (approximately 30 minutes) of the signal. A large ratio between the foreground and background will then show the event of seizure [37]. An analogue system was later developed to improve the technical drawbacks of the above system such as speed and noise [37].
There are many features that can be detected/measured from the EEGs for detection of epileptic seizures. Often seizures increase the average energy of the signals during the onset. For a windowed segment of the signal this can be measured as
where L is the window length and the time index n is the window centre. The seizure signals have a major cyclic component and therefore generally exhibit a dominant peak in the frequency domain. The frequency of this peak, however, decays with time during the onset of seizure. Therefore the slope of decay is a significant factor in the detection of seizure onset. Considering X(f, n), the estimated spectrum of the windowed signal x(n) centred at n, the peak at time n will be
The spectrum is commonly estimated using autoregressive modelling [38]. From the spectrum the peak frequency is measured. The slope of the decay in the peak frequency can then be measured and used as a feature. The cyclic nature of the EEG signals can also be measured and used as an indication of seizure. This can be best identified by incorporating certain higher order statistics of the data. One such indicator is related to the second- and fourth order statistics of the measurements as follows [39]:
where represents the Fourier coefficients of the fourth-order cyclic cumulant at zero lag and can be estimated as follows:
where xc(n) is the zeroed mean version of x(n), and an estimation of is calculated as
This indicator is also measured with respect to the time index n since it is calculated for each signal window centred at n. I in Equation (4.3) measures, for a frame centred at n, the spread of the energy. Over the range of frequencies before seizure onset, the EEG is chaotic and no frequency appears to control its trace. During seizure, the EEG becomes more ordered (rhythmic) and therefore the spectrum has a large peak.
The above features have been measured and classified using a support vector machine (SVM) classifier [38]. It has been illustrated that for both tonic–clonic and complex partial seizures the classification rate can be as high as 100%.
In a robust detection of seizure, however, all statistical measures from the EEGs together with all other symptoms, such as blood morphology, body movement, pain, changes in metabolism, heart rate variability, and respiration before, during, and after seizure, have to be quantified and effectively taken into account. This is a challenge for future signal processing/data fusion based approaches.
In another attempt for seizure detection a cascade of classifiers based on artificial neural networks (ANNs) has been used [40]. The classification is performed in three stages. In the first stage the following six features feed two perceptrons to classify peaks into definite epileptiform, definite nonepileptiform, and unknown waveforms. The features are selected based on the expected shape of an epileptic spike, such as the one depicted in Figure 4.2.
These features are:
Figure 4.2 An epileptiform spike with features used in the classification of epileptic and nonepileptic seizures
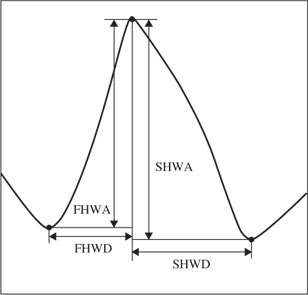
Since three outputs are needed after the first stage (also called the preclassifier) two single-layer perceptrons are used in parallel. One perceptron is trained to give +1 for definite nonepileptiform and −1 otherwise. The second network produces +1 for definite epileptiform and −1 otherwise. A segment that produces −1 at the output of both networks is assigned to the unknown group.
The update equation to find w, the vector of the weights of the perceptron ANNs, at the kth iteration can be simply presented as
where x is the input feature vector, d is the expected feature vector, y is the output, calculated as y = sign(wTx – Tr), where Tr is an empirical threshold, and µ is the learning rate set empirically or adaptively.
In the second stage the unknown waveforms (spikes) are classified using a radial-basis function (RBF) neural network. An RBF has been empirically shown to have a better performance than other ANNs for this stage of classification. The inputs are the segments of actual waveforms. The output is selected by hard-limiting the output of the last layer (after normalization).
Finally, in the third stage a multidimensional SVM with a nonlinear (RBF) kernel is used to process the data from its multichannel input. An accuracy of up to 100% has been reported for detection of generalized and most focal seizures [40].
Epilepsy is often characterized by the sudden occurrence of synchronous activity within relatively large brain neuronal networks that disturb the normal working of the brain. Therefore, some measures of dynamical change have also been used for seizure detection. These measures significantly change in the transition between the preictal and ictal states or even in the transition between the interictal and ictal states. In the latter case the transition can occur either as a continuous change in phase, such as in some cases of mesial temporal lobe epilepsy (MTLE), or as a sudden leap, e.g. in most cases of absence seizures. In the approaches based on chaos measurement by estimation of attractor dimension (as discussed later in this chapter), for the first case the attractor of the system gradually deforms from an interictal to an ictal attractor. In the second case, where a sharp critical transition takes place, it can be assumed that the system has at least two simultaneous interictal and ictal attractors all the time. In a study by Lopes da Silva et al. [41] three states (routes) have been characterized as illustrative models of epileptic seizures:
The authors concluded that under these routes it is possible to understand those circumstances where the transition from the ongoing (interictal) activity mode to the ictal (seizure) mode may, or may not, be anticipated. Also, any of the three routes is possible, depending on the type of underlying epilepsy. Seizures may be generally unpredictable, as most often in absence-type seizures of idiopathic (primary) generalized epilepsy, or predictable, preceded by a gradual change in dynamics, detectable some time before the manifestation of seizure, as in TLE.
Chaotic behaviour of the EEGs is discussed in Section 4.3 and is mainly exploited for the prediction of seizure, as a new research direction.
4.1.2 Detection of Neonate Seizure
Seizure occurs in approximately 0.5% of newborn (the first four weeks of life) babies. It represents a distinctive indicator of abnormality in the central nervous system (CNS). There are many causes for this abnormality, with the majority due to biochemical imbalances within the CNS, intracranial haemorrhage and infection, developmental (structural) defects, and passive drug addiction and withdrawal [42]. Analysis of neonatal EEG is a very difficult issue in the biomedical signal processing context. Unlike in adults, the presence of spikes may not be an indication of seizure. The clinical signs in the newborn are not always as obvious as those for an adult, where seizure is often accompanied by uncontrollable, repetitive, or jerky movements of the body, or the tonic flexion of muscles. The less obvious symptoms in the newborn, i.e. subtle seizures, may include sustained eye opening with ocular fixation, repetitive blinking or fluttering of eyelids, drooling, sucking, or other slight facial expressions or body movements. Therefore, detection of epileptic seizures for the newborn is far more complex than for adults and so far only a few approaches have been attempted. These approaches are briefly discussed in this section.
Although there is a significant spectral range for the newborn seizure signal [43] in most cases the seizure frequency band lies within the delta and theta bands (1–7 Hz). However, TF approaches are more popular due to the statistical nonstationarity of the data, but they can also be inadequate since the spikes may have less amplitude than the average amplitude of a normal EEG signal.
In most cases the signals are preprocessed before application of any seizure detection algorithm. Eye blinking and body movements are the major sources of artefacts. Conventional adaptive filtering methods (with or without a reference signal) may be implemented to remove interference [44,45]. This may be followed by the calculation of a TF representation of seizure [46] or used in a variable-time model of the epileptiform signal.
A model-based approach was proposed to model a seizure segment and the model parameters were estimated [47]. The steps are as follows:

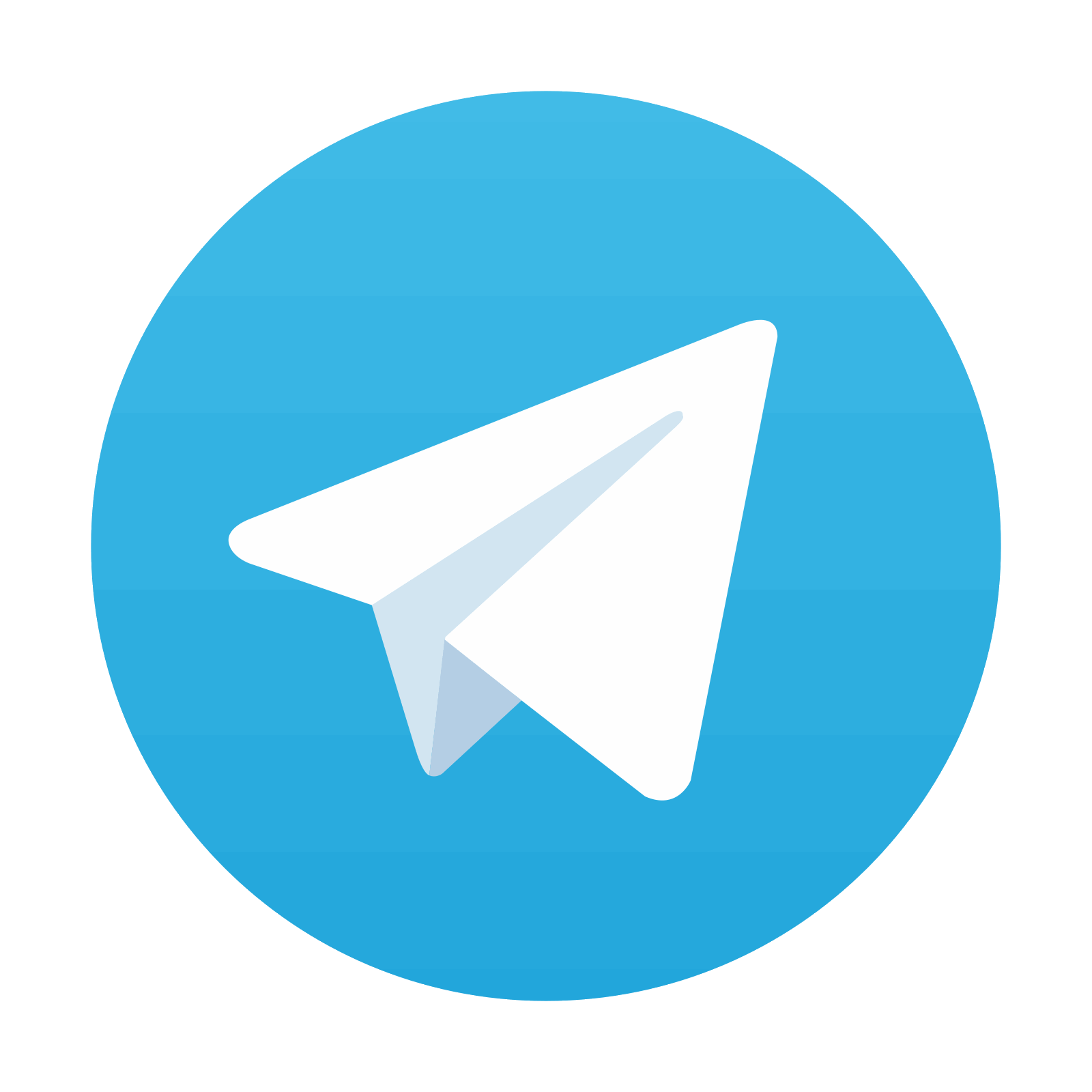
Stay updated, free articles. Join our Telegram channel

Full access? Get Clinical Tree
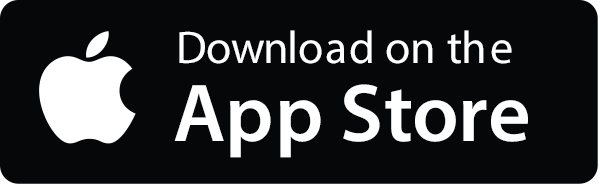
