Chapter 2
New Biomarkers of Cardiovascular Disease in Diabetes
Introduction
Diabetes mellitus (DM) is associated with a twofold increase in myocardial infarction (MI) and a twofold increase in stroke [1]. A study by Wannamethee et al. [2] demonstrated that the risk of cardiovascular disease (CVD) and all-cause mortality was proportional to the duration of diabetes. In particular, a mean duration of diabetes of about a decade appears to confer an equivalent risk of CVD to a prior history of MI. In addition, recent work has shown that a history of DM results in six years of life years lost, mostly from CVD [3]. These data highlight the importance of preventing diabetes and identifying patients early to modify the atherosclerotic disease process that is accelerated with duration of diabetes in this population.
To prevent the clinical manifestation of CVD among those with DM, clinical care focuses on optimizing the control of “traditional” risk factors, which include lipids, glycemia, blood pressure, smoking, weight, alcohol consumption, and uptake of exercise. There remains unmodifiable risk from family history, gender, and age. However, 20% of all vascular events occur in patients without any traditional risk factors, necessitating the need for more precise clinical tools that aid clinicians in identifying those at highest risk [4]. To help achieve this goal, there is growing interest in the development and exploitation of new biomarkers.
What Is a Biomarker?
A biomarker was defined by a National Institutes of Health (NIH) working group as “a characteristic that is objectively measured and evaluated as an indicator of normal biological processes, pathogenic processes, or pharmacologic responses to a therapeutic intervention” [5]. Biomarkers are used in a variety of ways, including screening, diagnosis, staging, prognostication, and monitoring of disease.
An example of an established biomarker is the use of an elevated troponin I or T in distinguishing between an acute myocardial infarction and unstable angina in a patient who presents with chest pain. It is important to stress that a biomarker is useful as an addition to a thorough clinical assessment rather than an alternative. All patients with a positive troponin have not necessarily had an acute myocardial infarction and similarly, a negative troponin does not mean that the patient does not have significant coronary artery disease.
A biomarker should meet several criteria to be deemed clinically useful. This is structured around three fundamental questions [6]:
- Is the biomarker measurable?
- Does the biomarker add new information?
- Will the biomarker help the clinician to manage patients?
Additional criteria include cost-effectiveness, safety, and replication of the biomarker in clinical scenarios.
Biomarker discovery is currently undertaken by two complementary processes: knowledge based, in which markers for different stages in a known biological process (e.g., atherosclerosis) are investigated; and an inductive strategy, which is more labor intensive, involving analysis of thousands of often unrelated molecules with respect to a disease stage [6].
How Are Biomarkers Evaluated?
An ideal biomarker should enable the clinician to change patient management and improve outcomes above that of current standard care. To achieve this goal, ideally, it has to hold true against four criteria: risk association, discrimination, calibration, and reclassification [6].
Risk Association
There has to be a statistically significant association between the biomarker and the outcome. When defining the outcome, it is probably more desirable to use “hard” endpoints such as death or myocardial infarction rather than “softer” ones such as coronary artery stenosis. Risk association is described using hazards ratios or odds ratios; however, these do not provide meaningful information for predicting whether or not an individual who is “positive” or has elevated levels will develop the outcome of interest over and above other factors. This is partly because the methods used in defining an abnormal biomarker threshold aim to minimize false-positive rates and as a consequence sensitivity declines.
There are three main methods for defining abnormal biomarker levels [6]: reference limits (usually arbitrarily chosen between the 95th and 99th percentiles) of the distribution of the biomarker values in a healthy population; discrimination limits, which are derived from the biomarker-level distribution curves in populations with and without disease; and identifying a threshold above which the risk of disease escalates significantly. It is clinically attractive to dichotomize biomarkers into either a “positive” or “negative” result, but it is important to bear in mind that certain biomarkers have a continuous relationship with risk, such that the higher the level of biomarker, the higher the risk.
Discrimination
Discrimination is the ability of a test to separate two outcome classes. It is performed by measuring the area under the receiver-operating- characteristic curve (AUC), which plots sensitivity (proportion of patients with the disease with a positive result) on the y-axis against 1-specificity (proportion of patients without the disease with a negative result) on the x-axis. The AUC is normally used in case-control studies and is similar to the c-statistic employed in prospective studies. A value of 0.5 suggests that the biomarker does not help to discriminate between individuals who will and will not develop the disease, whereas a value of 1.0 indicates perfect discrimination.
To put a novel biomarker into perspective, the Framingham risk scores for coronary heart disease give a c-statistic of approximately 0.75. The AUC method is dependent on sensitivity and specificity, so factors that affect these values will influence discrimination; for example, whether the sample population includes a predominantly high- or low-risk cohort.
Calibration
A biomarker should give an indication of predicted future risk that should agree with the actual observed outcome. A good model will have a goodness-of-fit statistic (e.g., Hosmer-Lemeshow) p value of >0.05. Clinically, this is useful as the model can be used to give an estimated 10-year risk for an event and hence identify higher-risk patients who would benefit from interventions in a cost-effective manner.
Reclassification
This is a relatively new concept, but potentially the most clinically relevant as it assesses the ability of a test to reclassify individuals correctly into a different risk category; for example, an intermediate-risk subject into a high-risk subject, or a low-risk subject into an intermediate-risk subject. If this results in a change in clinical decision-making as somebody moves into a category requiring treatment or one that does not, then this is referred to as net clinical reclassification. The ability of the new test to achieve reclassification can be statistically examined by net reclassification improvement (NRI) or integrated discrimination improvement (IDI). The NRI method, which is determined by the proportion of individuals whose risk is correctly escalated or de-escalated, is more useful in primary prevention, where well-accepted categories of risk exist. The IDI estimates the change in predicted probability of an outcome between those with and without the outcome after the biomarker is added to the prediction model. The larger the value of the NRI or the IDI, the better the biomarker.
Categories of Biomarkers of Cardiovascular Disease
The three main subtypes of biomarkers can be summarized as circulating, imaging, or genetic biomarkers, as in Figure 2.1, by linking them to different stages in the disease processes [7].
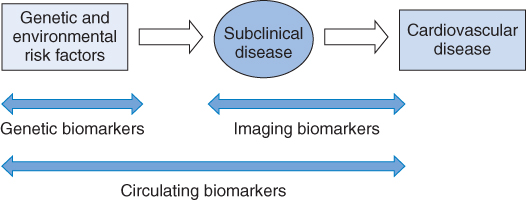
Figure 2.1 Progression from risk factors to cardiovascular disease. (Source: Wang 2011 [7]. Reproduced with permission of Wolters Kluwer Health.)
This chapter does not intend to be a comprehensive overview of all biomarkers in cardiovascular disease, but instead to provide a summary of the key ones that have the support of a large body of evidence and are hence more likely to have a clinical utility. We will discuss in turn each biomarker subtype briefly described above and present the evidence base for use of these novel biomarkers in cardiovascular disease, relating this as much as possible to the diabetic population.
Circulating Biomarkers
The National Lipid Association evaluated the use of selected biomarkers in clinical practice as either tools to improve risk assessment or markers to adjust therapy once a decision to treat had been made [8]. During the course of this chapter we will discuss some of the key biomarkers from Table 2.1 as well as other novel and emerging biomarkers that may be used in clinical practice.
Table 2.1 Summary and recommendations for measurement of inflammatory markers and advanced lipoprotein/subfraction testing in initial clinical assessment and on-treatment management decisions. (Source: Davidson et al. 2011 [8]. Reproduced with permission of Elsevier.)
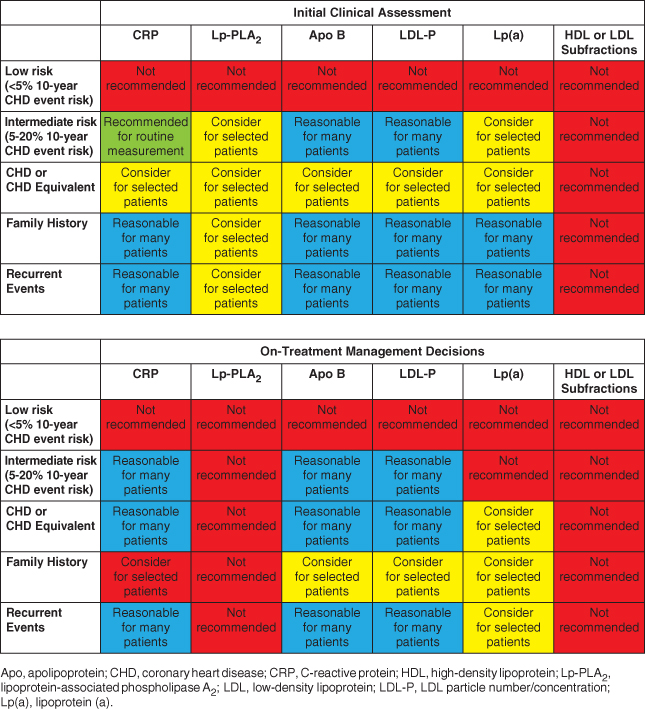
CRP
Pathophysiology
Considerable in vitro and clinical data link inflammation to cardiovascular disease. Hence, there has been considerable interest in a blood-based test that provides information on subclinical inflammation. CRP is a nonspecific acute phase protein produced by hepatocytes and is the most studied biomarker of inflammation and CVD risk. It is a downstream marker of inflammation with levels largely derived from Interleukin-6 mediated hepatic production, and recent genetic studies [9] do not suggest a causal role for CRP. In most cases, CRP indicates high-sensitivity CRP (hs-CRP), which detects levels of low-grade inflammation below the level of standard assays and is the assay that is recommended for use in clinical practice. Hs-CRP can be elevated in other inflammatory states, so its clinical utility is debatable in the setting of an active infection, malignancy, or chronic inflammatory disease for CVD risk prediction. In addition, although it is widely accepted that inflammation plays a role in atherosclerosis, there is little evidence from randomized clinical trials to suggest that CRP itself is directly a contributor to the atherogenic process. This being said, CRP via activation of complement may increase infarct size during ischemia. Studies suggest that CRP inhibitors used in animal models of ischemia may reduce infarct size, which is the only direct evidence to date for a direct causal role of CRP [10].
Technique and Measurement
Hs-CRP assays detect concentrations of CRP below 3 mg/L [11]. They are the assays used to assess cardiovascular risk because they are able to quantitate CRP within the range normally seen in asymptomatic patients (<3 mg/L). A statement from the Centers for Disease Control and Prevention and the American Heart Association (CDC/AHA) provides some guidance on the use of serum hs-CRP to estimate CVD risk [12]:
- Low-, average-, and high-risk values can be defined as <1, 1 to 3, and >3 mg/L, which also correspond to approximate tertile risk values.
- A value above 10 mg/L should initiate a search for a source of infection or inflammation.
- Due to the variability of measurements in an individual, the average of two assays, fasting or nonfasting, and optimally obtained two weeks apart provides a more stable estimate than a single value.
Population Studies
One of the earliest studies to demonstrate an association between hs-CRP and cardiovascular disease was the Women’s Health Study (WHS) [13], which had 28,263 apparently healthy postmenopausal women enrolled in it. The cohort was monitored prospectively for future risk of incident vascular events. In this study, several putative markers of risk were assessed and hs-CRP was found to be more predictive of CVD events, outperforming homocysteine, lipoprotein(a), and LDL-C. However, establishing an independent association does not necessarily improve clinical decision-making or target people more appropriately as likely to benefit from therapeutic interventions. The value of hs-CRP with regard to reclassification is uncertain. Addition of hs-CRP to standard risk models in the WHS reclassified 20% of intermediate-risk individuals. Approximately three-quarters of these individuals were reclassified down to low risk. Only 4% of the intermediate risk group were reclassified from intermediate to high risk. The NRI in this study was of modest magnitude (5.7%). This has been incorporated into the Reynolds risk score.
In the largest study to date, the Emerging Risk Factors Collaboration (ERFC) meta-analysed individual records of 160,309 people without a history of vascular disease from 54 long-term worldwide prospective studies, comprising 1.31 million person-years at risk and nearly 28,000 fatal or nonfatal coronary heart disease (CHD) outcomes [14]. Log-transformed hs-CRP concentrations were linearly associated with most established risk factors and several inflammatory markers, including interleukin-6. Log hs-CRP concentrations once adjusted for age and sex were also strongly associated with the risk of coronary heart disease (risk ratio [RR] 1.37, 95% CI 1.27–1.48), ischemic stroke (RR 1.44, 95% CI 1.32–1.57), vascular mortality (RR 1.71, 95% CI 1.53–1.91), and even nonvascular mortality (RR 1.55, 95% CI 1.41–1.69). However, adjustment for several conventional risk factors and plasma fibrinogen resulted in considerable weakening of associations of hs-CRP concentration with risk of coronary heart disease (RR 1.23, 95% CI 1.07–1.42). Such adjustment also attenuated associations of hs-CRP concentration with ischemic stroke (RR 1.32, 95% CI 1.18–1.49) and deaths from nonvascular diseases (RR 1.34, 95% CI 1.20–1.50). It can therefore be concluded from this study that hs-CRP associations with ischemic vascular disease depend considerably on conventional risk factors and other markers of inflammation (Figure 2.2). Furthermore, the nonspecific associations between CRP and a range of vascular and nonvascular endpoints make it unlikely that CRP is a causal factor for CHD.
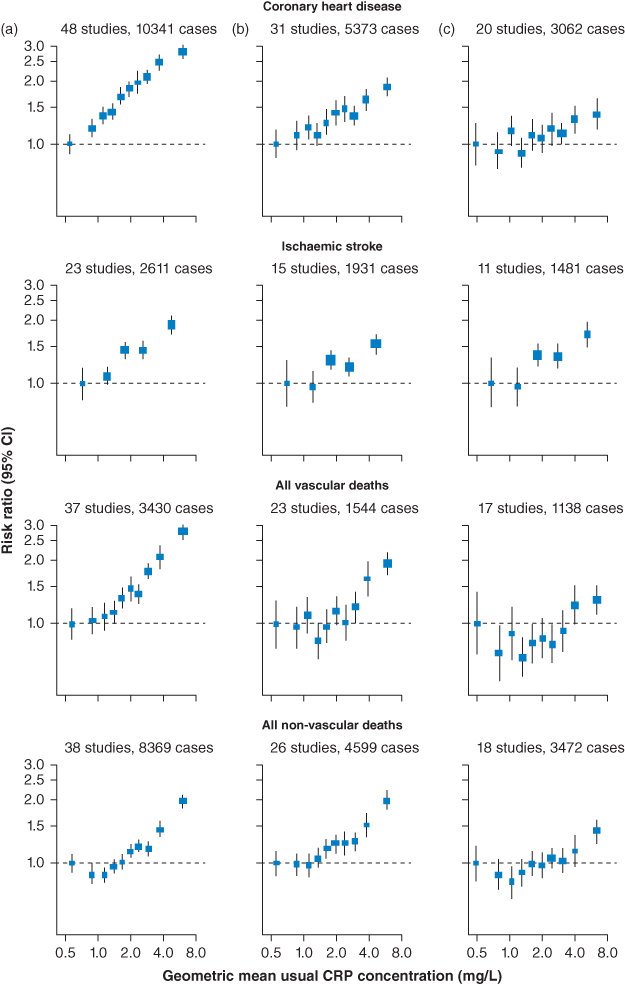
Figure 2.2 Risk ratios for coronary artery disease, stroke, and nonvascular outcomes by quantiles of C-reactive protein (CRP) concentration, with different degree of adjustment for potential confounders. Adjusted study-specific loge risk ratios were combined by use of multivariate random-effects meta-analysis. The adjustments were age, sex, and study only (a); age, sex, study, systolic blood pressure, smoking, history of diabetes, body-mass index, concentrations of loge triglycerides, non-HDL cholesterol, and HDL cholesterol, and alcohol consumption (b); and (a) plus (b) plus fibrinogen (c). Studies with fewer than 10 cases of any outcome were excluded from the analysis of that outcome. Error bars represent the 95% CIs, calculated using floating absolute risk technique. The sizes of the boxes are proportional to the inverse of the variance of the risk ratios. (Source: ERFC 2010 [14]. Reproduced with permission of Elsevier.)
Hs-CRP may also be a useful measure of residual risk among those who are aggressively treated with contemporary therapy. In the Pravastatin or Atorvastatin Evaluation and Infection Therapy-Thrombolysis in Myocardial Infarction (PROVE-IT-TIMI) trial, 4,162 ACS patients randomly assigned to intensive (Atorvastatin 80 mg) therapy had a lower risk of CVD compared to standard (Pravastatin 40 mg) statin therapy by virtue of having achieved a lower LDL-C level [15]. However, observational data from that study suggested that even among those subjects achieving a very low LDL-C level of <70 mg/dl, risk varied considerably in part based on hs-CRP levels, with those <2 mg/L having a lower risk of CVD than those >2 mg/L, and with the lowest rates observed among those with hs-CRP levels <1 mg/L. Based on this study, monitoring of CRP might offer a way to identify high-risk individuals for more intensive risk-factor control. A study by Ray et al. [16] in the same population showed that in both statin groups “on treatment CRP levels” correlated with the number of uncontrolled risk factors present, therefore among those with elevations in CRP, more intensive control of weight, blood pressure, triglycerides, glucose, raising HDL, and stopping smoking would be expected to lower CRP and potentially used as a motivational tool for patients.
Clinical Use
The Reynolds risk score (http://www.reynoldsriskscore.org) incorporates CRP measurement and family history in its scoring for CVD, components that are not used in the Framingham risk score. In studies by Ridker et al., the Reynolds risk score reclassified 40% to 50% of women [17] at intermediate risk into higher- or lower-risk categories and similarly, for men [18], reclassified approximately 20% of men into higher- or lower-risk categories when compared with the traditional Framingham risk score. Therefore, both of these studies show that a prediction model that incorporates hs-CRP and family history modestly improves global cardiovascular risk prediction. However, among high-risk individuals such as those with diabetes who are already candidates for treatment, it is unclear what these additional measurements would offer.
Currently, the ESC and the AHA offer no official recommendation with regard to the routine measurement of hs-CRP. However, the National Lipid Association panel recommends that in patients with intermediate risk (520% 10-year risk), hs-CRP should be measured routinely in men >50 years of age and women >60 years of age, given its capacity to enhance risk prediction, especially when used with the Reynolds risk score [8].
Apolipoproteins
Pathophysiology
Apolipoproteins are multifunctional proteins that serve as templates for the assembly of lipoprotein particles. They also maintain the structure and direct metabolism of lipoproteins by binding to membrane receptors and the regulation of enzyme activity. The most important clinical application of apolipoprotein measurements is in the assessment of those individuals where atherogenic risk may not be accurately captured by LDL-C alone; for example, in genetic dyslipidemia and for cardiovascular risk assessment among those with insulin resistance. Most of the data relating to cardiovascular risk revolves around ApoB and ApoAI.
The plasma concentration of ApoB is positively associated and that of ApoAI inversely associated with cardiovascular risk [19]. The ApoB/ApoAI ratio has been interpreted similarly to that for TC/HDL-C as a reflection of the pro-atherogenic potential of the fractions in total cholesterol versus the anti-atherogenic properties of HDL, although more accurately this ratio reflects the non-HDL-C/HDL-C ratio. There is evidence that ApoB is a better marker of cardiovascular risk than TC or LDL-C. This is in part intuitive, as TC includes HDL-C, which has an inverse relationship with CHD risk. LDL-C incompletely captures CHD risk in particular among those with insulin resistance syndromes where VLDL-C and IDL-C levels are high with relatively normal levels of LDL-C.
Technique and Measurement
Tests for either ApoB or ApoAI do not require fasting samples. The reference range for Apo B is 60–120 mg/dL and for ApoAI, 90–200 mg/dL. The ranges for both assays are age and sex dependent.
Population studies
The Apolipoprotein-Related Mortality Risk Study (AMORIS) [20] included 175,553 individuals followed up for approximately 65 months. The relative risk of fatal myocardial infarction associated with 1 standard deviation (SD) increase in ApoB concentration was approximately 2.7, which increased to 3.6 in individuals younger than 70 years. The ApoB/AI ratio was associated with an even higher relative risk of almost 4. However, this study only adjusted for age and sex and HDL-C levels were estimated from ApoA levels.
Since then, several individual studies of subjects free from CVD at baseline have failed to show a superiority of Apo B over non-HDL-C or Apo A over HDL-C. These include the MONICA/KORA Augsburg study of men and women followed up for 13 years, which demonstrated that the predictive power of ApoB/AI and TC/HDL-C was comparable [21]. Similar findings were observed in the Framingham cohort [22] and the WHS [23].
The largest prospective study to date included individual participant data on 302,430 subjects without initial vascular disease from 68 long-term prospective studies with 2.7 million person-years of follow-up (ERFC) [24]. This study standardized a range of lipid parameters and compared the values of non-HDL-C and HDL-C with ApoB and ApoAI in the assessment of vascular risk. After adjustment for standard CV risk factors, in fully adjusted models the associations with risk were similar for both the groups. The hazard ratios for CHD were 1.50 (95% CI, 1.38–1.62) with the ratio of non-HDL-C/HDL-C and 1.49 (95% CI, 1.39–1.60) with the ratio of Apo B/Apo AI. The group concluded that lipid assessment in vascular disease can be measured by either cholesterol levels or apolipoproteins, depending on what is cost-effective or more efficient to obtain. More recently, work from the same group showed that the addition of apolipoproteins to traditional risk parameters for CVD risk prediction only yielded modest improvement in the model’s discrimination, with C-index change of 0.0006 (95% CI, 0.0002–0.0009) for the combination of ApoB and A-I. There was also a modest change in net reclassification. Additional testing with a combination of ApoB and A-I reclassified 1.1% of people to a 20% or higher predicted CVD risk category and, therefore, in need of statin treatment under Adult Treatment Panel (ATP) III guidelines [25].
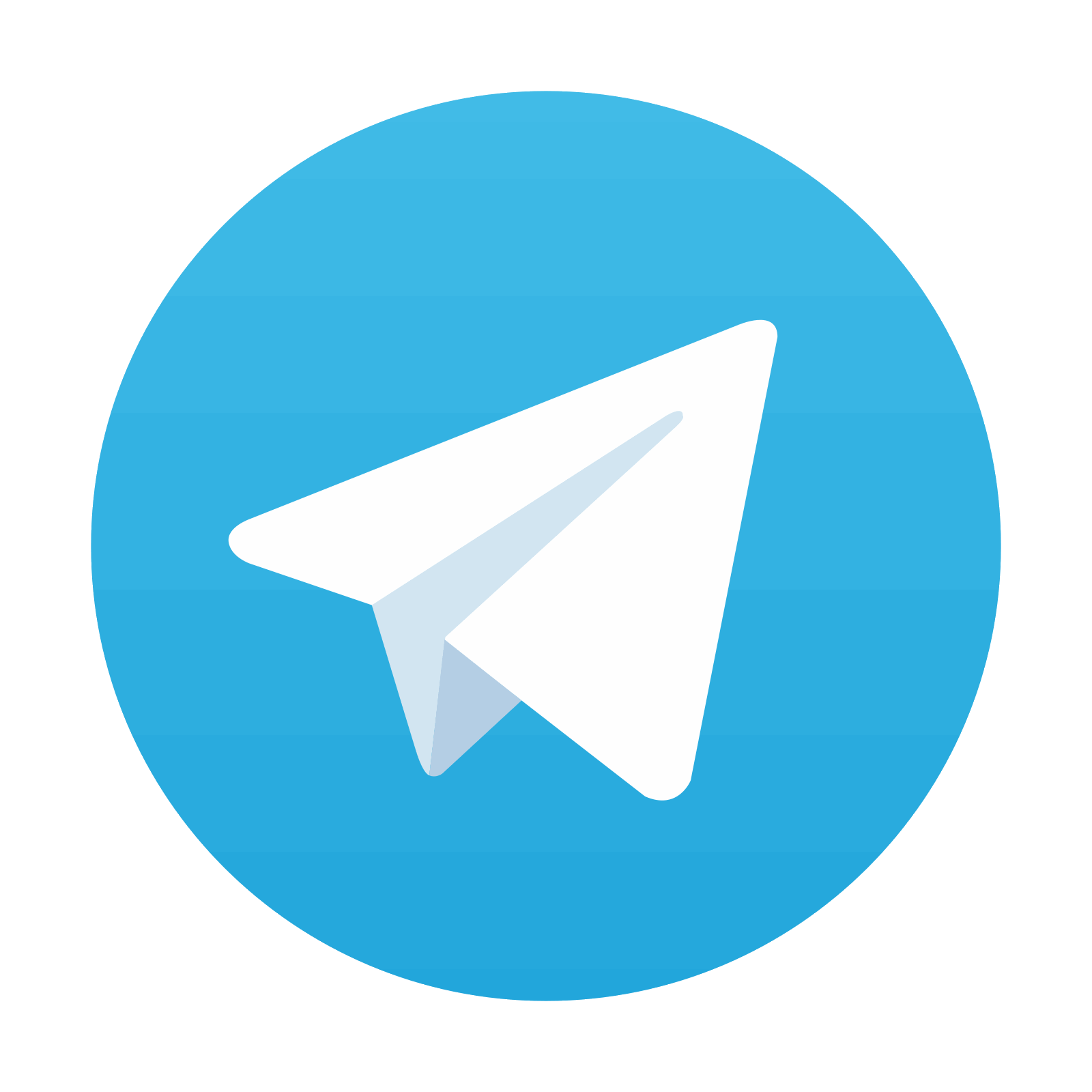
Stay updated, free articles. Join our Telegram channel

Full access? Get Clinical Tree
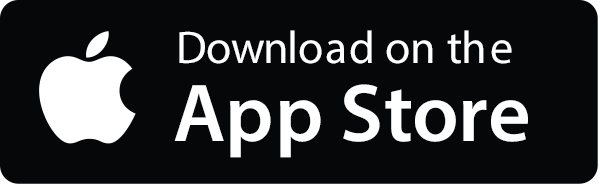
