Improved accuracy in predicting coronary heart disease (CHD) risk in patients with diabetes and kidney disease is needed. The addition of albuminuria to established methods of CHD risk calculation was reported in the Strong Heart Study (SHS) cohort. In this study, the addition of estimated glomerular filtration rate (eGFR) was evaluated using data from 4,549 American Indian SHS participants aged 45 to 74 years. After adjustment for Framingham CHD risk factors, hazard ratios for eGFR as a predictor of CHD were 1.69 (95% confidence interval 1.34 to 2.13) in women and 1.41 (95% confidence interval 0.94 to 2.13) in men. Models including albuminuria, eGFR, or both scored higher in discriminatory power than models using conventional risk factors alone in women; in men, the improvement was seen only for albuminuria and the combination of albuminuria and eGFR. Hosmer-Lemeshow assessments showed good calibration for the models using eGFR alone in both genders, followed by models including albuminuria alone in both genders. Adding eGFR improved the net reclassification improvement (NRI) in women (0.085, p = 0.0004) but not in men (0.010, p = 0.1967). NRI and integrated discrimination improvement (IDI) were improved in both genders using albuminuria and eGFR (NRI 0.135, p <0.0001, and IDI 0.027, p <0.0001 in women; NRI 0.035, p <0.0196, and IDI 0.008, p <0.0156 in men). Therefore, a risk calculator including albuminuria enhances CHD prediction compared to a calculator using only standard risk factors in men and women. Including eGFR alone improves risk prediction in women, but for men, it is preferable to include eGFR and albuminuria. In conclusion, this enhanced calculator should be useful in estimating CHD risk in populations with high prevalence of diabetes and renal disease.
In populations with high rates of diabetes mellitus, associated renal disease contributes substantially to coronary heart disease (CHD). Previous studies have addressed the effect of estimated glomerular filtration rate (eGFR) and albuminuria, either synergistically or independently, in predicting cardiovascular disease (CVD) risk; however, those data were based on randomized clinical trials or generated from population-based studies with various disease characteristics. A Norwegian study, composed of a population with high prevalence of diabetes and CVD, suggested that low eGFR and albuminuria were synergistic risk factors in predicting cardiovascular mortality in the elderly. The Strong Heart Study (SHS) is a unique source of information on CHD and renal disease in a population with high prevalence of diabetes mellitus and kidney abnormalities. Lee et al demonstrated the importance of including albuminuria in a CHD risk calculator, along with age, hypertension, low-density lipoprotein (LDL) cholesterol, and diabetes, to predict 10-year risk. In the present analysis, longitudinal data from the SHS were used to examine the value of incorporating eGFR as a measure of kidney function in CHD risk prediction, because eGFR is now commonly available and widely used in clinical settings.
Methods
The SHS was initiated in 1988 to investigate CVD and its risk factors in geographically diverse groups of American Indians. The SHS design, methods, and laboratory techniques have been published. The Indian Health Service, institutional review boards, participating tribes, and the MedStar Health Research Institute approved the study, and informed consent was obtained. This cohort includes 4,549 men and women aged 45 to 74 years. Three examinations took place in 1989 to 1991, 1993 to 1995, and 1998 to 2000. Excluded from the analyses were 182 participants with definite CHD (definite CHD, definite myocardial infarction [MI], or electrocardiographically evident MI) or stroke before the baseline examination.
Baseline and follow-up examinations consisted of a personal interview and physical examination. Weight, height, blood pressure, and waist and hip circumferences were measured. Body mass index was calculated using height and weight (kilograms per square meter). Hypertension was classified into 3 categories, according to Seventh Joint National Committee on Hypertension criteria : normal (systolic blood pressure [SBP] <120 mm Hg, diastolic blood pressure [DBP] <80 mm Hg, and no antihypertensive medication), prehypertension (SBP 120 to 139 mm Hg or DBP 80 to 89 mm Hg and no antihypertensive medication), and hypertension (SBP ≥140 mm Hg or DBP ≥90 mm Hg and/or taking antihypertensive medication). Blood was drawn and a urine sample obtained at the baseline examination after a 12-hour fast. Blood measures included plasma cholesterol, triglycerides, high-density lipoprotein (HDL) cholesterol, LDL cholesterol, and fasting glucose. Serum creatinine was measured via an automated alkaline picrate method; urinary albumin was measured using an automated nephelometric immunochemical procedure. Diabetes was defined as fasting plasma glucose ≥126 mg/dl (6.99 mmol/L) or receiving insulin or oral antihyperglycemic treatment). Albuminuria was categorized as follows: microalbuminuria: albumin/creatinine ≥30 to <300 mg/g, and macroalbuminuria: albumin/creatinine ≥300 mg/g. The eGFR was calculated using the abbreviated Modification of Diet in Renal Disease (MDRD) equation : eGFR = 186 × (serum creatinine) −1.154 × age −0.203 × 0.742 if female × 1.210 if African American. Because the SHS includes only American Indians, the MDRD term for ethnicity was excluded.
The eGFR was dichotomously categorized as either preserved (≥60 ml/min/1.73 m 2 ) or decreased (<60 ml/min/1.73 m 2 ). Age was used as a continuous variable in the prediction equation. LDL cholesterol was classified into 5 groups according to National Cholesterol Education Program Adult Treatment Panel III criteria : <100, 100 to 129, 130 to 159, 160 to 189, and ≥190 mg/dl (<2.59, 2.59 to 3.35, 3.36 to 4.13, and ≥4.14 mmol/L); HDL cholesterol was classified into 3 categories: <40, 40 to 59, and ≥60 mg/dl (<1.04, 1.04 to 1.54, and ≥1.55 mmol/L). The baseline examination was conducted before lipid-lowering therapy was widely used by Indian Health Service providers.
CHD events occurring during the follow-up were ascertained from the annual surveillance or at the second or third examinations. Medical records were abstracted, and CHD death and events were confirmed by mortality and morbidity review committees. The data used in these analyses consisted of incident fatal and nonfatal CHD events (definite MI, definite CHD, electrocardiographically evident definite MI, fatal definite MI, definite and possible fatal CHD, and sudden death due to CHD) occurring during the median 15-year follow-up period from the baseline examination through December 31, 2007; loss to follow-up was <1%. Time to event was calculated from the date of the baseline examination to the date of the CHD event or last follow-up. Baseline characteristics of the study population were calculated by gender and incident CHD status at the end of 2007. Chi-square tests were used to compare categorical risk factors, and independent Students’ t tests were used to compare continuous risk factors in those who did and did not develop CHD.
Cox proportional-hazards models were used with the basic Framingham risk covariates to develop CHD risk estimates. A number of models were considered in developing the optimal prediction equation, including models with various forms of the significant risk factors (i.e., as continuous and categorical variables). Interaction terms, such as age × LDL cholesterol, age × HDL cholesterol, age × SBP, age × DBP, and log(albumin/creatinine) × diabetes, also were considered, and none were significant. We sequentially added eGFR and albuminuria to the models to assess the improvement in CHD risk prediction when including measures of kidney function. We considered and compared the following models using SHS data in our analyses: (1) Framingham CHD risk model, (2) Framingham model with adjusted coefficients and SHS data, (3) model 2 plus data-specific cut-off points, (4) model 3 plus albuminuria, (5) model 3 plus eGFR, and (6) model 3 plus albuminuria and eGFR. Model 6 was used to develop the currently used SHS CHD risk calculator.
To assess the ability of the prediction equations to discriminate between participants who would develop CHD and those who would not, we used the c-statistic (area under the receiver-operating characteristic curve). A c-statistic value of ≥0.70 indicates relatively good discrimination. The Hosmer-Lemeshow chi-square statistic was calculated to assess model calibration. Values <20 (p ≥ 0.01) indicate good calibration.
We used the Hosmer-Lemeshow chi-square statistic to assess each model’s calibration and the c-statistic, a widely used measure of discrimination. However, the c-statistic is not sensitive for detecting differences in discrimination ability between models with and without new markers and may not be optimal for prospective risk prediction. Therefore, we further assessed the reclassification ability by using reclassification tables. Reclassification tables for participants with and without incident CHD were constructed using estimated CHD risk categories on the basis of Adult Treatment Panel III 10-year CHD risk criteria. We computed net reclassification improvement (NRI) to assess improvement in CHD prediction obtained by introducing eGFR and/or albuminuria into the models. We also computed the integrated discrimination improvement (IDI), which does not require a specific cut-off point for risk categories, to determine improvements in sensitivity and specificity. Tests to determine whether the improvements in NRI and IDI differed significantly from zero were performed.
Results
Among the 4,367 participants without CHD or definite stroke at baseline, 1,009 (529 women and 480 men) had developed CHD by the end of 2007. Men and women who developed CHD events were older, had higher LDL cholesterol and SBP and lower HDL cholesterol and eGFRs, and had higher prevalence of diabetes and albuminuria at baseline ( Table 1 ).
Variable | Without Incident CHD | With Incident CHD | p Value ⁎ |
---|---|---|---|
Women | |||
Age (years) | 56.2 ± 8.0 | 58.1 ± 8.0 | <0.001 |
Body mass index (kg/m 2 ) | 31.5 ± 6.7 | 31.7 ± 5.9 | 0.3877 |
Total cholesterol (mg/dl) | 190 ± 39 | 202 ± 43 | <0.001 |
HDL cholesterol (mg/dl) | 49 ± 13 | 45 ± 12 | <0.001 |
LDL cholesterol (mg/dl) | 114 ± 33 | 122 ± 36 | <0.001 |
SBP (mm Hg) | 126 ± 20 | 134 ± 24 | <0.001 |
DBP (mm Hg) | 75 ± 10 | 76 ± 10 | 0.0979 |
eGFR (MDRD equation) | 79.7 ± 23.0 | 76.4 ± 31.1 | 0.0069 |
Diabetes mellitus | 41.6% | 72.1% | <0.001 |
Microalbuminuria | 17.8% | 26.5% | <0.001 |
Macroalbuminuria | 8.0% | 23.7% | <0.001 |
Current smoker | 29.4% | 30.6% | 0.5756 |
Men | |||
Age (years) | 54.9 ± 7.8 | 57.2 ± 8.0 | <0.001 |
Body mass index (kg/m 2 ) | 29.7 ± 6.2 | 30.2 ± 5.4 | 0.1382 |
Total cholesterol (mg/dl) | 186 ± 40 | 197 ± 42 | <0.001 |
HDL cholesterol (mg/dl) | 44 ± 14 | 41 ± 12 | <0.001 |
LDL cholesterol (mg/dl) | 115 ± 33 | 125 ± 35 | <0.001 |
SBP (mm Hg) | 127 ± 18 | 131 ± 18 | <0.001 |
DBP (mm Hg) | 80 ± 10 | 80 ± 10 | 0.0798 |
eGFR (MDRD equation) | 88.0 ± 19.6 | 85.7 ± 19.5 | 0.0273 |
Diabetes mellitus | 35.7% | 52.2% | <0.001 |
Microalbuminuria | 17.0% | 20.8% | 0.0708 |
Macroalbuminuria | 8.1% | 12.6% | 0.0045 |
Current smoker | 40.2% | 43.5% | 0.2016 |
⁎ Student’s t tests were used for continuous variables, and chi-square tests were used for categorical variables.
To assess the association of incident CHD with albuminuria and eGFR, hazard ratios were calculated adjusted for Framingham CHD risk factors. Hazard ratios for eGFR were 1.69 (95% confidence interval [CI] 1.34 to 2.13) in women and 1.41 (95% CI 0.94 to 2.13) in men. Hazard ratios for microalbuminuria and macroalbuminuria were 1.56 (95% CI 1.24 to 1.95) and 2.90 (95% CI 2.26 to 3.71), respectively, in women and 1.23 (95% CI 0.96 to 1.57) and 1.88 (95% CI 1.39, 2.55), respectively, in men.
The coefficients underlying the CHD prediction equations for men and women were generated from models adding albuminuria (model 4), eGFR (model 5), or both (model 6) ( Table 2 ). With the subject’s risk factors (x = [x 1 , x 2 , …, x p ]), estimated coefficients (b 1 , b 2 , …, b p ), and Breslow’s estimate for S 0 (t), the probability of developing CHD (P[t, x]) within 10 years for a participant with a given set of risk factor values (x 1 , x 2 , …, x p ) could be calculated by the equation 1 − [ S 0 ( t ) ] exp ( ∑ b i x i ) . Further details of the equations are provided in the Appendix . To illustrate the application of Table 2 , for a 65-year-old male smoker with diabetes and macroalbuminuria, SBP of 120 mm Hg, DBP of 85 mm Hg, an eGFR of 80 ml/min/1.73 m 2 , LDL cholesterol of 130 mg/dl, and HDL cholesterol of 68 mg/dl, and no antihypertensive medication, the estimated probability of developing CHD in 10 years can be calculated as follows: Σb i x i = 0.0444 (age 65 years) + 0.3200 (LDL cholesterol 130 to 159 mg/dL) + (−0.0091) (HDL cholesterol >60 mg/dl) + 0.3440 (prehypertension) + 0.5733 (diabetes present) + 0.3797 (current smoker) + 0.6348 (macroalbuminuria present) + 0.1721 (eGFR <60 ml/min/1.73 m 2 ) = 5.3008; exp(Σb i x i ) = exp(5.3008) = 200.497; thus, P(t) = 1 − S 0 (t) 200.497 = 1 – 0.2932 = 0.7068. Thus, the estimated probability of developing CHD in 10 years for this participant is 71%.
Variable | Model 4 ⁎ | Model 5 † | Model 6 ‡ | |||
---|---|---|---|---|---|---|
Women | Men | Women | Men | Women | Men | |
Age | 0.0303 | 0.0463 | 0.0210 | 0.0434 | 0.0262 | 0.0444 |
LDL cholesterol (mg/dl) | ||||||
<100 | Referent | Referent | Referent | Referent | Referent | Referent |
100–129 | 0.3299 | 0.0847 | 0.3229 | 0.0766 | 0.3145 | 0.0661 |
130–159 | 0.3616 | 0.3235 | 0.3576 | 0.3081 | 0.3727 | 0.3200 |
160–189 | 0.3471 | 0.8157 | 0.3892 | 0.7928 | 0.3339 | 0.8117 |
≥190 | 1.0491 | 0.8264 | 1.0253 | 0.8438 | 0.9747 | 0.8235 |
HDL cholesterol (mg/dl) | ||||||
<40 | 0.1464 | 0.3198 | 0.1675 | 0.3076 | 0.1618 | 0.3049 |
40–59 | Referent | Referent | Referent | Referent | Referent | Referent |
≥60 | −0.0266 | 0.0136 | −0.0066 | 0.0489 | −0.0367 | −0.0091 |
Blood pressure | ||||||
Normal | Referent | Referent | Referent | Referent | Referent | Referent |
Prehypertension | 0.0862 | 0.3396 | 0.1381 | 0.3357 | 0.1056 | 0.3440 |
Hypertension | 0.4514 | 0.4857 | 0.6142 | 0.5133 | 0.4301 | 0.4819 |
Diabetes mellitus | 0.9263 | 0.5903 | 1.1888 | 0.7146 | 0.9296 | 0.5733 |
Current smoker | 0.4441 | 0.3825 | 0.3988 | 0.3555 | 0.4494 | 0.3797 |
Albuminuria | ||||||
Microalbuminuria | 0.4437 | 0.2049 | — | — | 0.4284 | 0.2034 |
Macroalbuminuria | 1.0640 | 0.6321 | — | — | 0.9942 | 0.6348 |
eGFR (MDRD equation) (ml/min/1.73 m 2 ) | ||||||
≥60 | — | — | Referent | Referent | Referent | Referent |
<60 | — | — | 0.5235 | 0.3461 | 0.3093 | 0.1721 |
Baseline survival function at 10 years, S 0 (10 years) | 0.9938 | 0.9946 | 0.9897 | 0.9935 | 0.9924 | 0.9939 |
Prediction models based on the underlying equation and coefficients used, and whether eGFR and albuminuria were included, were compared using the c-statistic, Hosmer-Lemeshow chi-square statistic, and NRI and IDI ( Table 3 ). C-statistics for models using albuminuria, eGFR, or both were slightly higher than models using conventional risk factors alone in women; in men, the improvement was seen only for albuminuria and the combination. In contrast, measures of discrimination showed that without adjustments, the Framingham CHD risk model performed poorly in this population. After adjustments for the prevalence of risk factors and the incidence of CHD in American Indians (model 3), CHD prediction was improved significantly. The Hosmer-Lemeshow statistics yielded by the addition of eGFR alone (model 5) showed better calibration for men and women than the addition of eGFR and albuminuria (model 6).
C-Statistic | Hosmer-Lemeshow (p Value) | NRI ⁎ (p Value) | IDI (p Value) | |
---|---|---|---|---|
Women | ||||
Model 1 † | 0.684 | 25.6 (0.0012) | — | — |
Model 2 ‡ | 0.711 | 9.16 (0.3287) | — | — |
Model 3 § | 0.713 | 7.20 (0.5152) | Model without albuminuria or eGFR | Model without albuminuria or eGFR |
Model 4 ∥ | 0.727 | 14.6 (0.0667) | 0.129 (<0.0001) | 0.022 (<0.0001) |
Model 5 (eGFR <60 ml/min/1.73 m 2 ) ¶ | 0.718 | 10.9 (0.2070) | 0.085 (0.0004) | 0.010 (0.0003) |
Model 6 # | 0.728 | 18.5 (0.0175) | 0.135 (<0.0001) | 0.027 (<0.0001) |
Men | ||||
Model 1 † | 0.663 | 40.7 (<0.0001) | — | — |
Model 2 ‡ | 0.697 | 11.3 (0.1879) | — | — |
Model 3 § | 0.689 | 14.6 (0.0667) | Model without albuminuria or eGFR | Model without albuminuria or eGFR |
Model 4 ∥ | 0.699 | 19.4 (0.0130) | 0.017 (0.1395) | 0.008 (0.0067) |
Model 5 (eGFR <60 ml/min/1.73 m 2 ) ¶ | 0.688 | 14.1 (0.0781) | 0.010 (0.1967) | −0.0005 (0.3558) |
Model 6 # | 0.696 | 22.8 (0.0036) | 0.035 (0.0196) | 0.008 (0.0156) |
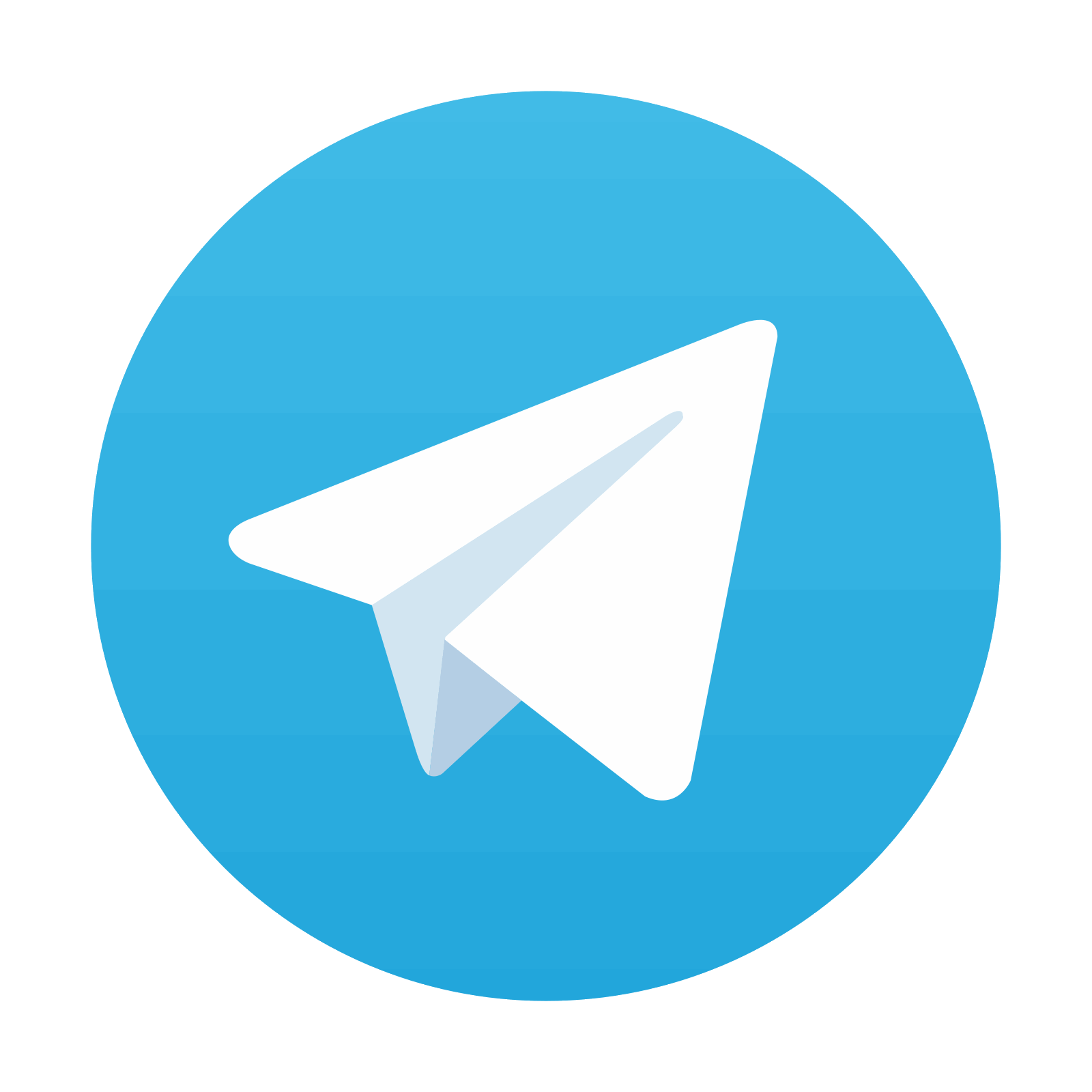
Stay updated, free articles. Join our Telegram channel

Full access? Get Clinical Tree
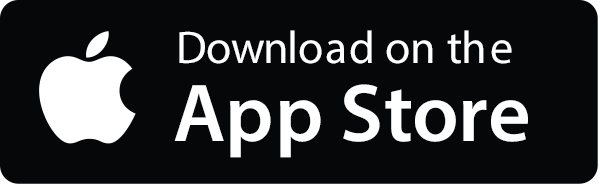
