In observational studies of patients hospitalized for heart failure (HHF), risk of death is highest immediately after discharge and decreases over time. It is unclear whether this population risk trajectory reflects (1) lowering of individual patient mortality risk with increasing time from index hospitalization or (2) temporal changes in population case-mix with earlier postdischarge death for “sicker” patients. Survival rate and longitudinal models were used to estimate temporal changes in postdischarge all-cause mortality risk in 3,993 HHF patients discharged alive in the Efficacy of Vasopressin Antagonism in Heart Failure Outcome Study with Tolvaptan (EVEREST) trial. After median follow-up of 9.9 months, 971 patients died (24.2%). Predicted mortality rate decreased from 15.9 per 100 patient-years immediately after discharge to 13.4 at 30 days and 12.8 at 90 days; mortality rate increased steadily thereafter. Risk variation between quintiles of risk was considerably larger than the temporal variation within risk strata. In a longitudinal model serially reassessing predicted patient mortality risk after each follow-up visit using data collected at these visits, predicted mortality risk increased during the 90 days preceding subsequent heart failure readmission and then followed a postdischarge trajectory similar to the index admission. In conclusion, although there is transiently elevated individual patient risk in the 90 days before and after discharge, the patient’s individual risk profile, rather than temporal change in risk relative to hospitalization, remains the main determinant of mortality. For purposes of reducing all-cause mortality in HF patients, preventative and therapeutic measures may be best implemented as long-term interventions for high mortality risk patients based on serial risk assessments, irrespective of recent hospitalization.
Mortality risk for chronic heart failure (HF) patients who experience hospitalization for HF may be threefold higher than patients who were never hospitalized. This risk decreases over time, from sixfold within the first month after discharge to twofold subsequently. Although the exact mechanism of this postdischarge “vulnerable phase” remains unclear, these data suggest hospitalized heart failure (HHF) is inherently different from chronic ambulatory HF. However, it remains unclear whether these 2 entities, currently defined by the location where patients receive their care (i.e., inpatient vs outpatient), are biologically different. One hypothesis is that hospitalization events are uniquely followed by a time-dependent, transient, biologic vulnerable state for all patients, characterized by hemodynamic and neurohormonal abnormalities and end-organ damage. Alternatively, the observed decrease in postdischarge risk could be secondary to change in population case-mix toward an increasingly lower risk cohort over time. Although many analyses have documented a descending risk trajectory during the early postdischarge phase, all have been unable to decipher between these 2 mechanisms due to lack of longitudinal detailed data on evolving patient characteristics. Such outpatient data are absolutely necessary for the serial recalculation of estimated patient-level mortality risk over time. To gain novel perspective regarding the mechanism of the postdischarge “vulnerable phase,” we used the comprehensive longitudinal data set from the Efficacy of Vasopressin Antagonism in Heart Failure Outcome Study with Tolvaptan (EVEREST) trial to conduct a post hoc analysis of population and individual patient-level mortality risk over time after discharge. In the subset of patients with subsequent rehospitalization for HF, we also compared preadmission and postadmission predicted mortality risk to gain additional insights.
Methods
The design and the primary results of the EVEREST trial have been previously published. Briefly, EVEREST was a prospective, global, randomized, double-blind, placebo-controlled study. Patients were enrolled within 48 hours after admission at 359 sites from 2003 to 2006. Eligible patients were aged >18 years, hospitalized for worsening HF with an ejection fraction ≤40%, and New York Heart Association class III or IV symptoms. Patients were randomly assigned to receive either oral tolvaptan or placebo, in addition to standard therapy at the discretion of their physician. Informed consent was obtained from all patients. The trial was conducted in full accordance with the Declaration of Helsinki and with institutional review board and ethics committee approval at all sites.
Per trial protocol, outpatient vital signs, laboratory, and cardiovascular assessment data were collected at postdischarge clinic visits at weeks 1, 4, 8, and every 8 weeks thereafter. An independent events committee adjudicated the mode of death and cause of hospitalizations for all participants. Readmission was defined as a nonelective hospital admission that extended over a change in calendar date. Hospitalization for HF was defined as hospitalization that included worsening of HF symptoms and/or signs resulting in augmentation of medications including administration of intravenous therapies. The primary event of interest for the present study was all-cause mortality. Assessment of mortality risk, rather than rehospitalization risk, was chosen because it represents a “hard” biologic end point less subject to variation in HF practice patterns, reimbursement, psychosocial, and socioeconomic factors between hospitals and global regions. Thus, examination of mortality risk may provide the best information regarding existence of a “biologic,” time-sensitive postdischarge vulnerable phase.
This analysis was conducted in 2 stages. In stage 1, the goal was to use EVEREST covariate data to develop a risk model for mortality that could closely predict the observed mortality within the trial. To do this, we developed a prediction model for all-cause mortality risk using an exponential survival model incorporating both covariate data collected at discharge and at serial outpatient visits. Specifically, we modeled short-term predicted mortality risk between one visit and the next. Given our objective was not to construct a mortality prediction model for external validation in other cohorts, covariates for the survival model were chosen purely on their ability to optimize the model for predicting observed mortality within EVEREST (i.e., variables were not chosen based on established clinical significance in existing HF literature). Candidate variables are listed in Table S1 and variables and 2-way interactions were sequentially deleted until those that remained all met the threshold for statistical significance (p <0.05). Covariates retained in the final prediction model were the following: age (recomputed at each follow-up visit), body weight, systolic blood pressure, heart rate, ejection fraction, QRS duration on ECG, New York Heart Association functional class, history of HF hospitalization, presence of a permanent pacemaker, concurrent medication use (beta-blockers, peripheral vasodilators, lipid-lowering agents, nesiritide, and amiodarone), pedal edema, patient-reported fatigue, blood urea nitrogen, albumin, total cholesterol, creatinine, lactic dehydrogenase, chloride, magnesium, total bilirubin, B-type natriuretic peptide, and NT-pro-B-type natriuretic peptide. When discharge data were not available, the in-hospital value measured closest to discharge was substituted. For each postdischarge study visit, the value of the covariate observed at the visit was used when available; otherwise, the most recent nonmissing value was used.
For internal validation, the model was used to assign EVEREST patients into quintiles of estimated risk based on the average of all their risk scores at discharge through end of follow-up. Within each risk score quintile, we used the model to predict the specific mortality risk. The observed mortality in each quintile closely followed that predicted by the exponential model ( Figure 1 ), thus internally validating the exponential model as a reliable predictor of mortality within the EVEREST cohort. Thus, for discharge and each follow-up visit, the exponential model was used to calculate a risk score that is a direct estimate of the patient-level mortality risk, expressed as deaths per 100 person-years.

In stage 2, we used the prediction model with the time-dependent covariate data (when available) to serially recalculate the predicted risk of mortality from a given visit to the next among surviving patients. We modeled the changes in risk scores over time, both relative to discharge from the index hospitalization and relative to the first subsequent HF rehospitalization. For the postdischarge longitudinal analysis, we used the logarithm of the risk scores computed from the survival model from stage 1 in a flexible mixed-effects model with time from discharge as the independent variable. This model used cubic splines with boundary knots at discharge and day 931 (the longest postdischarge observation time) and internal knots at 100, 300, and 500 days. The general shape of the estimated mean was relatively insensitive to the selection of the knots. The model included random effects for the intercept and cubic spline terms with an unstructured covariance matrix.
We used the mortality prediction model to conduct a separate rehospitalization longitudinal analysis including patients with a subsequent HF rehospitalization using a time scale relative to the times of admission and discharge for the first rehospitalization. Risk scores observed before readmission were assigned negative times and those observed postdischarge were assigned positive times. Except at admission and discharge, observations made during hospitalization were ignored and times of readmission and discharge were both considered “time 0,” allowing for a common time scale independent of length of stay. Cubic splines were used to independently model predischarge and postdischarge observations with boundary knots at 0 and ±900 days and internal knots at ±160 days. All analyses were conducted using R, version 2.15.3 (Vienna, Austria).
Results
Patient characteristics at index discharge, stratified by quintiles of mortality risk estimates, are shown in Table 1 . Of the total 4,133 patients enrolled in EVEREST, 4,017 (97.2%) patients were discharged alive and followed for a median of 9.9 (interquartile range, 5.1 to 16.1) months. Overall, 3,993 (96.6%) patients had sufficient covariate information to be used in the present study.
All (N = 3993) | Q1 (N = 799) | Q2 (N = 798) | Q3 (N = 799) | Q4 (N = 798) | Q5 (N = 799) | |
---|---|---|---|---|---|---|
Age (yrs) † | 65.7 ±11.8 | 61.1 ±10.9 | 65.0 ±11.1 | 66.3 ±11.6 | 67.9 ±11.4 | 68.4 ±12.4 |
Men | 2981 (74.7%) | 597 (74.7%) | 559 (70.1%) | 599 (75.0%) | 612 (76.7%) | 614 (76.8%) |
Race | ||||||
White | 3412 (85.5%) | 710 (88.9%) | 688 (86.2%) | 680 (85.1%) | 673 (84.4%) | 661 (82.7%) |
Black | 298 (7.5%) | 49 (6.1%) | 60 (7.5%) | 57 (7.1%) | 56 (7.0%) | 76 (9.5%) |
Other | 282 (7.1%) | 40 (5.0%) | 50 (6.3%) | 62 (7.8%) | 68 (8.5%) | 62 (7.8%) |
Region | ||||||
North America | 1201 (30.1%) | 115 (14.4%) | 192 (24.1%) | 233 (29.2%) | 299 (37.5%) | 362 (45.3%) |
South America | 669 (16.8%) | 121 (15.1%) | 124 (15.5%) | 163 (20.4%) | 152 (19.0%) | 109 (13.6%) |
Western Europe | 536 (13.4%) | 90 (11.3%) | 83 (10.4%) | 114 (14.3%) | 118 (14.8%) | 131 (16.4%) |
Eastern Europe | 1587 (39.7%) | 473 (59.2%) | 399 (50.0%) | 289 (36.2%) | 229 (28.7%) | 197 (24.7%) |
Body weight (kg) † | 79.8 ±18.4 | 85.7 ±18.2 | 81.8 ±18.4 | 79.8 ±18.8 | 77.0 ±17.6 | 74.6 ±16.9 |
Systolic blood pressure (mm Hg) † | 115.6 ±16.8 | 120.9 ±15.3 | 119.3 ±16.1 | 115.2 ±16.7 | 113.2 ±16.7 | 109.4 ±16.9 |
Heart rate (bpm) † | 74.2 ±12.2 | 71.4 ±10.9 | 72.9 ±11.5 | 75.2 ±12.0 | 74.5 ±12.2 | 76.8 ±13.4 |
Ejection fraction (%) † | 27.6 ±8.1 | 31.2 ±7.0 | 29.1 ±7.4 | 27.2 ±8.1 | 25.8 ±8.0 | 24.5 ±8.1 |
QRS (ms) † | 126.7 ±35.1 | 109.4 ±29.0 | 122.7 ±33.0 | 128.1 ±34.0 | 134.8 ±35.9 | 138.7 ±35.7 |
Ischemic etiology | 2585 (65.6%) | 493 (62.4%) | 530 (67.5%) | 515 (65.1%) | 515 (65.4%) | 532 (67.4%) |
NYHA Class † | ||||||
I | 178 (4.5%) | 57 (7.1%) | 37 (4.6%) | 43 (5.4%) | 28 (3.5%) | 13 (1.6%) |
II | 1736 (43.5%) | 475 (59.4%) | 414 (51.9%) | 358 (44.9%) | 288 (36.1%) | 201 (25.2%) |
III | 1892 (47.4%) | 261 (32.7%) | 329 (41.2%) | 383 (48.0%) | 444 (55.6%) | 475 (59.4%) |
IV | 186 (4.7%) | 6 (0.8%) | 18 (2.3%) | 14 (1.8%) | 38 (4.8%) | 110 (13.8%) |
Blood urea nitrogen (mg/dL) † | 32.2 ±17.2 | 23.7 ±10.6 | 28.5 ±13.5 | 31.0 ±14.7 | 34.6 ±15.5 | 43.1 ±22.7 |
Sodium (mEq/L) | 139.9 ±5.0 | 140.8 ±4.2 | 140.7 ±4.8 | 140.2 ±4.9 | 139.8 ±5.2 | 138.2 ±5.6 |
Albumin (g/dL) † | 3.8 ±0.5 | 4.2 ±0.4 | 4.0 ±0.5 | 3.8 ±0.5 | 3.7 ±0.5 | 3.4 ±0.6 |
Total cholesterol (mg/dL) † | 172.2 ±49.5 | 196.5 ±49.4 | 187.1 ±46.1 | 173.8 ±46.9 | 160.9 ±44.8 | 142.6 ±41.0 |
Creatinine (mg/dL) † | 1.4 ±0.5 | 1.2 ±0.4 | 1.3 ±0.4 | 1.4 ±0.5 | 1.5 ±0.5 | 1.7 ±0.6 |
Lactic dehydrogenase (U/L) † | 215.1 ±76.0 | 183.6 ±49.1 | 201.3 ±51.8 | 212.7 ±60.3 | 224.8 ±59.5 | 252.9 ±117.9 |
Chloride (mEq/L) † | 100.6 ±5.3 | 101.8 ±4.2 | 101.6 ±4.8 | 100.8 ±5.1 | 100.4 ±5.5 | 98.6 ±6.1 |
Magnesium (mg/dL) † | 2.2 ±0.3 | 2.1 ±0.2 | 2.1 ±0.3 | 2.2 ±0.3 | 2.2 ±0.3 | 2.2 ±0.5 |
Total bilirubin (mg/dL) † | 0.8 ±0.7 | 0.6 ±0.4 | 0.7 ±0.5 | 0.8 ±0.5 | 1.0 ±0.8 | 1.1 ±0.9 |
BNP (pg/mL) † | 455 (192, 1001) | 143 (63, 279) | 305 (163, 543) | 440 (233, 814) | 746 (422, 1367) | 1179 (679, 1984) |
NT-proBNP (pg/mL) † | 2941 (1244, 6292) | 835 (389, 1730) | 1724 (936, 3318) | 2531 (1381, 4693) | 4262 (2517, 8019) | 7670 (4234, 13,748) |
Dyspnea (frequent/continuous) | 658 (16.5%) | 78 (9.8%) | 111 (13.9%) | 107 (13.4%) | 154 (19.3%) | 208 (26.0%) |
Orthopnea (frequent/continuous) | 274 (6.9%) | 28 (3.5%) | 39 (4.9%) | 49 (6.1%) | 68 (8.5%) | 90 (11.3%) |
Pedal Edema (moderate/marked) † | 248 (6.2%) | 7 (0.9%) | 20 (2.5%) | 28 (3.5%) | 56 (7.0%) | 137 (17.1%) |
Jugular venous distention ≥10 cm | 165 (4.1%) | 17 (2.1%) | 13 (1.6%) | 17 (2.1%) | 39 (4.9%) | 79 (10.0%) |
Fatigue (frequent/continuous) † | 1075 (27.0%) | 162 (20.4%) | 173 (21.7%) | 173 (21.7%) | 233 (29.2%) | 334 (41.8%) |
Medical history | ||||||
Hypertension | 2839 (71.1%) | 593 (74.2%) | 609 (76.3%) | 566 (70.8%) | 549 (68.8%) | 522 (65.3%) |
Heart failure hospitalization † | 3139 (78.9%) | 523 (65.5%) | 609 (76.4%) | 636 (80.2%) | 662 (83.7%) | 709 (88.7%) |
Diabetes mellitus | 1546 (38.7%) | 248 (31.0%) | 307 (38.5%) | 288 (36.0%) | 350 (43.9%) | 353 (44.2%) |
Chronic renal insufficiency | 1053 (26.4%) | 53 (6.6%) | 119 (14.9%) | 186 (23.3%) | 301 (37.7%) | 394 (49.4%) |
Coronary artery bypass surgery | 833 (20.9%) | 101 (12.6%) | 133 (16.7%) | 170 (21.3%) | 202 (25.3%) | 227 (28.4%) |
Myocardial infarction | 2012 (50.4%) | 365 (45.7%) | 394 (49.4%) | 396 (49.7%) | 427 (53.5%) | 430 (53.8%) |
Pacemaker † | 678 (17.0%) | 50 (6.3%) | 101 (12.7%) | 130 (16.3%) | 182 (22.8%) | 215 (26.9%) |
Concomitant therapy | ||||||
ACE inhibitor/ARB | 3394 (85.0%) | 742 (92.9%) | 721 (90.4%) | 696 (87.1%) | 661 (82.8%) | 574 (71.8%) |
Beta-blocker † | 3001 (75.2%) | 707 (88.5%) | 649 (81.3%) | 591 (74.0%) | 566 (70.9%) | 488 (61.1%) |
Peripheral vasodilator † ‡ | 71 (1.8%) | 5 (0.6%) | 16 (2.0%) | 14 (1.8%) | 15 (1.9%) | 21 (2.6%) |
Lipid-lowering agents † | 2454 (61.5%) | 420 (52.6%) | 489 (61.3%) | 498 (62.3%) | 506 (63.4%) | 541 (67.7%) |
Digoxin | 1896 (47.5%) | 300 (37.5%) | 355 (44.5%) | 423 (52.9%) | 415 (52.0%) | 403 (50.4%) |
Nesiritide † | 84 (2.1%) | 9 (1.1%) | 17 (2.1%) | 18 (2.3%) | 24 (3.0%) | 16 (2.0%) |
Amiodarone † | 698 (17.5%) | 85 (10.6%) | 120 (15.0%) | 122 (15.3%) | 178 (22.3%) | 193 (24.2%) |
Spironolactone | 2337 (58.5%) | 492 (61.6%) | 511 (64.0%) | 452 (56.6%) | 440 (55.1%) | 442 (55.3%) |
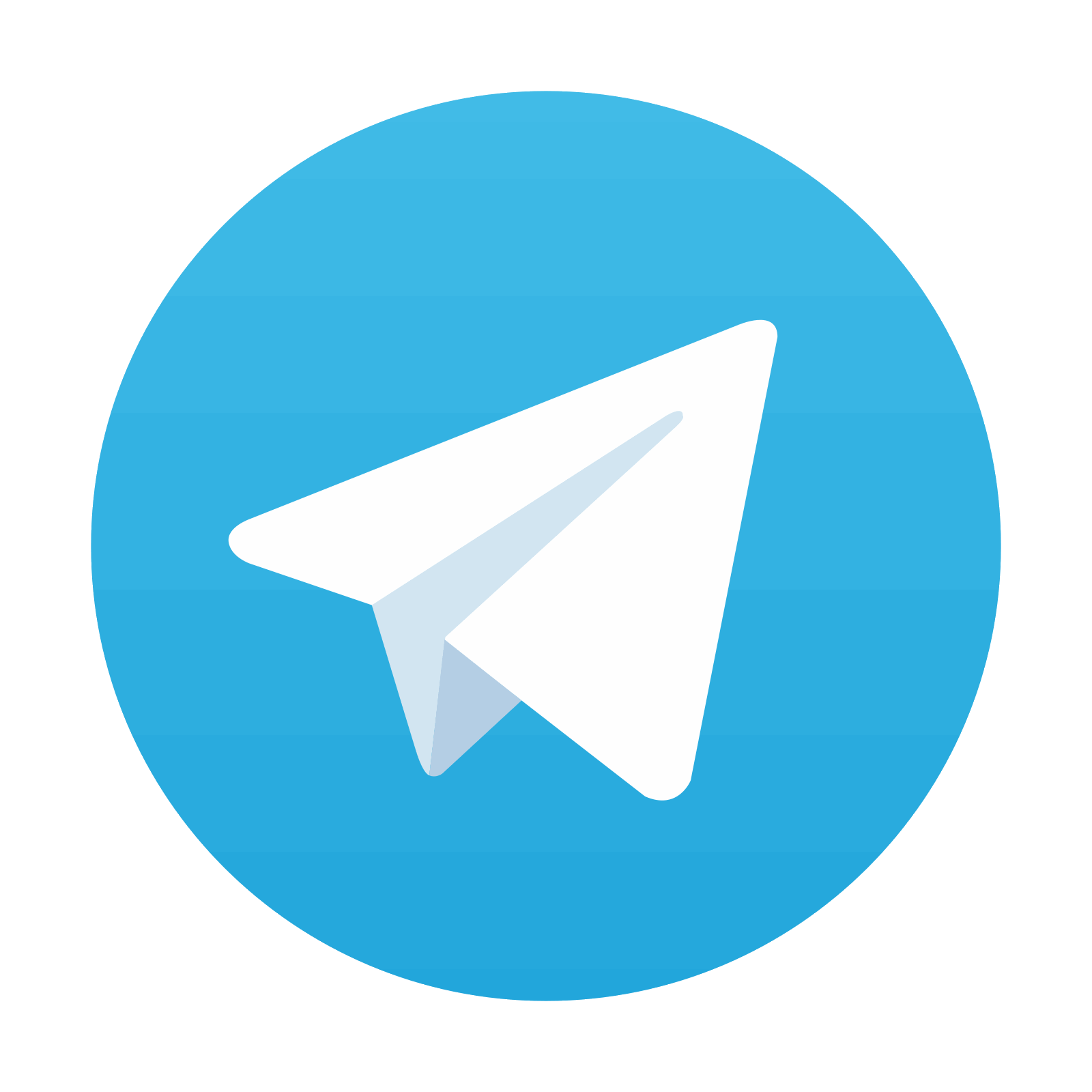
Stay updated, free articles. Join our Telegram channel

Full access? Get Clinical Tree
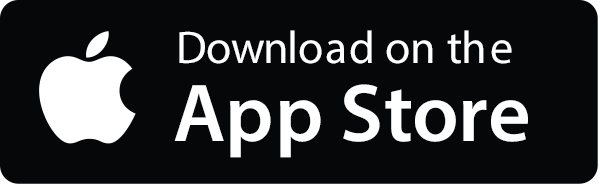

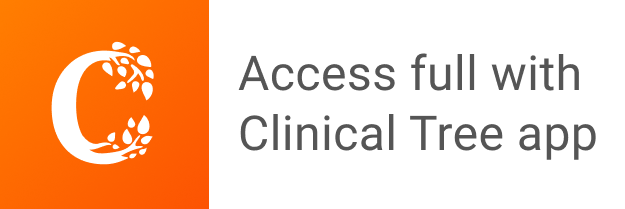