Cardiovascular disease (CVD) remains the leading cause of mortality in the world, with more than 15 million deaths each year. With the current rise in obesity and other cardiovascular risk factors in the general population, it is anticipated that we will see a further increase in the incidence of heart disease in the coming decades, leading to an increase in CVD-related mortality and morbidity and an increased burden on the health care system.
The pharmacologic treatment of acute coronary syndrome (ACS) and the secondary prevention of coronary artery disease (CAD) have grown more complex as the result of many clinical trials demonstrating reductions in major cardiovascular events with the use of multiple classes of medications, including antiplatelet agents (e.g., aspirin, clopidogrel, glycoprotein IIb/IIIa [GPIIb/IIIa] inhibitors), fibrinolytics (e.g., streptokinase, alteplase), anticoagulants (e.g., heparin, low-molecular-weight heparins, fondaparinux), as well as statins and neurohormonal inhibitors (e.g., beta blockers and angiotensin-converting enzyme inhibitors [ACEIs]). In addition, many patients have several risk factors (including dyslipidemia, hypertension, and diabetes) that are treated with a number of medications, raising safety issues related to drug interactions and adverse drug reactions (ADRs). However, although the efficacy and safety of these drugs in specific populations have been demonstrated, there are currently only limited data available for the health professionals to identify specific patients who benefit from these drugs (responders), those who require alternative dosing strategies, or those who are at greater risk of experiencing ADRs.
ADRs represent a leading cause of mortality, morbidity, and cost. , Mounting evidence has demonstrated that bleeding secondary to the treatment of ACS with antiplatelet agents, anticoagulants, or fibrinolytics adversely affects the prognosis of patients. Hence, the identification of predictors of this ADR has become an important focus of research. Moreover, there is no known marker that can reliably identify patients most likely to experience rare, yet devastating, idiosyncrasic ADRs from many of the drugs used in the treatment and secondary prevention of ACS (e.g., heparin-induced thrombocytopenia, ACEI-induced angioedema, statin-induced myotoxicity). Thus, although significant progress has been made in the treatment of CVD, clinicians and drug policy makers recognize the importance of developing effective strategies to address drug efficacy problems and reduce ADRs resulting from CVD treatment.
Age, gender, ethnicity, weight, and renal and hepatic function are known modulators of drug pharmacokinetics—what the body does to the drug (the relationship between medication dose and plasma concentration) and pharmacodynamics—what the drug does to the body (the relationship between plasma concentration and medication effect). Nonetheless, they explain only a limited fraction of intersubject variability of drug response. Genetic factors may also contribute to this intersubject variability. Some have estimated that 20% to 95% of this variability may be attributable to genetic factors. Given the extensive data supporting the impact of genetic variations on the metabolism of many drugs used in the treatment and secondary prevention of ACS and the heritability of many drug targets, genetic factors may significantly contribute to the intersubject variability observed in the response to ACS pharmacotherapy. The field of pharmacogenomics aims to discover these genetic determinants to identify individuals who are most likely to respond, experience an ADR, or benefit from an alternative dosing regimen for a given drug. Understanding the genes that influence cardiovascular drug efficacy and toxicity may eventually provide guidance to health professionals for the optimization of pharmacotherapy for ACS and other CVDs.
Unfortunately, pharmacogenomic data are limited in ACS. Moreover, given the fact that no genetic test is currently used in clinical practice for the treatment of CVD, the objective of this chapter will be to illustrate the complexity of genomics and pharmacogenomics and how they can potentially influence the treatment of ACS and the secondary prevention of CAD. Following is a glossary of terms used in this chapter.
Glossary
Adverse drug reaction An unwanted effect caused by the administration of a drug. The onset of the adverse reaction may be sudden or may develop over time.
Allele In association studies , the term allele is most commonly used when referring to markers. An allele would be one specific version of a marker inherited from a parent (e.g., A or T in the case of an A/T SNP). Allele is also used in a broader context when referring to a specific version of an entire gene.
Association testing and association-based approaches A genetic variant is genotyped in a population for which phenotypic information is available (such as disease occurrence or a range of different trait values). If a correlation is observed between genotype and phenotype, there is said to be an association between the genetic variant and the disease or trait.
Causal gene The specific gene responsible for the risk conferred by a genomic region identified by an association study.
Causal variant The specific DNA sequence that functionally gives rise to the increased risk conferred by a genomic region identified by an association study.
Genetic markers Genomic variants used as positional tools to associate specific DNA fragments to certain phenotype or disease.
Genome-wide linkage scan A linkage study performed with markers located across the entire genome. It is traditionally performed with approximately 300 markers of simple sequence length repeats (SSLPs) and, more recently, with approximately 5000 SNPs.
Genotyping A test designed to identify the genetic constitution of an individual—that is, the alleles present at one or more specific loci.
Haplotype A haplotype is a combination or pattern of alleles at multiple linked loci that are transmitted together.
HapMap Genetic resource created by the International HapMap Project ( www.hapmap.org ). The HapMap is a catalogue of common human genetic variants that occur. It describes these variants, where they occur in our DNA, and how they are distributed among people within populations and among populations in different parts of the world.
Linkage disequilibrium (LD) A situation in which alleles on the same chromosome occur together more often than can be accounted for by chance. This indicates that the alleles are physically close on the DNA strand and are most likely to be transmitted together within a population.
Linkage study A study aimed at establishing linkage between a genetic marker and a disease locus. Linkage is based on the tendency for genes and genetic markers to be inherited together because of their location near one another on the same chromosome.
Locus The specific position of a gene or a marker on a chromosome.
Penetrance The likelihood, under given environmental conditions, with which a specific phenotype is expressed by those individuals with a specific genotype. For example, if half (50%) of those with the gene “X” that is known to cause the disease actually have the disease, then the penetrance of the gene is 0.5.
Pharmacodynamics Refers to the study of effects produced by drugs through interactions with their targets (receptors, enzymes, others), their mechanism of action, and the relationship between drug concentrations and effects (what the drug does to the body).
Pharmacokinetics Refers to the study of the time course of a drug and its concentration during its absorption, distribution, metabolism, and excretion in the body (what the body does to a drug).
Poor metabolizer A person who metabolizes a drug at a slower rate than others. A person can be a poor metabolizer for one drug and an extensive metabolizer of another, depending on the enzymes implicated in the metabolism of the drugs and the genotypes of the patient for these enzymes.
Replication study A study designed to test (or replicate) a prior, and explicit, genetic hypothesis.
Single nucleotide polymorphism (SNP) A genomic variant in which a single base in the DNA differs from the usual base at that position.
Tag SNP A representative SNP in a region of the genome with high linkage disequilibrium. This allows genetic variation to be identified without genotyping every single SNP. Tag SNPs are significant in genome-wide association studies in which millions of SNPs across the genome are studied. Tag SNPs allow the number of SNPs to genotype to be reduced while generating the same amount of information. The HapMap Project has catalogued tag SNPs in the entire human genome.
Structure of the Human Genome
The human genome consists of 3.3 billion base pairs of DNA. The recent completion of the human genome sequence provides evidence for 20,000 to 30,000 genes encoded by the genome of every individual nucleated cell of the human body. The genomic code, consisting of four different nucleotides (most often referred to by their single-letter symbols—A, C, G, T), has long been established. More recent studies have demonstrated that there are numerous differences that can be identified when comparing the genome of any two individuals. These are known as genetic variants. Although there are many different forms of genetic variation (e.g., insertions and deletions, rearrangements), the most common form is that of the single nucleotide polymorphism (SNP; pronounced “snip”). There are approximately 10 million SNPs that are common in the human population (i.e., at a frequency of 5% or greater). Because of the recombination patterns in the genome, these SNPs are not all independent; some are in linkage disequilibrium (LD) and are often transmitted together. The recent mapping of this LD, available on the HapMap Project website, has allowed the use of LD between SNPs as a tool in genetic studies. By selecting tag SNPs as proxies for several other SNPs, it is possible to reduce significantly the number of SNPs to be genotyped in a study and thus decrease the costs.
Depending on the nature and location of a sequence difference, genetic variations such as SNP may be silent or may have an influence on the biologic pathway(s) in which the protein product encoded by the gene plays a role. For example, some genetic variations will lead to a difference in the amino acid structure of a protein and disturb its function, whereas others may modulate the levels at which the gene and its protein will be expressed. Furthermore, some of the genetic variations that we have observed with these types of functional consequences may influence normal phenotypic variation (e.g., height, hair color), whereas others may influence clinical phenotypes and thus be considered as risk factors. Certain genetic risk factors modulate an individual’s risk to develop disease; others modulate the ability to respond to a given medication or the propensity to develop specific side effects in response to therapy. It is these latter two issues that are considered within the context of pharmacogenomic studies.
Traditionally, genetic traits have been categorized as monogenic or polygenic. In a monogenic trait, the phenotype observed can be explained by variations in a single gene. Cystic fibrosis, sickle cell disease, and Huntington’s disease are example of monogenic disorders. Conversely, polygenic traits are expressed through the interactions of several genes and are also influenced by the environment; these are commonly named complex traits. Many complex disorders exist such as inflammatory bowel disease, rheumatoid arthritis, systemic lupus erythematosus (SLE), and type 1 diabetes (T1D). CVDs are among the most prevalent complex disorders. Height, skin color, and IQ are also in the complex trait category as are responses to medications, because several factors influence drug pharmacokinetics and pharmacodynamics. Thus, the field of pharmacogenomics tackles the challenge of identifying the drug genes using strategies similar to those used in the search for complex disorders genes.
Genetic Association Studies
Several recent scientific advances have dramatically changed our ability to examine genetic variation as it relates to human disease. The first key advance is the complete determination of the final sequence of the human genome. This advance has provided a direct means to connect a chromosomal region with its DNA sequence and gene content. The second key advance is the successful effort to define DNA sequence variation in the human genome. Specifically, a genome-wide SNP map has grown from an initial version in 1998, with 4000 SNPs, into a current map of approximately 10 million SNPs ( www.ncbi.nlm.nih.gov/SNP ). The third key advance is the definition of the long-range extent of LD among SNPs in the human genome and the identification of common recombination hot spots that punctuate the extent of LD into so-called haplotype blocks across the genome. , These advances have facilitated the search for complex trait genes.
Identifying the actual causal gene(s) and causal variant(s) for any given disorder is an important challenge. The most widely used strategy to locate the genes causing a complex trait is association testing because it is the most powerful approach for this purpose. Several genetic variations have been associated with cardiovascular phenotypes or risk factors ( Table 10-1 ). Genetic association studies seek evidence for a statistically significant association between the marker allele and a disease at the population level. Specifically, the association approach involves comparing the frequency of an allele at the marker locus between a sample of unrelated affected individuals and an appropriate, well-matched control sample that is representative of the allelic distribution in the general population. In the context of pharmacogenomics, a group of responder patients is compared with a group of nonresponder patients. Alternatively, the association of a SNP of interest and a continuous trait (e.g., blood pressure, cholesterol) can be investigated. Testing for association to disease is direct (testing the actual mutation) or indirect (testing a genetic variant that acts as a proxy for the mutation). It usually follows one of two common study designs, candidate gene testing or unbiased genome-wide association mapping ( Fig. 10-1 ).
Source (Year) | Gene | Chromosome | Phenotype |
---|---|---|---|
Willer et al (2008) | ABCA1 | 9q31 | CAD, lipid concentration |
WTCCC (2007) | ADAMTS7 | 15q25 | CAD |
Helgadottir et al (2004) | ALOX5AP | 13q12 | Myocardial infarction |
Diabetes Genetics Initiative; Saxena et al (2007) ; Willer et al (2008) | APOA5 | 11q23 | Triglyceride; CAD, lipid concentration |
Kathiresan et al (2008) | APOB | 2p24 | Lipid concentration (LDLs, high-density lipoproteins [HDLs], triglycerides) |
Fredriksson et al (2007) | APOE | 19q13 | CVD |
Willer et al (2008) | APOC1-APOC4-APOC2 cluster | 19q13 | CAD, lipid concentration |
Ozaki et al (2009) | BRAP | 12q24 | Myocardial infarction |
Scott et al (2007) ; Zeggini et al (2007) | CDKAL1 | 6p22 | Type 2 diabetes |
McPherson et al (2007) ; Scott et al (2007) ; WTCCC (2007) ; Zeggini et al (2007) | CDKN2A/B | 9p21 | CAD |
Kathiresan et al (2008) | CELSR2, PSRC1, SORT1 | 1p13 | Lipid concentration (LDL, HDL, triglycerides) |
Diabetes Genetics Initiative; Saxena et al (2007) | CETP | 6q13 | Lipid concentration (HDL, triglycerides) |
Vasan et al (2007) | CFTR | 7q31 | Echocardiographic dimensions (left ventricle); brachial artery endothelial function; treadmill exercise responses |
Pare et al (2007) | EDN1 | 6p24 | CAD, HDL |
Vasan et al (2007) | FAM5C | 1q31 | Echocardiographic dimensions (left ventricle); brachial artery endothelial function; treadmill exercise responses |
Kathiresan et al (2008) | GALNT2 | 1q42 | Lipid concentration (LDL, HDL, triglycerides) |
Diabetes Genetics Initiative; Saxena et al (2007) | GCKR | 2p23 | Triglycerides |
Willer et al (2008) | GCKR | 2p23 | CAD, lipid concentration |
Vasan et al (2007) | KCNB2 | 8q13 | Echocardiographic dimensions (left ventricle); brachial artery endothelial function; treadmill exercise responses |
Willer et al (2008) | LDLR | 19p13 | CAD, lipid concentration |
Diabetes Genetics Initiative; Saxena et al (2007) | LIPC | 15q22 | Triglycerides |
Kathiresan et al (2008) ; Willer et al (2008) | LPL | 8p21 | Lipid concentration (LDL, HDL, triglycerides), CAD |
Helgadottir et al (2006) | LTA4H | 12q23 | Myocardial infarction |
Erdmann et al (2009) | MRAS | 3q22.3 | CAD |
Samani et al (2007) | MIA3 | 1q41 | CAD |
Myocardial Infarction Genetics Consortium; Kathiresan et al (2009) | MRPS6-SLC5A3-KCNE2 | 21q22 | Myocardial infarction |
Samani et al (2007) | MTHFD1L | 6q25 | CAD |
Arking et al (2006) | NOS1AP | 1q23 | QT interval |
Dahlman et al (2007) | NPFFR2 | 4q13 | Leanness and increased lipolysis |
Vasan et al (2007) | OBFC1 | 10q24 | Echocardiographic dimensions (left ventricle); brachial artery endothelial function; treadmill exercise responses |
Cohen et al (2006) | PCSK9 | 1p32 | CAD, LDL concentration |
Myocardial Infarction Genetics Consortium (2009) | PHACTR1 | 6p24 | Myocardial infarction |
Samani et al (2007) ; Sandhu et al (2008) | PSRC1 | 1p13 | CAD, LDL cholesterol concentration |
Gudbjartsson et al (2009) | SH2B3 | 12q24 | Myocardial infarction |
Tregouet et al (2009) | SLC22A3-LPAL2-LPA | 6q26-q27 | Myocardial infarction |
Li et al (2007) | SLC2A9 | 4p16 | Serum uric acid level |
Vasan et al (2007) | SLIT2 | 4p15 | Echocardiographic dimensions (left ventricle); brachial artery endothelial function; treadmill exercise responses |
Samani et al (2007) | SMAD3 | 15q22 | CAD |
Kathiresan et al (2008) | TBL2 | 7q11 | Lipid concentration (LDL, HDL, triglycerides) |
Sandhu et al (2008) | TOMM40 | 19q13 | LDL cholesterol concentration |
Myocardial Infarction Genetics Consortium; Kathiresan et al (2009) | WDR12 | 2q33 | Myocardial infarction |
Vasan et al (2007) | WRN | 8p12 | Echocardiographic dimensions (left ventricle); brachial artery endothelial function; treadmill exercise responses |

Three main factors that directly influence the success of association studies are the size of the cohorts under study, matching of the different groups, and replication of the discovered association. Traditionally, collections of patients (or cases) and unrelated matched controls were tested for differences in allele frequencies. Initially, association-based genetic studies were generally limited to the testing of modestly sized cohorts of patients with a small number of genetic variants in one gene or in a small number of genes. Unfortunately, this led to a very large number of false-positive results. Today, given the focus on complex traits and the modest effects of individual genes, study design generally involves hundreds to thousands of samples; meta-analyses (i.e., combining the data of several independent studies) are becoming more popular and allow the identification of very modest effects of genes. One other major source of error in case-control studies is that of inadequate matching—that is, differences between the case and control populations that are unrelated to disease, also known as stratification. Careful selection of samples and corrections for stratification are now integrated into association study designs. In addition, replication of the association signals is essential to eliminate false-positive associations. Most studies are designed as two-stage experiments, with large cohorts for both stages. The first stage is for screening to detect associations and the second stage is for replication. More details concerning the limitations of association testing (i.e., statistical design, evaluation of the candidate gene, ethnic variability in disease susceptibility) as well as methods to detect and correct for stratification have been described in detail elsewhere. ,
Candidate Gene Approach
The candidate gene approach relies on selecting genes based on knowledge of their biologic function as likely to have an influence on disease susceptibility, and testing the known genetic variants in and around the gene for association to disease. The number of SNPs tested in such study, up to a few thousand, keep the cost of this strategy relatively low compared with the genome-wide alternative. For example, the role of apolipoprotein E in the metabolism of cholesterol and triglycerides made it a central candidate for CVD risk and its implication has been confirmed in several association studies. Unfortunately, of the approximately 20,000 genes that are believed to exist in the human genome, few have been annotated for specific function. Moreover, it is not that uncommon for functional annotation of genes to be incomplete. One recent example is that of the nicotinic acetylcholine receptor α7 subunit. The main function of the nicotinic acetylcholine receptor family was initially believed to be the transmission of the signals for the neurotransmitter acetylcholine at the neuromuscular junctions; more recently, it was shown to be an essential regulator of the inflammatory response. Many genes related to the pharmacokinetics and pharmacodynamics of drugs are the subject of candidate gene studies ( Table 10-2 ). However, candidate gene studies are often unsuccessful. This outcome is not that surprising, given the low likelihood that an investigator could select the few causal genes from the human genome, even armed with the best knowledge of disease pathogenesis. Inadequate sample size has also contributed to this lack of success.
Source (Year) | Gene | Chromosome | Gene description | Drug |
---|---|---|---|---|
Pharmacokinetics | ||||
Ismail and Teh (2006) ; Kirchheiner et al (2007) | CYP2D6 | 22q13 | Cytochrome P-450 2D6 | Metoprolol, carvedilol, flecainide, propafenone, codeine |
Sconce et al (2005) ; Limdi et al (2008) ; Schwarz et al (2008) | CYP2C9 | 10q24 | Cytochrome P-450 2C9 | Warfarin, irbesartan, losartan |
Collet et al (2009) ; Mega et al (2009) ; Sibbing et al (2009) | CYP2C19 | 10q24 | Cytochrome P-450 2C19 | Clopidogrel |
Zheng et al (2003) ; Wilke et al (2005) | CYP3A5 | 7q22.1 | Cytochrome P-450 3A5 | Atorvastatin, simvastatin, lovastatin, tacrolimus |
Drug Transporters | ||||
Pasanen et al (2006) ; Link et al (2008) | SLCO1B1 | 12p | OATP1B1 | Pravastatin, simvastatin, rosuvastatin |
Pharmacodynamics/Disease-Modifying Gene | ||||
McNamara et al (2004) ; Bhatnagar et al (2007) | ACE | 17q23 | Angiotensin-converting enzyme | RAAS inhibitors, blockers |
Terra et al (2005) ; Liggett et al (2006) | ADBR1 | 10q24 | β 1 -adrenergic receptor | Beta blockers |
Kaye et al (2003) ; Lanfear et al (2005) | ADBR2 | 5q31 | β 2 -adrenergic receptor | Beta blockers |
Kurland et al (2002) | AGT | 1q42 | Angiotensinogen | RAAS inhibitors, blockers |
Kurland et al (2002) | AGTR1 | 3q21 | Angiotensin II receptor, type 1 | RAAS inhibitors, blockers |
Mackenzie et al (2005) ; Li et al (2006) | ALDH2 | 12q24 | Aldehyde dehydrogenase | Nitroglycerin |
Gerdes et al (2000) ; Chiodini et al (2007) | APOE | 19q13 | Apolipoprotein E | Statins |
Medina et al (2008) | HMGCR | 5q13 | 3-hydroxy-3-methylglutaryl coenzyme A reductase | Statins |
Goodman et al (2007) | ITGB3 (GPIIIa) | 17q21 | Integrin β 3 | Aspirin |
Iakoubova et al (2008) ; Iakoubova et al (2008) | KIF6 | 6p21 | Kinesin-like protein 6 | Statins |
Lynch et al (2008) | NPPA | 1p36 | Atrial natriuretic peptide precursor | Antihypertensive agents |
Moore et al (2007) | REN | 1q32 | Renin | Aliskiren |
Sconce et al (2005) ; Schwarz et al (2008) | VKORC1 | 16p11 | Vitamin K epoxide reductase | Warfarin |
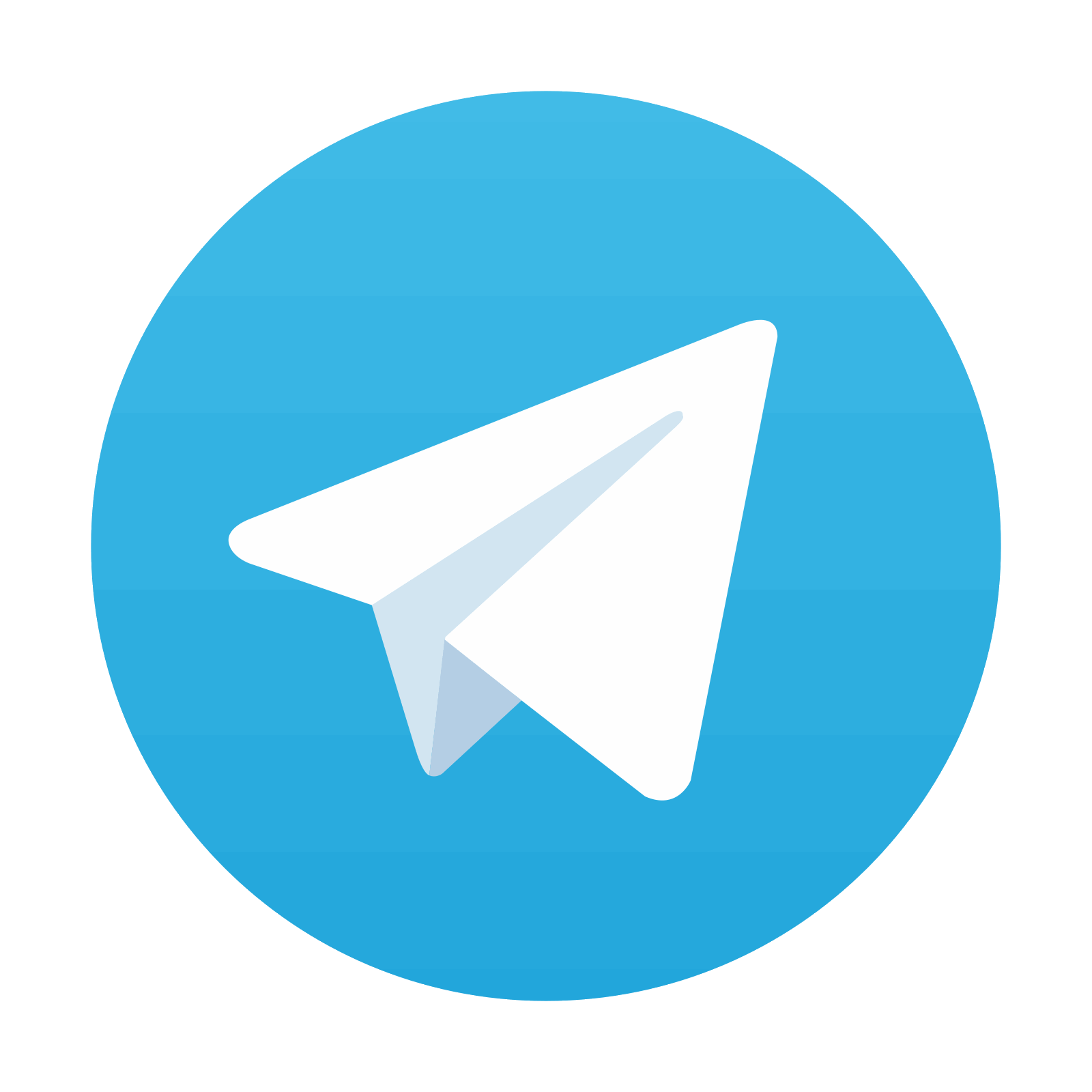
Stay updated, free articles. Join our Telegram channel

Full access? Get Clinical Tree
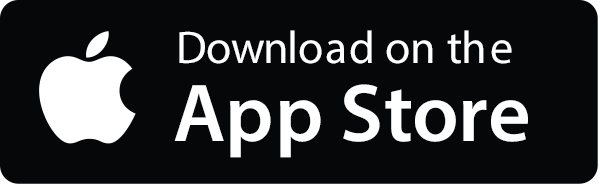
