The impact of physical inactivity on heart failure (HF) mortality is unclear. We analyzed data from the HF Adherence and Retention Trial (HART) which enrolled 902 patients with New York Heart Association class II/III HF, with preserved or reduced ejection fraction, who were followed for 36 months. On the basis of mean self-reported weekly exercise duration, patients were classified into inactive (0 min/week) and active (≥1 min/week) groups and then propensity score matched according to 34 baseline covariates in 1:2 ratio. Sedentary activity was determined according to self-reported daily television screen time (<2, 2 to 4, >4 h/day). The primary outcome was all-cause death. Secondary outcomes were cardiac death and HF hospitalization. There were 196 inactive patients, of whom 171 were propensity matched to 342 active patients. Physical inactivity was associated with greater risk of all-cause death (hazard ratio [HR] 2.01, confidence interval [CI] 1.47 to 3.00; p <0.001) and cardiac death (HR 2.01, CI 1.28 to 3.17; p = 0.002) but no significant difference in HF hospitalization (p = 0.548). Modest exercise (1 to 89 min/week) was associated with a significant reduction in the rate of death (p = 0.003) and cardiac death (p = 0.050). Independent of exercise duration and baseline covariates, television screen time (>4 vs <2 h/day) was associated with all-cause death (HR 1.65, CI 1.10 to 2.48; p = 0.016; incremental chi-square = 6.05; p = 0.049). In conclusion, in patients with symptomatic chronic HF, physical inactivity is associated with higher all-cause and cardiac mortality. Failure to exercise and television screen time are additive in their effects on mortality. Even modest exercise was associated with survival benefit.
The impact of complete physical inactivity on various cardiovascular outcomes in healthy subjects without heart failure (HF) is well established. Two recent cohort studies have demonstrated that in subjects without established heart disease, physical inactivity and sedentary time were associated with new-onset HF. The impact of physical inactivity, compared to modest activity, on HF mortality in patients with established HF is not well studied; this is particularly true for patients with HF with preserved ejection fraction (HFpEF). In this study, we investigated the relation between physical inactivity and the risk of mortality and HF hospitalization in patients not engaged in cardiac rehabilitation or structured exercise training.
Methods
We analyzed data from Heart Failure Adherence and Retention Trial (HART), which was a multihospital, partially blinded, behavioral efficacy randomized controlled trial, funded by the National Institutes of Health [HL065547]. HART assessed the impact of self-management counseling versus education alone on the primary outcome of death or HF-related hospitalization in patients with symptomatic HF. Details of the trial were reported elsewhere. The study enrolled patients from 10 centers in the Chicago metropolitan area and was approved by the institutional review board of each collaborating institution [ NCT00018005 ].
Briefly, HART enrolled patients with HF with New York Heart Association (NYHA) class II or III symptoms, having HF with reduced ejection fraction (HFrEF) or HFpEF. Reduced systolic function was defined as left ventricular ejection fraction ≤40%. Eligible patients had to have HF symptoms for no less than the previous 3 months and either (1) ejection fraction ≤40% or (2) diuretic therapy for at least 3 months and one or more previous HF hospitalization. This was a null trial; it showed no significant impact of the self-management intervention, relative to the education-only control, on the composite end point of death or HF hospitalization and other HF outcomes. For the purpose of the present study, we assessed the impact of self-reported exercise and television screen time on HF outcomes over a median follow-up of 36 months.
No subjects in HART were enrolled in cardiac rehabilitation or a structured exercise training program. To assess patients’ inactivity level, a standardized questionnaire assessed daily exercise and duration of television watched at baseline and in follow-up visits at years 1, 2, and 3. Patients were asked the frequency/week and duration/episode of walking for exercise or performing other physical activities to improve fitness. Patients were also asked to report on the duration and frequency of television watching ( Supplementary Table 1 ). On the basis of patients’ responses, the sum of weekly exercise duration (minutes/week) was calculated. Because exercise duration could have varied during the course of the study, particularly after HF hospitalizations, we analyzed it as a time-dependent variable, averaging duration reported in all visits preceding the first adverse event of death or HF hospitalization. On the basis of the calculated average exercise duration, we divided the cohort into 2 study groups: inactive group , not engaged in any exercise (0 min/week), and active group , engaged in ≥1 min/week of exercise. The active group was further classified into partially active (1 to 89 min/week) and fully active (≥90 min/week). On the basis of the self-reported time spent watching television, patients were categorized into 3 sedentary-time groups: <2 h/day, 2 to 4 h/day, and >4 h/day. Time spent in other sedentary activities, such as reading, computer work, or driving, was not available for analysis.
During baseline and yearly visits, data were gathered on demographics, psychosocial characteristics, co-morbidities, prescription medications, adherence, and NYHA class. Socioeconomic status was defined as low if the patient’s annual household income was <$30,000 or if the highest attained education level was high school or lower. Medication adherence for key HF medications was assessed as the proportion of pills consumed relative to the prescribed amount using electronic pill caps. The dosages of various loop diuretics were converted into furosemide dose equivalents using an established conversion guide ( Supplementary Table 2 ). During each follow-up visit, the 6-minute walk distance was measured. Depression was defined by self-reported diagnosis or scoring ≥10 on the Geriatric Depression Screening scale. Chronic kidney disease was defined as glomerular filtration rate <60 ml/min/1.73 m 2 (Cockcroft–Gault formula) or dialysis therapy. Coronary artery disease was defined as a previous history of coronary revascularization or confirmed myocardial infarction. Quality of life was assessed using the Physical Function subscale of the 36-item Short-Form Health Survey (SF-36). We indexed the burden of 12 HF symptoms using the cardiopulmonary subscale of the HF Symptom Checklist ( Supplementary Table 3 ).
The primary outcome was all-cause death. Secondary outcomes were cardiac death and HF hospitalization. The median follow-up was 36 months (interquartile range 27 to 36 months). Outcomes were determined by a blinded adjudication committee. All patients (or their family members) were contacted every 3 months by telephone to determine the occurrence of hospitalization or death. Reports of death were confirmed by medical records, death certificates, or queries from the Social Security Death Index. Cardiac death was defined as death caused by myocardial infarction, arrhythmias, or pump failure. HF admissions were adjudicated by the presence of shortness of breath, peripheral edema, or chest radiographic evidence of pulmonary edema. HF admissions were confirmed if the patient responded to HF therapy or had a documented decrease in left ventricular function.
The chi-square test was used to compare dichotomous variables, which were expressed as numbers (percentages). The independent-samples t test was used to compare normally distributed continuous variables, which were expressed as means ± standard deviations. The Wilcoxon test was used to compare skewed continuous data.
Because patients were not randomly assigned to physical activity groups, we matched patients according to their propensity to being physically inactive. A multivariate logistic regression model (propensity model) was fit to calculate the probability of being physically inactive based on 34 baseline variables listed in Table 1 (identified with †). The resultant probabilities were then transformed into propensity score logits [ Ln 1/(1 − probability)]. SF-36 physical function score was included in the propensity model to balance patient’s functional abilities to assess the effect of physical inactivity as a matter of lifestyle rather than physical incapacitation. Six-minute walk distance was not included in the propensity model because of collinearity with NYHA class and SF-36 physical function score. Each patient in the inactive group was then matched to 2 active patients (1:2 ratio) with a propensity score within a caliper width of 0.2 standard deviation of the propensity score logits. Matching was performed using an algorithm written in Python software version 2.6.7 (Python Software Foundation, Python.org ). Furthermore, a second propensity model was fit to calculate the probability of >4 h/day of sedentary time, based on 34 baseline variables listed in Table 1 (identified with †).
Covariates | Before Propensity Matching (N=902) | After Propensity Matching (N=513) | ||||
---|---|---|---|---|---|---|
Inactive (0 min/wk) N=196 | Active (>0 min/wk) N=706 | P value ∗ | Inactive (0 min/wk) N=171 | Active (>0 min/wk) N=342 | P value ∗ | |
Propensity score logit | -0.64 ± 0.43 | -0.98 ± 0.42 | <0.001 | -0.73 ± 0.38 | -0.75 ± 0.39 | 0.598 |
Age (years) † | 65 ± 14 | 63 ± 13 | 0.149 | 64 ± 14 | 65 ± 14 | 0.882 |
Men † | 95 (48%) | 380 (54%) | 0.184 | 85 (50%) | 167 (49%) | 0.851 |
White † | 121 (62%) | 419 (59%) | 0.547 | 105 (61%) | 188 (55%) | 0.165 |
Low socioeconomic status † | 143 (73%) | 426 (60%) | 0.001 | 122 (71%) | 234 (68%) | 0.498 |
HFrEF † | 139 (71%) | 554 (78%) | 0.027 | 122 (71%) | 252 (74%) | 0.574 |
NYHA class III (vs. class II) † | 83 (42%) | 202 (29%) | <0.001 | 63 (37%) | 131 (38%) | 0.748 |
HART treatment arm † | 94 (48%) | 357 (51%) | 0.518 | 82 (48%) | 170 (50%) | 0.708 |
Third heart sound † | 6 (3%) | 31 (4%) | 0.406 | 6 (4%) | 9 (3%) | 0.578 |
Jugular venous distention † | 17 (9%) | 58 (8%) | 0.837 | 13 (8%) | 28 (8%) | 0.818 |
Cardiopulmonary symptoms index † | 0.68 ± 0.57 | 0.56 ± 0.56 | 0.001 | 0.65 ± 0.56 | 0.69 ±0.62 | 0.786 |
SF-36, physical function † | 38.4 ± 23.7 | 50.8 ± 24.6 | <0.001 | 40.9 ± 23.6 | 41.0 ± 22.3 | 0.716 |
6-minute walk distance (m) | 222 ± 134 | 268 ± 134 | <0.001 | 229 ± 132 | 228 ± 125 | 0.787 |
Coronary artery disease † | 111 (57%) | 385 (55%) | 0.601 | 97 (57%) | 187 (55%) | 0.660 |
Atrial fibrillation † | 75 (38%) | 286 (41%) | 0.570 | 68 (40%) | 132 (39%) | 0.798 |
Hypertension † | 152 (78%) | 524 (74%) | 0.341 | 129 (75%) | 271 (79%) | 0.327 |
Diabetes mellitus † | 92 (47%) | 270 (38%) | 0.028 | 76 (44%) | 150 (44%) | 0.900 |
Tobacco use † | 22 (11%) | 63 (9%) | 0.329 | 19 (11%) | 36 (11%) | 0.840 |
Chronic kidney disease † | 84 (43%) | 297 (42%) | 0.843 | 73 (43%) | 166 (49%) | 0.211 |
Stroke † | 27 (14%) | 78 (11%) | 0.292 | 21 (12%) | 47 (14%) | 0.645 |
Depression † | 84 (43%) | 254 (36%) | 0.078 | 72 (42%) | 142 (42%) | 0.899 |
Chronic lung Disease † | 30 (15%) | 95 (13%) | 0.507 | 24 (14%) | 57 (17%) | 0.441 |
Arthritis † | 110 (56%) | 333 (47%) | 0.027 | 92 (54%) | 181 (53%) | 0.851 |
Body mass index (kg/m 2 ) † | 32.0 ± 7.6 | 30.8 ± 7.7 | 0.061 | 31.5 ± 7.5 | 31.8 ± 8.3 | 0.382 |
Hemoglobin (g/dL) † | 12.7 ± 1.6 | 13.1 ± 1.6 | 0.040 | 12.8 ± 1.5 | 12.7 ± 1.6 | 0.340 |
Serum sodium (mmol/dL) † | 140.3 ± 3.1 | 139.9 ± 3.1 | 0.106 | 140.3 ± 3.2 | 140.3 ± 3.1 | 0.694 |
Serum potassium (mEq/dL) | 4.58 ± 0.52 | 4.53 ± 0.53 | 0.397 | 4.56 ± 0.50 | 4.57 ± 0.52 | 0.921 |
Serum albumin (g/dL) † | 4.10 ± 0.56 | 4.13 ± 0.44 | 0.102 | 4.13 ± 0.57 | 4.08 ± 0.40 | 0.661 |
Serum total bilirubin (mg/dL) † | 0.66 ± 0.37 | 0.64 ±0.37 | 0.701 | 0.64 ± 0.33 | 0.64 ± 0.36 | 0.541 |
Serum creatinine (mg/dL) | 2.03 ± 2.56 | 1.64 ± 1.53 | 0.042 | 1.97 ± 2.61 | 1.76 ± 1.77 | 0.840 |
Sedentary television watching (h/day) | 0.011 | 0.740 | ||||
< 2 | 50 (26%) | 221 (31%) | 44 (26%) | 92 (27%) | ||
2 – 4 | 65 (33%) | 273 (39%) | 57 (33%) | 122 (36%) | ||
> 4 | 81 (41%) | 212 (30%) | 70 (41%) | 128 (37%) | ||
Medical Treatment | ||||||
Medication adherence (%) † | 72 ± 40 | 64 ± 58 | 0.009 | 57 ± 39 | 53 ± 39 | 0.404 |
ACEi/ARB use † | 156 (80%) | 617 (87%) | 0.006 | 144 (84%) | 279 (82%) | 0.460 |
β-Blocker use † | 126 (64%) | 510 (72%) | 0.031 | 114 (67%) | 215 (63%) | 0.397 |
Spironolactone use † | 47 (24%) | 195 (28%) | 0.309 | 46 (27%) | 80 (23%) | 0.384 |
Loop diuretic (mg/day) † | 76 ± 71 | 58 ± 56 | 0.003 | 73 ± 68 | 63 ± 60 | 0.148 |
Thiazide 2 nd diuretic use † | 10 (5%) | 42 (6%) | 0.653 | 9 (5%) | 23 (7%) | 0.519 |
Statin use † | 89 (45%) | 363 (51%) | 0.137 | 81 (47%) | 164 (48%) | 0.901 |
Aspirin use † | 89 (45%) | 333 (47%) | 0.662 | 80 (47%) | 158 (46%) | 0.900 |
∗ t -test (normally distributed continuous variables), X 2 test (dichotomous data), Wilcoxon test (skewed data).
† Covariates accounted for in the propensity-score matching.
Kaplan–Meier curves and the log-rank test were used to compare time to event occurrence. Risk was expressed as hazard ratio (HR) and 95% confidence interval (CI), calculated using univariate and multivariate Cox regression models. To confirm the findings of the propensity-matched analyses, we analyzed outcomes in the entire cohort using multivariate Cox proportional hazards models, adjusting for the calculated propensity scores. The risk (HR, CI) of adverse outcomes with graded levels of exercise (inactive, partially active, fully active) and television screen time (<2, 2 to 4, >4 h/day) was analyzed using inverse probability-weighted Cox regression models. The inverse probability weighting factors used were 1/probability, for the inactive and sedentary groups, and 1/(1 − probability), for the remainder of the samples. The proportional hazards assumption with respect to Cox regression modeling was confirmed using “ log minus log ” survival plots. The Mantel–Haenszel extension of the chi-square test for trend was used to demonstrate stepwise increase in event rates.
As an exploratory analysis, we studied within the propensity-matched cohort the impact of physical inactivity on the primary outcome within the prespecified subgroups of gender, age, ethnicity (white vs others), NYHA class (II vs III), HFrEF versus HFpEF, coronary disease status, chronic kidney disease status, obesity (body mass index ≥30 kg/m 2 ), and HART treatment arm. We also used multivariate Cox regression modeling to test for an interaction between physical inactivity and subgroup strata and adjusting for covariates with >10% postmatching absolute standardized difference. We then confirmed the findings from the subgroup analyses in the entire cohort using multivariate Cox regression models, adjusted for the calculated propensity score logits.
Two-tailed p values <0.05 were considered significant. The PASW 18.0 software (SPSS, Inc., Chicago, Illinois) and SAS 9.3 (SAS Institute, Cary, North Carolina) were used for statistical analyses.
On the basis of the observed rate of death in HART (20.8%), we calculated, post hoc, that the available propensity-matched cohort provided the study with 83% power to detect an increase in the rate of all-cause mortality by two-thirds (log-rank test, 2-tailed α = 0.05).
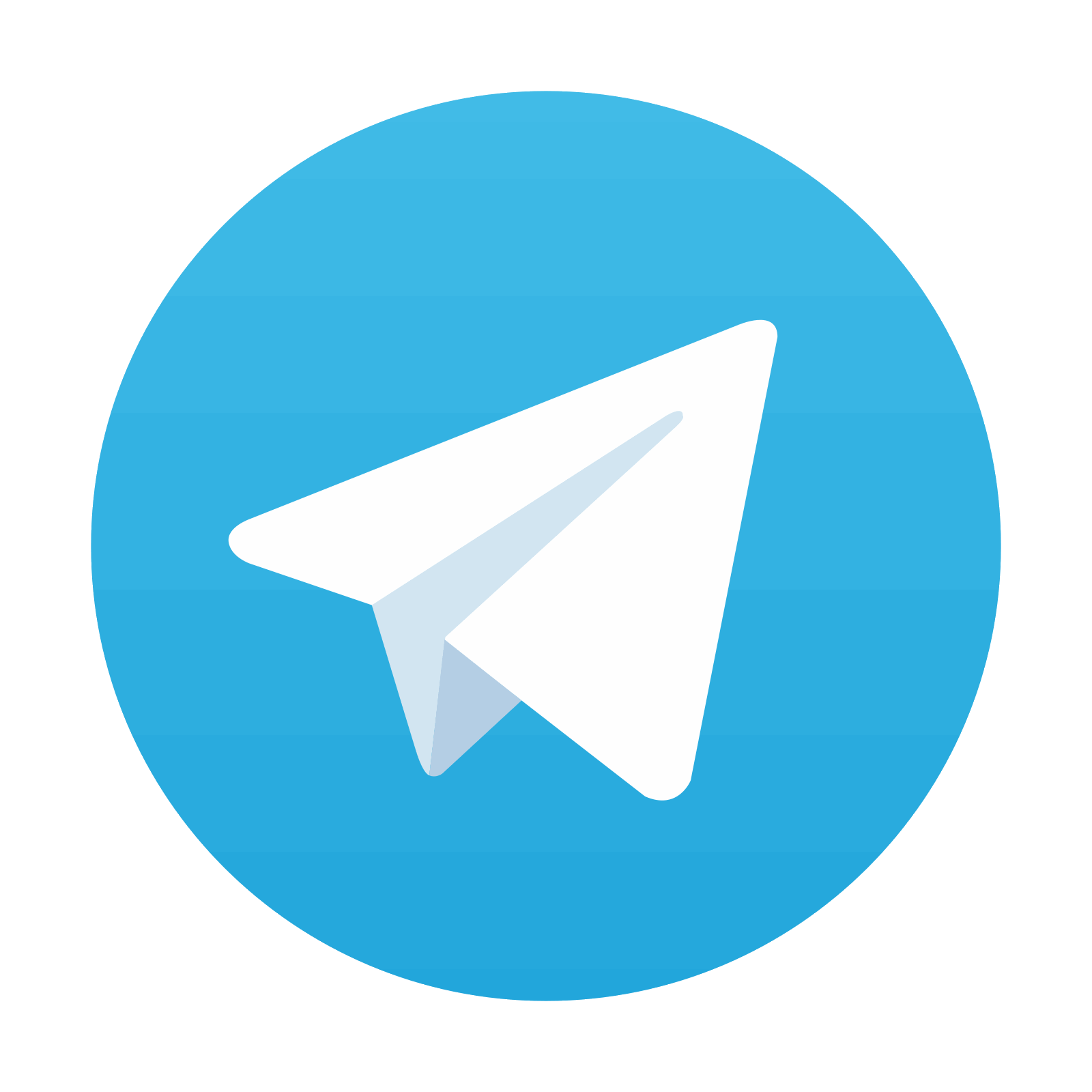
Stay updated, free articles. Join our Telegram channel

Full access? Get Clinical Tree
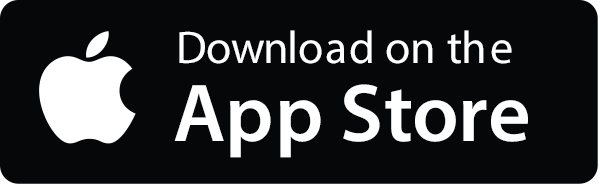
