Current cardiovascular randomized trials typically use composite outcomes. We hypothesized that the Bypass Angioplasty Revascularization Investigation (BARI) outcomes and conclusions would differ using a multistate model relative to the intervention for the composite outcome of death (D) and nonfatal Q-wave myocardial infarction (MI). We used a multistate model which uses transition paths to simultaneously assess multiple end points. Using the 10-year follow-up BARI data, we post hoc analyzed outcomes according to 3 transition paths: (1) from intervention to MI; (2) from intervention to death; and (3) from MI to death. Of 1,829 patients randomized to the intervention of percutaneous transluminal coronary angioplasty or coronary artery bypass grafting (CABG), 700 (38%) experienced the composite event D/MI which included 230 (13%) nonfatal MI and 470 (26%) death without antecedent nonfatal MI, whereas 79 of 230 (34%) experienced death after nonfatal MI. Outcomes of the 3 individual transition paths were analyzed by a multistate model. In contrast to standard survival analyses, after adjustment for baseline clinical covariates, outcomes after percutaneous transluminal coronary angioplasty or CABG were not significantly different for intervention to MI (p = 0.33) or intervention to death (p = 0.23), but MI to death favored CABG (p = 0.02). Deconstruction of the BARI data using a multistate model identifies a significant difference in individual transition-stage outcomes and therefore trial conclusions in contrast to the standard methods of survival analysis. These observations suggest multistate models should be considered in the design and analysis of randomized cardiovascular trials which use composite outcomes.
Multiple end points, such as death (D), nonfatal stroke, and nonfatal myocardial infarction (MI), are ubiquitous in cardiovascular clinical trials. To detect a treatment effect, composite outcomes, for example, death, stroke, MI, are commonly used. A given therapeutic intervention may selectively influence one of individual outcomes but not the other(s), potentially resulting in erroneous intervention conclusions. With more than 1 outcome of interest, separate analyses are ideally performed for each outcome; however, these are typically underpowered, and separate analyses fail to take into account correlations between different outcomes. In contrast, composite outcomes may disguise the treatment effect for specific outcomes. Multistate models analyze data with survival as the ultimate outcome when intermediate state(s) are identified. The treatment effect is investigated through patients’ transitions in various states. Previous work in a variety of noncardiologic medical interventions has identified the value of this modeling. For example, Anderson et al applied a multistate model to study liver cirrhosis and Putter et al used a multistate model to investigate the treatment for breast cancer. Multistate models have been used in a wide variety of health condition trials for both accuracy and cost considerations. We hypothesized that the Bypass Angioplasty Revascularization Investigation (BARI) outcomes and conclusions would differ using a multistate model to deconstruct the individual transitions relative to the intervention for mortality and the composite outcome of death and nonfatal Q-wave MI.
Methods
The BARI, a randomized clinical trial in 1,829 patients with multivessel coronary artery disease, tested coronary artery bypass grafting (CABG; n = 914) versus percutaneous transluminal coronary angioplasty (PTCA; n = 915) at 18 centers in the United States and Canada with 10-year follow-up. The primary outcome was mortality from all causes, and the secondary outcome was a composite measure of mortality from all-cause and the diagnosed nonfatal Q-wave MI. For illustration and comparison, we first conducted the Kaplan–Meier and Cox regression analyses for both the primary and secondary outcomes. Then, we conducted the analysis of the BARI data using a multistate model, which has been intensively investigated under the framework of stochastic (or random) processes.
Multistate models evaluate the movement of patients in various states. There are generally 3 kinds of states: (1) initial, (2) intermediate (or transitional), and (3) final (or absorbing). The initial state is defined to be the starting status for each patient, for example, just after randomization and before intervention. An example of an intermediate or transitional state is development of Q-wave nonfatal MI. Should the patient progress to death, the development of Q-wave nonfatal MI is an intermediate state. An example of the final or absorbing state is when the patient cannot move to other states once reaching this state, for example, death.
There are different types of multistate model formulations. The simplest one is a 2-state model with only 1 transition from the intervention state to the death state, illustrated in Figure 1 . The standard survival model belongs to this category. Another example is the competing risk model with an initial state and several absorbing states, such as death from different causes, illustrated in Figure 1 . The illness–death model is a multistate model with 1 initial state, 1 intermediate state, and 1 absorbing state, illustrated in Figure 1 . There are also multistate models with more than 1 intermediate state, such as repeated events and the bone marrow transplantation model.
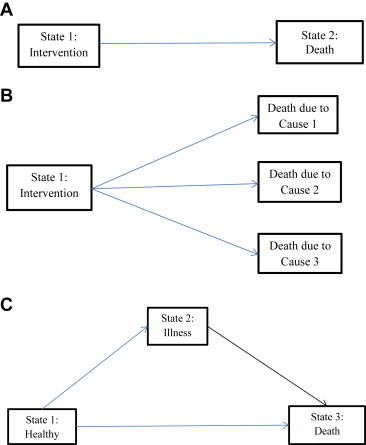
The BARI data belong to the illness–death model with the initial state being the intervention initiation, the intermediate state being diagnosed Q-wave nonfatal MI, and the final state being death. Figure 2 presents the illness–death graph for BARI data with corresponding sample sizes and event percentages for 3 transition paths—from intervention to death, from intervention to MI, and from MI to death. Both the Kaplan–Meier and Cox regression analyses are conducted for each transition path. We choose p values ≤0.05 to indicate statistical significance. We further stratified results according to procedural MI defined within 30 days after CABG (procedural CABG-MI) and within 7 days after PTCA (procedural PTCA-MI) for the transition path from MI to death. The multistate model analysis is carried out using the mstate R-package (R Development Core Team, 2010, Vienna, Austria).
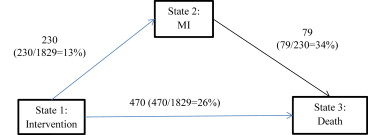
Results
Table 1 presents the baseline characteristics of the 1,829 patients from BARI data and shows that patients from CABG and PTCA groups were comparable regarding to their baseline (preintervention state) aspects. The Kaplan–Meier curves with 10-year follow-up for mortality and the composite outcome D/MI are illustrated in Figure 3 and demonstrate no significant treatment effects.
Coronary artery bypass grafting (n=914) | Percutaneous transluminal coronary angioplasty (n=915) | |
---|---|---|
Age (yr) mean (sd) | 60.6±9.6 | 61.3±9.2 |
Women | 26% | 27% |
White | 89.4% | 91.4% |
Black | 7.4% | 4.9% |
Other race | 3.2% | 3.7% |
High-school education or less | 71% | 71% |
Myocardial Infarction History | 55% | 54% |
Heart Failure | 9% | 9% |
Hypertension | 49% | 49% |
Renal Dysfunction | 2% | 3% |
Diabetes History | 20% | 19% |
Left Ventricular Ejection Fraction | 60.3 (12.5) | 60.2 (12.3) |
Cox regression analyses for mortality and the composite outcome D/MI adjusted by treatment, age, gender, high school education, race, previous history of MI, heart failure, hypertension, history of diabetes, renal dysfunction, and left ventricular (LV) ejection fraction are detailed in Table 2 . They show that the treatment effects for PTCA versus CABG are not significant for either mortality or composite outcome D/MI. For both outcomes, being older, having heart failure, hypertension, diabetes, renal dysfunction, and smaller LV ejection fraction are significantly related to shorter survival, but being female is significantly related to longer survival for the outcome being mortality and is not significant for the composite outcome. Because Q-wave nonfatal MI is time related, we then conducted the Cox regression analysis for mortality as the outcome adjusted by the time-dependent covariate nonfatal Q-wave MI with other covariates. Table 3 demonstrates that the time-dependent nonfatal Q-wave MI is significantly related to shorter survival, but there are no significant treatment effects for PTCA versus CABG. The other significant predictors include age, female, heart failure, hypertension, diabetes, renal dysfunction, and LV ejection fraction.
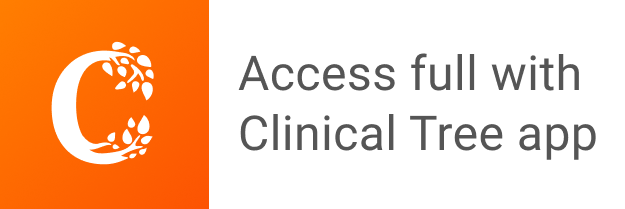