Quality of Care and Medical Errors in Cardiovascular Disease
Eric D. Peterson
Robert M. Califf
Overview
Definitions of Quality of Care and Patient Safety
Quality of care has been defined as “the degree to which health service for individuals and populations increase the likelihood of desired health outcomes and are consistent with current professional knowledge” (1). As characterized by the Institute of Medicine (IOM), quality of care has several dimensions, including safety, effectiveness, timeliness, efficiency, equitability, and patient-centeredness (2). Table 45.1 applies these principles to the care of cardiovascular patients. A medical error is defined as either having the wrong plan for an element of medical care or failure to execute the correct plan. These errors can be further subdivided into errors of omission, defined as a failure to apply therapies that are proven beneficial, and errors of commission, the inappropriate delivery of a medical treatment or intervention (3). Thus, quality of care and medical error avoidance are linked concepts, requiring caregivers both to do the right things and to do things right.
If asked a decade ago, most clinicians would have assumed cardiac care provided was nearly always appropriate and delivered in a safe, efficient manner. Careful scrutiny, however, has failed to confirm this rosy assumption. Patients often fail to receive lifesaving therapies (4,5,6,7,8), and still others are subjected to diagnostic and therapeutic procedures in situations where there is little proven benefit (9,10). Care delivery itself is often imperfect, burdened by unnecessary delays and technical mistakes (11,12,13,14). These and other studies prompted the IOM to conclude in 2002 that “there is not a gap between what healthcare is now and what could be, but rather a chasm” (2).
Such troubling findings have prompted government, payers, and the public to challenge physicians’ autonomy (15). These external parties are now demanding our health care provider professions both to monitor and to improve the consistency and quality of health care. Quality assessment and medical error reports are now being posted for the public (16,17,18,19). Even more challenging, payers are now differentially reimbursing hospitals and physicians based on the quality of care they deliver, initiating the new era of “pay for performance” (P4P) (20,21,22). Although these new policies have the potential to revolutionize health care quality and safety, they also may lead to unintentional adverse consequences for patients and providers alike (23). The balance between these alternative futures will depend on the how these new policies are implemented and the response of health care providers to them.
Given the importance of improving patient outcomes and the likely impact on the practice and income of cardiovascular specialists, clinicians need to gain an understanding of the methodology used for quality assessment as well as the means of improving quality of care. This chapter provides a historical perspective on the field and defines the major concepts of quality and medical errors. We review the current quality indicators for cardiovascular care and procedures as well as identify many of the methodologic challenges that belie their measurement. We summarize available information on the state of cardiovascular quality of care and then review means by which this care may be improved in the future.
TABLE 45.1 Defining Quality in Cardiovascular Care | ||
---|---|---|
|
Historical Perspective
Although the quality of care has been a part of the medical profession since its beginning, Florence Nightingale is generally credited with codifying many of its fundamental concepts in her book Notes on Hospitals (24). Published in 1863, this review of hospitals in London and the United Kingdom found a 91% death rate in the 24 hospitals in London, versus 40% in 55 county and regional facilities. She used these data to recommend sweeping changes in the operations, care processes, and location of the London hospitals (24,25). Her vision also extended to an understanding of the challenges involved with measuring quality of care when she wrote the following:
Accurate hospital statistics are much more rare than is generally imagined, and at best they only give the mortality which has taken place in the hospitals, and take no cognizance of those cases which are discharged in a hopeless condition, to die immediately afterwards, a practice which is followed to a much greater extent by some hospitals than others. We have known incurable cases discharged from one hospital, to which the deaths ought to have been accounted and received into another hospital, to die there in a day or two after admission, thereby lowering the mortality rate of the first at the expense of the second (24).
Nearly 145 years later, we are still coping with many of the same issues—suboptimal data collection, lack of adequate follow-up, interhospital transfer of high-risk patients who are “lost” in current report card measurement systems, and less-than-expected accountability.
In 1914, Ernest Amory Codman, a surgeon in Boston, started his own center known as the End-Result Hospital, based on the philosophy that caregivers should both study their outcomes and be rewarded with higher reimbursement when their outcomes are documented to be superior to those of their peers (26,27). In 1917, he outlined these principles:
So I am called eccentric for saying in public: that hospitals, if they wish to be sure of improvement, 1) must find out what their results are; 2) must analyze their results to find their strong and weak points; 3) must compare their results with those of other hospitals; 4) must welcome publicity not only for their successes but for their errors. Such opinions will not be eccentric in a few years hence (28).
Although Codman was a visionary in his outlook on quality, he slightly miscalculated the time course; it would take to the end of the century for these policy changes to take hold.
In 1986, the U.S. Health Care Financing Administration (HCFA) put quality concerns back in the spotlight when it analyzed and publicly released individual hospitals’ mortality rates (29,30). Like Nightingale’s analysis a century earlier, these data demonstrated substantial variability among centers. Yet similar concerns existed over the ability of these data to “adjust” for patients’ disease severity (27,31). For example, the hospitals reported with the highest mortality rates were, not surprisingly, cancer hospice facilities. Risk adjustment was an imperfect and underdeveloped science. Because of these methodologic limitations, the HCFA ultimately stopped the release of hospital mortality reports (29), yet public reporting of provider results had been released from Pandora’s box.
That clinicians can cause harm as well as cure has been understood since medicine’s beginnings. In ancient Greek, the same word, pharmakon, meant both “drug” and “poison” (32). In the sixteenth century, Paracelsus, the father of clinical pharmacology, updated this concept when he said: “All drugs are poisons; the benefit depends on the dosage” (33). Thus, as we will see, many therapies can result in avoidable iatrogenic harm if they are delivered in an improper setting, dose, or manner.
The review of treatment “failures” has also been a means of understanding how to avoid potential mistakes in the future. Morbidity and mortality conferences were therefore designed to determine whether clinician care was the cause of an individual patient’s poor outcomes. Similarly, systematic review of medical error also can lead to care improvements. As an example, in 1847, Dr. Semmelweis working in Vienna noticed that peripartum wound infection rates were killing 13% of women giving birth at his hospital, a rate four times that seen at a nearby hospital run by midwives. By following students in their daily care, Dr. Semmelweis noted that many went directly from the dissection room to deliveries without washing their hands. By establishing a new policy of strict disinfection between cases, he was able to drop infection rates to less than 2%, thus saving countless lives (34).
More recently, the impact of medical errors on public health was graphically summarized by the IOM report To Err Is Human. This study estimated that between 40,000 and 100,000 patients each year died in U.S. hospitals as a result of medical errors, and the financial cost of such events was estimated at 17 to 29 billion dollars (3). Although this estimate was hotly disputed by many health care providers, it is likely an underestimate, because it did not consider the full extent of errors of omission, especially for chronic cardiovascular disease. Multiple recent studies have estimated that at least 100,000 lives per year could be saved by optimizing the use of long-term cardiovascular medications.
The Quality Cycle
Cardiology has led the way in medicine in terms of amassing an evidence base for clinical decision making as well as for monitoring and improving care quality. The cycle of clinical therapeutics (Fig. 45.1) provides a model for the adoption of new findings into routine clinical practice (35). Large randomized clinical trials have become the standard in cardiology to define the safety and effectiveness of new therapies (36,37). The findings from these published trials are then reviewed by expert committees from the major cardiovascular professional societies and are summarized into evidence-based clinical practice recommendations. These recommendations define therapies for which the balance of safety and effectiveness is positive for a defined population (class I therapies) and those that are ineffective and perhaps harmful (class III). Most current therapies, unfortunately, are in an uncertain category (class II). These guidelines can be used to distill quality indicators that define specific situations when giving a therapy is uncontroversial; if not given, a quality concern exists. Based on these quantifiable quality indicators, the performance of the individual provider, provider group, hospital, or health system can be objectively measured (38). Such performance data can then be
benchmarked against peers and feedback given to providers as a means of driving care adoption (39). The ultimate goal of this cycle is to promote the consistent use of best practices to improve the outcomes of patients (see Fig. 45.1).
benchmarked against peers and feedback given to providers as a means of driving care adoption (39). The ultimate goal of this cycle is to promote the consistent use of best practices to improve the outcomes of patients (see Fig. 45.1).
This idealized cycle of evidence-based care, however, can become derailed at multiple junctures. Unfortunately, many decisions in medicine have not or cannot be systematically studied (40). The studies on which the quality cycle is based often lack an appropriate design or control population, or they may be conducted in highly selected patient populations and clinical settings for inadequate time periods with end points that cannot be used to have assurance about the balance of benefit and risk for the therapy. These methodologic limitations thereby hinder the development of evidence-based care guidelines (37).
The guidelines process itself introduces further challenges. Not all medical decisions (or patients) fall into simple care algorithms, and reductionist strategies often result in oversimplification. Guideline developers often use subgroup analyses despite their known limitations (41). Conflict-of-interest issues may cloud the objectivity of the guidelines developers (42), and the process itself is slow. After multiple rounds of review and consensus building, the guidelines are often out of date soon after publication (43).
Performance measurement and feedback can create further distortions. Similar to the guidelines process, long delays occur before new study findings are incorporated into existing performance indicators. An accurate picture of provider quality emerges only after examining multiple performance metrics, yet current measurement sets are severely constrained by the shortage of adequate supporting clinical trials and the expense of data collection in practice (6). Substantial delays often occur between care delivery and performance feedback, and this feedback often fails to reach front-line caregivers (44). Finally, providers have traditionally lacked adequate incentives for reviewing these data and/or changing practice.
Data Sources for Quality Assessment
Data availability is in many ways the linchpin for quality assessment. Peter Drucker summarized it thus: “If you can’t measure it, you can’t manage it.” This holds for medicine as well as for business. Yet the validity of quality of care measures cannot be understood without understanding where such data come from and their potential limitations. Specifically, there are major concerns that many of the data available to the public have previously relied on inaccurate sources and extrapolation beyond the limits of the data: bad data in, bad data out. Data sources for quality assessment include administrative databases (also known as claims or billing data), the patient’s paper medical record, and more recently, electronic health records and multicenter clinical data registries.
Administrative Databases
The primary purpose for administrative databases is to allow for patient billing and tracking. The U.S. Medicare claims system provides an example of administrative data sources. Medicare Part A fields cover inpatient hospital claims, whereas Part B fields cover outpatient and physician claims. Claims databases, such as Medicare, generally capture patient demographic information (age, gender, race), a limited number of diagnostic codes (e.g., primary diagnosis and secondary diagnoses), major procedure codes, and a few outcomes (length of hospital stay, mortality). Claims databases, however, usually do not capture details regarding treatment (e.g., medications used), patient counseling information, or many contraindications for treatments. As such, these databases are often unable to examine many process-based quality of care indicators.
The accuracy of claims databases also limits their role in comparing provider outcomes. For example, in one study the rate of agreement between claims-coded databases and those found on chart review ranged from a low of 9% for unstable angina to a high of 83% for diabetes (45). Claims data also do not differentiate complications of care received from preexisting comorbid conditions (46,47,48). For example, if a patient undergoing bypass surgery had congestive heart failure (CHF) coded as a secondary diagnosis, one could not easily tell whether this was a preoperative risk factor or a postoperative complication. Although the provider’s results should certainly be adjusted for the former, the latter should not, because the poor result represents an adverse outcome of the treatment. As such, administrative data sources cannot fully adjust for patient risk in provider outcome performance comparisons. Claims databases also generally do not capture detailed pharmacologic or laboratory information, although this is changing rapidly, and pharmaceutical quality is becoming a cornerstone of outpatient quality measurement. Until electronic health records are available, however, accurate information about patient counseling will not be available from claims data. As such, the use of this form of data has significant limitations for examining most process-based quality indicators.
Medical Record Review
Historically, the patient’s paper chart has been a primary data source for clinical data abstraction. However, the chart review process also has many deficiencies as a tool for quality assessment. The nomenclature used to describe similar phenomena varies from practitioner to practitioner. Pertinent negatives are frequently not mentioned, so the reviewer must assume that if a finding or procedure is not mentioned in the medical record, it was not evaluated or done. In an adverse event reporting study, a chart audit missed more than half of adverse events related to medications (49). Beyond these limitations, chart review can be exorbitantly costly, thereby precluding it as a means of longitudinal large-scale quality assessment.
Clinical Databases and the Electronic Clinical Record
As an alternative to the paper chart, health care policy experts have attempted to drive the adoption of the integrated, interoperable electronic health record in hospital and physician offices (50,51). Such systems incorporate standard nomenclature and database architecture and thus have the capacity to export their information among databases. In the near future, such systems also may have unique patient identifiers that will enable one to track quality of care in a longitudinal fashion.
In parallel to the growth in the electronic collection of clinical information, cardiovascular registries have been growing in both North America and Europe. In general, these clinical registries collect standardized clinical elements regarding a specific procedure or disease state, as well as the patient’s
in-hospital care processes and outcomes. These data are then often provided back to individual sites as a means of benchmarking care and stimulating quality improvement (QI). The earliest examples of such registries were procedure-specific for coronary bypass graft (CABG) surgery and percutaneous coronary intervention (PCI) (52,53,54,55,56,57). More recently, disease-specific clinical data registries have been formed for those suffering myocardial infarction (MI), acute coronary syndromes, CHF, and stroke.
in-hospital care processes and outcomes. These data are then often provided back to individual sites as a means of benchmarking care and stimulating quality improvement (QI). The earliest examples of such registries were procedure-specific for coronary bypass graft (CABG) surgery and percutaneous coronary intervention (PCI) (52,53,54,55,56,57). More recently, disease-specific clinical data registries have been formed for those suffering myocardial infarction (MI), acute coronary syndromes, CHF, and stroke.
Although these multicenter clinical databases have considerable promise as a means of quality assessment and improvement, they also have certain limitations. Sites that elect to participate in these registries may not always be representative of community care providers. Indeed, providers with the worst quality and outcomes may be the least likely to participate voluntarily in a registry measuring quality. Additionally, not all clinical data registries have active auditing systems to ensure that all eligible patients are entered and that entered information is accurate and complete. Finally, patient privacy concerns often limit the ability of these registries to track patients after discharge or if they transfer between facilities.
Selecting Quality Indicators
The selection of indicators to be used to assess quality of care is also challenging. All measures have inherent strengths and limitations. The following are general proposed principles that can guide in this selection process (58).
The measure should be meaningful. Any potential quality indicator must either be directly or closely linked to an important patient or society outcome. The measure should be valid and reliable. To serve as a useful marker of quality, a measure must be able to be consistently measured among multiple providers, in a standard and reproducible fashion.
The measure needs to be able to account or adjust for differences in the type of patients treated by various providers. Specifically, if the metric looks at a given treatment, one needs to be able to define who is and is not eligible for therapy. If the metric examines outcomes, it must be able to risk-adjust these results to reflect any differences in patients’ severity of illness or comorbidity.
The measure should be amenable to improvement. This requires that there be provider variablity in performance at baseline and room to improve. Second, ideally, there should be a known pathway or process for improving on the metric.
It should be economically feasible to measure provider performance over time and among systems. Specifically, although certain metrics such as longitudinal health status are certainly important quality measures, the economic burden of requiring all providers to collect these would be great for routine quality assessment. Advances in technologic and system delivery may alter a metric’s feasibility status.
With these principles in mind, Donabedian, considered by many as the father of quality assessment, classified three principal forms of quality measures—structure, process, and outcome (59).
Structure
The structure of health care delivery refers to characteristics inherent to the treating physicians, nurses, and other health care providers, as well as the facilities and health care system in which care is delivered. Examples of provider structural features include their training and specialty status, as well as level of experience (e.g., years in practice or annual procedural volume). Hospital structural features include center size, academic affiliation, specialty equipment and services provided, nurse-to-patient ratios, and types of disease management or transitional services offered.
Three factors limit the use of structural measures as quality indicators. First, the association between structure and care quality is often incomplete. For example, although studies have often demonstrated a general relationship between procedural volume and outcomes, the association is weak enough that it breaks down at the individual provider level, where there are many exceptions to this rule (44). Second, the reasons for the link between a structural measure and quality are often poorly understood, so remedies for quality problems that are identified are not clear. Third, structural characteristics are usually less amenable to change than are care processes.
Process
Process refers to actions performed in the delivery of care to a patient, including the use of diagnostic and therapeutic modalities, and patient counseling. Process also refers to the timing and technical competency of their delivery. The study of process of care has gained much traction in quality assessment for several reasons. When it is known that one diagnostic or therapeutic strategy is superior to an alternative, it is usually straightforward to measure whether an individual provider or group of providers is using this strategy (60). Thus, measurements of the proportion of patients who are treated with aspirin or β-blocker drugs after MI and the timing of delivery of reperfusion therapy to patients with acute MI have become a standard means of assessing quality. This approach of using explicit process-based performance criteria derived from results of randomized trials has increasingly supplanted traditional nonquantitative chart-based peer review by an independent expert. In particular, the consistency of standardized performance indicators provides for a means of nationally or internationally benchmarking provider quality in an objective fashion.
Although measurement of care processes is growing, there are definite limitations. These include questions of accurately defining the right “denominator”—including those patients who are eligible for a therapy while excluding all those who have contraindications. Measurement of care processes credits “doing something,” but it rarely attempts to gauge when no therapy is indicated. This can lead to overtreatment or rushed initiation of treatment. Additionally, process measures usually are binary (yes/no), with credit given if some treatment or procedure is given, without concern as to whether it was delivered in a technically competent and safe manner. However, this approach is amenable to rewarding giving the correct dose of a medication or behavioral intervention.
Outcomes
Outcomes refers to tangible measures of the consequences of care and can be considered in the categories of length of life, nonfatal adverse events (e.g., recurrent MI, stroke), and patient health status measures (symptoms, functional status, quality of life) (Fig. 45.2). On the positive side, patient outcome represents the summation of all structure and care processes, including the behavior of the patient/consumer. Improving this end product of what happens to the patient is ultimately the driving goal of medical care. On the negative side, however, the assessment of patient outcomes as quality metrics has its challenges. Adverse outcome events are often uncommon and therefore must be studied among large patient samples to detect stable provider differences. This limits what providers can compare or forces assessment over an extended period. Additionally, multiple factors beyond provider quality affect patient outcomes and must be accounted for before outcome comparisons are meaningful. Many important patient outcomes take years to manifest. This has led to increased use of intermediate outcome measures, surrogates closely associated with the
care process and long-term outcomes. Examples of such intermediate measures include the percentage of patients reaching target goals for blood pressure control, cholesterol levels, or hemoglobin A1c values.
care process and long-term outcomes. Examples of such intermediate measures include the percentage of patients reaching target goals for blood pressure control, cholesterol levels, or hemoglobin A1c values.
Beyond clinical events, there is increasing interest in assessing the impact of provider care on patients’ symptoms, health status, and overall quality of life. Several validated mechanisms have been developed for quantifying health status for cardiac patients (61,62). These scales not only measure symptoms but also assess the degree to which these influence patients’ perceptions of their well-being and activities of daily life. Although clearly meaningful to patients and society, collection of health status data is resource intensive, and methods for risk-adjusting these results are still under development (58). Furthermore, to the extent these types of measures are used for internal QI, they can be extremely variable, but in outpatients many factors other than the provider can influence the intermediate outcome, thereby leading to concern that “adverse selection” of patients (i.e., taking the most difficult patients into your clinic) can lead to inferior measures.
Patient Satisfaction
Patient perception of care (satisfaction) is a form of outcome of care delivery. Successful care should strive to leave the patient in the best condition possible, but also to have him or her satisfied with how the care was delivered. Such satisfaction data are gained through systematic survey of patients using standardized questionnaires (63). Patient satisfaction with providers has been linked to the likelihood of that patient returning to his or her provider and/or following medical advice. However, although it is important, patient satisfaction is a multidimensional feature that can be strongly influenced by factors not directly associated with the “hard” elements of care quality (safety and effectiveness). Instead, patient satisfaction often depends on elements such as staff friendliness, waiting delays, food services, and physical environment. Additionally, average responses to standardized patient satisfaction survey tools tend to vary by gender, race, and socioeconomic status; accordingly, just as with intermediate biologic measures, these measures may reflect the cultural composition of the patients served rather than the quality of the service delivered. For these reasons, satisfaction information should be complementary to other quality and safety data but not a substitute, because patients with a high satisfaction level can also have care that is poor in terms of safety and effectiveness.
Cost and Efficiency
Health care resource use or costs represent an outcome of great interest to payers, policy makers, and increasingly the patients who are being asked to shoulder more of the personal costs of health care. The term efficiency has been used to describe the provision of value for the resources used. Unfortunately, operational definitions of efficiency are difficult to establish, because a truly objective measure would require simultaneous definition of the safety, effectiveness, timeliness, and cost of the activity.
Because medical charges (bills) are not necessarily reflective of true costs in the United States, hospital charge information must first be adjusted using hospital-specific cost-to-charge ratios, conversion factors that allow for rough estimates of actual resources used (64). In many other countries, hospital bills do not exist (65). Alternative means of estimating costs are available for centers with more detailed microcost accounting systems (64). These systems collect and tally individual unit costs of health care inputs (e.g., labor, consumable materials, pharmacy, laboratories). Like patient satisfaction, measurement of the resources used in delivery of care should be a secondary or complementary metric relative to quality assessment. Yet as health care costs continue to escalate, there is increasing need for providers to improve both their effectiveness and their efficiency.
Medical Errors
Multiple types of medical errors exist (Table 45.2). Assessment of these errors can be captured as either process metrics (error reporting systems) or patient outcomes (adverse events). Each of these systems for identifying medical errors has promise and potential challenges. Voluntary provider reporting allows for
staff to acknowledge breakdowns in the care process. Review of these data can promote process redesign that prevents such errors from occurring in the future. However, error reporting is subjective, and staff may underreport to avoid admission of guilt or out of fear of retribution. Additionally, many errors in processes of care do not lead to adverse events or harm to a patient. A great deal of work can be put into fixing factors that may or may not influence the outcome of care.
staff to acknowledge breakdowns in the care process. Review of these data can promote process redesign that prevents such errors from occurring in the future. However, error reporting is subjective, and staff may underreport to avoid admission of guilt or out of fear of retribution. Additionally, many errors in processes of care do not lead to adverse events or harm to a patient. A great deal of work can be put into fixing factors that may or may not influence the outcome of care.
TABLE 45.2 Types of Medical Errors | |||
---|---|---|---|
|
Adverse event systems search for sentinel outcome events as signals that a medical error occurred. For example, rates of pressure ulcer or in-hospital patient falls may be indicative of less than ideal nursing care. More recently, electronic surveillance systems have also been employed to monitor for adverse outcomes automatically that may signal safety concerns. For example, pharmacy databases can be automatically searched for the use of naloxone (Narcan) or digitalis antibodies, potentially indicative of opiate or digoxin overdosing, respectively. Like other forms of outcome reporting, the interpretation of the summary of adverse event reporting varies depending on the diligence of the institution and its personnel in reporting events and collecting relevant data. Thus, those most committed to case finding may falsely appear worse than those who intentionally or inadvertently miss such events. The mere identification of an adverse sentinel event does not lead to insight into the event’s underlying cause or means of averting such events in the future.
An important construct in error analysis is that errors are far more common than adverse events; therefore, many adverse events are a subset of errors. Conversely, adverse events occur in the absence of errors when expected side effects or toxicities of therapy occur, so all adverse events are not a subset of errors. Newly funded active surveillance systems are now showing that fewer than 15% of adverse events are reported in voluntary reporting systems; therefore, many more errors occur.
Quality Indicators for Specific Cardiac Conditions
Table 45.3 summarizes current quality indicators defined by the Center for Medicare and Medicaid Services (CMS), the Joint Commission on Accreditation of Healthcare Organizations (JCAHO), and those set for by the National Quality Forum for selected cardiac disease states and procedures. As evident in these tables, in-hospital care processes tend to be the dominant metrics used to assess quality of care for disease states such as acute MI, CHF, or chronic cardiac disease. For revascularization procedures, less is known about what care process leads to better outcomes. Thus, for these conditions, quality tends to be assessed with structural measures (procedural volume) and/or outcomes (procedural mortality or morbidity).
Statistical Issues in Quality Assessment and Provider Profiling
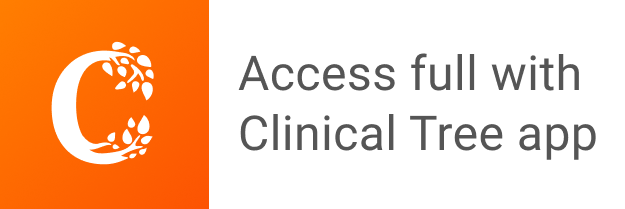