A distinct seasonal pattern in total and cardiovascular death rates has been reported. The factors contributing to this pattern have not been fully explored. Seven locations (average total population 71,354,000) were selected where data were available including relatively warm, cold, and moderate temperatures. Over the period 2004 to 2009, there were 2,526,123 all-cause deaths, 838,264 circulatory deaths, 255,273 coronary heart disease deaths, and 135,801 ST-elevation myocardial infarction (STEMI) deaths. We used time series and multivariate regression modeling to explore the association between death rates and climatic factors (temperature, dew point, precipitation, barometric pressure), influenza levels, air pollution levels, hours of daylight, and day of week. Average seasonal patterns for all-cause and cardiovascular deaths were very similar across the 7 locations despite differences in climate. After adjusting for multiple covariates and potential confounders, there was a 0.49% increase in all-cause death rate for every 1°C decrease. In general, all-cause, circulatory, coronary heart disease and STEMI death rates increased linearly with decreasing temperatures. The temperature effect varied by location, including temperature’s linear slope, cubic fit, positional shift on the temperature axis, and the presence of circulatory death increases in locally hot temperatures. The variable effect of temperature by location suggests that people acclimatize to local temperature cycles. All-cause and circulatory death rates also demonstrated sizable associations with influenza levels, dew point temperature, and barometric pressure. A greater understanding of how climate, temperature, and barometric pressure influence cardiovascular responses would enhance our understanding of circulatory and STEMI deaths.
Cardiovascular deaths and deaths from all causes (ALL) occur with seasonal variation with higher rates in winter. Seasonal variation of cardiovascular events occurs in cold Minnesota, moderate Los Angeles (LA), tropical Hawaii, and during winter in Australia from June to August. When studied individually, many factors are significantly associated with higher death rates, including low temperature, low moisture content, influenza, fewer hours of daylight, snow, precipitation, and others. However, many of these factors are interrelated, and less is known about their relative importance. In particular, the relative impact of cold temperatures versus respiratory infection is unclear. In contrast to cold, heat waves have also been associated with increased mortality. Temperature’s effect is often assumed to be universal; little is known about the influence of local climate on temperature’s effects. Other climactic factors are less well studied. To define the relative importance of these factors and to comprehensively describe seasonal variation of cardiac deaths, we use time series and regression modeling to explore the associations of death rate estimates with climatic factors (temperature, dew point, precipitation, and barometric pressure), influenza levels, and hours of daylight in 7 distinct locations across the United States. Air pollution levels and day of week were considered as potential confounders.
Methods
Geographic locations were selected to represent different climates, including the relatively cold northeast (Massachusetts and Pennsylvania), the relatively hot south (Georgia, Texas, Phoenix), and the relatively moderate west coast (LA County and the western portion of Washington). Data availability was required for location selection. Table 1 indicates the geographic source of all data.
Death certificate and air pollution data | Influenza-like illness data | Weather data ∗ |
---|---|---|
Massachusetts | Massachusetts | Boston |
Pennsylvania | Pennsylvania | Philadelphia |
Georgia | Georgia | Atlanta |
Texas | Texas | Texas average † |
Maricopa County | Arizona | Phoenix |
Los Angeles County | Los Angeles County | Los Angeles |
Western half of Washington ‡ | Washington | Seattle |
∗ Includes hours-of-daylight; climate data was obtained from the largest city within the geographic area which best estimates climate data for a large portion of the defined population and closely estimates the larger geographic area.
† Mean of Dallas, Houston, Austin, and San Antonio. Sixteen million of the 24 million people in Texas live in these 4 metropolitan areas and many of the rest live in close proximity.
‡ The 19 western-most counties in Washington state, including Clallam, Clark, Cowlitz, Grays Harbor, Island, Jefferson, King, Kitsap, Lewis, Mason, Pacific, Pierce, San Juan, Skagit, Skamania, Snohomish, Thurston, Wahkiakum, and Whatcom.
Daily death certificate data were obtained from the respective state health departments. Separate analyses were performed for ALL deaths and for 3 types of cardiac deaths: circulatory deaths (CIRC; International Classification of Diseases, Tenth revision [ICD-10] codes I00 to I99), coronary heart disease deaths (CHD; ICD-10 codes I20 to I25, but not I21), and ST-elevation myocardial infarction deaths (STEMI; ICD-10 codes I21). CHD deaths included non-STEMI, ischemic cardiomyopathy, and chronic ischemic heart disease. CIRC deaths (32.8% of ALL) included CHD (10.6%), STEMI (4.8%), heart failure, rheumatic heart disease, pulmonary hypertension, cerebrovascular, cardiac arrest, arrhythmia, and valvular heart disease. Data were analyzed from January 1, 2005, through December 31, 2009 (death certificate data were not available for 2009 for Massachusetts, LA or Georgia; the Georgia data also included 2004). The US Census Bureau ( http://www.census.gov/popest/data/intercensal/county/county2010.html ) provides annual (midyear) population estimates, which were used to convert number of deaths into death rates per 100,000 population using linear interpolation between annual population estimates. (These data were provided by the Bureau of Health Statistics and Research, Pennsylvania Department of Health. The Department specifically disclaims responsibility for any analysis, interpretations, or conclusions.)
We obtained weekly influenza-like illness (ILI) data from the Centers for Disease Control and Prevention’s Influenza-like Illness Surveillance Network (ILINet) ( http://gis.cdc.gov/grasp/fluview/fluportaldashboard.html ). ILI is defined as fever (temperature ≥37.8°C) and a cough and/or a sore throat in the absence of a known cause other than influenza. ILI data are collected by >3,000 providers throughout the United States and represent weekly estimates of respiratory illness (number of visits for ILI/number of total visits). The weekly ILI data were interpolated linearly to match daily data.
Data on hours of daylight were obtained from the Astronomical Applications Department of the United States Naval Observatory ( http://aa.usno.navy.mil/data/docs/RS_OneYear.php ). Weather data were obtained from Weather Underground, Inc. ( http://www.wunderground.com/ ). Weather data were used from the largest city within the region ( Table 1 ). To explore the effect of locally hot temperatures, we defined an indicator variable for the highest percentile of temperatures (labeled as locally hot) and included it in regression models.
Daily air pollution data, including carbon monoxide, ozone, particulate matter (10 and 2.5), and air quality index, were obtained from the US Environmental Protection Agency ( http://www.epa.gov/airquality/airdata/ad_data_daily.html ).
This investigation focused on seasonal variation and continuous factors that change over time. To maintain that focus, we did not analyze factors that vary little over time, including age, gender, and race; prevalence of diabetes and smoking; different health care systems; and the primary percutaneous coronary intervention network; and so forth. Moreover, these factors vary amongst individuals and small communities as opposed to the variables studied, which expose entire populations.
All statistical analyses were performed in SAS 9.3.
Death rate estimates and covariates may be considered as time series and viewed in terms of autoregressive integrated moving average models. To determine the relation of death rate to lags in covariate series, we found parsimonious cross correlations between the differenced death rate series (delta death rate) and an autoregressive model of each covariate. For example, the temperature series showed significant cross correlations at 0 and 1 with the delta death rate series. Cross correlations at 0 and 1 with the delta death rate series translate to lags 1 and 2 in the original (not differenced) death rate series. Subsequently, in regressions of death rates (see in the following), we were slightly more inclusive and considered series lags of 0, 1, 2, and 3 days for temperature. A similar time series analysis indicated the following lags to be adequate: dew point temperature, 3 days; barometric pressure, 3 days; air quality index, 2 days; all others, 0 days. Lags of 0, 7, 14, and 21 days were considered for ILI ( Supplementary Table 1 ).
All the potential covariates and interactions were considered in a variety of preliminary regression models using general linear models (GLM). Temperature consistently had the strongest association with ALL death rates. Therefore, we explored further the relation between ALL deaths and temperature.
The relations between ALL death rates and temperature across 7 locations and 5 years were examined using linear and nonlinear regression models treating 7 locations and 6 years as categorical variables. The best ALL, CIRC, and CHD models were cubic models with linear and quadratic functions of temperature interacting with location and with an additive term for year. Linear models had a comparable goodness-of-fit ( R 2 ) and were much simpler.
Considering the analyses of lags, interactions, and cubic terms, a simplified benchmark model was chosen:
death rate = year + location + temperature + location ∗ temperature
To determine the importance of other covariates, each covariate and its potential lags (mentioned previously) together with their interactions with location were added to the benchmark temperature model. The parsimonious (best) model was selected in a backward stepwise fashion ( Supplementary Table 2 ). Because the large sample size yielded significant p values for small effects, we considered the size of the F statistic and relative change in R 2 in addition to the p value in determining the best-fit model. An analysis of each covariate is called the univariate analysis herein.
Significant covariates (and lags and interactions) from univariate analyses were then considered in multivariate models. A similar backward selection process was used to obtain the parsimonious multivariate models. The final parsimonious models include only those covariates with sizable, independent effects as covariates with smaller effects are eliminated in the backward selection process.
Effect sizes were computed as standardized β values. For interaction effect sizes, we represent categorical variables (location, year) as a list of binary contrasts. For reporting purposes, interactive effect sizes are given as the largest standardized β in absolute value.
We considered day, week, month, and season in time-based seasonal models of death rates using location as an interaction term. Month was selected for the parsimonious time-based models ( R 2 ‘s of 0.809, 0.667, 0.632, 0.545 for ALL, CIRC, CHD, STEMI, respectively), represented as:
death rate = month ( a ) + location ( b ) + month ∗ location ( c )
The parsimonious explanatory models using other measurable factors (described in the following) are reported in Tables 2 and 3 . For example, the parsimonious multivariate model for ALL death rates is represented as:
All death rate = year ( a ) + location ( b ) + temperature ( c ) + temperature ∗ location ( d ) + temperature lag 3 ( e ) + ILI lag 14 ( f ) + ILI lag 14 ∗ location ( g ) + ILI lag 14 ∗ year ( h ) + dew point temperature lag 2 ( i ) + barometric pressure ( j )
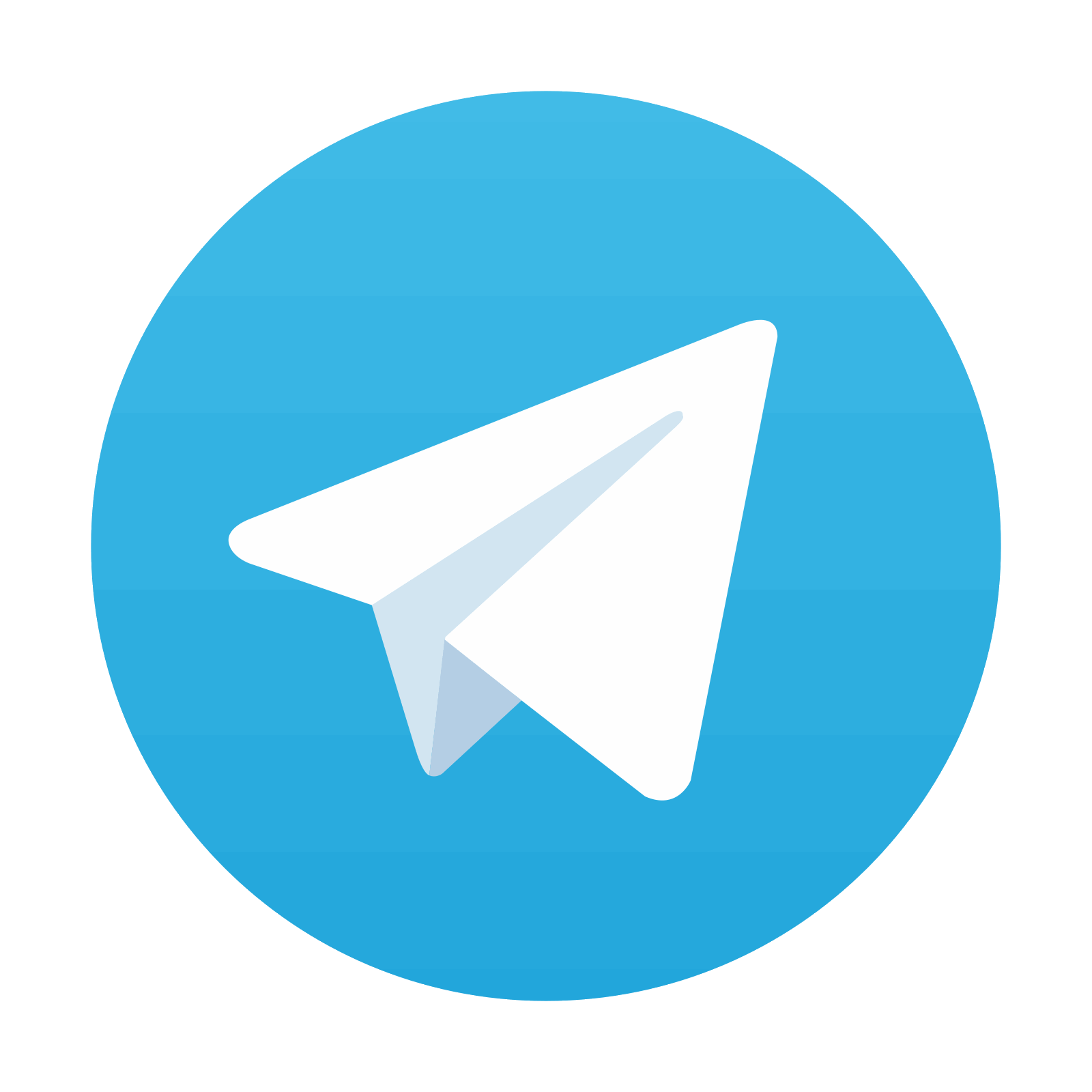
Stay updated, free articles. Join our Telegram channel

Full access? Get Clinical Tree
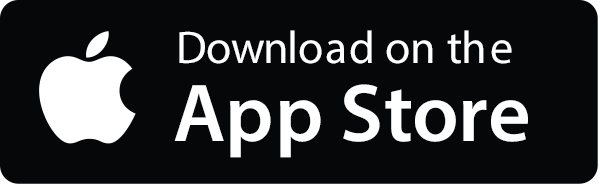
