Key Points
- •
Screening involves the routine testing of asymptomatic individuals for the purpose of detecting the presence of a condition or a disease. The ultimate goal of screening is to identify the disease or condition of interest in an early phase, when intervention may be more effective in reducing subsequent morbidity or mortality.
- •
A number of metrics are available to assess the performance of screening tests, including sensitivity, specificity, predictive values, model fit characteristics, discrimination measures, and risk reclassification.
- •
Unlike with cancer, screening for CVD has typically involved screening for risk factors, for which there is solid evidence of utility, rather than for CVD itself.
- •
Current CVD clinical practice guidelines recommend screening for global cardiovascular risk by use of multivariable risk equations to assist in decision making regarding the intensity of prevention strategies.
- •
Use of imaging modalities to screen for subclinical CVD is an area of intense research interest. Current guidelines do not recommend routine screening for atherosclerosis in asymptomatic individuals, but accumulating evidence may help define a role for these screening tests, perhaps in refining risk stratification among those at intermediate risk for CVD events by traditional risk factor levels.
Screening involves the routine evaluation or testing of asymptomatic individuals for the purpose of detecting the presence of a condition or a disease. The ultimate goal of screening is generally to identify the disease or condition of interest in an early, or latent, phase, when intervention may be more effective in reducing subsequent morbidity or mortality. Historically, many of the concepts that have been used to define the utility of screening tests (sensitivity, specificity, predictive values) in patients and populations arose from the assessment of tests designed to detect medical conditions with a clear pathologic diagnosis, such as cancer. In recent years, these classical measures of diagnostic utility and many novel metrics have been employed as screening increasingly is used for preclinical conditions in the causal pathways for clinical disease and for prognosis, rather than merely for diagnosis. In the realm of cardiovascular disease (CVD), screening is a topic of significant import and heated debate, given the high incidence of disease across the life span, the substantial burden of morbidity and mortality, and the potentially high costs of screening tests and therapies. This is especially true in the current health care and economic environment. Thus, understanding of the basic concepts related to screening for CVD is of paramount importance. A review of the conceptual framework for screening tests and the means for evaluating their utility will serve as background for a discussion of the relative merits of screening for CVD risk factors (traditional and novel), global cardiovascular risk, and subclinical atherosclerosis.
Concepts in Screening
Criteria for Screening
In 1968, the World Health Organization defined criteria for screening for disease in medicine. As shown in Box 26-1 , these criteria indicate that screening for disease should be considered when the disease has a significant impact (in terms of prevalence or severity), has an adverse natural history that is well understood, and is treatable or modifiable during its asymptomatic phase. Of equal importance in these criteria are the features of the screening test itself: it should be reliable, available, cost-effective relative to other strategies, and applicable in an ongoing fashion. These widely accepted criteria provide a useful framework for evaluation of new (and old) screening tests. Clearly, it is inadequate merely to use a test with face validity for detection of disease; rather, careful consideration of the potential benefits, risks, harms, and potential costs associated with screening must be undertaken before widespread clinical adoption.
The condition sought should be an important health problem for the individual and community.
There should be an accepted treatment or useful intervention for patients with the disease.
The natural history of the disease should be adequately understood.
There should be a latent or early symptomatic stage.
There should be a suitable and acceptable screening test or examination.
Facilities for diagnosis and treatment should be available.
There should be an agreed policy on whom to treat as patients.
Treatment started at an early stage should be of more benefit than treatment started later.
The cost should be economically balanced in relation to possible expenditure on medical care as a whole.
Case finding should be a continuing process and not a once and for all project.
Types of Screening
Mass or universal screening involves assessment of all individuals in a population or group (e.g., all school-aged children or all pregnant women). Case finding, or high-risk screening, involves application of a screening test to subgroups identified as being at higher risk than average because of the presence of known risk factors (e.g., a strong family history of disease). As discussed later, each of these approaches has merit, depending on the nature of the disease or condition being screened and the knowledge of important predisposing factors.
Assessment of Screening Tests
A list of commonly applied metrics for evaluation of screening tests in CVD is provided in Table 26-1 . Some discussion of these tests is warranted for a fuller understanding of their implications. Classical metrics of test characteristics (sensitivity, specificity, and related metrics) can be understood most easily in comparison with a “gold standard” test indicating the definitive presence or absence of disease. However, they are also applicable to prognostic tests, in which the gold standard is the development (incidence) of disease during the follow-up interval of observation after testing.
Measure | Definition | Comment |
---|---|---|
Sensitivity | Proportion of those with disease who have a positive test result [P(T+|D+)] | Detection rate, true-positive rate; high sensitivity is useful for ruling out disease |
Specificity | Proportion of those without disease who have a negative test result [P(T−|D−)] | True-negative rate; high specificity is useful for ruling in disease |
False-negative rate | Proportion of those with disease who have a negative test result [P(T−|D+)] | Equal to 1 − sensitivity; when low, useful for ruling out disease |
False-positive rate | Proportion of those without disease who have a positive test result [P(T+|D−)] | Equal to 1 − specificity; when low, useful for ruling in disease |
Positive predictive value (PV+) | Proportion of those with a positive test result who have disease [P(D+|T+)] | Dependent on the prevalence (incidence) of disease |
Negative predictive value (PV−) | Proportion of those with a negative test result who do not have disease [P(D−|T−)] | Dependent on the prevalence (incidence) of disease |
Likelihood ratio | Ratio of true-positive rate to false-positive rate [P(T+|D+)] / [P(T+|D−)] | Can be used to calculate post-test odds (and therefore post-test probability of disease) by multiplying with pretest odds |
Pretest probability | Probability of disease based on available information (prevalence, or risk-adjusted prevalence) | |
Post-test probability | Adjusted probability of disease after application of additional (screening) test | |
Area under the receiver operating characteristic curve (AUC, or C-statistic) | Function of true-positive rate and false-positive rate across all values of diagnostic (screening) test | Indicates discrimination ability of test; likelihood that a randomly selected case will have a positive (or more adverse) test result compared with a randomly selected non-case |
Model fit | Assessment of whether statistical model including test improves case detection/prediction compared with chance or base model | Information criteria or likelihood ratio test often used to assess the utility of the model |
Calibration | Degree to which screening (prediction) test or model accurately predicts absolute levels of observed event rates | Usually assessed with Hosmer-Lemeshow test |
Net reclassification improvement | Degree to which new test increases predicted risk (across a decision threshold) for those who subsequently have events and decreases predicted risk (across a decision threshold) for those who do not subsequently have events | |
Integrated discrimination index | Indicates how far individuals are moving, on average, along the continuum of predicted risk after application of test | Equivalent to the difference in R 2 between the two models being compared |
* In the case of screening tests related to prognosis (rather than to diagnosis), the definitions would be relevant to those who do or do not develop disease during observation.
As recently detailed by the American Heart Association, appropriate consideration of traditional and novel screening tests for CVD (which may include single tests or multivariable risk scores) should entail assessment of a number of different metrics beyond simple association, sensitivity, specificity, and predictive values. Demonstration that a screening test has a significant statistical association with the outcome of interest is necessary but clearly not sufficient for evaluation of its utility. A number of metrics are available to assist in the evaluation of the performance and utility of risk estimation models. These metrics assess characteristics of the test (similar to a diagnostic test), its ability to discriminate cases from non-cases, the calibration of the model, model fit, and the informativeness of the model for the outcome of interest. Newer methods of assessment, such as analysis of risk reclassification, also allow comparison of different risk stratification algorithms by use of novel markers or risk scores. Knowledge of a few of these metrics and concepts will suffice for most clinicians to interpret the utility of risk prediction models. Consideration of all of these factors is important to understanding the utility of a risk score.
Sensitivity, Specificity, and Predictive Values
As shown in Figure 26-1 and Table 26-1 , sensitivity and specificity reflect the true-positive and true-negative rates, respectively. In other words, a test with high sensitivity will detect a large proportion of individuals who have disease; a test with high specificity will correctly be negative in individuals without disease. These are useful test characteristics that in most cases do not change on the basis of the prevalence of disease in the groups being tested. However, they do not necessarily answer the question that is of interest to a clinician and patient: Is disease present? Positive and negative predictive values typically may be more useful as assessments of diagnostic and screening tests because they indicate the likelihood of having or developing disease given a positive (or higher) or negative (lower) test result, but their heavy reliance on the incidence and prevalence of disease in the population may make them difficult to translate from one clinical scenario to another.

Measures of Model Fit and Informativeness
Other measures, such as the Bayes information criterion, are now commonly used to assess the utility of statistical risk prediction models that include screening tests. These tests can indicate whether a risk model is predicting disease incidence better than chance alone. They can further indicate whether the addition of new screening tests to a base model provides better risk prediction than the base model alone, provided all of the same individuals are being assessed by both models.
Discrimination
One of the most widely reported measures of model discrimination for screening tests generally, and CVD risk prediction models specifically, is the area under the receiver operating characteristic curve (AUC), or C-statistic. The C-statistic is a function of both the true-positive and false-positive rates of the screening tool across all of its values, and it represents the ability of the score to discriminate (future) cases from non-cases. In other words, the C-statistic indicates the probability that a randomly selected patient who has or develops the disease (a “case”) will have a higher test result or risk score than a randomly selected non-case. The AUC or C-statistic can vary from 1.0 (perfect discrimination) to 0.5 (random chance, equivalent to flipping a coin to determine case status). Thus, a C-statistic of 0.75 for a given model would indicate that a randomly selected case has a higher score than a randomly selected non-case 75% of the time ( Fig. 26-2 ). C-statistics below 0.70 are generally considered to indicate inadequate discrimination by a test, whereas those between 0.70 and 0.80 are considered “acceptable,” and between 0.80 and 0.90, “excellent.”

The C-statistic is imperfect as a stand-alone metric for assessment of screening tools or risk prediction models. In general, the C-statistic indicates whether a test or risk score is generating appropriate rank-ordering of risk for cases and non-cases, not whether the predicted risk and observed outcome rates are similar (which is a function of calibration) or how much greater the estimated risk for disease is between selected cases and non-cases.
Pepe and coworkers have demonstrated that very large odds ratios (or relative risks) are required to reach meaningful levels in the C-statistic. For example, a univariate odds ratio of 9.0 or greater would be required to achieve a C-statistic that provides excellent discrimination of cases from non-cases for a continuous screening test (e.g., cholesterol or coronary calcium) in which the distribution of test scores differs by 2 or more standard deviations. These magnitudes of differences in distribution of test scores are rarely seen in clinical practice (where risk factor levels often overlap substantially), as are such high odds ratios. However, the combination of multiple, independent screening tests or risk markers, as in the Framingham risk score (FRS) and similar scores (see later), does provide these magnitudes of relative risk.
Calibration
Measures of calibration assess the ability of a screening test or risk prediction model to predict accurately the absolute level of risk that is subsequently observed. Demonstration that a risk prediction model is well calibrated would require that if the model estimates that the risk for a certain subgroup of individuals is 5% during 5 years, then the observed event rate should be close to 5%. Calibration is often assessed visually by dividing the population at risk into strata, such as deciles of predicted risk, and plotting the predicted risk versus the observed event rate for each decile ( Fig. 26-3 ). The statistical metric used to test for the calibration of a risk model most often is the Hosmer-Lemeshow χ 2 test. A P value <0.05 for such a test would indicate poor calibration of the model for the population.

Assessment of Appropriate Risk Reclassification
A newer paradigm to assess the utility of screening tests and risk prediction models, which is recommended by the American Heart Association for appropriate assessment of such tests, is risk reclassification analysis. This approach requires measurement of the proportion of individuals who are reclassified from one risk stratum (e.g., intermediate risk) based on the estimated risk provided from a first model to a different risk stratum (e.g., high risk) based on estimated risk from a model that contains the additional test information. Some of these risk reclassifications end up being appropriate (based on subsequent observed events): some individuals who have events are reclassified to higher predicted risk strata, and some who do not have events are reclassified to lower predicted risk strata. However, some reclassifications are inappropriate, moving future cases to lower predicted risk strata and future non-cases to higher predicted-risk strata.
Pencina and coworkers have proposed two indices, the net reclassification improvement (NRI) and the integrative discrimination index (IDI), to attempt to quantify the appropriateness and the amount of overall reclassification. In general, the NRI indicates how much more appropriate reclassification occurs than inappropriate reclassification with use of the new model. The NRI can vary from −2, indicating that all individuals are reclassified inappropriately, to +2, indicating that all are reclassified appropriately. In other words, if the newer test reclassifies all of the people who have events upward, and all of the people who do not end up with events downward, the NRI would be +2. For this test, a P value <0.05 suggests that a significantly greater number are being reclassified appropriately than are being reclassified inappropriately. The IDI can be thought of as indicating how far individuals are reclassified, on average, along the continuum of predicted risk. If the IDI is small (even if it is statistically significant), then a given individual’s change in predicted risk with the new model will be small, on average. As an example, consider a new risk prediction model or test that is being compared with the FRS for stratifying a population into risk categories. The new model might have a significant NRI, reclassifying a net of 10% of people more appropriately; but if the IDI is small (e.g., less than 1%), then most of the net reclassification is occurring immediately adjacent to the decision thresholds that separate the risk categories, such as a change from a predicted risk of 19.8% with an old model to a predicted risk of 20.4% with a new model. This change might cross the decision threshold for treatment, but it would indicate no real impact in understanding or forecasting of the patient’s risk, especially if the decision thresholds are relatively arbitrary. The significance of such small movements is also heavily dependent on the threshold selected. Indeed, this scenario is what is often observed in current studies comparing older and newer CVD risk prediction scores.
Interpretation of Risk Information Provided by Screening Tests
Different types of information about risk for disease may be garnered from screening tests. The relative risk of disease is the ratio of disease or disease incidence among those with a positive test result compared with those who have a negative test result. As such, relative risk measures the strength of the association between the test and disease, but relative risks are poor indicators of clinical utility, and physicians and patients often have difficulty interpreting relative risk estimates in the absence of an obvious comparison group. A relative risk for disease of 10 might seem very high, but if the incidence rate in the referent group is close to 0, it will also be close to 0 in the group with the relative risk of 10. Absolute risk of disease is often expressed as the estimated rate of development of new cases of disease per unit of time (or incidence) in individuals with a positive test result. Absolute risk estimates may be more easily understood than relative risks, and they allow clinical recommendations for interventions in individuals who exceed unacceptable risk thresholds. This approach has been widely adopted for 5- and 10-year estimation of absolute risks for coronary heart disease (CHD) and CVD to guide clinical decision making for CVD prevention. The attributable risk of a test result describes the proportion of the incidence of disease in a population associated with the test result, assuming a causal relationship exists. The population attributable risk takes into account the proportion of individuals in the population who test positive as well as the relative risk. Therefore, attributable risk is a useful concept in selecting screening tests that might be targeted for prevention programs.
In clinical trials, we often consider the concept of a number needed to treat (NNT) to prevent one event. The NNT is calculated as follows:
1 / ( rate of disease in the control group − rate of disease in the intervention group )
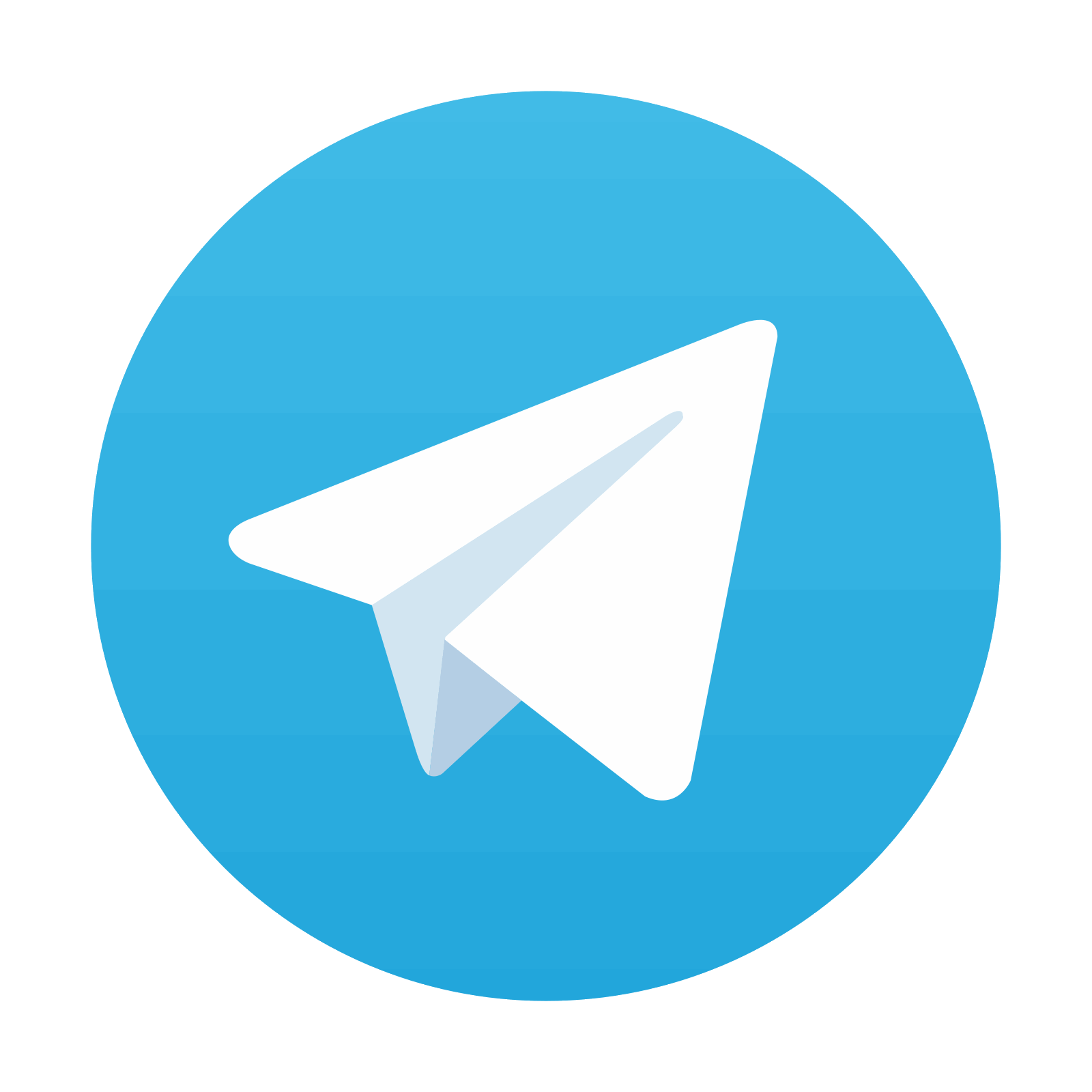
Stay updated, free articles. Join our Telegram channel

Full access? Get Clinical Tree
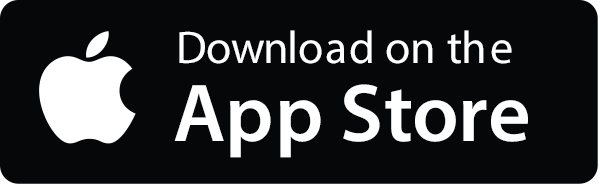
