7
Brain–Computer Interfacing
Brain–computer interfacing (BCI) (also called brain–machine interfacing (BMI)) is a challenging problem that forms part of a wider area of research, namely human–computer interfacing (HCI), which interlinks thought to action. BCI can potentially provide a link between the brain and the physical world without any physical contact. In BCI systems the user messages or commands do not depend on the normal output channels of the brain [1]. Therefore the main objectives of BCI are to manipulate the electrical signals generated by the neurons of the brain and generate the necessary signals to control some external systems. The most important application is to energize the paralysed organs or bypass the disabled parts of the human body. BCI systems may appear as the unique communication mode for people with severe neuromuscular disorders such as spinal cord injury, amyotrophic lateral sclerosis, stroke and cerebral palsy.
Approximately one hundred years after the discovery of the electrical activity of the brain the first BCI research was reported by Jacques Vidal [2,3] during the period 1973–7. In his research it was shown how brain signals could be used to build up a mental prosthesis. BCI has moved at a stunning pace since the first experimental demonstration in 1999 that ensembles of cortical neurons could directly control a robotic manipulator [4]. Since then there has been tremendous research in this area [5].
BCI addresses analysing, conceptualization, monitoring, measuring, and evaluating the complex neurophysiological behaviours detected and extracted by a set of electrodes over the scalp or from the electrodes implanted inside the brain. It is important that a BCI system be easy, effective, efficient, enjoyable to use, and user friendly. BCI is a multidisciplinary field of research since it deals with cognition, electronic sensors and circuits, machine learning, neurophysiology, psychology, sensor positioning, signal detection, signal processing, source localization, pattern recognition, clustering, and classification.
The main and prominent activities in BCI (especially noninvasive BCI) are:
In this chapter the fundamental concepts and the requirement for the BCI design using EEG signals are reviewed. Development of BCI approaches within the last two decades are given next, and finally the well-established and latest advances in BCI are discussed.
7.1 State of the Art in BCI
The correspondence between EEG patterns and computer actions constitutes a machine-learning problem since the computer should learn how to recognize a given EEG pattern. As for other learning problems, in order to solve this problem, a training phase is necessary, in which the subject is asked to perform prescribed mental activities (MAs) and a computer algorithm is in charge of extracting the associated EEG patterns. After the training phase is finished the subject should be able to control the computer actions with his or her thoughts. This is the major goal for a BCI system.
In terms of signal acquisition, the BCI systems are classified into invasive (intracranial) and noninvasive. Noninvasive systems primarily exploit EEGs to control a computer cursor or a robotic arm. The techniques in developing such systems have been under development recently due to their hazardless nature and flexibility [1,19–27]. However, despite the advantage of not exposing the patient to the risks of brain surgery, EEG-based techniques provide limited information mainly because of the existence of system and physiological noise and the interfering undesired signals and artefacts. However, despite these shortcomings, EEG-based methods can detect modulations of brain activity that correlate with visual stimuli, gaze angle, voluntary intentions, and cognitive states [5]. These advantages led to development of several classes of EEG-based systems, which differ according to the cortical areas recorded, the extracted features of the EEGs, and the sensory modality providing feedback to subjects.
In noninvasive BCI approaches, although there may be different EEG electrode settings for certain BCI applications, an efficient BCI system exploits all the information content within the EEG signals. In all cases detection and separation of the control signals from the raw EEG signals is probably the first objective. The event-related source signals can be effectively clustered or separated if the corresponding control signals are well characterized. Given that these control sources are likely to be moving inside the brain, an exciting research area is also to localize and track these sources in real-time. The first step in developing an effective BCI paradigm is therefore to determine suitable control signals from the EEG. A suitable control signal has the following attributes: it can be precisely characterized for an individual, it can be readily modulated or translated to express the intention, and it can be detected and tracked consistently and reliably.
There are two main approaches towards BCI: one is based on event related potentials (ERPs) and another is based on the multiple sensor EEG activities recorded in the course of ordinary brain activity. The latter approach is more comprehensive and does not require any particular stimulus. However, as mentioned before, in many cases such as those where there is a direct connection from the electrodes to the mechanical systems, the number of recording channels, electrodes, is generally limited. In such cases EEG channel selection across various subjects has become another popular research area within the BCI community [28]. The general idea behind these approaches is based on a recursive channel elimination (RCE) criterion; channels that are well known to be important (from a physiological point of view) are consistently selected whereas task-irrelevant channels are disregarded. Nonnegative matrix factorization (NMF) has also been used to analyse neural activity and identify local spatiotemporal patterns of neural activity in the form of sparse basis vectors [29].
An ERP appears in response to some specific stimulus. The most widely used ERP evoked potential (EP) is the P300 signal, which can be auditory, visual, or somatosensory. It has a latency of approximately 300 ms and is elicited by rare or significant stimuli, and its amplitude is strongly related to the unpredictability of the stimulus; the more unforeseeable the stimulus, the higher the amplitude [30]. Another type of visual EP (VEP) is those for which the ERPs have a short latency, representing the exogenous response of the brain to a rapid visual stimulus. They are characterized by a negative peak around 100 ms (N1) followed by a positive peak around 200 ms (P2). The ERPs can provide control when the BCI produces the appropriate stimuli. Therefore the BCI approach based on ERP detection from the scalp EEG seems to be easy since the cortical activities can be easily measured noninvasively and in real-time. Also, an ERP-based BCI needs little training for a new subject to gain control of the system. However, the information achieved through ERP extraction and measurement is not accurate enough for extraction of movement related features and they have vast variability in different subjects with various brain abnormalities and disabilities. More importantly, the subject has to wait for the relevant stimulus presentation [31,32].
There are also two other approaches used for BCI. One is based on steady-state visualevoked responses (SSVERs), which are natural responses for visual stimulations at specific frequencies. These responses are elicited by a visual stimulus that is modulated at a fixed frequency. The SSVERs are characterized by an increase in EEG activity around the stimulus frequency. With feedback training, subjects learn to voluntarily control their SSVER amplitude. Changes in the SSVER result in control actions occurring over fixed intervals of time [32,33]. The second approach is slow cortical potential shifts (SCPSs), which are shifts of cortical voltage lasting from a few hundred milliseconds up to several seconds. Subjects can learn to generate slow cortical amplitude shifts in an electrically positive or negative direction for binary control. This can be achieved if the subjects are provided with feedback on the evolution of their SCP and if they are positively reinforced for correct responses [34]. However, in both of the above methods the subject has to wait for the brain stimulus.
Generally, some BCI approaches rely on the ability of the subjects to develop control of their own brain activity using biofeedback [35–37] whereas others utilize classification algorithms that recognize EEG patterns related to particular voluntary intentions [38]. Initial attempts to enable the subjects to use the feedback from their own brain activity started in the 1960s. The system enables the subjects to gain voluntary control over brain rhythms. It has been claimed that after training with an EEG biofeedback, human subjects are able to detect their own alpha [39,40] and mu rhythms [41]. This was also tested on cats using their mu rhythms [42] and dogs [43] to control their hippocampal theta rhythm. Classification-based approaches have also been under research recently [38]. In a recent demonstration, subjects navigated through a virtual environment by imagining themselves walking [44]. These works paved the way for a vast amount of recent research developed by many workers in this area.
Beverina et al. [45] have used P300 and steady-state visual-evoked potentials (SSVEPs). They have classified the ERP feature patterns using support vector machines (SVMs). In their SSVEP approach they have used the signals from the occipital electrodes (Oz, O2, PO8). The stimulations have been considered random in time instants, and a visual feedback [35] has been suggested for training purposes. In normal cases it is possible through the feedback to make some subjects increment some brain wave activities. In another work, SSVEPs have been used in a visually elaborate immersive 3D game [46]. The SSVEP generated in response to phase-reversing checkboard patterns is used for the proposed BCI.
There are many attractions in using the normal EEGs (or spontaneous signals (SSs)) for BCI. A BCI system of this kind generates a control signal at given intervals of time based on the classification of EEG patterns resulting from particular mental activity (MA). In all types of BCI systems human factors such as boredom, fatigue, stress, or various illnesses are of great influence, and therefore motivating the subject often becomes very important.
Although most of the recent work in BCI research has been focused upon scalp EEGs, some work on invasive EEGs has also been reported. Invasive BCI approaches are based on recordings from ensembles of single brain cells or on the activity of multiple neurons. They rely on the physiological properties of individual cortical and subcortical neurons or combination of neurons that modulate their movement-related activities. These works started in the 1960s and 1970s through some experiments by Fetz and his coresearchers [47–52]. In these experiments, monkeys learnt how to control the activity of their cortical neurons voluntarily with the help of biofeedback, which indicated the firing rate of single neurons. A few years later, Schmidt [53] indicated that the voluntary motor commands could be extracted from raw cortical neural activity and used them to control a prosthetic device designed to restore motor functions in severely paralysed patients.
Most of the research on invasive BCI was carried out on monkeys. These works relied on single cortical site recordings either of local field potentials [54–57] or from small samples of neurons or multiple brain zones [58–60]. They are mostly recorded in the primary motor cortex [58,59], although some work has been undertaken on the signals recorded from the posterior parietal cortex [61]. Deep brain stimulators have recently been used to monitor patients with Parkinson’s disease [62].
Philip Kennedy and his colleagues [63] have presented an impressive result from cortical implanted electrodes. In another work [4] the implanted electrodes were used inside the brain of a monkey to control a robot arm remotely. These works, however, require solutions to possible risk problems, advances in robust and reliable measurement technologies, and clinical competence. On the other hand, the disadvantage of using scalp recordings lies in the very low quality of the signals, due to attenuation of the electrical activity signals on their way to the electrodes.
In the following subsections a number of features used in BCI are explained. Initially, the changes in EEG before, during, and after the externally or internally paced events are observed. These events can be divided into two categories: in one type the ERPs included evoked potentials and in the second type event-related desynchronization (ERD) and synchronization (ERS). The main difference between the two types is that the ERP is a stimulus-locked or, more generally, a phase-locked reaction, while the ERD/ERS is a non-phase-locked response. In a finger movement process, for example, often discussed in BCI, premovement negativity prominent prior to movement onset, and postmovement beta oscillations occurring immediately after movement offset are respectively phase-locked (evoked) and non-phase-locked processes [64].
7.1.1 ERD and ERS
ERD and ERS can be considered as event-related potentials (which can also include evoked potentials). The cortical mu rhythm is an example of ERD. ERD is due to blocking of alpha activity just before and during the real or imagery movement.
ERD is measured in terms of the power of the peak in the alpha band to the bandwidth, that is
where P (f, n) is the value of a signal power at a given time–frequency point of an average power map and Pref(f) is an average power during some reference time calculated for frequency f. This represents the level of rhythmic activity within the alpha band just before or during the movement. Any attention dramatically attenuates the alpha rhythms, while an increase of task complexity or attention results in an increased magnitude of ERD.
Increased cellular excitability in thalamocortical systems results in a low amplitude desynchronized EEG. Therefore ERD may be due to an electrophysiological correlate of various activated cortical regions involved in processing sensory or cognitive information or production of a motor reaction. Involvement of more neurons increases the ERD magnitude. In the BCI context, explicit learning of a movement sequence, e.g. key pressing with different fingers, is accompanied by an enhancement of the ERD over the contralateral central regions. As the learning progresses and becomes more automatic the ERD decreases.
The cortical mu rhythm is of particular interest in BCI mainly because it can be modulated/translated through imaginary and can be monitored via a noninvasive technique. The overall alpha band may be divided into lower and higher alphas. Lower alpha ERD (6–10 Hz) is a response to any type of task and is topographically spread over almost all electrodes. Higher alpha ERD, restricted to parietooccipital areas, is found during visually presented stimulations.
The level of ERD is closely linked to semantic memory processes. Those with good memory show a larger ERD in the lower alpha band [65]. In an auditory memory task, the absence of ERD can be explained by the anatomical localization of the auditory cortex below the surface. Detection of auditory ERD from the EEGs is therefore normally difficult.
As related to BCI, voluntary movement also results in a circumscribed desynchronization in the upper alpha and lower beta bands, localized over sensorimotor regions [66]. ERD starts over the contralateral rolandic region and, during the movement, becomes bilaterally symmetrical with execution of movement. It is of interest that the time course of the contralateral mu desynchronization is almost identical to brisk and slow finger movements, starting about two seconds prior to movement onset. Generally, brisk and slow finger movements have different encoding processes. Brisk movement is preprogrammed and the afferents are delivered to the muscles as bursts. On the other hand, slow movement depends on the reafferent input from kinaesthetic receptors evoked by the movement itself.
Finger movement of the dominant hand is accompanied by a pronounced ERD in the ipsilateral side, whereas movement of the nondominant finger is preceded by a less lateralized ERD [66]. Circumscribed hand area mu ERD can be found in nearly every subject, whereas a foot area mu ERD is hardly localized close to the primary foot area between both hemispheres.
In another study [67] with subdural electrodes, it was discovered that mu rhythms are not only selectively blocked with arm and leg movements, but also with face movement. Using this method the electrodes are inserted under the skull and acquire the signals from over the cortex. These signals are called electrocorticograms (ECoGs). The ECoGs capture more detailed signals from smaller cortical areas than the conventional EEG-based systems. These signals also contain low-amplitude high-frequency gamma waves. Consequently, ECoG-based BCIs have better accuracy and require a shorter training time than those of EEGs [68].
In ERS, however, the amplitude enhancement is based on the cooperative or synchronized behaviour of a large number of neurons. In this case, the field potentials can be easily measured even using scalp electrodes. It is also interesting to note that approximately 85% of cortical neurons are excitatory, with the other 15% being inhibitatory.
7.1.2 Transient Beta Activity after the Movement
This activity, also called postmovement beta synchronization (PMBS), is another interesting robust event that starts during the movement and continues for about 600 ms [66]. It is found after finger or foot movement over both hemispheres without any significant bilateral coherence. The frequency band may vary from subject to subject; for finger movement the range is around 16–21 Hz [69] whereas for foot movement it is around 19–26 Hz [70]. The PMBS has similar amplitude for brisk and slow finger movements. This is interesting since brisk and slow movements involve different neural pathways. Moreover, this activity is significantly larger with hand movement as compared to finger movement [66]. Also, larger beta oscillations with wrist movement as compared to finger movement can be interpreted as the change of a larger population of motor cortex neurons from an increased neural discharge during the motor act to a state of cortical disfacilitation or cortical idling [66]. This means that movement of more fingers results in a larger beta wave. Beta activity is also important in the generation of a grasp signal, since it has less overlap with other frequency components [71].
7.1.3 Gamma Band Oscillations
Oscillation of neural activity (ERS) within the gamma band (35–45 Hz) has also been of interest recently. Such activity is very obvious after visual stimuli or just before the movement task. This may act as the carrier for the alpha and lower beta oscillations, and relate to binding of sensory information and sensorimotor integration. Gamma, together with other activities in the above bands, can be observed around the same time after performing a movement task. Gamma ERS manifests itself just before the movement, whereas beta ERS occurs immediately after the event.
7.1.4 Long Delta Activity
Rather than other known ERS and ERD activities within alpha, beta, and gamma bands a long delta oscillation starts immediately after the finger movement and lasts for a few seconds. Although this has not been reported often in the literature, it can be a prominent feature in distinguishing between movement and nonmovement states.
The main task in BCI is how to exploit the behaviour of the EEGs in the above frequency bands before, during, and after the imaginary movement, or after certain brain stimulation, in generation of the control signals. The following sections address this problem.
7.2 Major Problems in BCI
A simple BCI system setup is illustrated in Figure 7.1. As mentioned previously, the major problem in BCI is separating the control signals from the background EEG. Meanwhile, cortical connectivity, as an interesting identification of various task related brain activities, has to be studied and exploited. Detection and evaluation of various features in different domains will then provide the control signals. To begin, however, the EEG signals have to be preprocessed since the signals are naturally contaminated by various internal and external interferences. Data conditioning such as prewhitening may also be necessary before implementation of the source separation algorithms.
7.2.1 Preprocessing of the EEGs
In order to have an artefact-free EEG to extract the control signals, the EEGs have to be restored from the artefacts, such as eye-blinking, electrocardiograms (ECGs), and any other internal or external disturbing effects.
Figure 7.1 A typical BCI system using scalp EEGs
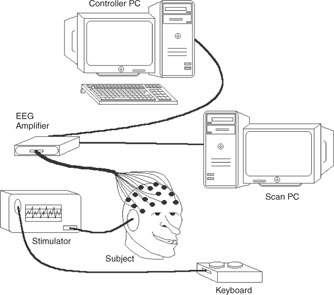
Eye-blinking artefacts are very clear in both frontal and occipital EEG recordings. ECGs, on the other hand, can be seen more over the occipital electrodes. Many attempts have been made by different researchers to remove these artefacts.
Most of the noise, external and even internal artefacts, such as ECGs, are filtered out by the hardware provided in new EEG machines. As probably the most dominant remaining artefact, interfering eye blinks (ocular artefact (OA)) generate a signal within EEGs that is on the order of ten times larger in amplitude than cortical signals, and can last between 200 and 400 ms.
There have been some works by researchers to remove OAs. Certain researchers have tried to estimate the propagation factors, as discussed in Reference [72], based on regression techniques in both the time and frequency domains. In this attempt there is a need for a reference electrooculogram (EOG) channel during the EEG recordings.
PCA and SVMs have also been utilized for this purpose [73]. These methods rely on the uncorrelatedness assumption of the EEGs and OAs. Adaptive filtering has also been utilized [74]. This approach has considered the EEG signals individually and therefore ignored the mutual information among the EEG channels. ICA has also been used in some approaches. In these works the EEG signals are separated into their constituent independent components (ICs) and the ICs are projected back to the EEGs using the estimated separating matrix after the artefact-related ICs are manually eliminated [75]. In Reference [76] a BSS algorithm based on second order statistics separates the combined EEG and EOG signals into statistically independent sources. The separation is then repeated for a second time with the EOG channels inverted. The estimated ICs in both rounds are compared, and those ICs with different signs are removed. Although, due to the sign ambiguity of the BSS the results cannot be justified it is claimed that by using this method the artefacts are considerably mitigated. As noticed, there is also a need to separate EOG channels in this method.
In another recent attempt, an iterative SOBI-based BSS method followed by classification of the independent components using SVMs has been designed to effectively remove the EOG artefacts [77]. The method can also be easily extended to removal of the ECG artefacts. The proposed algorithm consists of BSS, automatic removal of the artefact ICs, and finally reprojection of the ICs to the scalp, providing artefact-free EEGs. This is depicted in Figure 7.2. Iterative SOBI as previously discussed has been effectively used to separate the ICs in the first stage. In the second stage only four features were carefully selected and used for classification of the normal brain rhythms from the EOGs. These features are as follows:
Figure 7.2 A hybrid BSS–SVM artefact removal system [77]
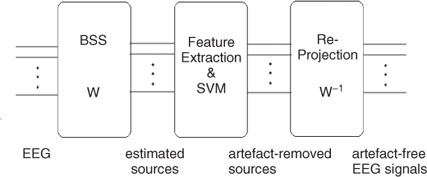
Feature I. A large ratio between the peak amplitude and the variance of a signal suggests that there is an unusual value in the data. This is a typical identifier for the eye blink because it causes a large deflection on the EEG trace. This is described mathematically as:
where u(n) is one of the N ICs, max(.) is a scalar valued function that returns the maximum element in a vector, σu is the standard deviation of u(n), and | · | denotes absolute value. Normal EEG activity is tightly distributed about its mean. Therefore a low ratio is expected while the eye-blink signals manifest a large value.
Feature II. This is a measure of third order statistics of the data, skewness. This feature is defined as
for zero mean data. The EEG containing eye-blinks typically has a positive or negative skewness since the eye-blinking signal has a considerably larger value for this feature.
Feature III. The correlation between the ICs and the EEG signals from certain electrodes is significantly higher than those of other ICs. The electrodes with most contributed EOG are frontal electrodes Fp1, Fp2, F3, and F4 and occipital lobe electrodes O1 and O2 (in total six electrode signals). The reference dataset, i.e. the EEG from the aforementioned electrodes, is distinct from the training and test datasets. This will make the classification more robust by introducing a measure of the spatial location of the eye-blinking artefact. Therefore, the third feature can be an average of the correlations between the ICs and the signals from these six electrodes:
where xi0 (n) are eye-blinking reference signals and i indexes each of the aforementioned electrode locations. The value of this feature will be larger for ICs containing the eye-blinking artefact, since they will have a larger correlation for a particular value of τ, in contrast to ICs containing normal EEG activity.
Feature IV. The statistical distance between distributions of the ICs and the electrode signals that are more likely to contain EOG is used. This can be measured using the Kullback–Laibler (KL) distance, defined as
where p(.) denotes the PDF. When the IC contains OA the KL distance between its PDF and the PDF of the reference IC will be approximately zero, whereas the KL distance to the PDF of a normal EEG signal will be larger.
An SVM with an RBF nonlinear kernel is then used to classify the ICs based on the above features. Up to 99% accuracy in detection of the EOG ICs has been reported [77].
After the artefact signals are marked, they will be set to zero and all the estimated sources are reprojected to the scalp electrodes to reconstruct the artefact-free EEG signals. Figure 7.3 shows the EEG signals containing OA and the restored signals after following the above procedure.
Figure 7.3 The EEG signals: (a) original EEG signals including eye-blinking artefacts mainly around the frontal electrodes and (b) the same set of EEGs after removing the artefact using the hybrid BSS–SVM system
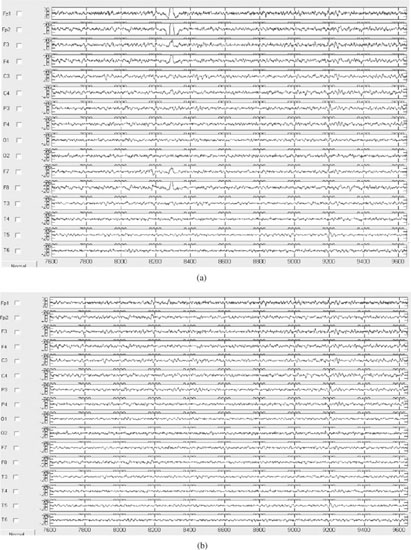
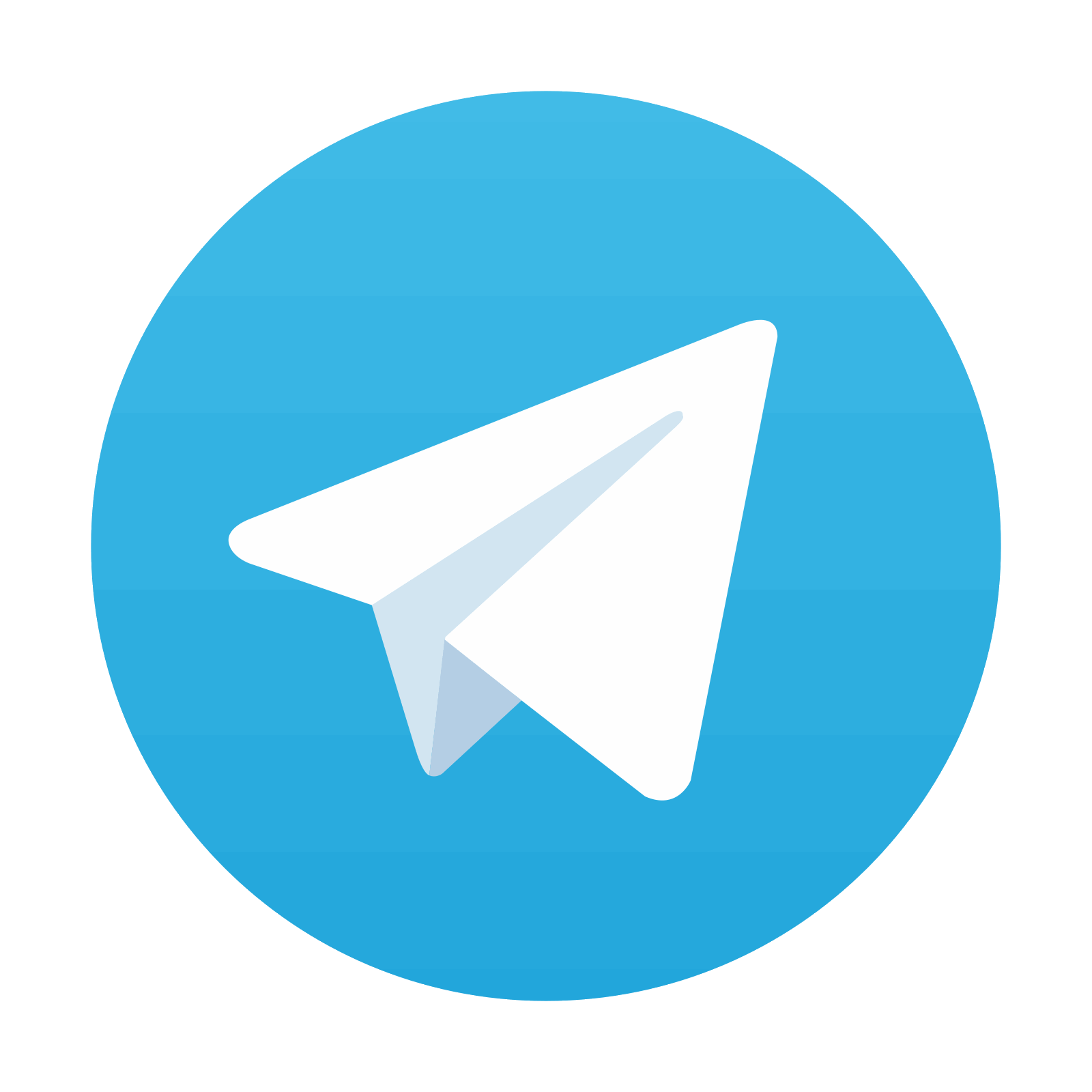
Stay updated, free articles. Join our Telegram channel

Full access? Get Clinical Tree
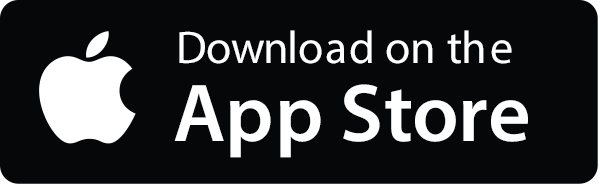
