The relation between glycemic index, glycemic load, and subclinical coronary atherosclerosis is unknown. The aim of the study was to evaluate the associations between energy-adjusted glycemic index, glycemic load, and coronary artery calcium (CAC). This study was cross-sectional analysis of 28,429 asymptomatic Korean men and women (mean age 41.4 years) without a history of diabetes or cardiovascular disease. All participants underwent a health screening examination between March 2011 and April 2013, and dietary intake over the preceding year was estimated using a validated food frequency questionnaire. Cardiac computed tomography was used for CAC scoring. The prevalence of detectable CAC (CAC score >0) was 12.4%. In multivariable-adjusted models, the CAC score ratios (95% confidence intervals) comparing the highest to the lowest quintile of glycemic index and glycemic load were 1.74 (1.08 to 2.81; p trend = 0.03) and 3.04 (1.43 to 6.46; p trend = 0.005), respectively. These associations did not differ by clinical subgroups, including the participants at low cardiovascular risk. In conclusion, these findings suggest that high dietary glycemic index and glycemic load were associated with a greater prevalence and degree of CAC, with glycemic load having a stronger association.
The glycemic index, which indicates the quality of the carbohydrates in the diet, is a measure of the ability of carbohydrates to increase blood glucose level. The glycemic load is the glycemic index of a certain food multiplied by the amount of carbohydrates in a serving, representing both the quality and quantity of dietary carbohydrates. The glycemic index and the glycemic load have been shown to predict postprandial glycemia and insulinemia. Moreover, the glycemic index and glycemic load are also associated with unfavorable changes in fasting lipid levels and inflammation marker and development of chronic diseases, including diabetes and cardiovascular disease. Coronary artery calcium (CAC) scoring using cardiac computed tomography (CT) is a valid tool for identifying subclinical coronary atherosclerosis that predicts future coronary heart disease (CHD) risk. The degree of CAC detected by CT reflects the coronary atherosclerosis burden and provides information on the underlying pathologic changes in coronary arteries. To date, no study has investigated the associations between dietary glycemic index, glycemic load, and CAC accumulation. We therefore examined these relationships in young and middle-aged asymptomatic adults without a history of CVD or diabetes.
Methods
The Kangbuk Samsung Health Study is a cohort study of Korean men and women who underwent comprehensive annual or biennial examinations at the Kangbuk Samsung Hospital Total Healthcare Centers in Seoul or Suwon, South Korea. The study population for the current analysis consisted of 30,485 participants who underwent a comprehensive health checkup, which included cardiac CT for CAC measurements, and who also completed a validated Food Frequency Questionnaire (FFQ) from March 2011 to April 2013. Annual or biennial health screening examinations are widely performed in Korea as health examinations are mandatory for all workers under the Industrial Safety and Health Law.
We excluded 437 participants with a history of CVD and 1,250 participants with a history of diabetes, which was defined as a self-reported previous diagnosis or the use of the blood glucose-lowering agents. These participants were excluded because these diagnoses may lead to change in dietary pattern. We also excluded 474 participants who had implausible estimated energy intake levels (>3 standard deviations from the log e -transformed mean energy intake). The final sample size for this analysis was 28,429 participants ( Figure 1 ). The study was approved by the Institutional Review Board of Kangbuk Samsung Hospital. The requirement of informed consent was waived as we used only de-identified data that were routinely collected during the health screening examinations.
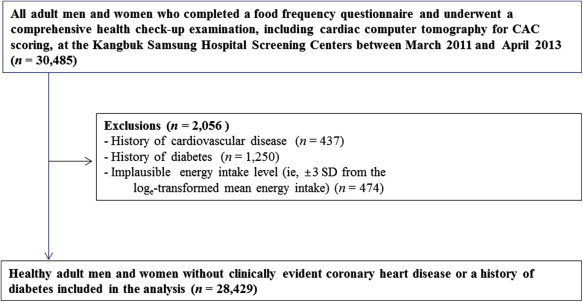
Dietary intake was assessed using a 103-item, self-administered FFQ designed to capture usual dietary habits of Koreans. The reproducibility and validity of the nutrient information provided by the FFQ have been described elsewhere. Participants were asked how often, on average, they had consumed each type of food or beverage over the previous 12 months. The FFQ had 3 predefined categories of portion size, ranging from small to large, and 9 predefined categories of frequency, ranging from never or seldom to ≥3 times/day for foods and from never or seldom to ≥5 times/day for beverages. Participants were also asked to report the consumption period (i.e., 3, 6, 9, or 12 months) for seasonal consumption of fruits.
Average daily nutrient intakes were estimated using a food composition table derived from the Korean Nutrition Society Database. Glycemic load was calculated by first multiplying the carbohydrate content of each food item by its glycemic index value and then by its frequency of intake; the total glycemic load was then calculated as the sum of all food items. Each unit of dietary glycemic load represents the equivalent of 1 g of carbohydrate from pure glucose. The overall glycemic index for a participant was calculated by dividing the glycemic load by the average daily carbohydrate intake. The glycemic index values of individual food items were obtained from international tables, from an online database of the University of Sydney and from published values for Korean foods. To control for total energy intake, glycemic index, glycemic load, and all nutrient intakes were energy adjusted using the regression-residual method.
CAC measurement was performed with a Lightspeed VCT XTe-64 slice multidetector CT scanner (GE Healthcare, Tokyo, Japan) in both the Seoul and Suwon centers using the same standard scanning protocol with 2.5-mm slice thickness, 400-ms rotation time, 120-kV tube voltage, and 124-mAs (310 mA × 0.4 seconds) tube current under electrocardiographic-gated dose modulation. Quantitative CAC scores were calculated using Agatston method. The interobserver and intraobserver reliability values of CAC scores were both excellent, with intraclass correlation coefficients of 0.99.
Information on sociodemographic characteristics, medical history, medication use, family history, physical activity, alcohol intake, and smoking habit was collected using a self-administered questionnaire. Anthropometric variables and blood pressure were measured by trained staff. Physical activity level was assessed by the Korean-validated version of short form of the International Physical Activity Questionnaire; participants were classified as inactive, minimally active, or health-enhancing physically active.
Blood specimens were sampled from the antecubital vein after at least 10 hours of fasting. Methods for analyzing serum biochemical parameters, including serum levels of glucose, hemoglobin A1c (HbA1c), insulin, total cholesterol, triglycerides, LDL-C, and HDL-C have been described in detail elsewhere. The Department of Laboratory Medicine at Kangbuk Samsung Hospital has been accredited by the Korean Society of Laboratory Medicine and participates annually in inspections and surveys by the Korean Association of Quality Assurance for Clinical Laboratories and the College of American Pathologists Proficiency Testing program. Insulin resistance was assessed with the homeostasis model assessment–insulin resistance calculation (fasting glucose [mg/dl] × fasting insulin [μIU/ml]/405).
We investigated the associations between energy-adjusted dietary glycemic index, glycemic load, and CAC score using multiple analytic approaches. For the primary analyses, we used Tobit models to estimate CAC score ratios and their 95% confidence intervals (CIs) by comparing each quintile of energy-adjusted glycemic index and glycemic load intake to the lowest quintile. We modeled log e (CAC score + 1) using robust Tobit regression models with Huber–White estimation of standard errors. We log e -transformed the CAC scores because the distribution was skewed to the right and used Tobit models because the CAC scores were left censored at 0 Agatston units (nondetectable CAC scores). We presented the estimates of Tobit models as exponentiated Tobit regression coefficients (CAC score ratios), representing approximately the relative CAC score increment comparing each quintile of intake of glycemic index or glycemic load to the lowest quintile. For example, a CAC ratio of 1.50 can be interpreted as a 50% increase in the CAC score for the specific quintile compared to the lowest quintile. For secondary analyses, we categorized CAC scores into 3 groups (CAC score 1 to 100 and >100 compared with CAC score 0) and used multinomial logistic regression to estimate the prevalence ratios of CAC scores in the 1 to 100 and >100 groups by quintiles of glycemic index and glycemic load. In addition, we categorized CAC scores as a dichotomous variable (CAC score >0 compared with CAC score 0) and used logistic regression to estimate the odds ratios for detectable CAC by quintiles of glycemic index and glycemic load.
The basic model (model 1) estimating the associations of dietary glycemic index and glycemic load with CAC was adjusted for age, gender, study center, year of screening examination, total energy intake (quintiles), and energy-adjusted protein and fat intake (quintiles). Model 2 included all variables that were used in model 1 plus education (high school graduate or less, college graduate, graduate school or high, or unknown), physical activity (inactive, minimally active, health-enhancing physically active, or unknown), smoking (never, former, current, or unknown), body mass index (BMI, kg/m 2 ), parental history of CHD (yes or no), self-reported history of hypertension (yes or no), self-reported history of hypercholesterolemia (yes or no), alcohol intake (0, <5, 5 to <20, ≥20 g/day, or unknown), and energy-adjusted fiber intake (quintiles). Further adjustment for fasting glucose or HbA1c, as a potential intermediate factor, did not appreciably change the results. Tests for linear trends were conducted by assigning the median value to each quintile and modeling this variable as a continuous variable in the regression models. We also modeled energy-adjusted glycemic index and glycemic load as continuous variables using restricted quadratic splines (with knots at the fifth, fiftieth, and ninety-fifth percentiles of their sample distributions) to provide a flexible dose–response relation with detectable CAC (CAC >0).
We also conducted subgroup analyses to determine whether the associations of glycemic index and glycemic load with CAC score were modified by age (<40 or ≥40 years), gender, smoking status (never/past or current), alcohol intake (nondrinker or drinker), BMI (<25 or ≥25 kg/m 2 ), history of hypertension (yes or no), history of hypercholesterolemia (yes or no), or 10-year Framingham CHD risk score (<10 or ≥10%). Interactions were tested by including the cross product terms in the models and using Wald tests to determine the statistical significance level. All analyses were conducted using STATA version 13.1 (Stata Corp., College Station, Texas). Statistical tests were 2 sided; p values <0.05 were considered statistically significant.
Results
The mean age of study participants was 41.4 years (SD 7.5 years), and 79.4% of them were men. The average carbohydrate intake was 263.8 g/day (SD 39.4 g/day), which accounted for 65.9% of the total energy intake. The average glycemic index and glycemic loads were 55.1 (SD 5.4) and 146.9 (SD 32.5), respectively. The main foods that contributed to the glycemic load were rice (57.3% of the mean intake of the glycemic load), instant noodles (4.2%), and any kind of beverages (except soft drinks; 4.2%). The prevalence of a CAC score >0 was 12.4% (n = 3,524), which included the 10.6% of participants (n = 3,008) with a CAC score 1 to 100 and the 1.8% (n = 516) of participants with a CAC score >100. Compared with the participants in the lowest quintile of dietary glycemic load, those in the highest quintile were more likely to be older and women but less likely to be educated, current smokers, physically active, and obese ( Table 1 ). Those in the highest quintile also tended to have no history of hypercholesterolemia. Compared with participants in the lowest quintile of dietary glycemic load, those in the highest quintile had higher level of HbA1C but had lower levels of homeostasis model assessment–insulin resistance, systolic blood pressure, and total cholesterol and tended to consume less total energy, protein, fat, fiber, fruits, vegetables, red or processed meat, and alcohol but more carbohydrates. Similar associations were observed for glycemic index (data not shown).

Full access? Get Clinical Tree
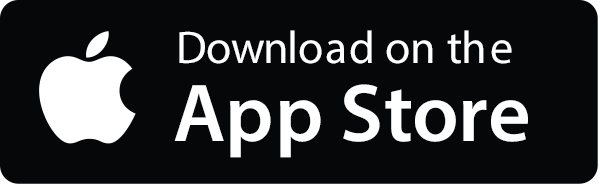
