A risk score for atrial fibrillation (AF) has been developed by the Framingham Heart Study; however, the applicability of this risk score, derived using data from white patients, to predict new-onset AF in nonwhites is uncertain. Therefore, we developed a 10-year risk score for new-onset AF from risk factors commonly measured in clinical practice using 14,546 subjects from the Atherosclerosis Risk In Communities (ARIC) study, a prospective community-based cohort of blacks and whites in the United States. During 10 years of follow-up, 515 incident AF events occurred. The following variables were included in the AF risk score: age, race, height, smoking status, systolic blood pressure, hypertension medication use, precordial murmur, left ventricular hypertrophy, left atrial enlargement, diabetes, coronary heart disease, and heart failure. The area under the receiver operating characteristics curve (AUC) of a Cox regression model that included the previous variables was 0.78, suggesting moderately good discrimination. The point-based score developed from the coefficients in the Cox model had an AUC of 0.76. This clinical risk score for AF in the Atherosclerosis Risk In Communities cohort compared favorably with the Framingham Heart Study’s AF (AUC 0.68), coronary heart disease (CHD) (AUC 0.63), and hard CHD (AUC 0.59) risk scores and the Atherosclerosis Risk In Communities CHD risk score (AUC 0.58). In conclusion, we have developed a risk score for AF and have shown that the different pathophysiologies of AF and CHD limit the usefulness of a CHD risk score in identifying subjects at greater risk of AF.
Risk factors for atrial fibrillation (AF) include increasing age, male gender, obesity, hypertension, diabetes, and cardiac structural abnormalities, such as increased left ventricular wall thickness. Although the relation between some of these risk factors with incident AF has been well studied on a population level, formulas for predicting a person’s individual risk of AF are scarce. A risk score for AF has recently been developed by the Framingham Heart Study. The discrimination of the Framingham risk score was good for whites (C statistic 0.78); however, its utility to predict the 10-year risk of developing AF in nonwhites is uncertain. This is particularly relevant given the lower risk of AF among blacks. Therefore, we aimed to develop a risk score for predicting AF incidence using risk factors that can be easily assessed in clinical practice from a cohort of blacks and whites, the Atherosclerosis Risk In Communities (ARIC) study.
Methods
The ARIC study is a prospective cohort investigation aimed at identifying the risk factors for atherosclerosis and cardiovascular disease. ARIC recruited probability samples of adults aged 45 to 64 years from 4 United States communities: Forsyth County, North Carolina; Jackson, Mississippi; Minneapolis suburbs, Minnesota; and Washington County, Maryland. Blacks were oversampled from Forsyth County and exclusively sampled from Jackson. A total of 15,792 participants (8,710 women and 4,266 blacks) were enrolled from 1987 to 1989 and completed a home interview and clinic visit. Three triennial follow-up clinic visits were conducted (1990 to 1992, 1993 to 1995, and 1996 to 1998). In addition, the participants are followed-up by annual telephone interviews (with a >93% response rate) and active surveillance of the ARIC community hospitals. Institutional review boards at each participating center approved the ARIC study, and the participants provided informed consent at every clinic visit.
Electrocardiograms (ECGs) during the baseline visit were used to identify those subjects with prevalent AF or atrial flutter for exclusion. Incident AF diagnoses within 10 years of the baseline examination were identified from 3 sources: ECGs performed during the study follow-up visits through 1998, and hospital discharge records and death certificates through 10 years of follow-up.
All ARIC examination ECGs were recorded using Macintosh PC Personal Cardiographs (Marquette Electronics, Milwaukee, Wisconsin). At each clinic visit, a standard supine 12-lead resting ECG was recorded ≥1 hour after the subject had smoked tobacco or ingested caffeine. The ECGs were then transmitted by modem to the ARIC ECG Reading Center for computer coding. ECG recordings during follow-up that were computer coded as AF were visually rechecked by a cardiologist to confirm the diagnosis.
Annual follow-up telephone calls were made to the cohort participants to identify hospitalizations and deaths. In addition, the ARIC community hospitals were surveyed for potential cardiovascular events. Hospital discharge International Classification of Diseases codes were recorded for all hospitalizations, and AF was identified by an International Classification of Diseases , ninth revision, discharge code of 427.31 or 427.32 among any of the discharge diagnoses. AF was also identified when any listed cause of death on a death certificate was coded as AF ( International Classification of Diseases , ninth revision, code 427.3 or International Classification of Diseases , tenth revision, code I48). AF occurring simultaneously with heart revascularization surgery ( International Classification of Diseases , ninth revision, code 36.X) or other cardiac surgery involving the heart valves or septa ( International Classification of Diseases , ninth revision, code 35.X) was not considered an incident event, and follow-up was continued beyond that episode for incident AF not associated with cardiac surgery. A previous analysis within the ARIC cohort to determine the validity of hospital discharge diagnoses for AF reported 84% sensitivity and 98% specificity for the ascertainment of AF.
Study participants were asked to fast for 12 hours before the clinic visit, during which a blood sample was obtained and a physical examination performed. Blood collection and processing techniques for the ARIC study have been previously described. Enzymatic methods were used to measure total cholesterol and triglycerides. High-density lipoprotein cholesterol was measured enzymatically after dextran sulfate-Mg 2+ precipitation of other lipoproteins. Low-density lipoprotein cholesterol levels were estimated using the Friedewald formula for subjects with triglycerides levels <400 mg/dl. In a scrub suit and without shoes, standing height and waist circumference (at the level of the umbilicus) were measured to the nearest centimeter. Body mass index was calculated as weight (in kilograms) divided by height (in meters) squared.
Race, smoking status, and drinking status were determined by participant self-report. The sports index for physical activity during leisure time ranged from 1 (low) to 5 (high) and was determined using the questionnaire developed by Baecke et al. Blood pressure was measured 3 times with the subject in the sitting position after 5 minutes of rest using a random-zero sphygmomanometer, and the last 2 measurements were averaged. Participants were asked to bring all medications with them to the clinic visits. A prescription bottle or self-report was used to determine cholesterol and blood pressure medication use.
The presence of a systolic or diastolic murmur was identified during the physical examination by a trained clinician using a stethoscope. A 12-lead ECG at rest was used to define the PR interval and the presence of left ventricular hypertrophy (LVH) and left atrial enlargement (LAE). ECG-diagnosed LVH was considered present if the Cornell voltage was >28 mm in men or >22 mm in women. LAE found on the ECG was defined as a P-wave duration of ≥120 ms. A participant was categorized as diabetic if they had a fasting glucose of ≥126 mg/dl (or nonfasting glucose level of ≥200 mg/dl), reported a physician diagnosis of diabetes, or was currently taking medication for diabetes. Prevalent coronary heart disease (CHD) at baseline included a history of myocardial infarction, myocardial infarction adjudicated from the baseline ECG, or a history of coronary bypass or angioplasty. Prevalent heart failure was identified using the Gothenburg criteria or self-report of heart failure medication use in the previous 2 weeks.
All analyses were conducted using Statistical Analysis Systems, version 8.2 (SAS Institute, Cary, North Carolina). For the development of this risk score, we considered the following variables at baseline: age (45 to <50 [reference], 50 to <55, 55 to <60, 60 to 64 years), gender (male, female [reference]), race (black, white [reference]), body mass index (<20, 20 to <25 [reference], 25 to <30, ≥30 kg/m 2 ), height (<164 [reference], 164 to <173, ≥173 cm), waist circumference (<88/<102 [reference], ≥88/102 cm in men/women), sports score (<2.0, 2.0 to <3.0, 3.0 to <4.0, ≥4.0 [reference]), smoking status (current, former, never [reference]), drinking status (current, former, never [reference]), systolic blood pressure (<100, 100 to <120 [reference], 120 to <140, 140 to <160, ≥160 mm Hg), diastolic blood pressure (<70, 70 to <80 [reference], 80 to <90, 90 to <100, ≥100 mm Hg), hypertension medication use (no [reference], yes), total cholesterol (<200 [reference], 200 to <240, ≥240 mg/dl), low-density lipoprotein cholesterol (<100 [reference], 100 to <130, 130 to <160, 160 to <190, ≥190 mg/dl), high-density lipoprotein cholesterol (<40, 40 to <60, ≥60 mg/dl [reference]), triglycerides (<150 [reference], 150 to <200, ≥200 mg/dl), cholesterol medication use (no [reference], yes), precordial murmur (no [reference], yes), heart rate (<60, 60 to <90 [reference], ≥90 beats/min (beats/min]), PR interval (<160 [reference], 160 to <200, ≥200 ms), LVH on ECG (no [reference], yes), LAE on ECG (no [reference], yes), diabetes (no [reference], yes), CHD (no [reference], yes), and heart failure (no [reference], yes). Continuous variables were categorized according to clinical criteria or the distribution of the variable in the ARIC study.
Of the 15,792 ARIC participants, we excluded those who were not of black or white race (n = 48), blacks from Minneapolis and Washington County (n = 55), those with prevalent AF (n = 37) or missing AF status (n = 244) at baseline, those with unreadable ECGs (n = 85), and those with missing values for any variable in our final risk score (n = 777). Person-years of follow-up were computed from the baseline examination until a first AF diagnosis, death, lost to follow-up, or a follow-up of 10 years, whichever came first. Univariate associations of AF with potential risk factors were run first using Cox proportional hazards models. Significant (p <0.10) risk factors from the univariate models were then pooled into a single multivariate Cox model, and a backward stepwise elimination was used to identify significant (p <0.10) predictors in our multivariate model. All possible interactions of risk factors with age and race were then tested. Interaction tests between risk factors identified in our multivariate model and log of follow-up time confirmed the proportional hazards assumption was met.
Once the final Cox model was determined, we followed the method used by the Framingham Heart Study to calculate points associated with each level of our risk factors and to determine the 10-year probability of developing AF by point total. We calculated a score for all participants in our data set by calculating a point total using the risk score. The discrimination of both the Cox regression model and the actual point-based risk score was estimated using the area under the receiver operating characteristics curve (AUC). The calibration, a measure of the goodness of model fit, was assessed by comparing the observed and predicted number of AF events in deciles of predicted risk, as calculated by the Grønnesby-Borgan chi-square statistic. We also calculated a point-based score for participants using the Framingham Heart Study’s AF, CHD, and hard CHD risk scores, as well as ARIC’s CHD risk score, to estimate how well these risk scores predicted AF compared to our newly developed AF risk score.
Finally, 1,000 bootstrap samples were generated, sampling subjects with replacement, to compare our Cox regression model to the point-based score and to conduct an internal validation of our risk score. Bootstrapping methods provide more stable estimates with a lower bias compared to other methods of internal validation. However, because we used the same cohort to generate the 1,000 bootstrap samples for validation of our risk score that we had used to develop the risk score, we adjusted our AUC obtained from the internal validation for optimism.
Results
After exclusions, 14,546 subjects remained at risk of AF. During the 10 years of follow-up, 515 incident AF events occurred. The baseline characteristics of the study sample, along with age-, race-, and gender-adjusted hazard ratios for AF by potential risk factor category, are listed in Table 1 . The final risk score model included the following variables: age, race, height, systolic blood pressure, hypertension medication use, smoking status, precordial murmur, LVH and LAE on the ECG, diabetes, CHD, and heart failure. An interaction with race and LVH and interactions of diabetes and CHD with age were also found. Table 2 lists all risk factors in our risk score, along with the points derived for each category. The oldest age group (60 to 64 years) had the greatest points assigned of any risk factor category. Blacks were given a point value of −4, indicating a lower risk of developing AF compared to whites. The presence of ECG-diagnosed LVH increased the probability of developing AF in whites, but not blacks. Also, diabetes and CHD were associated with AF in younger subjects but not among the oldest in our cohort.
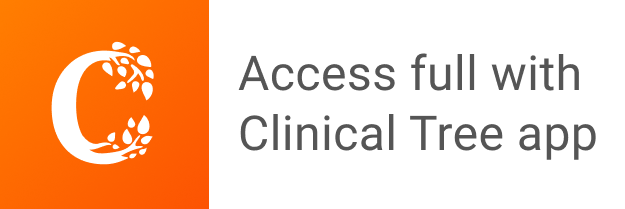