The predictive effect of non–high-density lipoprotein cholesterol (non-HDL-C) for cardiovascular disease (CVD) in Chinese general population has not been well demonstrated. The aim of our study was to examine the relation between non-HDL-C and CVD and compare the predictive effect of non-HDL-C and low-density lipoprotein cholesterol (LDL-C) for CVD in Chinese population. The baseline examination of 27,020 participants aged 35 to 74 years from the China Cardiovascular Health Study and the China Multicenter Collaborative Study of Cardiovascular Epidemiology was conducted in 1998 to 2001. Follow-up evaluation was conducted in 2007 to 2008 with a response rate of 79.8%. Cox proportional hazards regression models were used to obtain the multivariable-adjusted hazard ratios and 95% confidence intervals (CIs) for CVD. Compared with those with non-HDL-C level of <130 mg/dl, multivariable-adjusted hazard ratios of CVD were 1.30 (95% CI 1.04 to 1.62) and 1.93 (95% CI 1.50 to 2.47) in participants with non-HDL-C levels of 160 to 189.9 and ≥190 mg/dl, respectively. An increase of 30 mg/dl in non-HDL-C level would correspond to 15%, 24%, and 12% increase in risk of CVD, coronary heart disease, and stroke, respectively. Using likelihood ratio tests, non-HDL-C appeared to be a similar predictor for CVD incidence as LDL-C (chi-square for non-HDL-C, 18.02, p <0.001; chi-square for LDL-C, 18.90, p <0.001). In conclusion, higher non-HDL-C level is associated with the increased CVD incidence and has a similar effect as LDL-C on predicting CVD risk in Chinese.
Cardiovascular diseases (CVDs) contribute to the greatest proportion of deaths related to noncommunicable diseases. Although dyslipidemia has been identified as a major risk factor for CVD, whether low-density lipoprotein cholesterol (LDL-C) and non–high-density lipoprotein cholesterol (non-HDL-C) should be both used as the predictors for CVD primary prevention is still under consideration. Many studies showed that non-HDL-C was more predictive than LDL-C in coronary heart disease (CHD) incidence, CHD mortality, CVD incidence, and CVD mortality. In contrast, few studies observed that LDL-C calculated by Friedewald equation was superior to non-HDL-C in predicting CVD. Several studies among Chinese population suggested that non-HDL-C was a stronger predictor than LDL-C for prediction of CHD among residents living in township and for prediction of ischemic stroke in an occupational cohort. The association between non-HDL-C and CVD is still not clear among Chinese general population. The objective of the present study was to investigate the association of non-HDL-C levels with CVD incidence and to further compare the predictive effect of non-HDL-C with that of LDL-C in 2 large cohorts among Chinese general population.
Methods
Participants for the current analysis were from 2 cohorts: the China Cardiovascular Health Study and the China Multicenter Collaborative Study of Cardiovascular Epidemiology (ChinaMUCA). Details of the design and survey methods of the China Cardiovascular Health Study and the ChinaMUCA study have been described previously. Briefly, the China Cardiovascular Health Study used a 4-stage stratified sampling method to select a nationally representative sample of the general population aged 35 to 74 years in China during 2000 to 2001. A total of 15,540 participants from 10 provinces completed the survey and examination. The ChinaMUCA study was conducted in 15 clusters selected on the basis of geographic region and urbanization of China in 1998. Approximately 1,000 participants aged 35 to 59 years (nearly half were men) were sampled in each cluster using cluster random sampling. A total of 11,480 participants were used for subsequent follow-up survey.
In 2007 and 2008, participants from the China Cardiovascular Health Study and the ChinaMUCA study were invited to participate in the follow-up survey. Overall, 27,020 participants (13,063 men and 13,957 women) aged 35 to 74 years at baseline examination were eligible for the follow-up study. Of 21,556 (79.8%) study participants (or their proxies) who were followed up, 2,288 participants with missing information on non-HDL-C or LDL-C values (n = 981), not passing quality control (n = 982), or having CVD at baseline (n = 325) were excluded. Finally, a total of 19,268 participants were included in the current analysis. This study was approved by the Cardiovascular Institute and Fuwai Hospital Ethnics Committee. Written informed consent was obtained from each participant before data collection.
Baseline data were collected in examination centers at local health stations or community clinics. A standard questionnaire was administered to obtain information on demographic characteristics (including age, gender, education, and occupation and personal medical history), and lifestyle risk factors (including cigarette smoking, alcohol consumption, and physical activity) by trained staff. Education was classified as high school education or less than high school education. High school education was defined as ≥10 years of education received. Smoking was defined as having smoked at least 1 cigarette per day for at least 1 year or having smoked at least 400 cigarettes during lifetime, and 1 cigarette was considered to be equivalent to 1.25 g of tobacco. Alcohol consumption was defined as drinking alcohol at least once a week over the last year. Hypertension was defined as systolic blood pressure (BP) ≥140 mm Hg and/or diastolic BP ≥90 mm Hg and/or use of antihypertensive medication within the past 2 weeks. Type 2 diabetes was defined as a fasting glucose ≥7.0 mmol/L, previously diagnosed diabetes mellitus, or the self-reported use of insulin or oral hypoglycemic agents. Work-related physical activities were assessed because leisure-time physical activities were uncommon at that time. Work-related physical activities in the past year were classified into vigorous activity, moderate activity, light activity, sedentary activity, and invalid based on participants’ occupation.
Participants were invited to take a physical examination after the interview. Body weight and height were measured to the nearest 0.5 kg and 0.5 cm, respectively, with the participant wearing lightweight clothing and no shoes. Body mass index (BMI) was calculated as weight in kilograms divided by height in square meters. Three BP measurements were obtained using standard mercury sphygmomanometer according to a standard protocol, and the mean of 3 BP measurements was used in all analyses.
Overnight fasting blood samples were drawn by venipuncture to measure serum total cholesterol (TC), HDL-C, triglyceride (TG), and glucose. Blood specimens of participants from the China Cardiovascular Health Study were processed at the field center and shipped by air to the central clinical laboratory at the department of population genetics, Fuwai Hospital of the Chinese Academy of Medical Sciences in Beijing, where the specimens were stored at −70°C until laboratory assays could be performed. This laboratory participates in the Lipid Standardization Program of the US Centers for Disease Control and Prevention. TC, HDL-C, TG, and glucose levels were analyzed enzymatically on a Hitachi 7060 Clinical Analyzer (Hitachi High-Technologies Corp., Tokyo, Japan) using commercial reagents. TC, HDL-C, TG, and glucose levels of participants from the ChinaMUCA study were measured at local laboratory with stringent quality control. LDL-C levels were calculated using the Friedewald formula (LDL-C = TC − HDL-C − TG/5) for the participants whose TG levels were <400 mg/dl.
During the follow-up examination, study participants or their proxies were interviewed to ascertain disease status and vital information and obtain hospital records or death certificates verified by the local public health department or police department. If death occurred during a hospitalization, the participant’s hospital records and autopsy results were also abstracted. An end point assessment committee reviewed medical history information and death certificates. Two committee members assessed the end point independently, and discrepancies were discussed with additional committee members. The committee members were unaware of participants’ baseline characteristics for risk factors. Causes of death were coded according to the International Classification of Diseases, Tenth Revision ( ICD-10 ). All study investigators and staff have been trained and certified.
For this analysis, CVD was defined as a confirmed diagnosis of angina, acute myocardial infarction, stroke, or heart failure or mortality with a cardiovascular event ( ICD-10 I20 to I25, I46, I50, and I60 to I69) during the follow-up period. CHD was defined as a confirmed diagnosis of CHD or death due to CHD ( ICD-10 I20 to I25) during the follow-up period. In addition, stroke was defined as a confirmed diagnosis of stroke or death due to stroke ( ICD-10 I60 to I69) during the follow-up period.
Non-HDL-C levels were calculated as TC minus HDL-C. Participants were divided into 4 groups according to non-HDL-C levels (group 1: <130 mg/dl; group 2: 130 to 159.9 mg/dl; group 3: 160 to 189.9 mg/dl; group 4: ≥190 mg/dl) or LDL-C levels (group a: <100 mg/dl; group b: 100 to 129.9 mg/dl; group c: 130 to 159.9 mg/dl; group d: ≥160 mg/dl). Person-years of follow-up were calculated from the date of baseline examination to the date of cardiovascular event, death, or follow-up interview for each study participant.
Baseline characteristics were compared between non-HDL-C groups. Trend tests were examined using the Mantel-Haenszel chi-square test for categorical variables and linear trend test for continuous variables. In the linear trend test, the median value in each group was used as a continuous variable in the linear regression model. Cox proportional hazards regression models were used to examine effects of non-HDL-C and LDL-C levels on predicting CVD, CHD, and stroke events, with adjustment for age (<45, 45 to 54, 55 to 64, ≥65), gender, geographic region (north vs south), urbanization (rural vs urban), smoking status, alcohol consumption, education level, physical activity, BMI, and hypertension. Multivariable-adjusted hazard ratios (HRs) were measured using non-HDL-C <130 mg/dl or LDL-C <100 mg/dl as the reference categories. Then the HRs and corresponding group-specific proportions of participants with different non-HDL-C or LDL-C levels in our study population were used to estimate the population attributable risk (PAR) and 95% confidence intervals. The PAR was estimated using the following equation (in which P is the prevalence of different non-HDL-C or LDL-C groups): PAR = P × [HR − 1] ÷ ( P × [HR − 1]+1). We used the prevalence of our study for lack of prevalence data of non-HDL-C in the Chinese general population. Subgroup analysis according to gender (men vs women) and TG (<200 vs ≥200 mg/dl) was conducted using non-HDL-C <130 mg/dl as the reference.
Furthermore, sensitivity analyses were conducted for the association between non-HDL-C and CVD, CHD, and stroke incidence assessing the effect of (1)excluding participants who developed CVD within the first year of follow-up and (2)excluding participants who took lipid-lowering medication during follow-up.
In addition, we estimated HRs of per-unit (30 mg/dl) increase of non-HDL-C or LDL-C level for overall population and among subgroups for hypertension, diabetes, and BMI and TG. We also compared the predictive value of non-HDL-C and LDL-C for CVD incidence, using the Cox proportional hazards regression models with adjustment for the aforementioned multivariable. To compare the predictive effects between non-HDL-C and LDL-C, we used likelihood ratio test. The changes of the −2 logarithm likelihood (−2ln[L]) after adding non-HDL-C or LDL-C levels as continuous variables to the models, which follow an approximate chi-square distribution, were used to indicate the goodness of fit of predictive models. A bigger change indicates a better model fit.
Statistical analyses were conducted using SAS, version 9.3 (SAS Institute, Inc., Cary, North Carolina). All tests were 2 sided, and p <0.05 was considered statistically significant.
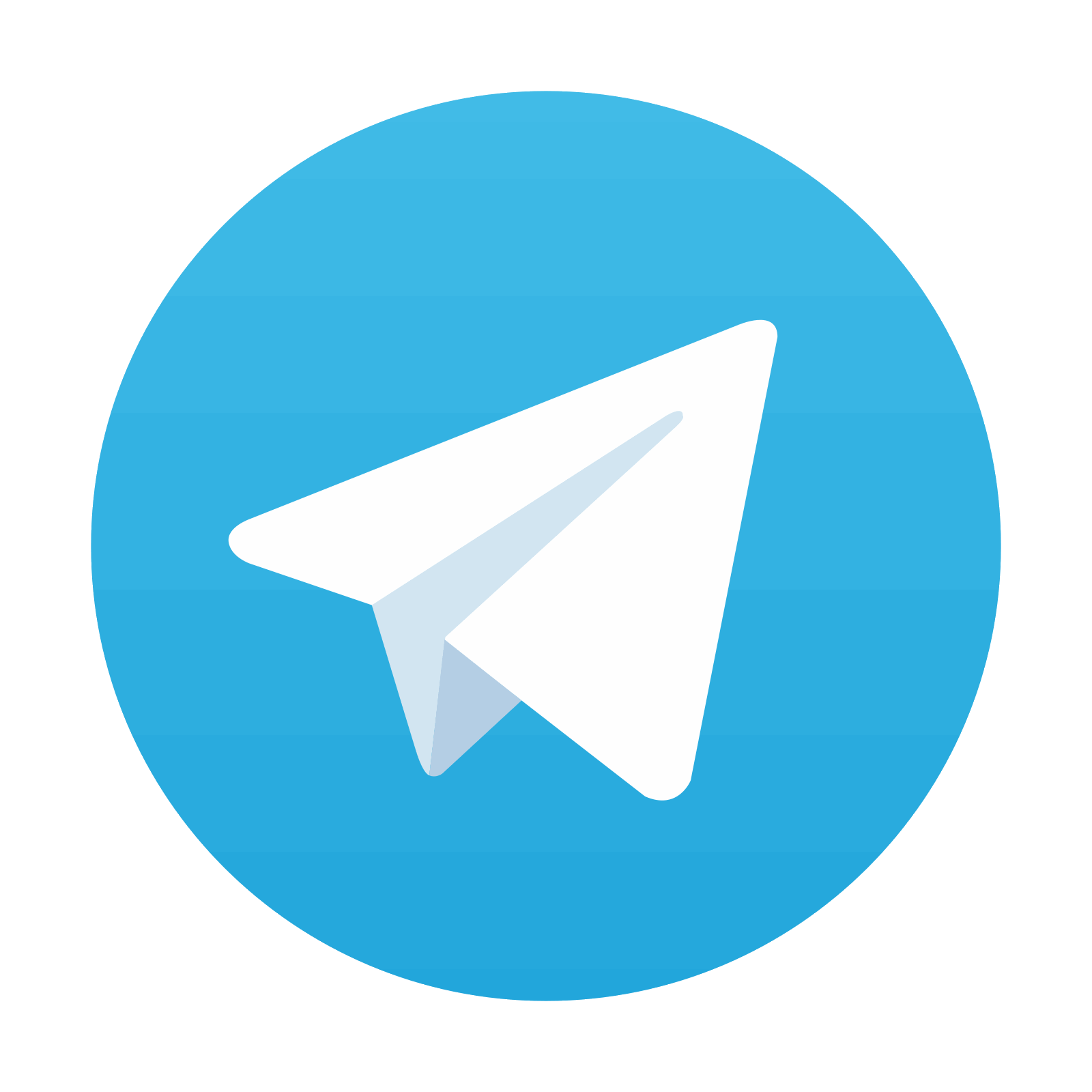
Stay updated, free articles. Join our Telegram channel

Full access? Get Clinical Tree
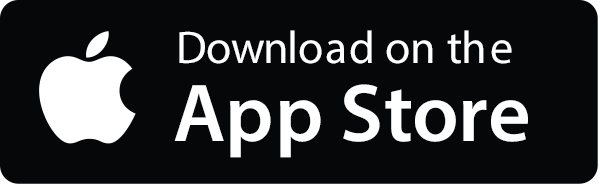
