Cardiovascular disease continues to be a major health problem, estimated to be responsible for about 30% of all global deaths. Currently, cardiovascular disease is the leading cause of death in the United States, and 17% of national health care expenditures are related to cardiovascular disease. The direct medical costs related to cardiovascular disease in the United States are projected to increase from $272.5 billion in 2010 to $818.1 billion by 2030; the indirect costs (lost productivity) are projected to increase from $171.7 billion to $275.8 billion over the same time period. Primary drivers for these increases in costs include the aging of the population, a growth in per capita medical spending, and the epidemic of such general medical problems as obesity and diabetes. Thus, selection of therapeutic strategies for patients with cardiovascular disease must be evidence based and requires consideration of comparative effectiveness, cost effectiveness, and involvement of the patient, when capable, in shared decision making. The appropriate balance of evidence, cost, and patient involvement has not yet been vigorously established. Furthermore, there is increasing recognition that although office practice remains valuable, the effective practitioner will use a variety of tools to create a “cardiovascular team” that can give the patient the best chance of avoiding disease progression and major cardiovascular events. This more complex environment, one that recognizes the value of teamwork in clinical care, creates a challenging but effective approach to improving decision making in cardiovascular care.
Therapeutic decision making in the office and hospital practice of cardiovascular medicine should proceed through an orderly sequence of events that begins with elicitation of the pertinent medical history and performance of a physical examination (Braunwald, Chapter 12 ). In the ideal situation, a variety of diagnostic tests are ordered, and the results are integrated into an assessment of the probability of a particular cardiac disease state. Based on this information and on an assessment of the evidence to support various treatments, a therapeutic strategy is formulated. In the arena of primary and secondary prevention, evidence points to the importance of reaching the patient at home or in the work environment by leveraging Internet-based technologies—such as e-mail, text messages, and so on—and midlevel providers. Despite the evolution in our understanding of these nontraditional settings, the fundamental structure by which evidence informs choices remains essential. The purpose of this chapter is to provide an overview of the quantitative tools used to interpret diagnostic tests, evaluate clinical trials, and assess comparative efficacy and cost effectiveness when selecting a treatment plan. The principles and techniques discussed here serve as a foundation for placing the remainder of the book in perspective, and they form the basis for the generation of guidelines for clinical practice. Appropriate application of the therapeutic decision-making tools that are described and adherence to the guideline documents based on the tools translate into improved patient outcomes, an area where cardiovascular specialists have distinguished themselves among the various medical specialties.
Interpretation of Diagnostic Tests
A useful starting point for interpreting a diagnostic test is the standard 2 × 2 table describing the presence or absence of disease, as determined by a gold standard, and the results of the test. Even before the results of the test are known, clinicians should estimate the pretest likelihood of disease based on its prevalence in a population of patients with clinical characteristics similar to the patient being evaluated. Because no diagnostic test is perfect, a variety of quantitative terms are used to describe its operating characteristics, thereby enabling statistical inference about the value of the test ( Figure 1-1 ). Sensitivity refers to the proportion of patients with the disease who have a positive test. Specificity is the proportion of patients without the disease who have a negative test. The probability that a test will be negative in the presence of disease is the false-negative rate , and the probability that a test will be positive in the absence of disease is the false-positive rate . Other useful terms are positive predictive value , which describes the probability that the disease is present if the test is positive, and negative predictive value , which describes the probability that the disease is absent when the test is negative. The Standards for Reporting of Diagnostic Accuracy (STARD) initiative sets forth guidelines on how studies of reports on diagnostic accuracy should be prepared.

Because the results of diagnostic tests are dependent on the profiles of patients being studied, the likelihood ratio has been introduced to express how many times more or less likely a test result is to be found in patients with disease compared with those without disease (see Figure 1-1 ; this is analogous to Bayes’ rule, in which the prior probability of a disease state is updated based on the conditional probability of the observed test result to form a revised or posttest probability of a disease state). By multiplying the pretest odds of disease by the likelihood ratio, clinicians can establish a posttest likelihood of disease and determine whether that likelihood crosses the threshold for treatment. For example, in a patient with chest discomfort, the presence of ST-segment elevation on the 12-lead electrocardiogram—the diagnostic test—not only increases the probability that myocardial infarction (MI)—the disease state—is present, but also moves the decision-making process to the treatment threshold for reperfusion therapy without the necessity for further diagnostic testing. In the same patient, a nondiagnostic electrocardiogram does not appreciably alter the posttest likelihood of an MI. Additional tests (e.g., biomarkers of cardiac damage) are needed to establish the diagnosis of MI.
The example shown in Figure 1-1 is for a diagnostic test that produces dichotomous results, either positive or negative. Many tests in cardiology provide results on a continuous scale. Typically, diagnostic cutoffs are established based on tradeoffs between sensitivity and specificity made with knowledge of the goal of the testing. In the example shown in Figure 1-2 , a diagnostic cutoff in the region of point A would have high sensitivity because it identifies the majority of patients with disease (true-positive results), but it does so at the expense of reduced specificity because it falsely declares the test to be abnormal in patients without disease. Using a range of diagnostic cutoffs for a positive test (e.g., see Figure 1-2, A to C ), a receiver operating characteristic (ROC) curve can be plotted to illustrate the relation between sensitivity and specificity. Better tests are those in which the ROC curve is positioned close to the top left corner. Comparison between two tests over a range of diagnostic cutoffs is accomplished by calculating the area under the ROC curve; the test with the larger area is generally considered to be superior, although at times the goal may be to optimize either sensitivity or specificity, even if one comes at the expense of the other. In practice, it is difficult for many clinicians to apply the quantitative concepts illustrated in Figure 1-2 at the bedside. This has led many laboratories to provide annotated reports to assist practitioners in forming a probabilistic estimate of the likelihood of a disease state being present.

Many diagnostic tests, risk scores, and models are used to predict future risk of events in patients who may or may not exhibit symptoms of cardiovascular risk. Important concepts when assessing the prognostic value of such tools include calibration, discrimination, and reclassification.
Calibration refers to the ability of the prognostic test or risk score to correctly predict the proportion of subjects within a given group to experience events of interest. Calibration assesses the “goodness of fit” of the risk model and is evaluated statistically using the Hosmer-Lemeshow test. In contrast to the usual situation, for example, in a classical superiority trial, a low χ value and corresponding high P values with the Hosmer-Lemeshow test are desirable, indicating little deviation of the observed data from the model and therefore a good fit.
Discrimination describes how well the model or risk score discriminates subjects at different degrees of risk. This is typically expressed mathematically with the C statistic, which literally ranks every pair of patients; it determines the proportion of pairs that have occurred with the predicted outcome in the person with the higher predicted probability from the test. In the case of a dichotomous outcome variable, this is equivalent to the area under the curve for an ROC curve. A test or model with no discrimination would have a C statistic of 0.50 because randomly choosing the patient more likely to have the outcome would be correct half the time, and a test that has perfect discrimination would have a C statistic of 1.0, indicating that every prediction correctly ranked the pairs.
Of particular interest to clinicians is a statement about the anticipated treatment effect of a therapeutic strategy stratified by a given risk score value. This is assessed via an interaction test. Given the large number of therapeutic options, burgeoning healthcare costs, and the emergence of an array of new risk markers, considerable efforts have been directed at refinement of the assessment of risk to provide treatments to those subjects at greatest risk. When new tests or models of risk are introduced, they are evaluated in terms of their ability to correctly reclassify subjects into higher or lower risk categories. Quantitative assessments of correct reclassifications are provided using the net reclassification improvement and integrated with classification improvement approaches. A critical component of reclassification estimates is the understanding of risk thresholds that should prompt a different clinical decision, such as recommending more tests, admitting the patient to the intensive care unit, adding a medication, or performing an invasive procedure. As this field evolves, the imprecision of clinical practice comes into focus because different physicians and patients have different views of these thresholds.
Clinical Trials
Need for Clinical Trials
Uncontrolled observation studies of populations provide valuable insight into pathophysiology and serve as the source for important hypotheses regarding the potential value of particular interventions; however, medical therapy rarely has the dramatic effectiveness of penicillin for pneumococcal pneumonia, for example, for which epidemiologic data alone are sufficient for scientific acceptance and adoption into clinical practice. In view of the variability of the natural history of cardiovascular illnesses and the wide range of individual responses to interventions, clinical investigators, representatives of regulatory agencies, and practicing physicians have come to recognize the value of a rigorously performed clinical trial with a control group before widespread acceptance or rejection of a treatment (Braunwald, Chapter 6 ).
A contemporary view of the clinical/translational spectrum of scientific investigations that result in therapeutic recommendations for various cardiovascular diseases is shown in Figure 1-3 . Basic biomedical advances that have successfully progressed through the discovery and preclinical phases are ready to cross the threshold to human investigation. A series of translational blocks—labeled T 1 , T 2 , T 3 , and T 4 —must be overcome for the biomedical discovery to ultimately improve global health. T 1 refers to research that yields knowledge about human physiology and the potential for intervention; it involves first-in-human and proof-of-concept experiments. T 2 research tests new interventions in patients with the disease under study to yield knowledge about the efficacy of interventions in optimal settings, such as phase II and many types of phase III trials (see Tables 1-1 and 1-2 ). T 3 research yields knowledge about the effectiveness of interventions in real-world practice settings. T 4 research focuses on factors and interventions that influence the health of populations.

PHASE | FEATURES | PURPOSE |
---|---|---|
I | First administration of a new therapy to patients | Exploratory clinical research to determine whether further investigation is appropriate (T 1 research) |
II | Early trials of new therapy in patients | Designed to acquire information on dose-response relationship, estimate incidence of adverse reactions, and provide additional insight into pathophysiology of disease and potential impact of new therapy (T 2 research) |
III | Large-scale comparative trial of new therapy | Definitive evaluation of new therapy to determine if it should replace standard of practice; randomized, controlled trials required by regulatory agencies for registration of new therapeutic modalities (T 2 research) |
IV | Monitoring the use of therapy in clinical practice | Postmarketing surveillance to gather additional information on impact of new therapy on treatment of disease; rate of use of new therapy and more robust estimate of incidence of adverse reactions established from registries (T 4 research) |
STAGE | ACTIVITIES DURING STAGE | EVENT MARKING END OF STAGE |
---|---|---|
Initial design | Formulation of scientific question, outcome measures established, sample size calculated | Initiation of funding |
Protocol development | Trial protocol and manual of operations written, case report forms developed, data management systems and monitoring procedures established, training of clinical sites completed | Initiation of patient recruitment |
Patient recruitment | Channels for patient referrals established; development of regular monitoring procedures of trial data for accuracy, patient eligibility, and site performance; preparation of periodic reports to DSMB for review of adverse or beneficial treatment effects | Completion of patient recruitment |
Treatment and follow-up | Continued monitoring of patient recruitment, adverse effects, and site performance; updated trial materials sent to enrolling sites; reports sent to DSMB and recommendations reviewed; adverse event reports filed with regulatory agency; timetable for trial close-out procedures established | Initiation of close-out procedures |
Patient/trial close-out | Identification of final data items that require clarification so database can be “cleaned and locked”; initiation of procedures for unblinding of treatment assignment, termination of study therapy, and monitoring of adverse events after discontinuation of treatment; preparation of final reports to DSMB; preparation of draft of final trial report | Completion of close-out procedures |
Termination | Verify that all sites have completed close-out procedures, including disposal of unused study drugs; review final trial findings and submit manuscript for publication; submit final report to regulatory agency | Termination of funding for original trial |
Posttrial follow-up (optional) | Recontact enrolling sites to acquire long-term follow-up on patients in trial; link follow-up data with initial trial data and prepare manuscript summarizing results | Termination of all follow-up |
Cardiovascular medicine has made a transition from practice based in large part on nonquantitative pathophysiologic reasoning to practice oriented around evidence-based medicine. The importance of this concept has been reinforced by clinical trials that have demonstrated widely accepted concepts to be associated with a substantial adverse effect on mortality rates (Braunwald, Fig. 6-5 ). The initial major alert about this issue occurred in the Cardiac Arrhythmia Suppression Trial (CAST), when type I antiarrhythmic drugs, often prescribed because of frequent premature beats, were demonstrated to increase the risk of death. Since then, the cardiovascular community continues to be surprised by the failure of therapies that seemed to be highly effective based on observation studies and selected small trials.
Despite current limitations, evidence-based therapeutic recommendations that involve drugs, devices, and procedures are in demand, with managed care, cost-saving measures, and guidelines published by authoritative groups playing increasingly prominent roles in the fabric of clinical medicine. Thus, the proper design, conduct, analysis, interpretation, and presentation of a clinical trial represent an “indispensable ordeal” for investigators. Practitioners must also acquire the tools to critically read reports of clinical trials and, when appropriate, to translate the findings into clinical practice without the lengthy delays that occurred in the past (T 3 research). This is an especially important task for generalist physicians because of the increased emphasis on primary care physicians to control health care costs by managing chronic disease with appropriate testing and referral.
The sheer volume and broad range of clinical trials in cardiology are too large for even the most conscientious individual to digest on a regular basis. This has stimulated increased interest in biostatistical techniques to combine the findings from randomized controlled trials (RCTs) of the same intervention into a meta-analysis or an overview.
Clinical Trial Design
When interpreting the evidence from a clinical trial, it is important to have a framework for dealing with a complex set of issues ( Figures 1-4 and 1-5 ). Because of the importance of clinical trial findings, it is essential that investigators thoughtfully formulate the scientific question to be answered and have realistic estimates of the sample size required to show the expected difference in treatments. Trials that result in the conclusion that the difference between treatment A and treatment B is not statistically significant are often undersized and lack sufficient power to detect a difference when one truly exists. A well-coordinated organizational structure made up of experienced trialists, biostatisticians, and data analysts is important to prevent pitfalls in trial design, such as unrealistic assessments of the ease of patient recruitment and the timetable for completion of the trial (see Figures 1-4 and 1-5 ).


The stages of a clinical trial are summarized in Table 1-2 . These should be viewed as a rough guide to the orderly sequence of events that characterizes the clinical trial process, as the dividing lines between stages are often indistinct. For example, sites at which patients are randomized may be brought into the trial in a rolling fashion such that some of the features of the protocol development stage may overlap with the patient recruitment phase. It is possible that some of the early sites that enroll patients gain sufficient experience with the protocol to achieve different results than those sites that join the trial later, as demonstrated in the Valsartan in Acute Myocardial Infarction (VALIANT) trial, which showed a clear learning curve characterized by a greater proportion of errors in trial protocol conduct in the initial phase of the trial. Evidence of this phenomenon is typically sought by performing a test for interaction between the enrolling site and treatment effect when the data are analyzed. The situation can rapidly become quite complex when international differences in treatment effect are observed, especially if benefit is noted predominantly in one international region and not in others. Of note, even after a fully executed development sequence from phase I through phase III trials, important adverse consequences of a new treatment may not be apparent. Although in theory, postmarketing trials (phase IV; see Table 1-1 ) are supposed to catch such problems and identify treatments that should be withdrawn from clinical use, such trials are infrequently conducted, leaving several authorities to propose new methods for surveillance of the safety of marketed medical products.
The term control group refers to participants in a clinical trial who receive the treatment against which the test intervention is being compared. Requirements for the control and test treatments are outlined in Box 1-1 . Randomized controlled trials typically incorporate both test and control treatments and are considered the gold standard for the evaluation of new therapies. However, the previously noted definition of a control does not require that the treatment be a placebo, although frequently this is the case because new treatments may have to be compared with the current standard of practice to determine whether they are more efficacious (e.g., new antithrombin agents versus unfractionated heparin; see Chapters 9 and 10 ) or fall within a range of effectiveness deemed to be clinically not inferior (e.g., a bolus thrombolytic versus an accelerated infusion regimen of alteplase; see Chapters 9 and 10 ). This definition does not require that the control group be a collection of participants distinct from the treatment group studied contemporaneously and allocated by random assignment. Other possibilities include nonrandomized concurrent and historic controls; crossover designs and withdrawal trials, with each patient serving as a member of both the treatment and control groups; and group or cluster allocations, in which a group of participants or a treatment site is assigned as a block to either test or control (Braunwald, Fig. 6-3 ).

They must be distinguishable from one another.
They must be medically justifiable.
There must be an ethical base for use of either treatment.
Use of the treatments must be compatible with the health care needs of study patients.
Both treatments must be acceptable to study patients and to physicians administering the treatment.
A reasonable doubt must exist regarding the efficacy of the test treatment.
There should be reason to believe that the benefits will outweigh the risks of treatment.
The method of treatment administration must be compatible with the design needs of the trial (e.g., method of administration must be the same for all the treatments in a double-blind trial), and they should be as similar to real-world practice as possible.
Two broad types of controlled trials exist: the fixed sample size design, in which the investigator specifies the necessary sample size before patient recruitment, and the open or closed sequential design, in which sequential pairs of patients are enrolled—one to test and one to control—only if the cumulative test-control difference from previous pairs of patients remains within prespecified boundaries. The sequential trial design is usually less efficient than the fixed sample size design and is practical only when the outcome of interest can be determined soon after enrollment. In addition, trials with the fixed design can be organized such that randomization and/or follow-up continue until the requisite number of endpoints is reached. This “event-driven” trial design ensures that inadequate numbers of endpoints will not hamper the trial interpretation.
Case-control studies , which involve a comparison of people with a disease or outcome of interest ( cases ) with a suitable group of subjects without the disease or outcome ( matched controls ), are integral to epidemiologic research; however, they are not strictly clinical trials and are therefore not discussed in this chapter.
Randomized Controlled Trials
The RCT is the standard against which all other designs are compared, for several reasons. In addition to the advantage of incorporating a control group, this type of trial centers around the process of randomization, which has the following three important influences:
- 1
It reduces the likelihood of patient selection bias that may occur either consciously or unconsciously in allocation of treatment.
- 2
It enhances the likelihood that differences between groups are random, so that comparable groups of subjects are compared, especially if the sample size is sufficiently large.
- 3
It validates the use of common statistical tests such as the χ test for a comparison of proportions and Student t test for a comparison of means.
Randomization may be fixed over the course of the trial, or it may be adaptive, based on the distribution of prior randomization assignments, baseline characteristic frequencies, or observed outcomes (Braunwald, Fig. 6-2 ). Fixed randomization schemes are more common and are specified further according to the allocation ratio (uniform or nonuniform assignment to study groups), stratification levels, and block size (i.e., constraining the randomization of patients to ensure a balanced number of assignments to the study groups, especially if stratification is used in the trial). Ethical considerations related to randomization have been the subject of considerable discussion in clinical trial literature.
Clinicians usually participate in an RCT if they are sufficiently uncertain about the potential advantages of the test treatment and can confidently convey this uncertainty to the research participants, who must provide informed consent. It is important that clinicians realize that in the absence of rigorously obtained data, many therapeutic decisions believed to be in the best interest of the patient may be ineffective or even harmful. To identify the appropriate therapeutic strategies from a societal perspective, RCTs are needed.
A difficult philosophic dilemma arises when patients are enrolled in a trial, evidence is accumulating that tends to favor one study group over the other, and the degree of uncertainty about the likelihood of benefit or harm is constantly being updated. Because clinicians may feel uneasy about enrolling a patient who may be randomized to a treatment that the accumulating data suggest might be inferior, although that has not yet been proved statistically to be so with a conventional level of significance, the outcome data from the trial are not revealed to the investigators during the patient recruitment stage. The responsibility of safeguarding the welfare of patients enrolled in the trial rests with an external monitoring team known as a data safety monitoring board (DSMB) or data safety monitoring committee (DSMC). Several prominent examples of the early termination of large RCTs because of compelling evidence of benefit or harm from one of the treatments under investigation are evidence that the DSMB has become an integral element of clinical trial research.
When both the patient and the investigator are aware of the treatment assignment, the trial is said to be unblinded . Trials of this nature have the potential for bias, particularly during the process of data collection and patient assessment, if subjective measures are tabulated, such as the presence or absence of congestive heart failure (CHF). Because blinding of one or more of the treatment arms in an RCT can be challenging, investigators may use a prospective, randomized, open-label blinded endpoint (PROBE) design. In an effort to reduce bias, progressively stricter degrees of blinding may be introduced. Single-blind trials mask the treatment from the patient but permit it to be known by the investigator, and double-blind trials mask the treatment assignment from both the patient and investigator. Triple-blind trials mask both of these and also mask the actual treatment assignment from the DSMB, and they provide data referenced only as “group A” and “group B.”
The specialty of cardiology is replete with examples of RCTs. The recent requirement for most clinical trials pertinent to the United States to be registered in the National Institutes of Health (NIH) managed registry, ClinicalTrials.gov, has enabled researchers to assess the portfolio of trials according to specialties, which include cardiovascular medicine. In a review of more than 96,000 registered trials, 58% had fewer than 100 volunteers, and 96% had fewer than 1000 participants. Cardiovascular trials are larger on average than other trials and more often have DSMCs, but as with all specialties, major gaps in evidence are relative to the need to inform decision making. An area particularly rich in this regard is the study of treatments for ST-segment elevation MI (see Chapter 10 ), in which multiple types of RCTs have been performed. However, in other areas, such as valvular and congenital heart disease, very few trials have been completed.
Efforts are under way to create an ontology to describe clinical research, but until this work is completed, it is useful to broadly classify these trials into minitrials and megatrials. A further subdivision of the minitrials includes those that are of limited sample size and focus almost exclusively on mechanistic data, and those with a sample size an order of magnitude larger and hybrid goals that focus on mechanistic data as they relate to clinical outcomes, such as mortality. In trials of new cardiovascular therapies, because of the practical limitations of the very large sample size required when death is used as the primary endpoint and the fact that other outcomes are important to patients and their families and to health care systems, interest has arisen in the use of composite endpoints, such as the sum of death, nonfatal recurrent MI, and stroke as the primary endpoint. Because most treatments have a modest effect (10% to 20%), it is important to be clear regarding the rationale for choice of endpoints; in some cases, such as treatment of angina and hypertension, endpoints other than death are essential. In acute treatment of ST-segment–elevation MI, however, understanding of the effect of size on death is required.
Nonrandomized Concurrent Control Studies
Trials in which the investigator selects the subjects to be allocated to the control and treatment groups are nonrandomized concurrent control studies . The advantages of this simpler trial design are that clinicians do not leave to chance the assignment of treatment in each patient, and patients do not need to accept the concept of randomization. Implicit in this type of design is the assumption that the investigator can appropriately match subjects in the test and control groups for all relevant baseline characteristics. This is a difficult task, and it can produce a selection bias that may result in conclusions that differ in direction and magnitude from those obtained from RCTs (Braunwald, Fig. 6-3 ).
Observation analyses contain many of the same structural characteristics as randomized trials, except that the treatment is not randomized. These studies should use prospectively collected data with uniform definitions managed by a multidisciplinary group of investigators that includes clinicians, biostatisticians, and data analysts. Outcomes must be collected in a rigorous and unbiased fashion, just as in the randomized trial.
Historic Controls
Clinical trials that use historic controls compare a test intervention with data obtained earlier in a nonconcurrent, nonrandomized control group (Braunwald, Fig. 6-3 ). Potential sources for historic controls include previously published medical literature and unpublished data banks of clinic populations. The use of historic controls allows clinicians to offer potentially beneficial therapies to all participants, thereby reducing the sample size for the study. Unfortunately, the capacity to understand the bias engendered in the selection of the control population is extremely limited, and failure of the historic controls to reflect contemporary diagnostic criteria and concurrent treatment regimens for the disease under study produces even more uncertainty. Thus, although historic controls are alluring, they should be used in the definitive assessment of a therapy only when a randomized trial is not feasible and a concurrent nonrandomized control is not available.
It should be noted, however, that prospectively recorded registry data may be more representative of actual clinical practice than the control groups in RCTs. Reports from such registries are useful for identifying gaps in translation into routine practice of therapies proven to be effective in clinical trials. Accordingly, it seems appropriate to use RCTs to define the effectiveness of a treatment and then to fill in gaps by means of carefully conducted observation studies with a preference for the use of comprehensive clinical practice registries.
Crossover Design
The crossover design is a type of RCT in which each participant serves as his or her own control (Braunwald, Fig. 6-3 ). A simple, two-period, crossover design randomly assigns each subject to either the test or control group in the first period and to the alternative in the second period. The appeal of this design lies in its ability to use the same subject for both test and control treatments, thereby diminishing the influence of interindividual variability and allowing a smaller sample size. However, important limitations to crossover design are the assumptions that the effects of the treatment assigned during the first period have no residual effect on the treatment assigned during the second period and that the patient’s condition remains stable during both periods. The validity of these assumptions is often difficult to verify either clinically or statistically (e.g., testing for an interaction between period and intervention); this has led some authorities to discourage the use of crossover designs, although one possible use of the crossover trial design is for the preliminary evaluation of new antianginal agents for patients with chronic, stable, exertional angina.
Withdrawal Studies
In withdrawal studies, patients with a chronic cardiovascular condition are either taken off therapy or undergo a reduction in dosage with the goal to evaluate the response to discontinuation of treatment or reduction in its intensity. An important limitation is that only patients who have tolerated the test intervention for a period of time are eligible for enrollment because those with incapacitating side effects would have been taken off the test intervention and would therefore not be available for withdrawal. This selection bias can overestimate benefit and underestimate toxicity associated with the test intervention. However, if the goal is to understand the duration of benefit of a treatment, or to assay a signal of efficacy without attempting to estimate the magnitude of the effect in a patient just beginning therapy, this design has an advantage.
In addition, changes in the natural history of the disease may influence the response to withdrawal of therapy. For example, if a therapeutic intervention is beneficial early after the onset of the disease but loses its benefit over time, the withdrawal of therapy late in the course of treatment might not result in deterioration of the patient’s condition. A conclusion that the intervention was not helpful because its withdrawal during the chronic phase of treatment did not result in a worsening of the patient’s condition provides no information about the potential benefit of treatment in the acute phase or subacute phase of the illness. Thus, withdrawal trials can provide clinically useful information, but they should be conducted with specific goals and with the same standards that are applied to controlled trials of prospective treatment, including randomization and blinding if possible.
One withdrawal trial in cardiology—the Randomized Assessment of Digoxin on Inhibitors of the Angiotensin-Converting Enzyme (RADIANCE)—illustrates many of the features previously discussed. Although digitalis has been used by physicians for more than 200 years, its benefits for the treatment of chronic CHF, particularly in patients with normal sinus rhythm, remain controversial. To assess the consequences of withdrawing digoxin from clinically stable patients with New York Heart Association functional class II to III CHF who are receiving angiotensin-converting enzyme inhibitors, investigators randomly allocated 178 patients in a double-blind manner to continue to receive digoxin or switch to a matched placebo. Worsening heart failure that necessitated discontinuation from the study occurred in 23 patients who were switched to placebo but in only four patients who continued to receive digoxin ( P < .001). The results of the RADIANCE trial seem to indicate that withdrawal of digoxin in patients with mild to moderate CHF as a result of systolic dysfunction is associated with adverse consequences, but it does not provide information on the potential mortality benefit of digoxin when added to a regimen of diuretics and angiotensin-converting enzyme inhibitors. One classic RCT, the Digitalis Investigation Group (DIG) trial, showed that digoxin therapy was not associated with a mortality benefit but did provide symptomatic improvement in that it reduced the need for hospitalization for decompensated CHF.
Factorial Design
When two or more therapies are tested in a clinical trial, investigators typically consider a factorial design , in which multiple treatments can be compared with controls through independent randomization within a single trial (Braunwald, Fig. 6-3 ).
Factorial design trials are more easily interpreted when there is believed to be no interaction between the various test treatments, as is often the case when drugs have unrelated mechanisms of action. If no interactions exist, multiple drug comparisons can be efficiently performed in a single, large trial that is smaller than the sum of two independent clinical trials. When interactions are detected, each intervention must be evaluated individually against a control and each of the other interventions in which an interaction exists.
The factorial trial design has an important place in cardiology, in which multiple therapies are typically given to the same patient for important conditions such as MI, heart failure, and secondary prevention of atherosclerosis. Therefore, in practical terms, the factorial design is more reflective of actual clinical practice than trials in which only a single intervention is randomized. Clinicians need to know how much incremental value comes from the administration of one more drug to the patient, and whether any drug interactions exist. It is worth noting, however, that it is probably an insurmountable task to rule out the possibility of a drug interaction because of the imprecision with which interaction effects are estimated (i.e., wide confidence intervals), the poor power of tests for statistical significance of interactions between the test interventions, and the vast number of non–protocol-related drugs a patient may receive.
Trials that Test Equivalence of Therapies
Advances in cardiovascular therapeutics have dramatically improved the treatment of various diseases, such that several therapies of proven efficacy may coexist for the same treatment. However, it may still be desirable to develop new therapies that are equally efficacious but have an important advantage, such as reduced toxicity, improved patient tolerability, a more favorable pharmacokinetic profile, fewer drug interactions, or lower cost. Testing such new therapies using placebo-controlled trials poses problems on ethical grounds because half of the patients would be denied treatment when an accepted therapy of proven efficacy exists. This has led to a shift in clinical trial design to demonstrate the therapeutic equivalence of two treatments rather than the superiority of one of the treatments.
It is not possible to show two active therapies to be completely equivalent without a trial of infinite sample size. Instead, investigators resort to specifying a value (δ) and consider the test therapy to be equivalent to the standard therapy if the true difference in treatment effects is less than δ with a high degree of confidence ( Figure 1-6, A ).

The nomenclature related to trials of tests of equivalence between two therapies can be confusing. In a classic equivalence trial, if the confidence intervals (CIs) for the estimate of the effects of the two treatments differ by more than the equivalence margin (δ) in either direction, then equivalence is said not to be present. For most clinical trials of new therapies, the objective is to establish that the new therapy is not worse than the standard therapy (active control) by more than δ. Such one-sided comparisons are referred to as noninferiority trials . The new therapy may satisfy the definition of noninferiority but, depending on the results, may or may not actually show superiority compared with the standard therapy.
Specification of the appropriate margin, or δ, is often problematic. Clinicians prefer to set δ based on a clinical perception of a minimally important difference they believe would affect their practice. Regulatory authorities, who are bound by a legal mandate “to show that drugs work,” assess the effect of the standard therapy based on prior trials, in which it was compared with placebo. Rather than specifying the point estimate for the full effect of the standard therapy over placebo, a more conservative approach is taken by selecting the lower bound of a CI for superiority of the standard therapy over placebo for setting the noninferiority margin.
Figure 1-6, B , provides an example of the design of noninferiority trials and interpretation of six hypothetical trial results; the difference in events between the test drug and the standard drug is plotted along the horizontal axis. Based on trials against placebo, the standard drug provides a benefit over placebo at the +4 position, but the lower bound of its superiority over placebo is at the +2 position; thus, the noninferiority margin is set at +2. The six hypothetical trials (A to F) are shown, with the point estimate of the difference between the test drug and standard drug displayed as filled squares, and the width of the 95% CI for the difference is shown as blue horizontal lines.
The results of trial A fall entirely to the left of zero (i.e., the upper bound does not enter the zone of noninferiority); thus, it is possible to declare the test drug to be superior to the standard drug. In trials B and C, the upper bound falls within the zone of noninferiority, and in loose parlance, the test drug is declared to be “equivalent” to the standard drug. Note that in trials D and E, the noninferiority requirement is not satisfied—that is, the upper bound exceeds the margin in trial D, and the entire CI exceeds the margin in trial E—and the test drug is said to be inferior to the standard drug.
It is important to prespecify the noninferiority margin before starting the trial; if it is specified after the results are known, the trial could be criticized for a potential subjective bias. For example, if the results of trial D were known, and the noninferiority margin was set at +3, rather than +2, the test drug would satisfy the definition of noninferiority, but such an approach would be highly suspect. It is also important to have a sample size sufficient to draw meaningful conclusions. For example, although the point estimate for trial F is in favor of the test drug, the wide CIs are due to a small sample size. Trial F does not allow the investigators to claim superiority of the test drug compared with the standard drug, and it would be inappropriate to claim it to be “equivalent” to the standard drug, simply because superiority could not be demonstrated (note that the upper bound of trial F clearly exceeds the noninferiority margin).
Investigators can prespecify that a trial is being designed to test superiority and noninferiority simultaneously. For a trial that is configured only as a noninferiority trial, it is acceptable to test for superiority at the conclusion of the trial. However, because of the subjective bias mentioned, the reverse is not true: trials configured for superiority cannot later test for noninferiority unless the margin was prespecified.
An important commonality between superiority and noninferiority trials is that the clinical experts involved in trial design should consciously consider the minimally important clinical outcome difference. A common understanding of the difference between outcomes with two therapies forms the basis for providing the appropriate perspective on the interpretation of test statistics; in essence, the difference between “statistically significant” and “clinically important” is determined by the common view of the difference that would lead to a change in practice.
Noninferiority trials, a more recent addition to the RCT repertoire, are prone to controversy, especially when disagreement exists over the noninferiority margin (i.e., the percentage of the treatment benefit of the gold standard therapy over placebo that would be retained by the new treatment and still be considered clinically equivalent). The reporting of noninferiority trials in the medical literature is often deficient and fails to provide an adequate justification for the noninferiority margin or the sample size. In a fashion similar to that for reporting a superiority trial, the Consolidated Standards of Reporting Trials (CONSORT) Group has published recommendations for a checklist and visual display of the results of noninferiority trials.
Of course, one of the most fragile assumptions in a noninferiority trial is the assumption of constancy, in which the trials that established the benefit of the gold standard over placebo are assumed to achieve the same result if the placebo-controlled trial were to be conducted in the era of the noninferiority trial. In essence, the statistical inference is based on a historic control, with all of the attendant issues previously discussed.
Selection of Endpoint
A critical decision when designing a clinical trial is the selection of the outcome measure. In trials comparing two treatments in cardiovascular medicine, the outcome measure, or endpoint of the trial, characteristically is a clinical event. The characteristics of an ideal primary outcome measure are that it is easy to diagnose, free of measurement error, observable independent of treatment assignment, and clinically relevant, and it should be selected prior to the start of data collection for the trial.
Improvements in cardiovascular treatments have, gratifyingly, led to a reduction in mortality rates and therefore a lower event rate in the control arm of clinical trials with an attendant increase in the required sample size. The desire to evaluate new therapeutic approaches in the face of rising costs to conduct large clinical trials has resulted in two primary approaches to the selection of endpoints. The first is to use a composite endpoint that combines mortality with one or more nonfatal negative outcomes, such as MI, stroke, recurrent ischemia, or hospitalization for heart failure. Trials with a logical grouping of composite endpoints that are likely to each be affected by the treatments being studied are clinically valuable and have been used to advance treatments for heart failure and acute coronary syndromes. However, interpretation of composite endpoints becomes problematic when elements of a composite endpoint go in opposite directions in response to treatment (e.g., reduced mortality but increased nonfatal MI). To date, no consensus exists on an appropriate weighting scheme for composite endpoints.
Another approach is to use a biomarker or putative surrogate endpoint as a substitute for clinical outcomes. A valid surrogate endpoint not only must be predictive of a clinical outcome, it must also evidence that modification of the surrogate endpoint captures the effect of a treatment on clinical outcomes because the surrogate is in the causal pathway of the disease process. Few biomarkers have met the high threshold for classification as a valid surrogate, but biomarkers remain highly valuable in developing therapies and therapeutic concepts. Examples of a successful surrogate endpoint and failed surrogate endpoints are schematically illustrated in Figure 1-7 (also see Braunwald, Fig. 6-5 ). Whether or not a surrogate endpoint is useful for determining whether a treatment is efficacious, a single surrogate cannot be used to develop a balanced view of risk and benefit, particularly compared with alternative therapies. This increasingly recognized critical element of therapeutic development and evaluation requires measurement of clinical outcomes in the relevant population over a relevant period of time.

Sample Size Estimations and Sequential Stopping Boundaries
Estimation of the sample size for trials involves a statement of the scientific question in the form of a null hypothesis (H 0 ) and an alternative hypothesis (H A ). For example, in the case of dichotomous variables (e.g., a primary outcome variable such as mortality), the null hypothesis states that the proportion of patients dying in the test group (PTest) is equal to that in the control group (PControl; see Figure 1-6, A ), such that for
H 0 : P Test − P Control = 0
The alternative hypothesis is that for
H A : P Test − P Control ≠ 0
False-Positive and False-Negative Error Rates and Power of Clinical Trials
To determine whether the null hypothesis may be rejected before initiation of the trial, the type I (α) and type II (β) errors, sometimes referred to as the false-positive and false-negative rates , are specified (see Figure 1-6, A ). The conventional α of 5% indicates that the investigator is willing to accept a 5% likelihood that an observed difference as large as that projected in the sample size calculation occurred by chance and would lead to rejection of the null hypothesis when, in fact, the null hypothesis was correct (see Figure 1-5 ). The β value reflects the likelihood that a specified difference might be missed or not found to be statistically significant because of an insufficient number of events in the trial at the time of analysis. The quantity (1 – β) is referred to as the power of the trial and quantifies the ability of the trial to find true differences of a given magnitude between the groups (see Figure 1-5 ). The relations among estimated event rates, the prespecified α level, and the desired power of the trial determine the number of patients who must be randomized to detect the anticipated difference in outcomes according to standard formulas. Similar concepts are applied to response variables that are not dichotomous but are measured on a continuous scale (e.g., blood pressure) or represent time to failure (e.g., Kaplan-Meier survival curves).
Statistical methods are also available for monitoring a trial during the patient recruitment phase at certain prespecified intervals to determine whether the accumulated evidence strongly suggests an advantage of one treatment in the trial. During such interim checks of the data, the differences between treatment groups, expressed as a standardized normal statistic ( Z i ), are compared with boundaries such as those shown in Figure 1-8 . If the Z i statistic falls outside the boundaries at an i th interim look, the DSMB may seriously consider recommending termination of the trial. Typically, the data are arranged as test: control , so crossing of the upper boundary denotes statistically significant superiority of the test therapy over the control, and crossing of the lower boundary denotes superiority of the control therapy over the test therapy. Because of the considerable expense of large clinical trials, in some cases it may be desirable to discontinue a trial at an interim analysis if the accumulated data suggest that the probability of a positive result, if the trial proceeds to completion, has become quite low. A futility index that describes the likelihood of a positive result based on accumulated data has been developed that allows investigators to discontinue a nonproductive trial and concentrate limited resources on alternative trial options.

Considerable clinical and statistical wisdom is required of DSMB members because they must consider and integrate five key aspects: 1) the consistency and timeliness of the trial data reviewed at each interim analysis, 2) random variation in event rates during the course of the trial, 3) the type and severity of the disease under study, 4) the magnitude of the benefit versus the risks of the therapy being investigated, and 5) emerging data from other trials and clinical experience. Whether to stop an RCT early because of an apparent strong treatment benefit favoring one of the arms is a complex decision. Although investigators, sponsors funding the trial, and journal editors are likely to become caught up in the excitement and publicity surrounding an announcement of early stopping of a trial for benefit, it should be noted that there is a precedent for unrealistically large treatment effects to be disproved by subsequent RCTs. A systematic review of RCTs stopped early for benefit reported that investigators often fail to report relevant information about the decision-making process, and such decisions to stop an RCT early tend to provide unrealistic estimates of the true treatment benefit, when the total number of events observed is small. In the case of new, unapproved treatments, early stopping for benefit may place regulatory authorities in the uncomfortable position of not having enough safety data on which to base approval of the new treatment.
To minimize the risk of overestimation of the treatment effect when a trial is stopped early, it has been proposed that a low P value threshold (e.g., <.001) be used such that stopping should not occur until a large number of endpoint events have been observed—for example, at least 200 to 300—and that enrollment and follow-up should continue for an additional period to be certain that a positive trend will continue after a threshold has been crossed.
Although it may occasionally appear that an extreme treatment effect is present in a particular subgroup, this must be interpreted cautiously to be certain that this effect is consistent with a prior hypothesis and that it remains significant after adjusting for multiple comparisons, interactions, and the interim nature of the analysis (Braunwald, Fig. 6-7). DSMB members must balance common sense, formal statistical stopping guidelines, ethical obligations to patients, and obligation to the clinical community to ensure that the willingness of patients to consent to participation in the trial leads to an advance in the state of knowledge about the optimal therapeutic strategy.
A controversial approach to the design, monitoring, and interpretation of clinical trials is the use of a Bayesian methodology. Compared with the classic or frequentist approach described earlier, Bayesian methods formally use prior information, specifying it as a prior probability distribution. Instead of presenting the results of the trial in the form of P values and CIs, Bayesian analysts present plots of the posterior distribution of the treatment effect. Interim monitoring procedures, such as those shown in Figure 1-8 for the frequentist approach, are replaced with posterior distribution plots. By using a “skeptical” prior probability, a conservative approach to stopping rules can be developed according to Bayesian analysis. At present, the frequentist approach is the standard approach accepted by regulatory authorities for the approval of new therapies because of concerns about the sources and uncertainties regarding the prior probability distribution, but particularly with devices, flexibility on this matter is increasing. In the future, a Bayesian approach may be used more frequently in RCT design and analysis.
How to Read and Interpret a Clinical Trial
To properly interpret a clinical trial report and to apply what has been learned in practice, clinicians must have a working knowledge of the statistical and epidemiologic terms used to describe the results. By reviewing the concepts illustrated in Figure 1-4 , asking three main sets of questions, such as those in Box 1-2 , adapted from the McMaster Group, and by summarizing the trial findings as per the example in Figure 1-9 , physicians will be equipped to integrate into their own practices the information in articles that describe clinical trials of cardiovascular therapeutics.

Are the Results of the Study Valid?
Primary Guides
- 1
Was the assignment of patients to treatment randomized?
- 2
Were all patients who entered the trial properly accounted for and attributed at its conclusion?
- ▪
Was follow-up complete?
- ▪
Were patients analyzed in the groups to which they were randomized?
- ▪
Secondary Guides
- 1
Were patients, their clinicians, and study personnel “blind” to treatment?
- 2
Were the groups similar at the start of the trial?
- 3
Aside from the experimental intervention, were the groups treated equally?
What Were the Results?
- 1
How large was the treatment effect?
- 2
How precise was the treatment effect?
Will the Results Help Me Care for My Patients?
- 1
Does my patient fulfill the enrollment criteria for the trial? If not, how close is my patient to the enrollment criteria?
- 2
Does my patient fit the features of a subgroup in the trial report? If so, are the results of the subgroup analysis in the trial valid?
- 3
Were all the clinically important outcomes considered?
- 4
Are the likely treatment benefits worth the potential harm and costs?

The physician must first determine that the study was of sufficient caliber to provide valid results and must extract the essential trial data and enter it into a 2 × 2 table. Figure 1-9 shows an example of 10,000 patients who met the enrollment criteria for a clinical trial and were randomized with an allocation ratio of 1 : 1, so that 5000 patients received treatment A and 5000 received treatment B. Because only 600 primary outcome events occurred in group A (12% event rate) and 750 occurred in group B (15% event rate), it appears that treatment A is more effective than treatment B. Is this difference statistically significant, and is it clinically meaningful? When the data are arranged in a 2 × 2 table (see Figure 1-9 ), a χ test or Fisher exact test can be readily performed according to standard formulas.
Although the investigators of the trial will likely have analyzed the results using one of the methods illustrated in Figure 1-9 , it is useful to have a measure of the precision of the findings and an impression of the potential impact of the results on clinical practice. Even a well-designed clinical trial can provide only an estimate of the treatment effect of the test intervention owing to random variation in the sample of subjects studied, who are selected from the entire population of patients with the same disease. The imprecision of the statement regarding treatment effect can be estimated and incorporated into the presentation of the trial results by calculating the 95% CIs around the observed treatment effect. If the 95% CIs are not reported in the trial, inspection of the P value may be useful to indicate whether the CI spans a null effect. Alternatively, the 95% CIs may be estimated as the treatment effect ± twice the standard error of the treatment effect (if reported), or it may be calculated directly.
Missing Data
Despite the best efforts at appropriate design and conduct of clinical trials, missing data occur for a variety of reasons. Trial subjects may not have a scheduled visit, or there may have been equipment failure that resulted in failure to ascertain data that might bear on a trial endpoint. Missing data are broadly classified based on the mechanism leading to their “missingness” (see Table 1-3 ). In general, when data are missing completely at random or missing at random, the impact on the assessment of the treatment effect is less than when the data are not missing at random. Although in theory, data missing at random or completely missing at random are “ignorable” and data not missing at random are not, in practical terms, investigators usually cannot rigorously test the assumptions that distinguish the different classes of missing data, which have been a concern in outcomes research (patients are not randomized) but also are of concern to regulatory authorities when they assess the data from pivotal RCTs submitted for registration of a new cardiovascular therapeutic. A report from the National Academies of Science on the prevention and treatment of missing data in clinical trials offers a series of recommendations that cover trial design, dropouts during the course of a trial, and sensitivity analyses that should take place at the data analysis phase. Of course, the most important recommendation is to make every effort to design trials in ways that minimize missing data.
MECHANISM MISSING DATA | ASSUMPTION | EXAMPLE | ASSESSMENT OF ASSUMPTION |
---|---|---|---|
MCAR | Missing data are not related to observed and unobserved outcomes or covariates. | A box of CDs containing data are damaged because of a water leak. | Examine the difference in mean covariate values for observed variables between subjects with no missing data and subjects with missing data. |
MAR | Missing data are not related to unobserved outcomes after adjustment for observed outcomes and observed covariates. | Older patients are more likely to have missing information on chest pain than younger patients. | Condition on as much data as possible. |
NMAR | None of the above apply. | Because of side effects, some patients do not return for measurement, and side effect data are unavailable. | Not possible to use data to demonstrate; need to review literature to determine whether key confounders are missing and not related to measured confounders. |
Measures of Treatment Effect
When the outcome is undesirable and the data are arranged as test group: control group comparison, a relative risk (RR) or odds ratio (OR) of less than 1 indicates benefit of the test treatment. The RR of 0.80 (95% CI, 0.72 to 0.88) and OR of 0.77 (95% CI, 0.69 to 0.87) in Figure 1-9 are indicative of benefit associated with treatment A. When the control rate is low, the OR will approximate the RR, and the OR may be thought of as an estimator of the RR. As the control rate increases, the OR deviates further from the RR, and clinicians should rely more on the latter. The treatment effect, expressed as an RR reduction in this example, is 20%, but its 95% CI ranges from 0.12 to 0.28. Such statements should be interpreted in the context of the absolute risk of the adverse outcome it is designed to prevent. The absolute risk difference (ARD) is even more meaningful if expressed as the number of patients who must be treated (= 1/ARD) to observe the beneficial effect, if it is as large as reported in the trial.
If practitioners are given clinical trial results only in the form of RR reduction, they tend to perceive a greater effectiveness of the test intervention than if a more comprehensive statement is provided, including ARD and the number needed to treat. Thus, in light of the baseline risk of 15% in the control group, a value that might represent the 1-month mortality rate of contemporary patients with MI not treated with fibrinolytic agents, the 12% event rate in the test group represents an ARD of 3%, which corresponds to 1/0.03, or approximately 33 patients who require treatment to prevent the occurrence of one adverse event. This statement is sometimes given as the number of lives saved per 1000 patients treated, or 30 lives in this example. Against this benefit must be weighed the risks associated with treatment (e.g., hemorrhagic stroke with fibrinolytic therapy for MI), which can be expressed as the number needed to harm (NNH = 1/ARI, where ARI is the absolute increase in events in the treatment group). A composite term referred to as net clinical benefit has been introduced to incorporate both benefit and harm. In this example, if compared with treatment B, treatment A is associated with a 0.5% excess risk of an adverse outcome, such as stroke, then for every 1000 patients who receive treatment A, 30 lives would be saved at the expense of five strokes, for a net clinical benefit of 25 stroke-free lives saved.
These types of comparisons require the clinical community to make a judgment regarding the relative importance of various outcomes. How many deaths have to be prevented to offset one stroke? Another example is the possibility that some therapies (inotropic agents) may improve symptoms but at the same time may increase mortality rates, a scenario that may be acceptable to patients incapacitated by severe symptoms but not to patients with mild symptoms. This issue can be addressed by decision analysis (see Cost-Effectiveness Analysis section).
The NNT is a complex concept that becomes even more difficult when the impact of therapies for chronic disease are considered. For acute therapies with only a short-term effect, such as thrombolytic therapy, the simple version of NNT is adequate. However, saving 10 lives per 100 patients treated in the first 30 days is quite different from the same effect over 5 years. In some therapies, the concept is even more complex because the more effective treatment may have an early hazard, leading to a reversal of the treatment effect over time.
When weighing the evidence from clinical trials for a treatment decision in an individual patient, physicians must consider more than the level of significance of the findings. In addition to the rationale for a given treatment, practitioners need to know which patients to treat, what drug and dose to use, and when and where therapy should be initiated. Not all clinical trial reports provide all the information required to form a complete assessment of the validity, precision, and implications of the results, nor do they answer the questions previously noted. In addition, clinicians are cautioned against overinterpreting subgroup analyses from RCTs because most RCTs lack sufficient power to assess adequately the treatment effects in multiple subgroups. Repeated statistical testing across several subgroups can lead to false-positive findings by chance; it is therefore preferable to present subgroup results in a visual format that depicts the point estimate and CIs to illustrate the range of possible treatment effects (Braunwald, Fig. 6-7). In an attempt to introduce consistency in the reporting of clinical trials in the biomedical literature, a checklist of information for trialists, journal editors, peer-review panels, and the general medical audience is available ( Table 1-4 ). The presentation of a minimal set of uniform information in clinical trial reports should assist clinicians in making treatment decisions.
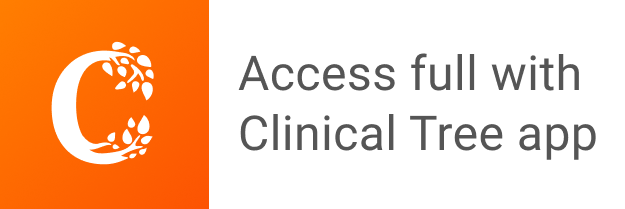