The aim of this study was to create a simple risk index to predict new-onset atrial fibrillation (AF) after coronary artery bypass grafting in patients with histories of AF. AF after coronary artery bypass grafting (referred to here as AF) is associated with increased morbidity and mortality. Identifying patients at high risk for developing AF may help identify a group of patients who might benefit from strategies to prevent postoperative AF. A cohort of 18,517 patients enrolled from January 1, 1996, to December 31, 2009, was used to derive a risk index for AF prediction. A multivariate logistic regression model determined the independent predictive impact of clinical and demographic characteristics on the occurrence of AF. A subset of these variables was used to construct a risk index to predict AF. This risk index was validated in a sequential cohort of 1,378 consecutive patients who underwent coronary artery bypass grafting from January 1, 2010, to June 30, 2011. AF occurred in 3,486 patients in the calibration cohort (18.83%) and in 269 patients in the validation cohort (19.52%). After considering patients’ demographics, co-morbid conditions, and severity of illness, advanced age appeared as the most powerful predictor of AF (odds ratio 1.059/year, 95% confidence interval 1.055 to 1.063). Age, height, weight, and the presence of peripheral vascular disease contributed most to the prediction model. An AF risk index including these variables had adequate discriminatory power, with a concordance index of 0.68. In conclusion, using a large cohort of patients, a simple risk index relying only on preoperative clinical variables was developed, which will help predict AF. This risk index can be used clinically to identify patients at high risk for the development of AF.
Postoperative atrial fibrillation (AF) occurs in 15% to 30% of patients who undergo isolated coronary artery bypass grafting (CABG). Post-CABG AF (referred to as AF throughout this report) is associated with prolonged hospital stay, increased perioperative mortality and morbidity, and long-term mortality. Preventive strategies aimed at reducing AF expose patients unnecessarily to the cost and risk of antiarrhythmic drugs. Hence, identifying specific patients at high risk for developing AF may help define a population that is more likely to benefit from antiarrhythmic drugs or other preventive strategies. Previous studies of AF have not excluded patients who underwent concomitant valve surgery and have included preoperative, intraoperative, and postoperative factors in the risk model, which have made risk indexes cumbersome and less useful clinically. The aim of this study was to derive a simple clinical tool on the basis of preoperative patient characteristics to identify patients at high risk for AF.
Methods
This was a retrospective observational cohort study with a sequentially external validation group designed to develop a predictive algorithm for AF. The study included 2 sequential samples: a calibration sample collected from January 1, 1996, to December 31, 2009, and a validation sample of patients who underwent CABG from January 1, 2010, to June 30, 2011. Prediction models were constructed (calibrated) in the calibration sample and then applied to the validation sample to test their predictive ability on an independent group of patients, thus providing an independent, external validation with a homogenous group of patients.
An analysis of the Society of Thoracic Surgeons Adult Cardiac Database was used to identify consecutive patients with no histories of AF or atrial flutter who underwent isolated CABG at Emory University Hospital or Emory Crawford Long Hospital. The calibration sample consisted of 18,517 patients and the validation sample of 1,378 patients, for a total of 19,895 CABG patients. CABG was performed by 1 of 19 faculty surgeons. The Emory University institutional review board approved the protocol in compliance with Health Insurance Portability and Accountability Act standards and the Declaration of Helsinki and waived the requirement for any additional individual informed consent for these patients.
The primary study end point was new onset of AF after CABG. AF was defined in this study according to the established Society of Thoracic Surgeons definition: the documentation of postoperative AF or atrial flutter requiring treatment (i.e., β blockers, calcium channel blockers, amiodarone, anticoagulation, or cardioversion). The selected variables that could predispose to AF include demographic factors, clinical characteristics, and preexisting medical or surgical co-morbidities. Demographic variables included age, race. and gender. Clinical characteristics included height, weight, body mass index (BMI), body surface area, last creatinine level, angina, the ejection fraction, the presence of left main coronary artery disease, the use of immunosuppressive therapy, preoperative insertion of an intra-aortic balloon pump, and the number of diseased vessels (a numeric discrete variable). Preexisting medical conditions included any history of peripheral vascular disease (PVD), cerebrovascular disease, chronic lung disease, diabetes mellitus, diabetes control method, family history of coronary artery disease, hypertension, renal failure, dialysis, smoking, previous CABG or valve surgery, congestive heart failure, myocardial infarction, and cardiogenic shock. We also included the Society of Thoracic Surgeons predictive risk for mortality and CABG type (on cardiopulmonary bypass or off bypass) as possible predictors.
Dedicated extractors collected data, and checks for data quality were made at the institutional level and also after submission to the Society of Thoracic Surgeons national database.
To statistically evaluate the bivariate association of each predictor with AF, the calibration sample was analyzed for significant predictor variables. Simple logistic regressions were constructed in addition to means, standard deviations, frequency counts, and proportions for patients with and without AF. Predictors with significant associations were noted and set aside for consideration in a multivariate logistic regression predictive model.
Age appeared as the single most significant predictor of AF. To find additional predictors of AF in the presence of age, 3 criteria were considered. First, the net reclassification index (NRI) was measured for each variable in a model that already contained age. The NRI for a new variable measures the additional proportion of cases that are correctly classified when the variable is added. Second, the change in the concordance index (C-index) due to the addition of a new variable to patient age was calculated. The C-index is not as ideal as the NRI in these situations, but it still contains valuable information concerning the worth of a new predictor. Finally, the change in the Nagelkerke R 2 value was measured with the addition of a new variable to a model containing subject age. Positive changes in this value indicate an increased ability of the model to explain variance resulted by adding the new variable. These 3 measures were used to help optimize the selection of a predictive model for AF.
This process created a final multivariate model. Once a multivariate model was selected, its C-index and R 2 value were noted for future comparison to the validation sample.
Once a formal statistical model was chosen, we developed a 4-point clinical scale on the basis of the weights of the coefficients called the AF risk index. This easily implemented tool consisted of a discrete 5-point scale (0 to 4), designed to be similar to common clinical scores such as the Thrombolysis In Myocardial Infarction (TIMI) and CHADS 2 scores. Because the chosen variables were heavily demographic, the scale was indexed separately for men and women. AF proportions were calculated on each index value (0 to 4) to check for positive trends.
The logistic regression model and the AF risk index were evaluated using the external validation sample. Using the predictive logistic model from the calibration sample, a predicted probability of AF was calculated for each validation patient and evaluated in a new bivariate logistic model. The C-index and R 2 value were noted and compared to the corresponding calibration values.
The AF risk index was also calculated for each of the validation patients. Proportions of AF at the 5 levels of the index (0, 1, 2, 3, and 4) were calculated and compared to the corresponding values in the calibration set.
Results
The clinical characteristics of patients in the calibration and the validation model are listed in Table 1 . AF occurred in 18.8% and 19.5% of patients in the calibration and validation cohorts, respectively.
Variable | Calibration | Validation | p Value |
---|---|---|---|
(n = 18,517) | (n = 1,378) | ||
AF | 3,486 (18.8%) | 269 (19.5%) | 0.52 |
Age (years) | 62.5 ± 10.9 | 62.3 ± 10.8 | 0.51 |
Caucasian race | 14,738 (81.3%) | 1,010 (73.3%) | <0.001 |
Male gender | 13,276 (71.7%) | 985 (71.5%) | 0.90 |
Ejection fraction (%) | 50 ± 12.4 | 53 ± 12.6 | <0.001 |
Congestive heart failure | 3,103 (16.8%) | 314 (22.8%) | <0.001 |
Diabetes mellitus | 6,651 (35.9%) | 590 (42.8%) | <0.001 |
Hypertension | 14,219 (76.8%) | 1,249 (90.6%) | <0.001 |
Patients with AF were generally older (67.5 vs 61.3 years), were more likely to be Caucasian (87.0% vs 79.8%), were taller (172.3 vs 171.2 cm), were heavier (86.6 vs 85.7 kg), and generally had more preoperative co-morbidities than those who did not have AF. There was no statistically significant difference in BMI between the 2 groups (29.1 kg/m 2 ). Results for the calibration model are listed in Table 2 .
Risk Factor | Postoperative AF | Unadjusted Odds Ratio (95% Confidence Interval) | p Value | |
---|---|---|---|---|
No (n = 15,031) | Yes (n = 3,486) | |||
Age (years) | 61.33 ± 10.86 | 67.52 ± 9.47 | 1.059 (1.055–1.063) | <0.001 |
Caucasian race | 11,759 (79.7%) | 2,979 (87.0%) | 1.70 (1.53–1.90) | <0.001 |
Male gender | 10,731 (71.4%) | 2,545 (73.0%) | 1.084 (0.998–1.177) | 0.057 |
Height (cm) | 171.2 ± 10.39 | 172.3 ± 17.70 | 1.008 (1.004–1.012) | <0.001 |
Weight (kg) | 85.69 ± 18.73 | 86.59 ± 19.69 | 1.002 (1.000–1.004) | 0.018 |
PVD | 1,307 (8.7%) | 440 (12.6%) | 1.52 (1.35–1.70) | <0.001 |
Body mass index (kg/m 2 ) | 29.1 ± 5.6 | 29.1 ± 5.8 | 0.999 (0.992–1.006) | 0.77 |
Body surface area (m 2 ) | 2.03 ± 0.26 | 2.04 ± 0.27 | 1.23 (1.06–1.42) | 0.008 |
Cerebrovascular disease | 1,910 (12.7%) | 634 (18.2%) | 1.53 (1.38–1.69) | <0.001 |
Chronic lung disease | 2,066 (13.7%) | 586 (16.8%) | 1.27 (1.15–1.40) | <0.001 |
Cerebrovascular accident | 1,178 (7.8%) | 346 (9.9%) | 1.30 (1.14–1.47) | <0.001 |
Diabetes mellitus | 5,380 (35.8%) | 1,271 (36.5%) | 1.03 (0.95–1.11) | 0.46 |
Family history of coronary artery disease | 6,102 (40.6%) | 1,286 (36.9%) | 0.86 (0.79–0.92) | <0.001 |
Hypertension | 11,406 (75.9%) | 2,813 (80.7%) | 1.33 (1.21–1.46) | <0.001 |
Immunosuppressive therapy | 364 (2.4%) | 117 (3.4%) | 1.40 (1.13–1.73) | 0.002 |
Last creatinine level (mg/dl) | 1.21 ± 1.08 | 1.32 ± 1.20 | 1.083 (1.049–1.118) | <0.001 |
Renal failure | 859 (5.7%) | 293 (8.4%) | 1.52 (1.32–1.74) | <0.001 |
Dialysis | 242 (1.6%) | 85 (2.4%) | 1.53 (1.19–1.96) | <0.001 |
Smokers | 6,409 (42.6%) | 1,353 (38.8%) | 0.85 (0.79–0.92) | <0.001 |
Previous CABG | 872 (5.8%) | 203 (5.8%) | 1.004 (0.86–1.18) | 0.96 |
Previous valve surgery | 32 (0.2%) | 8 (0.2%) | 1.08 (0.50–2.35) | 0.85 |
Angina | 12,345 (82.1%) | 2,765 (79.3%) | 0.834 (0.761–0.915) | 0.001 |
Heart failure | 2,352 (15.7%) | 751 (21.5%) | 1.48 (1.35–1.62) | <0.001 |
Myocardial infarction | 7,492 (49.8%) | 1,838 (52.7%) | 1.12 (1.04–1.21) | 0.002 |
Cardiogenic shock | 120 (0.8%) | 59 (1.7%) | 2.14 (1.56–2.93) | <0.001 |
Ejection fraction (%) | 50.87 ± 12.49 | 50.17 ± 12.97 | 0.996 (0.993–0.999) | 0.007 |
Left main coronary artery disease | 3,381 (22.5%) | 924 (26.5%) | 1.24 (1.14–1.35) | <0.001 |
Number of diseased vessels | 2.55 ± 0.69 | 2.62 ± 0.64 | 1.15 (1.09–1.22) | <0.001 |
Preoperative intra-aortic balloon pump | 433 (2.9%) | 133 (3.8%) | 1.34 (1.10–1.63) | 0.004 |
Off-pump CABG | 7,096 (47.2%) | 1,616 (46.4%) | 0.966 (0.898–1.040) | 0.36 |
Predicted risk for mortality | 1.86 ± 2.48 | 2.87 ± 3.98 | 1.104 (1.091–1.118) | <0.001 |
Patient age appeared to be the single most important predictor of AF (odds ratio 1.059/year, 95% confidence interval 1.055 to 1.063, p <0.001). In the presence of increasing age, the 3 most consistently predictive variables were height (NRI = 0.049, area under the receiver-operating characteristic curve [AUROC] = 0.006, R 2 = 0.008), weight (NRI = 0.083, AUROC = 0.011, R 2 = 0.013), and PVD (NRI = 0.027, AUROC = 0.002, R 2 = 0.002) ( Table 3 ). The model including age, height, weight, and PVD had an AUROC of 0.680. No other variables significantly increased the NRI, AUROC, or R 2 value of this 4-variable model. This model performed consistently well.
Variable Added | Change in C-Index (Baseline = 0.665) | NRI (p Value) | Change in Nagelkerke R 2 (Baseline = 0.082) |
---|---|---|---|
Caucasian race | 0.004 | 0.032 (<0.001) | 0.004 |
Male gender | 0.002 | 0.019 (0.023) | 0.003 |
Height | 0.006 | 0.049 (<0.001) | 0.008 |
PVD | 0.002 | 0.027 (<0.001) | 0.002 |
Cerebrovascular disease | 0.001 | 0.013 (0.018) | 0.001 |
Chronic lung disease | 0.000 | 0.020 (<0.001) | 0.000 |
Cerebrovascular accident | 0.000 | −0.001 (0.85) | 0.000 |
Diabetes mellitus | 0.000 | 0.002 (0.58) | 0.000 |
Family history of coronary artery disease | 0.000 | 0.000 (1.0) | 0.000 |
Hypertension | 0.001 | 0.011 (0.04) | 0.001 |
Immunosuppressive treatment | 0.000 | 0.004 (0.21) | 0.000 |
Last creatinine level | 0.005 | 0.007 (0.22) | 0.006 |
Renal failure | 0.002 | 0.008 (0.13) | 0.001 |
Dialysis | 0.001 | −0.001 (0.84) | 0.001 |
Smoker | 0.000 | 0.002 (0.63) | 0.000 |
Weight | 0.011 | 0.083 (<0.001) | 0.013 |
Previous CABG | 0.000 | 0.000 (1.00) | 0.000 |
Previous valve surgery | 0.000 | −0.003 (0.07) | 0.000 |
Angina | 0.000 | 0.017 (0.002) | 0.000 |
Myocardial infarction | 0.001 | 0.020 (0.006) | 0.001 |
Cardiogenic shock | 0.001 | −0.001 (0.82) | 0.002 |
Ejection fraction | 0.002 | −0.011 (0.10) | 0.001 |
Left main coronary artery disease | 0.000 | 0.006 (0.22) | 0.000 |
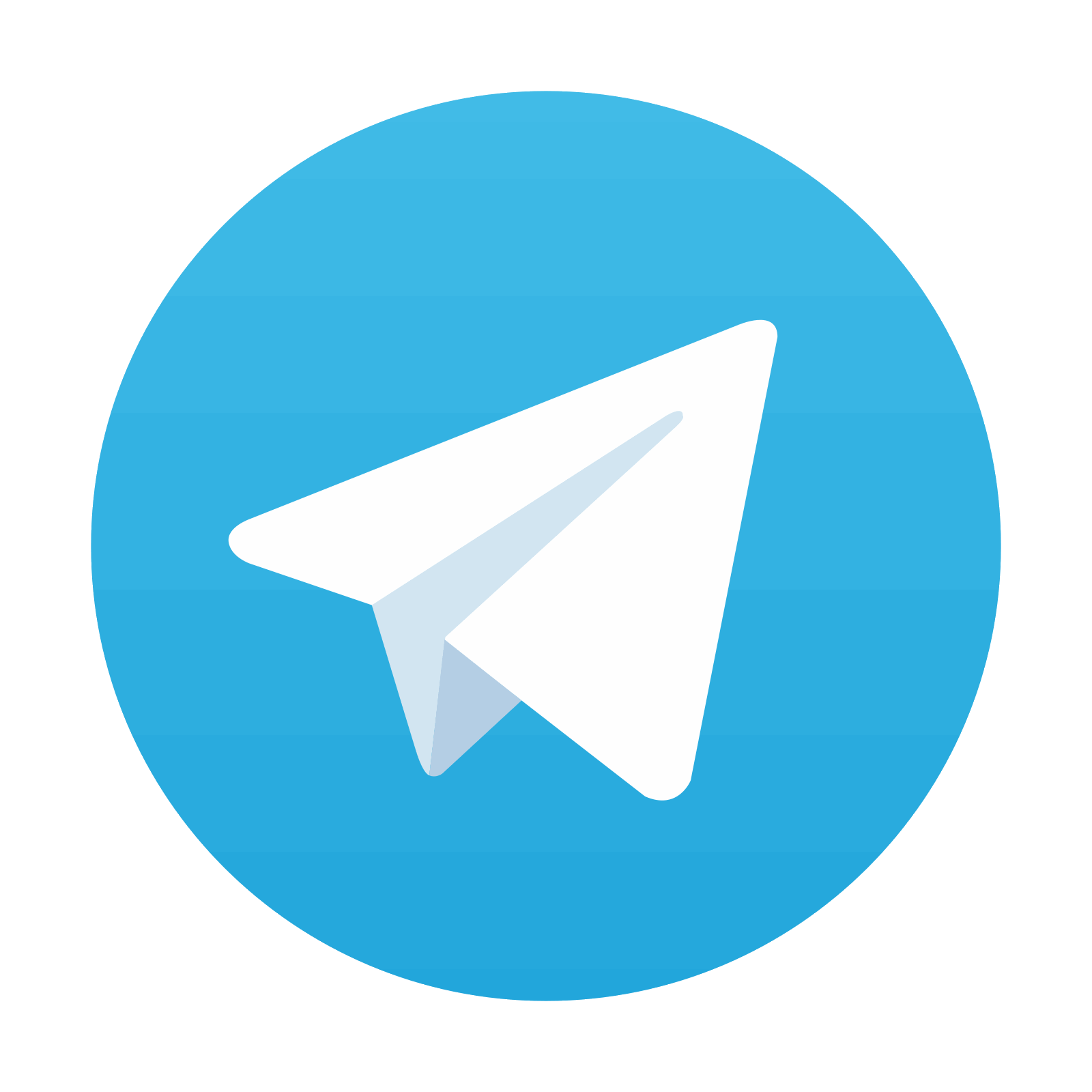
Stay updated, free articles. Join our Telegram channel

Full access? Get Clinical Tree
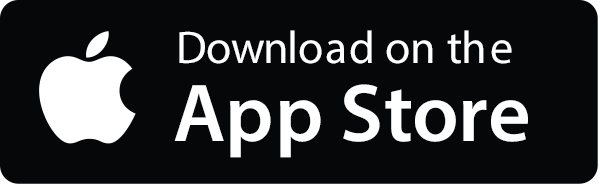

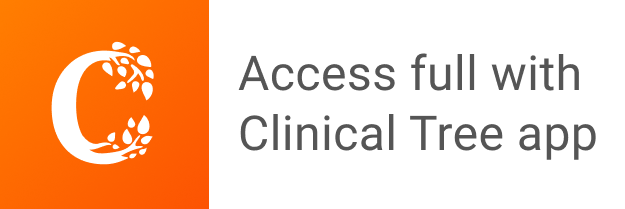