Although fast food is affordable and convenient, it is also high in calories, saturated fat, and sodium. The frequency of fast food intake at the time of and after acute myocardial infarction (AMI) is modifiable. However, patterns of fast food intake and characteristics associated with its consumption in patients with AMI are unknown. The aim of this study was to study fast food consumption at the time of AMI and 6 months later in 2,481 patients from the prospective, 24-center Translational Research Investigating Underlying Disparities in Acute Myocardial Infarction Patients’ Health Status (TRIUMPH) study of patients with AMI. Fast food intake was categorized as frequent (weekly or more often) or infrequent (less than weekly). Multivariate log-binomial regression was used to identify patient characteristics associated with frequent fast food intake 6 months after AMI. At baseline, 884 patients (36%) reported frequent fast food intake, which decreased to 503 (20%) 6 months after discharge (p <0.001). Male gender, white race, lack of college education, current employment, and dyslipidemia were independently associated with frequent fast food intake 6 months after AMI. In contrast, older patients and those who underwent coronary bypass surgery were less likely to eat fast food frequently. Documentation of discharge dietary counseling was not associated with 6-month fast food intake. In conclusion, fast food consumption by patients with AMI decreased 6 months after the index hospitalization, but certain populations, including younger patients, men, those currently working, and less educated patients were more likely to consume fast food, at least weekly, during follow-up. Novel interventions that go beyond traditional dietary counseling may be needed to address continued fast food consumption after AMI in these patients.
We sought to describe patterns of fast food consumption at the time of acute myocardial infarction (AMI) and in follow-up in patients with AMI in the 24-center Translational Research Investigating Underlying Disparities in Acute Myocardial Infarction Patients’ Health Status (TRIUMPH) study. We ascertained the prevalence of frequent fast food intake at the time of admission with AMI and 6 months later. To identify populations of patients who frequently consume fast food after discharge, we then the examined patient characteristics associated with the postdischarge consumption of fast food more often than once a week. Finally, given the potential importance of discharge dietary counseling, which has been proposed as a potential quality measure in AMI care and adopted as an indicator of quality in heart failure care, we examined the association of dietary counseling during the index hospitalization with frequent fast food consumption 6 months later.
Methods
We studied patients enrolled from April 11, 2005, to December 31, 2008, in the TRIUMPH study, a prospective observational registry of patients with AMI from 24 centers across the United Status. Patients were eligible for inclusion if they were aged ≥18 years and had elevated cardiac biomarkers (troponin or creatine kinase-MB within 24 hours of admission), had supporting evidence of AMI (electrocardiographic changes or prolonged ischemic signs or symptoms), and presented to the enrolling institution or were transferred to that facility within 24 hours of presentation. Patients were excluded if they had elevated biomarkers after elective revascularization, refused participation, could not provide consent, or were currently incarcerated. Trained data collectors performed detailed baseline chart abstractions to document patients’ medical histories, processes of inpatient care, laboratory results, and treatments. Each patient also underwent a standardized interview by research staff members to document further demographic, socioeconomic, and clinical data. Patients were then contacted for 6-month follow-up interviews. All patients provided written informed consent, and the study protocol was approved by the institutional review board at each participating institution.
Of 4,340 patients who provided consent and were enrolled into TRIUMPH, we excluded 24 patients who died before discharge and 55 patients who did not respond to the fast food question on the baseline interview. We also excluded 1,780 patients missing 6-month fast food consumption data (1,093 were lost to follow-up before 6-month follow-up interviews, 180 refused follow-up interviews, 157 died, 93 were too ill to complete the interview, and 257 did not respond to the 6-month fast food assessment), leaving a final study cohort of 2,481 patients.
To assess the frequency of fast food consumption, patients were interviewed before discharge and 6 months later and asked, “How often do you eat at fast food restaurants?” Five responses were possible: “Less than once a month,” “1 to 3 times a month,” “1 to 2 times a week,” “3 to 4 times a week,” and “5 or more times a week.” The latter 2 categories were collapsed into a single fast food group, because both represent very heavy fast food intake. We further categorized fast food intake into frequent fast food consumption (weekly or greater) and infrequent consumption (less than weekly).
Although our fast food assessment has not been previously validated, a similar single-item question assessing fast food intake was shown to be associated with poor diet quality in the Multi-Ethnic Study of Atherosclerosis (MESA) cohort. In this study, patients who reported frequent fast food intake were more likely to have poor overall diet quality as assessed by detailed instruments, including a higher intake of fats and processed meats. We also compared the mean red blood cell omega-3 fatty acid levels by category of fast food consumption in a TRIUMPH substudy of 1,487 patients who had concurrent red blood cell omega-3 level assessment and dietary assessment data. Omega-3 levels reflect overall diet quality over a 3-month time span, similar to glycosylated hemoglobin as a time-averaged marker of blood glucose levels. The clinical logic was that fast food is low in omega-3 fatty acids and high in omega-6 fatty acids and that those with greater fast food consumption should have lower omega-3 levels. A red blood cell omega-3 level <4% has been shown to be associated with increased mortality and sudden cardiac death, while a level >8% is protective. We found that omega-3 levels <4% were present in 102 of 537 patients (19%) who consumed fast food monthly or less, in 120 of 423 patients (28%) eating fast food 1 to 3 times per month, in 85 of 295 (29%) patients eating fast food 1 to 2 times a week, and in 88 of 234 (38%) patients eating fast food ≥3 times per week (p <0.001). Conversely, favorable omega-3 levels of >8% were seen in 39 (7%), 25 (5.9%), 7 (2.4%), and 4 (1.7%) patients, respectively (p <0.001). These comparisons were highly statistically significant and support the validity of the primary outcome for this study.
An important goal of our study was to describe the relation between socioeconomic status and fast food eating patterns. To accomplish this, we collected information on patients’ levels of education, which was categorized as having or not having a college degree. Patients’ monthly financial status was assessed with the following question: “In general, how do your finances usually work out at the end of the month? Do you usually end up with: some money left over, just enough to make ends meet or not enough to make ends meet?” Patient-reported employment status was collected by asking “Do you currently work for pay?” with responses recorded as “Yes, full time,” “Yes, part time,” or “No.” A patient was considered currently employed for these analyses if he or she reported full- or part-time work at the time of enrollment. We assessed depressive symptoms using the Patient Health Questionnaire–-9, using a threshold score of ≥10 to identify depressed patients.
Baseline characteristics of patients with and without frequent fast food intake were compared using independent Student’s t tests for continuous variables and chi-square tests for categorical variables. Given that the outcome of frequent fast food intake was common, we used multivariable log-binomial regression to identify independent predictors, at the time of AMI, of frequent fast food intake at 6-month follow-up. Hierarchical analyses were conducted, with hospital site as a random effect, to account for clustering of patient characteristics within hospitals. We selected, a priori, the following variables for inclusion in the model: sociodemographic characteristics (age, gender, race [white vs other]), education (college vs no college degree, monthly financial difficulty, employment status), clinical factors (current smoking, obesity [body mass index ≥30 kg/m ], diabetes mellitus, hypertension, chronic heart failure, dyslipidemia, history of previous myocardial infarction, depression), characteristics of the index AMI (ST-segment elevation vs non–ST-segment elevation AMI, treatment with percutaneous coronary intervention or coronary artery bypass grafting), and the provision of dietary counseling at discharge. Among those with 6-month follow-up data, a total of 101 patients were missing data on the body mass index variable. A smaller number of observations were missing for several other variables (race in 6 patients, education in 4 patients, smoking status in 13 patients, working status in 15 patients, and financial status in 38 patients). To allow the models to run on the entire cohort with 6-month follow-up, we imputed these missing data using sequential regression imputation with IVEWARE software.
To assess for selection bias in our findings due to differences between those patients with and without 6-month follow-up data, we created a nonparsimonious model for the propensity of missing 6-month follow-up data. The reciprocal of this probability was used to weight the associations among responders in the regression model. Results with and without weighting were similar, so only the original analyses are reported.
Results
During the index AMI hospitalization, 884 patients (36%) reported eating fast food frequently. Very frequent fast food intake (≥3 times weekly) was reported by 391 patients (16%; Figure 1 ). The proportion frequently consuming fast food decreased by 6-month follow-up (weekly or greater, 503 patients [20%]; ≥3 times weekly, 139 patients [6%]). Although only 877 patients (35%) reported rarely eating fast food (less than monthly) at the time of AMI, 1,279 (52%) reported less than monthly fast food consumption at 6 months. There were several important differences in the baseline characteristics of patients reporting frequent and infrequent 6-month fast food intake ( Table 1 ). Among patients who did and those who did not eat fast food frequently, most patients had documented dietary counseling before discharge from the hospital.
Frequent Fast Food Intake (n = 503) | Infrequent Fast Food Intake (n = 1,978) | p Value | |
---|---|---|---|
Age (years) | 57.3 ± 11.4 | 60.5 ± 11.8 | <0.001 |
Men | 380 (75.5%) | 1,308 (66.1%) | <0.001 |
Caucasian | 384 (76.3%) | 1,442 (73.1%) | 0.143 |
Education (less than college) | 265 (52.7%) | 916 (46.4%) | 0.012 |
Health insurance payer | 0.270 | ||
Preferred provider organization | 230 (46.2%) | 851 (43.8%) | |
Health maintenance organization | 56 (11.2%) | 248 (12.8%) | |
Medicare/Medicaid/United States Department of Veterans Affairs | 105 (21.1%) | 473 (24.3%) | |
Self | 90 (18.1%) | 297 (15.3%) | |
Other | 17 (3.4%) | 75 (3.9%) | |
Avoids care because of cost | 118 (23.7%) | 446 (22.8%) | 0.652 |
Monthly finances | 0.266 | ||
Some money left over | 224 (45.3%) | 914 (46.9%) | |
Just enough to make ends meet | 172 (34.7%) | 705 (36.2%) | |
Not enough to make ends meet | 99 (20.0%) | 329 (16.9%) | |
Married | 285 (56.8%) | 1,134 (57.4%) | 0.794 |
Work full- or part-time | 290 (58.0%) | 927 (47.2%) | <0.001 |
Body mass index (>30 kg/m 2 ) | 204 (42.3%) | 772 (40.7%) | 0.511 |
Diabetes mellitus | 150 (29.8%) | 550 (27.8%) | 0.370 |
History of hypertension | 316 (62.8%) | 1,300 (65.7%) | 0.223 |
History of dyslipidemia | 262 (52.1%) | 992 (50.2%) | 0.438 |
Chronic kidney disease | 32 (6.4%) | 113 (5.7%) | 0.580 |
Current smoker | 210 (42.1%) | 690 (35.0%) | 0.004 |
Chronic heart failure | 29 (5.8%) | 142 (7.2%) | 0.264 |
Depression (Patient Health Questionnaire–9 score ≥10) | 96 (20.0%) | 321 (17.2%) | 0.154 |
Peripheral vascular disease | 24 (4.8%) | 100 (5.1%) | 0.794 |
Previous stroke | 20 (4.0%) | 92 (4.7%) | 0.515 |
History of myocardial infarction | 102 (20.3%) | 401 (20.3%) | 0.998 |
History of coronary artery bypass grafting | 61 (12.1%) | 236 (11.9%) | 0.904 |
Final myocardial infarction diagnosis | 0.985 | ||
ST-segment elevation myocardial infarction | 223 (44.3%) | 876 (44.3%) | |
Non–ST-segment elevation myocardial infarction | 280 (55.7%) | 1,102 (55.7%) | |
In-hospital revascularization | 0.096 | ||
None | 134 (26.6%) | 482 (24.4%) | |
Percutaneous coronary intervention | 330 (65.6%) | 1,281 (64.8%) | |
Coronary artery bypass grafting | 39 (7.8%) | 215 (10.9%) | |
Number of coronary arteries diseased | 1.7 ± 0.9 | 1.6 ± 0.9 | 0.526 |
Total cholesterol (mg/dl) | 156.8 ± 36.3 | 156.1 ± 38.0 | 0.767 |
High-density lipoprotein (mg/dl) | 38.8 ± 10.4 | 41.6 ± 13.0 | <0.001 |
Low-density lipoprotein (mg/dl) | 99.7 ± 35.0 | 97.9 ± 35.4 | 0.307 |
Triglycerides (mg/dl) | 167.4 ± 136.3 | 145.3 ± 100.5 | <0.001 |
Glycosylated hemoglobin (%) | 6.5 ± 1.7 | 6.6 ± 2.0 | 0.872 |
Glucose (mg/dl) | 160.2 ± 85.5 | 157.7 ± 83.1 | 0.548 |
Discharge diet counseling | 461 (91.7%) | 1,795 (90.7%) | 0.529 |
Referral for outpatient cardiac rehabilitation | 208 (41.4%) | 755 (38.2%) | 0.191 |
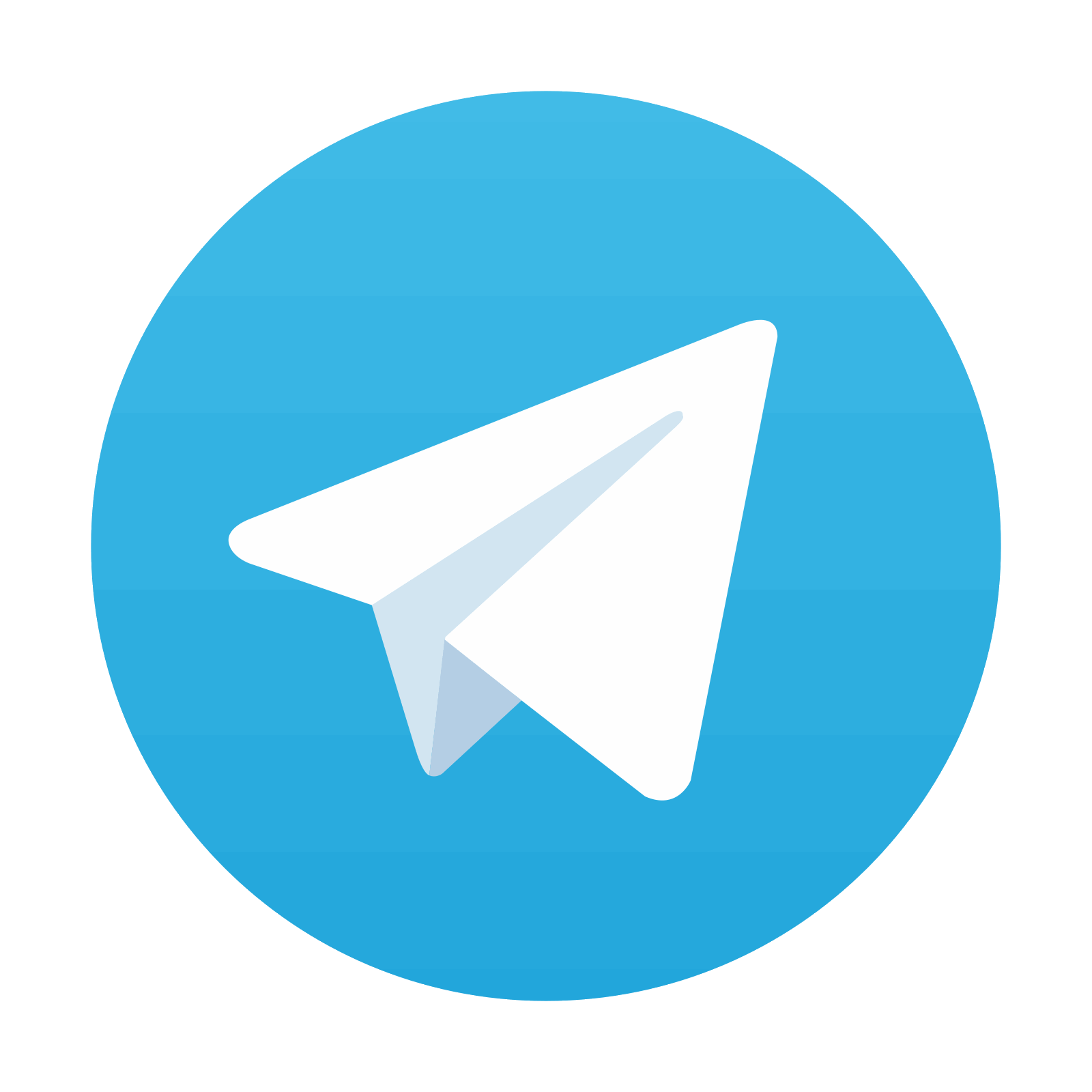
Stay updated, free articles. Join our Telegram channel

Full access? Get Clinical Tree
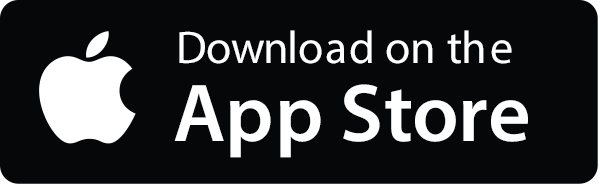

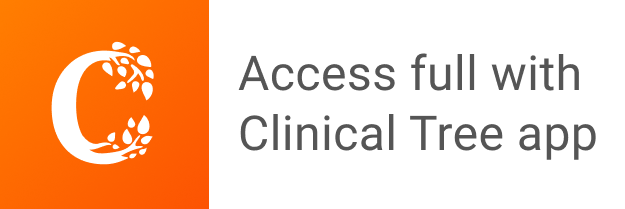