This prospective study assessed whether gender differences in health insurance help explain gender differences in delay in seeking care for patients in the US, with acute myocardial infarction (AMI). We also assessed gender differences in such prehospital delay for AMI in Spain, a country with universal insurance. We used data from 2,951 US and 496 Spanish patients aged 18 to 55 years with AMI. US patients were grouped by insurance status: adequately insured, underinsured, or uninsured. For each country, we assessed the association between gender and prehospital delay (symptom onset to hospital arrival). For the US cohort, we modeled the relation between insurance groups and delay of >12 hours. US women were less likely than men to be uninsured but more likely to be underinsured, and a larger proportion of women than men experienced delays of >12 hours (38% vs 29%). We found no association between insurance status and delays of >12 hours in men or women. Only 17.3% of Spanish patients had delays of >12 hours, and there were no significant gender differences. In conclusion, women were more likely than men to delay, although it was not explained by differences in insurance status. The lack of gender differences in prehospital delays in Spain suggests that these differences may vary by health care system and culture.
Prehospital delays in seeking medical care for acute myocardial infarction (AMI) are vastly longer among women compared with men. Such delays are harmful and increase the risk of mortality. However, the causes of the gender difference in delays are unknown. Inadequate health insurance is a known barrier to timely care seeking. Adults with lower income, those covered by Medicaid, and the uninsured all delay seeking care for emergencies compared with those with private insurance. This relation has been established in mostly elderly cohorts. However, unlike the elderly, who are nearly universally covered (i.e., Medicare), a large portion of younger adults are uninsured or underinsured, especially women. Although the Affordable Care Act aims to increase access, it is unknown if adequate insurance affects care seeking for AMI and whether similar levels of delay exist in countries with national insurance. Accordingly, we sought to assess whether gender differences in insurance contribute to gender differences in prehospital delay in young patients with AMI. We used data from Variation in Recovery: Role of Gender on Outcomes in Young AMI Patients (VIRGO), a prospective study of adults aged 18 to 55 years with AMI in the US and Spain. We aimed to (1) compare the extent of prehospital delay among young US women and men; (2) compare health insurance adequacy among young US women and men; and (3) assess whether gender differences in prehospital delay are mediated by gender disparities in insurance in the US. Because VIRGO included patients from Spain, a country with a national insurance system that does not require payment from the patient, we conducted secondary analyses on data from the Spanish cohort to examine gender differences in prehospital delay there.
Methods
We used data from patients, aged 18 to 55 years, in the US and Spain who were enrolled in VIRGO. US patients were enrolled from August 2008 to January 2012 from 108 hospitals at geographically diverse sites; Spanish patients were enrolled from April 2009 to December 2011 from 24 hospitals. Designed to focus on women, VIRGO enrolled consecutive women and every other man to achieve a 2:1 gender ratio (3,572 in total and 516 in Spain). Detailed eligibility and exclusion criteria for VIRGO were previously published. We further excluded those with unknown prehospital delay times (32 in the US and 19 in Spain) or insurance status (2 in the US and 1 in Spain) to create a final study cohort of 2,951 patients in the US and 496 in Spain. Institutional Review Board approval was obtained at each participating institution, and patients provided informed consent for their study participation including interviews during the initial hospitalization.
The dependent variable was prehospital delay, defined as the time between symptom onset and presentation to the first hospital. The time of symptom onset was obtained as part of the structured interview; the time of arrival at the emergency department of the first hospital was obtained through abstraction of medical records. Prehospital delay >6 hours was confirmed by 2 VIRGO investigators at Yale for patients lacking exact symptom onset information. Consistent with previous research, we combined the continuous prehospital delay time (symptom onset to arrival at the first hospital) and dichotomized prehospital delay (<6 and >6 hours) to clinically relevant categories: ≤2, >2 to 6, >6 to 12, and >12 hours. Patients who had continuous prehospital delay times available were categorized based on the calculated time. For patients who only had dichotomized prehospital delay time (<6 and >6 hours), those with a prehospital delay within 6 hours were categorized as >2 to 6 hours. Patients with a prehospital delay >6 hours were categorized as >6 to 12 hours. To examine prehospital delays <12 and >12 hours, <2, >2 to 6, and >6 to 12 were combined to create the <12 hours category.
The main independent variables were health insurance status and gender. Participants were divided into 3 groups according to health insurance information obtained during the interview during the initial hospitalization: (1) adequately insured, (2) underinsured, and (3) not insured. We stratified patients with insurance into those who were underinsured and adequately insured based on questions assessing financial barriers to care. Patients were underinsured if in the past year they reported avoidance of care or nonadherence to medications because of costs or because of the perception of medical costs as an economic burden ( Appendix ). Additional variables known to be associated with prehospital delay were gathered through structured interviews and medical record review during the initial hospitalization. These included sociodemographic characteristics (age, gender, race, marital, education, and employment status) and social support, as assessed by the 7-item Enhancing Recovery in Coronary Heart Disease Patients Social Support Inventory (ESSI-7). Perceived stress level was assessed by the 14-item Perceived Stress Scale (PSS-14). Pertinent medical history collected included depression, diabetes, renal dysfunction, obesity, and cardiac risk factors, such as family history, smoking, alcohol abuse, and recreational drug use. Clinical characteristics of AMI admission included Killip class on arrival (classified as class I vs class II to IV), absence of chest pain, and arrival to the hospital within regular work hours (weekday, non–night-time admission).
We examined the associations between gender and insurance groups (not insured, underinsured, and adequately insured), between gender and patient characteristics/prehospital delay, and between insurance groups and patient characteristics/prehospital delay in the US separately using the chi-square test for categorical variables and F-test or the median score test for continuous variables. Heterogeneity of the effects of gender on prehospital delay across insurance groups was tested using the Cochran-Mantel-Haenszel test.
We used hierarchical logistic models to account for the clustering effects at the site level and made sequential adjustments for patient characteristics. Separate hierarchical logistic models were used to evaluate the independent effect of the 3 insurance groups on prehospital delay of >12 hours and the independent effect of gender on prehospital delay of >12 hours, sequentially adjusting for site clustering, sociodemographics, medical history, and clinical characteristics on admission (Killip class, absence of chest pain, and arrival to the hospital within regular work hours). We also assessed interaction between gender and insurance groups using these models. Missing data were <1% except for Killip class (1.3%), ejection fraction (2.9%), number of dependents (4.0%), PSS-14 (6.2%), and ESSI (2.1%). For the multivariable model analyses, we imputed the missing values to the most common category for categorical variables and median value for the continuous variables and to 4 dummy indicators representing the missing values of the 5 variables mentioned earlier. Identical categories of delay were used for the Spanish cohort. Whether gender differences existed in the Spanish cohort in prehospital delay of >12 hours was examined using the chi-square test.
For all comparisons, we considered a p value <0.05 to be statistically significant. All analyses were performed using SAS 9.3 (SAS Institute Inc., Cary, North Carolina).
Results
The study population in the US included 2,951 patients with AMI (67% women) with a median age of 48 years (interquartile range 44 to 52). Table 1 lists the differences in demographics and socioeconomic characteristics and medical co-morbidities among women and men in our cohort.
Women n = 1987 | Men n = 964 | P | |
---|---|---|---|
Demographics/Socioeconomic Characteristics | |||
Age, median (IQR) (Years) | 48 (44, 52) | 48 (44, 52) | |
Race | <0.001 | ||
Black | 21% | 11% | |
White | 73% | 82% | |
Other | 6% | 7% | |
Has health insurance | 78% | 75% | <0.05 |
Insurance payer | <0.001 | ||
Private health insurance (commercial/PPO, HMO) | 50% | 58% | |
Medicare | 8% | 6% | |
Medicaid | 11% | 4% | |
Veterans Affairs | <1 % | 1% | |
Other | 9% | 6% | |
Insurance status | <0.001 | ||
Adequately insured | 37% | 42% | |
Underinsured | 41% | 33% | |
Not insured | 22% | 25% | |
Number of dependents, median | 2 | 3 | <0.001 |
Marital status | <0.001 | ||
With partner | 52% | 62% | |
Without partner | 47% | 37% | |
Other | 1% | <1% | |
Education status | 0.75 | ||
Unknown | <1% | <1% | |
< high school | 2% | 2% | |
Some high school | 41% | 41% | |
> high school | 56% | 56% | |
Employment status | <0.001 | ||
Working full-time | 43% | 65% | |
Working part-time | 13% | 7% | |
Not working | 44% | 28% | |
Depression diagnosis before AMI | 49% | 24% | <0.001 |
Major psychiatric disorder | 6% | 2% | <0.001 |
Perceived stress level by PSS-14, median | 27 | 24 | <0.001 |
Low social support by ESSI-7, median | 27 | 28 | 0.05 |
Chronic obstructive pulmonary disease | 14% | 6% | <0.001 |
Diabetes mellitus | 40% | 27% | <0.001 |
Renal dysfunction | 13% | 9% | <0.01 |
Dyslipidemia | 66% | 72% | <0.01 |
Hypertension | 67% | 65% | 0.18 |
Prior peripheral artery disease | 3% | 2% | 0.57 |
Prior angina/stable CAD | 28% | 26% | 0.36 |
Family history of CAD | 74% | 73% | 0.75 |
Prior cardiac catheterization | 23% | 22% | 0.53 |
Congestive heart failure | 6% | 3% | <0.001 |
Sleep apnea | 5% | 6% | 0.44 |
Alcohol abuse | 5% | 11% | <0.001 |
Smoked within last 30 days | 58% | 56% | 0.46 |
Cocaine use | 5% | 5% | 0.94 |
Illicit drug use | 8% | 9% | 0.81 |
Body mass index, median | 31 | 30 | <0.001 |
Clinical characteristics at AMI admission | |||
ST-segment elevation | 46% | 58% | <0.001 |
Ejection fraction <40% | 11% | 11% | 0.49 |
Killip class at arrival | <0.01 | ||
I | 90% | 92% | |
II or III or IV | 5% | 2% | |
Unknown | 5% | 5% | |
No chest pain | 5% | 6% | 0.31 |
Arrival to hospital within regular work hours | 36% | 33% | 0.20 |
Insurance status among the US patient cohort was highly variable: 1,141 (39%) were adequately insured, 1,132 (38%) were underinsured, and 678 (23%) were uninsured. Of the underinsured, 46% qualified as underinsurance because of avoidance of medical care in the past year because of costs, 49% from medication nonadherence in the past year because of costs, and 84% from feeling that medical costs were an economic burden in the past year. Although men had higher rates of being uninsured (25% vs 22%), more women were underinsured (41% vs 33%, p <0.001) or covered by Medicaid (11% vs 4%, p <0.001).
A substantial portion of the US cohort experienced clinically significant prehospital delays: 35% presented within 2 hours of symptom onset; 20%, between 2 and 6 hours; 10%, between 6 and 12 hours; and 35% presented >12 hours after symptom onset. US women were more likely than men to experience delays of >12 hours ( Figure 1 , p <0.001). This gender disparity persisted after sequential adjustment for sociodemographic factors, medical history, and clinical characteristics on admission (odds ratio 1.3; 95% confidence interval 1.1 to 1.6).
We found no significant association between prehospital delays >12 hours and insurance status (34% of the adequately insured, 34% underinsured, and 38% uninsured had >12 hours of delays; p = 0.17) or between delays of any length and insurance status (p = 0.30). Women in all insurance categories had higher rates of >12 hours prehospital delay compared with men ( Figure 2 , p = 0.12). We also did not find any significant interaction between gender and insurance status on prehospital delay (p = 0.30).
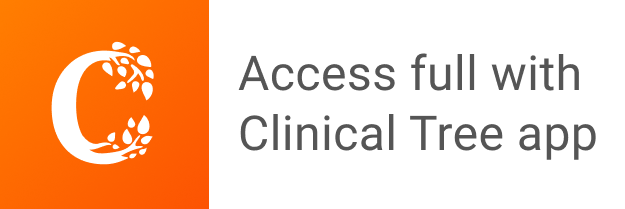