Introduction
The human genome comprises approximately 3.2 billion base pairs. With the exception of identical twins, each human being has a unique DNA sequence. There are at least 10 million locations in the genome where DNA sequence varies between individuals. These locations are referred to as “polymorphic” when at least two variants (also known as “alleles”) are present at a frequency greater than 1%. Most human diseases are the result of the interplay between these genetic polymorphisms and environmental exposures. The first step in any investigation of the genetic causes of a disease or phenotype is to determine the relative importance of these two causes of the disorder among the population of interest. To begin this process, one must determine the heritability of the disease of interest. Heritability is defined as the percentage of phenotypic variation that is due to variation in genetic factors. Often the first step is to determine if the trait, disease, or phenotype aggregates in families, but this will not prove that the trait of interest is genetic because traits can aggregate in families for purely environmental reasons, such as cigarette smoking, or because the prevalence of the trait is high, such as obesity. The most direct way to estimate the contribution of genetic variation to a disease is to measure heritability. Heritability can be estimated using families. For example, in twin studies a greater concordance of the phenotype between identical (monozygotic) twins than fraternal (dizygotic) twins can provide evidence of heritability of that phenotype. For lung disorders, heritabilities range from 20% to 90% depending on the type of lung disease, the mode of inheritance, and the degree of environmental influence.
There are two primary types of genetic disorders, monogenic (due to variation in a single gene) or complex (due to variation in multiple genes). Monogenic disorders demonstrate high heritability, segregate in families in a predictable way, and are caused by variation in a single major gene with less obvious environmental influence. The single gene usually has specific variation in the coding region of the gene that leads to an abnormal protein that causes an obvious clinical phenotype. Often the phenotype has multiple components, suggesting multiple effects of the gene variant(s). This is called “pleiotropy,” in which one variant has many effects. There are currently over 10,000 monogenic disorders that have been identified and are characterized in the Online Mendelian Inheritance in Man ( http://www-ncbi-nlm-nih-gov.easyaccess1.lib.cuhk.edu.hk/sites/entrez?db=omim ). Positional cloning (linkage mapping followed by association mapping; described later) has been the primary means of the identification of these genetic variants until recently. With the completion of the Human Genome Project and the rapid advancement of genotyping technologies, attention has turned to identification of genetic variation associated with complex genetic disorders. Those efforts initially used positional cloning but now primarily rely on genetic association studies.
In contrast to monogenic disorders, complex genetic disorders are caused by variation in multiple genes and multiple environmental exposures, with each genetic variant having a much smaller effect than those seen in monogenic disorders. Because of the multiple gene-gene and gene-environment interactions, there is no obvious mendelian mode of inheritance in families for complex traits. One of the most prominent hypotheses for the genetic basis of common disease is the common disease/common variant hypothesis. This hypothesis suggests that key genetic determinants of common diseases have a relatively high allele frequency (i.e., 5% to 40%) and modest effect sizes. Given the modest effect sizes (odds ratios on the order of 1.1 to 1.4), large sample sizes are necessary to identify the genetic variants associated with complex traits despite the high allele frequency expected in these disorders. It is likely that there is a range of allele frequencies that predispose to complex diseases, with a corresponding range of effect sizes, but large-scale studies to evaluate the evidence for rare variation as a contributor to complex disease are only beginning to emerge.
The dichotomy described earlier between monogenic and complex disease is somewhat artificial because the clinical phenotype of many monogenic disorders varies as a result of the specific mutation present, other modifier genes, and environmental exposures. As genes for complex traits begin to be identified, their role in monogenic disorders is also being elucidated.
Scope of the Problem
Some complex genetic disorders, such as age-related macular degeneration, are oligogenic , in which a small number of genes, three to five, explain the bulk of the clinical phenotype. However, for most complex traits, literally hundreds of genes with small effects are likely involved in disease causation. Thus a series of challenges have faced complex trait geneticists in the genome era of medicine.
The field of human genetics has continued to expand as the type of genomic variation that can be measured expands. Parallel advances in data analysis strategies are necessary to ensure efficient and valid inferences based on the ever-increasing volume of data that can be collected on large numbers of individuals. This cyclical pattern of advancement has been typical of the last several decades and is likely to be typical going forward. For example, initial problems relating to genotyping reliability and completeness for common variants (those with frequency > 5% in a given population) that were present at the time of release of the initial sequence of the genome have been largely resolved, as have the methods necessary to detect and control for population stratification (confounding by allele frequency differences in cases and controls, discussed later) and to account appropriately for the hundreds of thousands or millions of tests conducted in a genome-wide association study. Since 2010, large efforts have been focused on resequencing technologies, which sequence the same site in multiple individuals to capture sequence variation and thus capture uncommon and rare variation (frequency ≤ 5%). As we are able to measure a larger variety of genomic data (e.g., transcriptomic and epigenetic data) on ever-increasing numbers of individuals, the major analytic challenges will be in developing methods for integration of the different types of data. For all types of genetic variation, the ability to determine if genetic effects are real or not requires replication of results in independent populations, a process that can be difficult with the presence of phenotypic heterogeneity across populations. In particular, varying genetic backgrounds and environmental influences can result in variability in the effects of genetic variants across populations. Finally, the ultimate challenge of finding and verifying the functional variants in putative disease genes is still a laborious process without a clear-cut methodology for success.
Potential Impact of Human Genetics
Genetics has the potential, because of its hypothesis-free nature, to identify novel mechanisms of disease pathobiology and hence to identify novel targets for a therapeutic intervention or disease prevention. In addition, genetics has the potential to predict specific subgroups of patients with a different clinical course or response of their disease, or differences in treatment. Finally, genetics has the potential to allow for early detection of susceptible individuals at risk for a specific disease phenotype or to allow avoidance of environmental factors that are known to cause the disease or to institute preventive therapy before disease develops. These genetic insights are still just beginning to be applied, and it will take time for genetics to become routinely used at the bedside.
Molecular Characterization of Genetic Variation
Molecular genetics is elegant in its simplicity. Just four base pairs (two purines [adenine and guanine] bind to two pyrimidines [thymine and cytosine]) code for 20 amino acids that form the molecular building blocks of complex proteins. However, the assemblage of inherited genes (genotypes), control mechanisms, resultant proteins, and posttranslational modifications have the capacity to create a complex panoply of unique biologic, physiologic, or visible traits of an organism (phenotypes). The relationship between these rather simple molecular characteristics and the vast array of complex phenotypes is, in part, explained by a number of seminal discoveries that were made more than 50 years ago.
Gregor Mendel was the first to demonstrate that discrete traits could be inherited as separable factors (genes) in a mathematically predictable manner. Mendel’s laws describe the relationship between genotype and phenotype and established the concept that each gene has alternative forms (alleles). Charles Darwin made the observation that evolution represents a series of environmentally responsive “genomic” upgrades. Thomas Morgan established the concept of linkage by using Drosophila to discover that genes were organized (and inherited) on individual chromosomes, and that genetic material was recombined or exchanged between maternal and paternal chromosomes during meiosis and that the frequency of recombination could be used to establish the relative genomic distance between genes. However, it was not until 1944 that Avery, MacLeod, and McCarty while working with Pneumococcus discovered that DNA was identified as the essential molecule that transmitted the genetic code. The double-helix structure of DNA was discovered by Watson, Crick, Chargaff, Franklin, and Wilkins in 1953, and over the next 50 years genetics assumed a central role in understanding the biologic and physiologic differences between and among species and between states of health and states of disease. In aggregate, these seminal discoveries led to a number of fundamental principles in molecular genetics that provide the basic mechanisms that link the four base pairs (adenine [A] binding to thymine [T] and guanine [G] binding to cytosine [C]) to health and disease.
Genomic Maps
Over the past several decades genomic maps have evolved from karyotypes (microscopic visualization of chromosomes during metaphase) to restriction enzyme sites to genetic maps to maps with specific base pair sequence. In fact, to date there are hundreds of vertebrate, invertebrate, protozoan, plant, fungal, bacterial, and viral genomes that have been sequenced and are available on the National Center for Biotechnology Information (NCBI) Web site ( www.ncbi.nlm.nih.gov ). These genomic maps have not only been essential for identifying which genes and sequence changes cause disease or enhance the risk for adverse outcomes, these species-specific maps have also led to a very clear understanding of molecular evolution and have provided essential tools for understanding aspects of molecular biology. The construction of these genomic maps is based on the observation that the sequence of DNA is different from one organism to the next within the same species, and these allelic/sequence differences have been exploited to develop a number of commonly used DNA markers to create genomic maps ( Table 3-1 ).
|
Genetic maps are based on the frequency of recombination events, defined as a specific form of exchange of genetic material between the maternal and paternal chromosomes during meiosis. Although Mendel’s second law states that traits (or genes) are inherited independently, we now know that some genes do not segregate independently because they are on the same chromosome. Humans have 24 linkage groups, corresponding to the 22 autosomes, plus X and Y chromosomes. Genes on the same chromosome that are closer together are more likely to be inherited together (linked) than genes that are farther apart, which may demonstrate independent assortment. In general, the greater the distance between genes on the same chromosome, the higher the recombination frequency ( Fig. 3-1 ). Thus the recombination frequency represents a measure of genetic linkage and is the fundamental event used to create a genetic linkage map. The unit of measurement for genetic linkage maps is the centimorgan (cM), with 1 cM equivalent to a recombination frequency of 1% (one recombinant event per 100 meioses). Although recombination frequencies vary across the chromosome, in general, at least for the human genome, a recombination frequency of 1% is equivalent to approximately 1 million base pairs. Genetic maps are constructed by identifying the number of recombinant events observed in parental meioses and are dependent on several factors: (1) the meioses observed, (2) the heterozygosity of the marker, (3) the physical distance between markers, and (4) the likelihood of recombination at that site. Genetic maps use a rather indirect method to estimate the order of genes and the relative distance between genes.

Genetic maps are routinely used in family-based linkage studies to identify the general location of genes that influence human traits and conditions. Highly polymorphic markers can be used as tags to identify regions of DNA that are linked with a disease locus in families. These regions (often 20 to 40 cM in length) of DNA then serve as the targets to interrogate via association studies. However, a genetic linkage map should not be confused with a physical map of the genome because genetic maps are based on the rate of recombination, not the physical distance, between markers, although these two features are related.
In contrast to a genetic map, a physical map describes the physical location of genes and the physical relationship between genes on each chromosome. Although the gene order from a genetic map and a physical map should theoretically be the same, the relative distance between genes may be quite different when comparing a genetic map (based on recombination frequency) to a physical map (based on genetic distance along the chromosome). The reason for this discrepancy is that the recombination rate across chromosomes is not constant. However, genetic maps provided the first framework for the construction of physical maps.
Although we typically think of physical maps as sequence based, many years before sequence-based maps became available, investigators relied on lower-resolution cytogenetic (chromosomal) and radiation hybrid maps. Cytogenetic maps are based on chromosomal banding patterns and have been used to locate genes in patients with chronic granulomatous disease, Duchenne muscular dystrophy, and fragile X syndrome.
Two major breakthroughs at the end of the 20th and beginning of the 21st centuries changed human genetics forever—the sequencing of the human genome and the creation of the International HapMap Project to identify the common sequence differences and similarities between individuals. With the sequencing of the human genome, investigators for the first time had a detailed road map of the human genome that identified the genes, regulatory units, and noncoding sequence with a very high degree of resolution. Of the 3.2 billion base pairs in the human genome, less than 1% uniquely identify each human being. There are approximately 12 million single nucleotide polymorphisms (SNPs) in the human genome. These are single base pair changes that result in allelic variation at a locus. Although only a small proportion of these SNPs will result in amino acid changes, these SNPs provide some of the genetic diversity that underlies the variable susceptibility to environmental stimuli and the variable risk for disease development and progression. Although the initial HapMap project focused on populations from African, Asian, and European ancestry, more recent work expanded the collection to include a wide variety of ancestry groups. Because the HapMap project was focused on common variation, the 1000 Genomes Project was developed to catalog uncommon and rare variation among human populations using resequencing. Like the HapMap project, this publically available resource provides the empirical data necessary for scientists to design disease-specific studies aimed at understanding the role of uncommon and rare variation.
In aggregate, the human DNA sequence and the shared genetic patterns between individuals have enabled geneticists to define the organization of genetic variants on chromosomes and the common inheritance of genetic variants. These developments have provided the polymorphic markers and the regions that are tagged to these markers to facilitate the identification of regions and genes that contribute to risk for disease. Consequently, real progress is now being made in identifying common and rare genetic variations that contribute to complex diseases such as asthma, age-related macular degeneration, type 2 diabetes, and prostate cancer.
Comparative Genomics
Because DNA structure and protein functions are often conserved through evolution, the use of model organisms can enhance the efficiency of gene discovery and can provide insights into biologic responses to endogenous and exogenous forms of stress. Although genes present in humans often have counterparts in other species, the homology between gene and chromosomal structure across species does not necessarily lead to conserved protein function. However, the conservation of gene sequence and chromosomal structure in different organisms has resulted in accelerated gene discovery, insights into human biology, and a data-driven understanding of evolution. Despite this excitement, the field of comparative genomics is at a very early stage of development and is dependent on evolving databases, like Gene Ontology functional classifications, to facilitate these cross-species comparisons.
Comparative genomics is a powerful approach to searching for disease-causing genes. Identifying similar regions of DNA associated with concordant phenotypes in multiple species enhances the confidence that a gene causing that disease resides in that locus. The overlapping DNA for concordant phenotypes between species can be used to narrow the region of interest substantially. Because there are approximately 340 known conserved segments between mice and humans, a mouse region of interest associated with a trait can be used to narrow the human region of interest associated with a disease. Additionally, the genomes of model organisms, such as mice, flies, worms, or yeast, can be manipulated through genetic engineering (resulting in deficiency or overexpression of a gene, or controlled expression of a human allele) to understand the function of a specific gene. For example, the importance of the Toll-like receptors in innate immunity in mammals was discovered as a direct result of the observation that a defective receptor in flies caused them to be much more susceptible to Aspergillus fumigatus . The importance of this finding is clearly illustrated in the variations in the Toll-like receptors that alter the response to microbial pathogens and modify the risk for developing a variety of diseases that are associated with innate immunity. The ease with which we can observe and apply knowledge across model systems should be exploited so that we can efficiently understand the biologic and clinical importance of key regulatory genes.
Public Databases
If compiled in books, the data produced in defining the human genome would fill 200 volumes, each the size of a 1000-page phone book. Reading it would require 26 years working around-the-clock. Although new tools are being developed to analyze, store, and present the data from genome maps and sequences, several databases presently exist that can be accessed through the Internet.
Important lessons can be learned from a detailed consideration of the characteristics of identified mutations in mendelian diseases using online resources such as the Online Mendelian Inheritance in Man, the Human Gene Mutation Database, and LocusLink. For example, the data on the relative frequency of types of mutations underlying disease phenotypes indicate that mendelian disease genes most often have alterations in the normal protein-coding sequence. For general information on accessing sequence information, several available reviews provide details on available databases and searching strategies. The NCBI is responsible for the final and reference assembly of the human genome. Each DNA sequence is annotated with sequence features and other experimental data, including location of SNPs, expressed sequence tags, and clones. Up-to-date genetic sequence information can be obtained from Ensembl ( http://www.ensembl.org ), the University of California at Santa Cruz Genome Browser, and the NCBI’s GenBank. The NCBI’s Map Viewer provides a tool through which genetic maps and sequence data can be visualized and is linked to other tools such as Entrez, the integrated retrieval system providing access to numerous component databases. The database of Single Nucleotide Polymorphisms at the NCBI allows the user to search for SNPs within a region of interest ( http://www-ncbi-nlm-nih-gov.easyaccess1.lib.cuhk.edu.hk/SNP ). The HapMap ( http://hapmap.ncbi.nlm.nih.gov.easyaccess1.lib.cuhk.edu.hk/ ) and 1000 Genomes ( http://www.1000genomes.org/ ) databases provide raw and summary genotype and linkage disequilibrium data about common and rare genetic variation across several racial and ethnic groups.
Genetic Epidemiology
Positional Cloning
Advances in our understanding of the variation across the human genome have allowed wide application of the positional-cloning approach to identification of genetic variants that contribute to phenotypes of interest. Positional cloning refers to identification of a chromosomal position that is related to the phenotype based on scanning the genome for a relationship between each locus and the phenotype, rather than relying on the known biochemical properties of a gene to identify it as a candidate for being related to the phenotype. The first approach to positional cloning relied on linkage analysis, most often followed by association analysis; since 2007, genome-wide association analyses have been the standard positional-cloning approach. Whole-exome resequencing studies are now feasible for sample sizes in the hundreds, and whole-genome resequencing studies are rapidly decreasing in cost.
Linkage Studies
Linkage analysis encompasses a group of statistical methods to examine the inheritance pattern of DNA markers within families to determine if there is a relationship between a particular region of the genome and a phenotype of interest. Most linkage studies have been based on short tandem repeat (repeats of a short sequence of nucleotides) or SNP markers distributed through the genome.
Linkage analyses use family data, which can be made up by a wide range of pedigree structures from extended pedigrees to affected sibling pairs. There are two broad types of linkage analysis, parametric and nonparametric; both rely on the coinheritance of disease alleles with genetic markers used in the analysis. When a mutation arises on a particular chromosome, initially there is a large shared segment of DNA and hence linkage disequilibrium around it. With each subsequent generation, this region of linkage disequilibrium becomes smaller as a result of meiotic recombination. The basic approach in parametric linkage analysis is to determine if alleles at a genotyped marker segregate with the alleles at a putative disease locus together more often than one would expect by random assortment, or chance. This can be assessed by comparing the frequency of recombinant chromosomes in which a crossing over event has rearranged the parental chromosomes to the frequency of nonrecombinant chromosomes. When two loci are linked, parental chromosomes are more common than recombinant chromosomes. The strength of the linkage between a marker and a putative disease locus is expressed as the recombination fraction. Parametric linkage analyses require that a particular genetic model be specified. Thus the approach is ideal for classic mendelian, monogenic disorders but is less well adapted for complex traits, where these parameters are often not known. Nonparametric linkage analysis refers to a group of analysis methods that, in contrast to parametric linkage analysis, do not require assumptions about a particular form of inheritance. The general approach for nonparametric linkage analysis is to contrast observed allele sharing between affected relatives to that expected given their relationship (e.g., siblings) at a given locus. Regions that show statistically significant excess sharing among affected relatives are regions that may harbor loci important for the phenotype of interest. This nonparametric approach has been combined with genetic association within the linked region to identify disease susceptibility genes for asthma, Crohn disease, and pulmonary fibrosis.
Linkage results are usually expressed as an LOD score, which is a function of a statistical test for linkage. LOD scores are log of base 10 of the odds that the loci are linked, and their distribution depends on the study design.
Association Studies
Genetic association studies are the most commonly used study designs to find disease genes in complex traits. Association studies can be used in conjunction with linkage studies, de novo with candidate genes or genome wide association studies (GWAS). There are two basic types of study designs used for genetic association studies, the case-control study and the family-based study; both types rely on the concept of linkage disequilibrium between the alleles at a genotyped marker(s) and the disease allele(s).
When two SNPs are on separate chromosomes, they will segregate randomly (i.e., carrying the minor allele at SNP A does not affect your chances of carrying the minor allele at SNP B). If, on the other hand, the alleles at the SNPs are in linkage disequilibrium, with little or no recombination between them, the genotype at SNP B can serve as a surrogate for the genotype at SNP A. Thus testing of all SNPs in a gene or a region of the genome is unnecessary. One need only genotype a subset of SNPs to capture the linkage disequilibrium pattern among common variants (>5%) of the region of interest to be comprehensive. Importantly, detecting phenotype association with a genetic marker does not indicate that the genetic marker is causally related to the phenotype, but may only reflect linkage disequilibrium with the causal variant.
Case-control studies are the most frequently performed type of genetic association studies because they are simpler to implement than family-based designs. In their simplest form, population-based genetic association studies are similar to epidemiologic case-control studies and involve identifying genetic markers with significant allele, genotype, or haplotype frequency differences between individuals with the phenotype of interest (cases) and a set of unrelated control individuals. A statistical association between genotypes at a marker locus and the phenotype can arise for three reasons: (1) the allele is the actual disease allele, (2) the allele being studied is in linkage disequilibrium with the true disease allele, and (3) there is a spurious association due to population stratification. Indeed, case-control genetic association studies have already contributed to identifying genes associated with complex disorders, as in the cases of apolipoprotein E-4 with late-onset Alzheimer disease and factor V gene with venous thrombosis. Thus, performing valid case-control studies remains important in elucidating genetic risk factors for complex traits. Silverman and Palmer have reviewed these factors that may adversely affect the results of any association study in complex diseases. They have recommended five key elements in the performance of valid case-control genetic association studies for complex diseases: (1) proper selection of gene polymorphisms, (2) accounting for population stratification, (3) assessment of Hardy-Weinberg equilibrium, (4) replication, and (5) adjustment for multiple comparisons.
Ideally, cases and control study subjects should be drawn from the same base population. Failure to do so will often result in biased selection that can adversely influence the results, often resulting in a spurious association. When subjects with different evolutionary histories and hence different genetic backgrounds are differentially selected to be cases and controls, this can cause spurious results, termed “population stratification.” An approach useful in case-control studies to control this problem is to detect and control for population stratification in case and control groups by genotyping randomly distributed polymorphic markers. If population stratification is demonstrated between the case and control populations, methods to detect significant disease gene associations have been developed based on correction for the degree of stratification. A second major problem with early case-control studies has been that often too-small sample size is used to allow for robust evaluation of the evidence for association. Because of the small genetic effect sizes seen and expected for complex traits, sample sizes in the thousands are generally required in addition to replication to generate rigorously validated association results.
Family-based genetic association tests are based on the transmission disequilibrium test, which provides a test of linkage and association without bias from population stratification or admixture. For the transmission disequilibrium test, parents and an individual offspring (child or proband) with the disease phenotype are recruited. Only trios with at least one heterozygous parent at the genetic marker of interest are used for testing. The test is predicated on the assumption that if a genetic locus is uninvolved (neither linked nor associated) in the phenotype of interest, one would expect the two parental alleles at that locus to be transmitted equally to an affected child (i.e., mendelian, transmitted 50% of the time). However, if the locus is actually linked and associated with disease, there will be overtransmission (or undertransmission) of one allele at that locus—and its transmission will differ significantly from the expected 50%.
Family-based association studies cost more than case-control studies because one has to recruit and genotype three people instead of two. In addition, not all diseases can use the family-based design because often parents have died and hence are not available for study. Despite these disadvantages, there are also powerful reasons to use trios if possible. First, unlike the case-control study, the family-based association study is immune to population stratification because the parental genotype is used as the control. Second, within each trio, only one person (the subject) must be phenotyped. This is particularly useful when phenotyping is very expensive or invasive. Family-based testing also offers an important method of assessing genotyping quality, because data can be analyzed for mendelian errors. The transmission disequilibrium test has been extended in many ways, for instance, to allow family-based tests of association for extended pedigrees.
Genome-Wide Association Studies
In GWAS, SNPs are selected to cover as much of the genome as possible. SNPs across all chromosomes and most genes are selected to assess association with a phenotype of interest. There are two currently two companies, Affymetrix and Illumina, which have different SNP selection strategies and different chip chemistry for performing GWAS. Both platforms provide excellent coverage of common variants across the genome for most racial/ethnic groups based on millions of markers.
GWAS are the standard approach to identification of phenotype-associated common genetic variations, and there are well-accepted approaches to the design, data collection, data cleaning, and data analysis required for these large, complex studies. The approximate cost for the most recent genotyping platforms is about $250 per subject with a per-SNP cost of less than 1 cent. Although this genotyping cost is reasonable, the large sample size required for these studies results in millions of dollars for a single case-control study. In addition, the computational speed and electronic storage capacity required for the data analyses increase the cost of the studies. The investment in these studies has yielded great insight into complex disease genetics, because the number of robustly associated genetic loci for complex diseases has increased dramatically between 2000 and 2013.
Gene by Environment Interaction
Since both genes and environmental exposures are known to be related to complex traits, performing studies to test both factors in one study design is of great value. Both family-based and case-control designs allow for testing for association while considering both the genetic and environmental exposures and perhaps their interaction. The two major challenges for these studies are statistical power and accurate measurement of exposure. Tests for gene-environment interaction require larger sample sizes than tests for the genetic effect alone.
Much has been made of the difficulties of accurately measuring the environment as an exposure for genetic association studies. Although some environmental factors such as diet are hard to measure accurately and require complex techniques, other exposures such as lifetime cigarette smoking can be measured with relatively high precision. Accurate measures of exposure will greatly enhance the ability of these studies to provide new insights into disease pathogenesis. It will likely be challenging to replicate these types of studies because finding several studies with similar exposures measured in similar ways is often impossible.
Genetic Epidemiology
Positional Cloning
Advances in our understanding of the variation across the human genome have allowed wide application of the positional-cloning approach to identification of genetic variants that contribute to phenotypes of interest. Positional cloning refers to identification of a chromosomal position that is related to the phenotype based on scanning the genome for a relationship between each locus and the phenotype, rather than relying on the known biochemical properties of a gene to identify it as a candidate for being related to the phenotype. The first approach to positional cloning relied on linkage analysis, most often followed by association analysis; since 2007, genome-wide association analyses have been the standard positional-cloning approach. Whole-exome resequencing studies are now feasible for sample sizes in the hundreds, and whole-genome resequencing studies are rapidly decreasing in cost.
Linkage Studies
Linkage analysis encompasses a group of statistical methods to examine the inheritance pattern of DNA markers within families to determine if there is a relationship between a particular region of the genome and a phenotype of interest. Most linkage studies have been based on short tandem repeat (repeats of a short sequence of nucleotides) or SNP markers distributed through the genome.
Linkage analyses use family data, which can be made up by a wide range of pedigree structures from extended pedigrees to affected sibling pairs. There are two broad types of linkage analysis, parametric and nonparametric; both rely on the coinheritance of disease alleles with genetic markers used in the analysis. When a mutation arises on a particular chromosome, initially there is a large shared segment of DNA and hence linkage disequilibrium around it. With each subsequent generation, this region of linkage disequilibrium becomes smaller as a result of meiotic recombination. The basic approach in parametric linkage analysis is to determine if alleles at a genotyped marker segregate with the alleles at a putative disease locus together more often than one would expect by random assortment, or chance. This can be assessed by comparing the frequency of recombinant chromosomes in which a crossing over event has rearranged the parental chromosomes to the frequency of nonrecombinant chromosomes. When two loci are linked, parental chromosomes are more common than recombinant chromosomes. The strength of the linkage between a marker and a putative disease locus is expressed as the recombination fraction. Parametric linkage analyses require that a particular genetic model be specified. Thus the approach is ideal for classic mendelian, monogenic disorders but is less well adapted for complex traits, where these parameters are often not known. Nonparametric linkage analysis refers to a group of analysis methods that, in contrast to parametric linkage analysis, do not require assumptions about a particular form of inheritance. The general approach for nonparametric linkage analysis is to contrast observed allele sharing between affected relatives to that expected given their relationship (e.g., siblings) at a given locus. Regions that show statistically significant excess sharing among affected relatives are regions that may harbor loci important for the phenotype of interest. This nonparametric approach has been combined with genetic association within the linked region to identify disease susceptibility genes for asthma, Crohn disease, and pulmonary fibrosis.
Linkage results are usually expressed as an LOD score, which is a function of a statistical test for linkage. LOD scores are log of base 10 of the odds that the loci are linked, and their distribution depends on the study design.
Association Studies
Genetic association studies are the most commonly used study designs to find disease genes in complex traits. Association studies can be used in conjunction with linkage studies, de novo with candidate genes or genome wide association studies (GWAS). There are two basic types of study designs used for genetic association studies, the case-control study and the family-based study; both types rely on the concept of linkage disequilibrium between the alleles at a genotyped marker(s) and the disease allele(s).
When two SNPs are on separate chromosomes, they will segregate randomly (i.e., carrying the minor allele at SNP A does not affect your chances of carrying the minor allele at SNP B). If, on the other hand, the alleles at the SNPs are in linkage disequilibrium, with little or no recombination between them, the genotype at SNP B can serve as a surrogate for the genotype at SNP A. Thus testing of all SNPs in a gene or a region of the genome is unnecessary. One need only genotype a subset of SNPs to capture the linkage disequilibrium pattern among common variants (>5%) of the region of interest to be comprehensive. Importantly, detecting phenotype association with a genetic marker does not indicate that the genetic marker is causally related to the phenotype, but may only reflect linkage disequilibrium with the causal variant.
Case-control studies are the most frequently performed type of genetic association studies because they are simpler to implement than family-based designs. In their simplest form, population-based genetic association studies are similar to epidemiologic case-control studies and involve identifying genetic markers with significant allele, genotype, or haplotype frequency differences between individuals with the phenotype of interest (cases) and a set of unrelated control individuals. A statistical association between genotypes at a marker locus and the phenotype can arise for three reasons: (1) the allele is the actual disease allele, (2) the allele being studied is in linkage disequilibrium with the true disease allele, and (3) there is a spurious association due to population stratification. Indeed, case-control genetic association studies have already contributed to identifying genes associated with complex disorders, as in the cases of apolipoprotein E-4 with late-onset Alzheimer disease and factor V gene with venous thrombosis. Thus, performing valid case-control studies remains important in elucidating genetic risk factors for complex traits. Silverman and Palmer have reviewed these factors that may adversely affect the results of any association study in complex diseases. They have recommended five key elements in the performance of valid case-control genetic association studies for complex diseases: (1) proper selection of gene polymorphisms, (2) accounting for population stratification, (3) assessment of Hardy-Weinberg equilibrium, (4) replication, and (5) adjustment for multiple comparisons.
Ideally, cases and control study subjects should be drawn from the same base population. Failure to do so will often result in biased selection that can adversely influence the results, often resulting in a spurious association. When subjects with different evolutionary histories and hence different genetic backgrounds are differentially selected to be cases and controls, this can cause spurious results, termed “population stratification.” An approach useful in case-control studies to control this problem is to detect and control for population stratification in case and control groups by genotyping randomly distributed polymorphic markers. If population stratification is demonstrated between the case and control populations, methods to detect significant disease gene associations have been developed based on correction for the degree of stratification. A second major problem with early case-control studies has been that often too-small sample size is used to allow for robust evaluation of the evidence for association. Because of the small genetic effect sizes seen and expected for complex traits, sample sizes in the thousands are generally required in addition to replication to generate rigorously validated association results.
Family-based genetic association tests are based on the transmission disequilibrium test, which provides a test of linkage and association without bias from population stratification or admixture. For the transmission disequilibrium test, parents and an individual offspring (child or proband) with the disease phenotype are recruited. Only trios with at least one heterozygous parent at the genetic marker of interest are used for testing. The test is predicated on the assumption that if a genetic locus is uninvolved (neither linked nor associated) in the phenotype of interest, one would expect the two parental alleles at that locus to be transmitted equally to an affected child (i.e., mendelian, transmitted 50% of the time). However, if the locus is actually linked and associated with disease, there will be overtransmission (or undertransmission) of one allele at that locus—and its transmission will differ significantly from the expected 50%.
Family-based association studies cost more than case-control studies because one has to recruit and genotype three people instead of two. In addition, not all diseases can use the family-based design because often parents have died and hence are not available for study. Despite these disadvantages, there are also powerful reasons to use trios if possible. First, unlike the case-control study, the family-based association study is immune to population stratification because the parental genotype is used as the control. Second, within each trio, only one person (the subject) must be phenotyped. This is particularly useful when phenotyping is very expensive or invasive. Family-based testing also offers an important method of assessing genotyping quality, because data can be analyzed for mendelian errors. The transmission disequilibrium test has been extended in many ways, for instance, to allow family-based tests of association for extended pedigrees.
Genome-Wide Association Studies
In GWAS, SNPs are selected to cover as much of the genome as possible. SNPs across all chromosomes and most genes are selected to assess association with a phenotype of interest. There are two currently two companies, Affymetrix and Illumina, which have different SNP selection strategies and different chip chemistry for performing GWAS. Both platforms provide excellent coverage of common variants across the genome for most racial/ethnic groups based on millions of markers.
GWAS are the standard approach to identification of phenotype-associated common genetic variations, and there are well-accepted approaches to the design, data collection, data cleaning, and data analysis required for these large, complex studies. The approximate cost for the most recent genotyping platforms is about $250 per subject with a per-SNP cost of less than 1 cent. Although this genotyping cost is reasonable, the large sample size required for these studies results in millions of dollars for a single case-control study. In addition, the computational speed and electronic storage capacity required for the data analyses increase the cost of the studies. The investment in these studies has yielded great insight into complex disease genetics, because the number of robustly associated genetic loci for complex diseases has increased dramatically between 2000 and 2013.
Gene by Environment Interaction
Since both genes and environmental exposures are known to be related to complex traits, performing studies to test both factors in one study design is of great value. Both family-based and case-control designs allow for testing for association while considering both the genetic and environmental exposures and perhaps their interaction. The two major challenges for these studies are statistical power and accurate measurement of exposure. Tests for gene-environment interaction require larger sample sizes than tests for the genetic effect alone.
Much has been made of the difficulties of accurately measuring the environment as an exposure for genetic association studies. Although some environmental factors such as diet are hard to measure accurately and require complex techniques, other exposures such as lifetime cigarette smoking can be measured with relatively high precision. Accurate measures of exposure will greatly enhance the ability of these studies to provide new insights into disease pathogenesis. It will likely be challenging to replicate these types of studies because finding several studies with similar exposures measured in similar ways is often impossible.
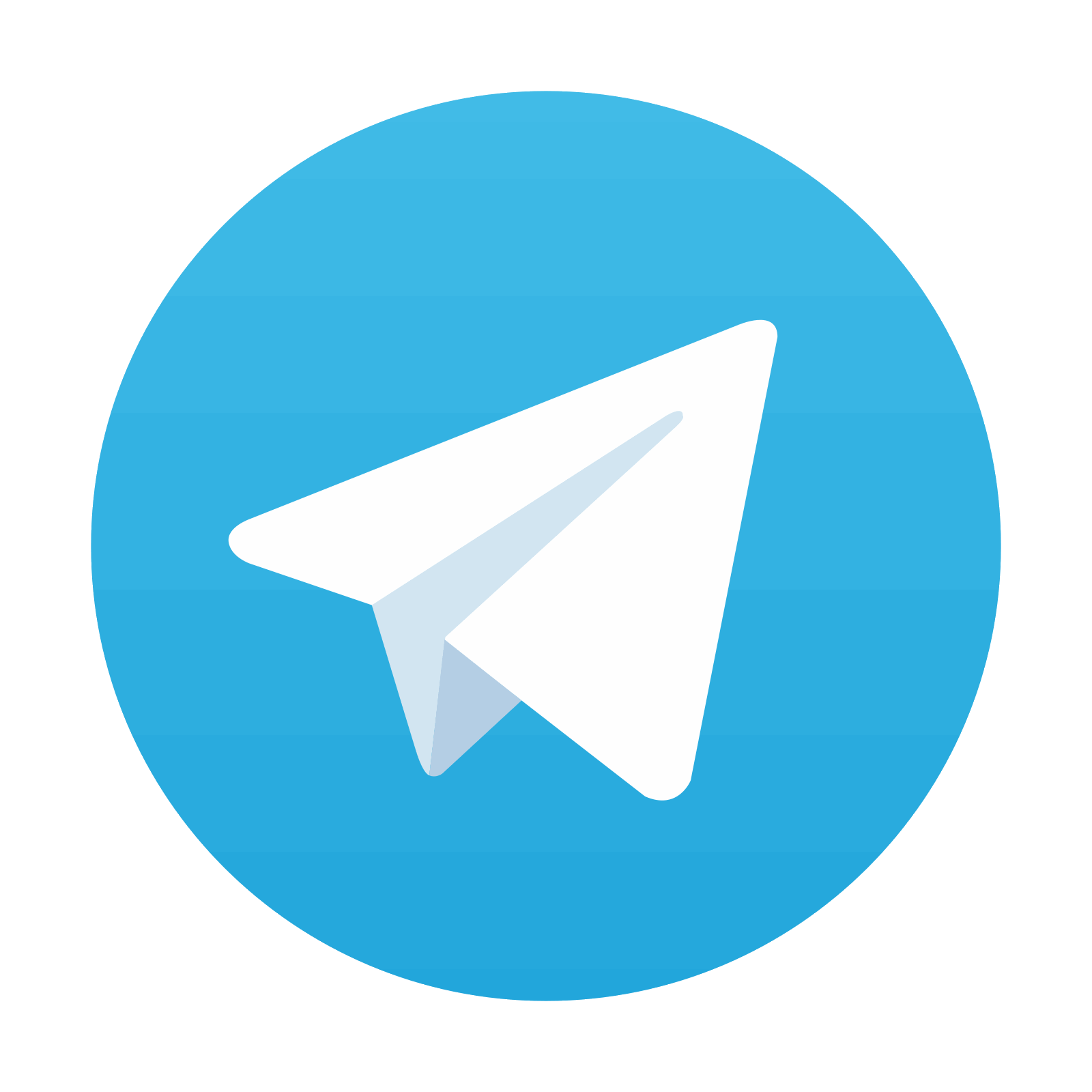
Stay updated, free articles. Join our Telegram channel

Full access? Get Clinical Tree
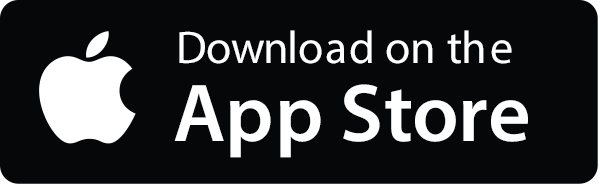
