Peter Libby, Robert E. Gerszten, Paul M Ridker
Biomarkers, Proteomics, Metabolomics, and Personalized Medicine
We use biomarkers daily in the practice of cardiovascular medicine. Moreover, the use of biomarkers has the potential to continue to improve our ability to provide clinically effective and cost-effective cardiovascular medicine in the years to come. Appropriate risk stratification and targeting of therapies should not only help improve patient outcomes but also assist in responding to the urgent need to “bend the cost curve” of medical care. In particular, excessive use of imaging biomarkers increases the cost of medical care and can jeopardize patient outcomes (for example, radiation exposure or complications of administering contrast material or investigating incidental findings). Inappropriate use or interpretation of blood biomarkers (e.g., cardiac troponin levels) can lead to unnecessary hospitalization or procedures as well.
Despite the current usefulness of biomarkers, their future promise, and the critical need to use them appropriately, a great deal of misunderstanding surrounds their current clinical application. In addition, contemporary technologies have the potential to greatly expand the gamut of biomarkers relevant to cardiovascular practice. Emerging genetic, proteomic, metabolomic, and molecular imaging strategies will surely transform the landscape of cardiovascular biomarkers (see also Chapters 7, 8, 9, and 42). This chapter provides a primer on cardiovascular biomarkers by defining some terms and discussing how the application of biomarkers can assist in clinical care, in addition to exploring some emerging technologies. Finally, we discuss an approach to the rigorous evaluation of the clinical usefulness of biomarkers. Advances in cardiovascular biology and the application of novel technologies have identified a plethora of novel cardiovascular biomarkers of potential clinical usefulness—begging the question of whether a novel biomarker adds value to existing and often better-validated biomarkers. Thus clinicians need tools to evaluate these emerging biomarkers, adoption of which may elevate clinical practice and improve patient outcomes.
What is a Biomarker?
For regulatory purposes, the U.S. Food and Drug Administration (FDA) first defined a biomarker in 1992 as “a laboratory measure or physical sign that is used in therapeutic trials as a substitute for a clinically meaningful end point that is a direct measure of how a patient feels, functions, or survives and is expected to predict the effect of the therapy.” At that time the FDA considered a surrogate endpoint as “reasonably likely, based on epidemiologic, therapeutic, pathophysiologic, or other evidence to predict clinical benefit.”1 The National Institutes of Health (NIH) convened a working group in 1998 that offered some parallel operating definitions to guide the biomarker field (Table 10-1).2 They defined a biologic marker—biomarker for short—as “a characteristic that is objectively measured and evaluated as an indicator of normal biologic processes, pathogenic processes, or pharmacologic responses to a therapeutic intervention.” Thus the NIH definition embraces not only soluble biomarkers in circulating blood but also “bedside biomarkers” such as anthropomorphic variables obtainable with a blood pressure cuff or a tape measure at the point of care. This broad definition encompasses not only measurements of biomarkers in blood (Fig. 10-1A) but also those derived from a variety of techniques, including measurements from imaging studies (Fig. 10-1B). Imaging biomarkers can include those derived from classic anatomic approaches. Imaging modalities now offer functional information, such as estimates of ventricular function, myocardial perfusion, and the like. Molecular imaging has the potential to target specific molecular processes. A functional classification of biomarkers helps sort through the plethora encountered by the clinician inasmuch as biomarkers can reflect a variety of biologic processes or organs of origin. For example, as a first approximation, cardiac troponin reflects myocardial injury, brain natriuretic peptide reflects cardiac chamber stretch, C-reactive protein (CRP) reflects inflammation, and the estimated glomerular filtration rate reflects kidney function (see Fig. 10-1B).
The NIH working group also provided further definitions relevant to the field of biomarkers. They defined a “surrogate endpoint” as “a biomarker intended to substitute for a clinical endpoint. A surrogate endpoint is expected to predict clinical benefit (or harm), or lack of benefit (or harm) based on epidemiologic, therapeutic, pathophysiologic, or other scientific evidence.” (Note that the NIH definitions do not include the commonly used term “surrogate marker.”) (Table 10-1). Thus a surrogate endpoint is a biomarker that has been “elevated” to surrogate status. This distinction has particular importance in the regulatory aspects of cardiovascular medicine. For example, the FDA previously accepted a certain degree of reduction in hemoglobin A1c (HbA1c) as a criterion for registration of a novel oral hypoglycemic agent—thus HbA1c was considered a biomarker accepted as a surrogate endpoint. Current FDA guidance now requires a cardiovascular safety study for the registration of new medications that target diabetes.3 This policy indicates doubts about the fidelity of a drop in HbA1c as a surrogate endpoint for reduced cardiovascular risk in the eyes of regulatory authorities despite its value as a biomarker of glycemia.
The NIH working group defined a “clinical endpoint” as “a characteristic or variable that reflects how a patient feels, functions, or survives” (Table 10-1). Pivotal or phase III cardiovascular trials aspire to use clinical endpoints as defined above. The distinction between biomarkers, surrogate endpoints, and clinical endpoints has crucial implications as practitioners, regulators, and payers increasingly demand evidence of improvements in actual clinical outcomes rather than mere manipulation of biomarkers as a criterion for adoption of a treatment in clinical practice.
Clinical Applications of Cardiovascular Biomarkers
Much of the prevailing confusion regarding biomarkers involves framing the question that one wants to answer with the use of a biomarker (Fig. 10-1C). We can classify the goals of application of cardiovascular biomarkers into several rubrics.
4. Targeting of therapy: In clinical practice, using biomarkers to target therapy has great usefulness and promise as we move toward a more comprehensive “personalized medicine” approach to practice (see Chapter 8). Examples of biomarkers used to target therapy include troponin measurements to triage patients with acute coronary syndromes for early invasive management or measurement of high-sensitivity C-reactive protein (hsCRP) to allocate statin treatment to individuals with below-average LDL cholesterol.
Clinical use of cardiovascular biomarkers requires a clear understanding of how they should be used. Many biomarkers provide clinically useful information when measured once at “baseline.” A baseline measurement of high-density lipoprotein (HDL) cholesterol, for example, indubitably correlates inversely with future risk for cardiovascular events. Yet serial measurement of biomarkers to document a change does not always guarantee a clinical benefit. In the case of HDL, recent large-scale trials that have measured clinical endpoints have cast doubt on the fidelity of a rise in HDL cholesterol as a predictor of clinical benefit (see Chapter 45).
Biomarkers require rigorous validation before adoption into clinical practice. In cardiovascular medicine, LDL cholesterol has high reliability as a biomarker; it satisfies the modified Koch postulates. LDL levels prospectively predict cardiovascular risk, and drops in LDL generally correlate with improved outcomes. Not all biomarkers, though, have proved as faithful in predicting clinical events. In the 1960s and 1970s, for example, most of the cardiovascular community considered ventricular premature depolarizations on the electrocardiogram as important biomarkers for lethal arrhythmias. Numerous strategies have been aimed at suppressing ventricular ectopy. CAST (Cardiac Arrhythmia Suppression Trial), however, showed that drugs capable of suppressing ventricular premature depolarizations actually worsened clinical endpoints. The short-term improvements in indices of cardiac contractility produced by inotropic agents similarly led to worsened clinical outcomes, including increased mortality. These examples illustrate the necessity of rigorous validation of biomarkers before adoption into clinical practice.
Another important consideration in the use of cardiovascular biomarkers involves the question of causality. LDL cholesterol exemplifies a causal biomarker, one that clearly participates in the pathogenesis of atherosclerosis. Its levels prospectively correlate with risk for cardiovascular events and the development of atherosclerotic lesions identified by a variety of imaging modalities. A variety of independent manipulations of LDL levels correlate with clinical outcomes. Finally, very strong genetic evidence based on mendelian disorders (e.g., familial hypercholesterolemia) and unbiased genome-wide association scans, as well as mendelian randomization analyses, has established LDL cholesterol as a causal risk factor in atherosclerotic cardiovascular disease and as a generally valid surrogate endpoint offering great value in clinical practice (see Chapter 45).4,5 For a biomarker that has a causal role, the expected random population distribution of a polymorphism that determines high or low biomarker concentrations would be skewed in individuals, depending on their disease status.
Other biomarkers, although clearly clinically useful, do not participate in the causal pathway for disease. For example, fever has served since antiquity as an important biomarker of infection. Resolution of fever correlates with successful resolution of infectious processes. Yet fever does not participate causally in the pathogenesis of infection but merely serves as a biomarker of the host defenses against the infectious process. Similarly, the use of hsCRP measurements improves the prediction of cardiovascular risk, and reductions in CRP correlate with clinical benefit in many cases. Yet, evidence supporting a causal role for CRP in the pathogenesis of cardiovascular disease lacks strength.6
These examples illustrate how a biomarker does not have to reside in the causal pathway of a disease to have clinical usefulness. A clear and early exposition of the uses and pitfalls in the application of biomarkers emerged from the landmark work of Fleming and DeMets (Fig. 10-2).7 Biomarkers have the greatest potential for validity when there is one causal pathway and when the effect of intervention on true clinical outcomes is mediated directly through the biomarker surrogate (Fig. 10-2A). But, biomarker development can fail when the biomarker turns out not to be in the causal pathway, when the biomarker is insensitive to the specific intervention’s effect, or when the intervention of interest has a mechanism of action (or a toxicity) that is independent of the pathway described by the biomarker (Fig. 10-2B-E). These examples do not mean that biomarkers lack value. Quite the contrary, few—if any—novel biologic fields could develop without biomarker discovery and validation. Yet surrogate endpoints probably will not replace large-scale randomized trials that address whether interventions reduce actual event rates.
Novel Technologies in the Identification of Biomarkers
The limitations of currently available biomarkers for screening or prognostic use underscore the importance of identifying “uncorrelated” or “orthogonal” biomarkers associated with novel disease pathways. Most current biomarkers have been developed as an extension of targeted physiologic studies investigating known pathways such as tissue injury, inflammation, or hemostasis. By contrast, emerging technologies now enable the systematic, unbiased characterization of variation in proteins and metabolites associated with disease conditions.
Introduction to Proteomics and Metabolomics
Of the emerging platforms for biomarker discovery, perhaps none have garnered more recent attention than proteomics and metabolomics. Proteomics aims to catalogue the entire protein products of the human genome. By contrast, metabolomics attempts to systemically capture smaller biochemical compounds, including simple amino acids and related amines, as well as lipids, sugars, nucleotides, and other intermediary metabolites. Although still in their infancy with respect to other approaches, proteomics and metabolomics offer insight into the full complexity of a given disease phenotype (Fig. 10-3). Because proteins and metabolites are downstream of genetic variation and transcriptional changes, they provide instantaneous “snapshots” of the state of a cell or organism. They can rapidly change in response to environmental stressors such as exercise or directly by the ingestion of foods or other compounds. A growing body of literature suggests unanticipated roles of small proteins and metabolites in the control of biologic functions such as blood pressure and energy homeostasis.8,9 Thus metabolomics and proteomics may not only identify novel biomarkers but also provide information on biology and highlight potential therapeutic targets.
The term proteome was coined in the 1990s with the increasing realization that although all cells of a given organism contain an equivalent genomic content, their protein content does not represent all possible proteins that the genome can express. Selective gene expression during development and differentiation and in response to external stimuli results in each cell expressing only a subset of the encoded proteins at any given time. One can speak not only of the general human proteome but also more specifically about the proteome of tissues such as the heart, of specific cells such as cardiac myocytes, and even of subproteomes that correspond to particular organelles or biologic compartments, such as mitochondria.
The proteome provides information beyond the messenger RNA (mRNA) expression profile of a particular genome. Studies suggest that gene expression often correlates poorly with protein levels.10 Protein expression depends not only on transcription but also on mRNA stability and rates of protein synthesis and degradation, so the presence or absence of mRNA may not accurately reflect levels of the corresponding protein. Following transcription and translation, proteins may undergo one or more of dozens of potential post-translational modifications (such as phosphorylation, glycosylation, acetylation, or sulfation) at multiple sites. Subsequent enzymatic and nonenzymatic alterations greatly expand the number of simultaneously existing protein species.
When compared with proteomics techniques, metabolomics technologies focus on smaller compounds, generally less than 2 kDa in size. Metabolites are usually easily separated from protein constituents by simple extraction techniques and precipitation and removal of the proteins. As early as the 1970s, Arthur Robinson and Linus Pauling postulated that the quantitative and qualitative pattern of metabolites in biologic fluids reflected the functional status of the complex biologic system from which they were derived.11 The term “metabolic profiling” was introduced to describe data obtained from gas chromatographic analysis of a patient sample.12
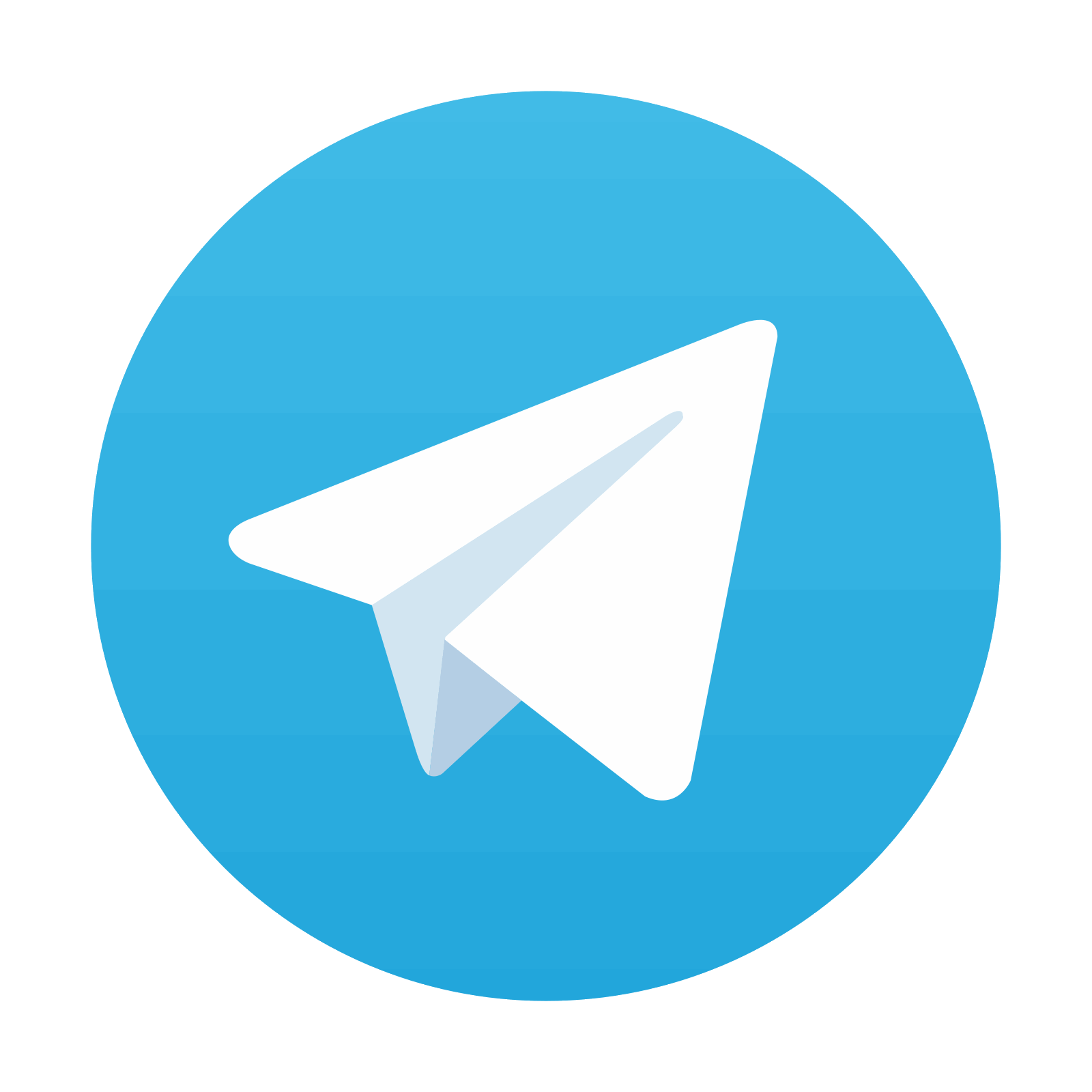
Stay updated, free articles. Join our Telegram channel

Full access? Get Clinical Tree
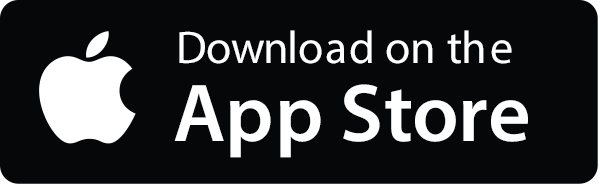
