Background
Parameters describing intraventricular time differences are increasingly assessed in both adults and children. However, to appreciate the implications of these parameters in children, knowledge of the applicability of adult techniques in children is essential. Hence, the aim of this study was to assess the applicability of speckle-tracking strain–derived parameters in children, paying special attention to age and heart rate dependency.
Methods
One hundred eighty-three healthy subjects (aged 0–19 years) were included. Left ventricular global peak strain, time to global peak strain, and parameters describing intraventricular time differences were assessed using speckle-tracking strain imaging in the apical two-chamber, three-chamber, and four-chamber views (longitudinal strain) and the parasternal short-axis view (radial and circumferential strain). Parameters describing intraventricular time differences included the standard deviation of time to peak strain and differences in time to peak strain between two specified segments. Age and heart rate dependency were evaluated using regression analysis, and intraobserver and interobserver variability were tested.
Results
Acquisition and analysis of longitudinal six-segment time-strain curves was successful in 94.8% of subjects and radial and circumferential time-strain curves in 89.5%. No clinically significant linear relation was observed between age or heart rate and parameters describing intraventricular time differences. The coefficient of variation of time to global peak strain parameters was <10, while it was >10 for parameters describing intraventricular time differences.
Conclusions
The feasibility of speckle-tracking strain analysis in children is relatively good. Furthermore, no linear relation was observed between age or heart rate and parameters describing intraventricular time differences. However, the limited reproducibility of some parameters describing intraventricular time differences will confine their applicability in clinical practice.
Normal left ventricular (LV) myocardial motion involves a synchronous movement. Disturbance of this coordinate wall motion can result in inefficient pump function, which may lead to depressed LV systolic properties and heart failure. To evaluate this possible pathophysiologic phenomenon, several techniques have been introduced to assess intraventricular time differences. The ultimate goal of these techniques is to detect cardiac dyssynchrony and to predict response to cardiac resynchronization therapy (CRT) as therapy for heart failure. Although electrical dyssynchrony has proven to be useful, the realization of these goals for mechanical dyssynchrony is still a long way off. Even in adults, there is still considerable debate about the need and optimal method to detect mechanical dyssynchrony for CRT therapy. Nevertheless, from a pathophysiologic point of view, the description of intraventricular time differences is certainly interesting, as increased time differences have been shown in several groups of adult patients with heart failure compared with healthy controls.
Recently, intraventricular time differences were also evaluated in children, and significantly higher time differences were observed in pediatric cardiomyopathy patients compared with controls. Furthermore, the observed intraventricular time differences correlated to the severity of LV dysfunction. These studies may imply the presence of dyssynchrony in pediatric patients with heart failure.
However, it is difficult to appreciate the meaning of these findings without knowledge of the applicability of techniques used in adults to describe intraventricular time differences in children. For example. age and heart rate variations, which are more pronounced in children, may demand for corrections.
Speckle-tracking strain analysis is one of the techniques often used in adults to assess LV intraventricular time differences. In children, studies defining normal values, age and heart rate dependency, and reproducibility of speckle-tracking strain–derived time to peak strain are limited. Although some studies have touched on these topics, a solid analysis in a sizable group of healthy children is scarcely available.
Accordingly, the objective of the present study was to provide more insight into the applicability of LV speckle-tracking strain–derived parameters describing intraventricular time differences and time to peak strain in children. In addition, special attention was paid to the age and heart rate dependency of these parameters.
Methods
Study Subjects
All consecutive healthy children (aged 1 month to 19 years) who were referred to the Leiden University Medical Center with an asymptomatic innocent heart murmur, cardiac palpitations, or idiopathic chest pain were prospectively evaluated for inclusion in our study. Subjects were eligible for inclusion if routine echocardiographic evaluation showed structurally normal hearts and normal cardiac function. Furthermore, to affirm that the subjects were in general good health, a physical examination was performed and the medical history was checked. Children using medication or in whom any type of chronic disease had been diagnosed were not eligible for inclusion. Approval for this study was obtained from the institutional review board, and written informed consent was obtained from the children and/or their parents.
Demographic parameters including age, body weight, length, sex, body surface area and, body mass index were documented at study inclusion. Furthermore, a 12-lead electrocardiogram was made in every subject. On the electrocardiogram, QRS duration and corrected QT interval (QTc = QT/√RR) were measured in milliseconds. Two-dimensional echocardiography including speckle-tracking strain imaging was performed in all subjects to define the normal ranges of LV strain, time to peak strain, and intraventricular time differences.
Echocardiography
Echocardiography was performed using a Vivid 7 scanner (GE Vingmed Ultrasound AS, Horten, Norway). All recordings were performed while the subject was in sinus rhythm with a stable heart rate. Images were stored in a digital format for offline analysis using EchoPAC software version 11.1.8 (GE Vingmed Ultrasound AS).
In each subject, M-mode recordings of the LV long axis were recorded. Furthermore, Doppler flow recordings of the LV outflow tract were recorded to determine aortic valve closure, which was identified by the delineation of the aortic valve click. Finally, two-dimensional grayscale harmonic images of the apical two-chamber, three-chamber, and four-chamber views and images of the parasternal short-axis views at the level of the papillary muscle were recorded. Each image consisted of at least three consecutive cardiac cycles. Images were obtained with optimized sector width and frame rate (preferably 60–80 frames/sec). A frame rate < 60 frames/sec was accepted only if the heart rate was <80 beats/min.
Using Simpson’s biplane method of disks, LV ejection fraction was calculated from the apical two-chamber and four-chamber views.
Speckle-Tracking Strain Imaging
Global peak strain, time to global peak strain, and LV intraventricular time differences in healthy children were studied with speckle-tracking strain imaging in the apical two-chamber, three-chamber, and four-chamber views (longitudinal strain) and in the parasternal short-axis view (radial and circumferential strain).
To allow speckle-tracking strain analysis, manual endocardial border tracing at end-systole was used to set the region of interest in the two-dimensional grayscale images. Subsequently, the region of interest was automatically divided into six segments for further analysis. In the four-chamber view, this included the basal, mid, and apical segments of the LV lateral wall and of the interventricular septum. The two-chamber view was divided into basal, mid, and apical segments of the LV inferior and anterior walls. In the three-chamber view, the segments included basal, mid, and apical segments of the LV posterior and anteroseptal walls. Finally, the short-axis image was divided into septal, anteroseptal, anterior, lateral, posterior, and inferior segments to evaluate both radial and circumferential strain. In each segment, tracking quality was automatically evaluated, and this resulted in automatic rejection or acceptation of the segment. Although the observer could override this automatic decision on the basis of visual evaluation, this was used very conservatively. Accordingly, if a segment was rejected, tracking was further optimized by visual reevaluation. If all six segments were accepted by the observer, the analysis was judged successful.
Data obtained by speckle-tracking were displayed in longitudinal time-strain curves for each segment in the two-chamber, three-chamber, or four-chamber view and radial and circumferential time-strain curves for each segment in the short-axis image ( Figure 1 ). From these time-strain curves, segmental peak strain was obtained. Peak strain was defined as the highest strain value at any time point during one cardiac cycle. In addition, segments that had peak strain after aortic valve closure in the longitudinal four-chamber view were identified. In these segments, the absolute difference between strain at aortic valve closure and peak strain at any point in the cardiac cycle was assessed. To ensure reliability of this analysis, heart rates had to be similar when measuring aortic valve closure and the images taken for strain measurements. Finally, time to peak strain was assessed in each segmental time-strain curve using the beginning of the QRS complex as a reference point.

Individual peak strain and time to peak strain measurements were subsequently combined in several models. For longitudinal strain, these models included segments of the four-chamber (six segments), four-chamber and two-chamber (12 segments), and four-chamber, three-chamber, and two-chamber (18 segments) views, respectively. For radial and circumferential strain, these models each included the six segments of the short-axis view. Global peak strain parameters were calculated as the mean of the peak strain measurements in one model. Time to global peak strain parameters were calculated as the mean of the time to peak strain measurements in one model.
Furthermore, parameters describing intraventricular time differences were calculated, including the standard deviation of time to peak strain of several segments (SDt) and the differences in time to peak strain between two specified segments. To describe longitudinal intraventricular time differences, the six-segment, 12-segment, and 18-segment models were used to calculate the standard deviation, yielding longitudinal SDt-6, SDt-12, and SDt-18 parameters. Furthermore, the difference in time to peak strain between the interventricular septum and the basal LV lateral wall (septal-lateral [S-L] delay) was determined to describe longitudinal intraventricular time differences. Radial time-strain curve–derived parameters describing intraventricular time differences included radial SDt-6 and the difference in time to peak strain between the anteroseptal and posterior segment (anteroseptal-posterior [AS-P] delay). Finally, regarding circumferential strain, parameters describing intraventricular time differences included circumferential SDt-6 and circumferential AS-P delay.
Intraobserver and interobserver variability were determined in 39 randomly selected subjects. To assess intraobserver variability, the first observer (L.M.K.) generated time-strain curves anew from the images ≥3 months after the first evaluation. Interobserver variability was assessed by comparing the results of the first observer (L.M.K.) with results of a second observer (A.A.W.R.). The second observer was blinded to the results of the first observer during the evaluation.
Statistical Analysis
Normally distributed continuous data are expressed as mean ± SD. Not normally distributed data are expressed as median (interquartile range). The relations of QRS and QTc duration with intraventricular time differences were evaluated using scatterplots and Pearson’s correlation coefficient. This was also used to evaluate the correlation between longitudinal SDt-6 and SDt-12 and SDt-18, respectively.
Furthermore, the influence of age and/or heart rate on echocardiographic measurements, including global peak strain, time to global peak strain, and intraventricular time differences, was evaluated using univariate and multivariate linear regression analysis. Heart rate was evaluated using two different parameters, including RR and √RR. Finally, interobserver variability was evaluated using Bland-Altman analysis (including mean bias and 95% limits of agreement) and the coefficient of variation. For this analysis, only the first measurement of observer 1 was used. The coefficient of variation was calculated as follows: (standard deviation of the difference between the measurements of the two observers)/(mean of all measurements) × 100%. Intraobserver variability was evaluated using the intraclass correlation coefficient. All statistical analyses were performed using SPSS version 20 (IBM Corp, Armonk, New York), with P values < .05 considered statistically significant.
Results
Study Subjects
A total of 183 subjects were eligible for inclusion in our study. After echocardiographic evaluation, 11 subjects were excluded on the basis of poor echocardiographic windows, leaving a total of 172 subjects (98 male) for further evaluation. Subject characteristics and conventional echocardiographic parameters are presented in Table 1 . Age and √RR were significantly correlated ( R 2 = 0.73).
Variable | Age (y) | ||||
---|---|---|---|---|---|
<1 | 1–4 | 5–9 | 10–14 | 15–19 | |
( n = 37) | ( n = 35) | ( n = 37) | ( n = 45) | ( n = 18) | |
Male | 17 (46%) | 19 (54%) | 20 (54%) | 34 (76%) | 8 (44%) |
Age (y) | 0.1 (0.1–0.3) | 3.2 (2.3–3.8) | 6.9 (6.2–8.2) | 12.5 (11.1–13.8) | 16.0 (15.9–16.9) |
Heart rate (beats/min) | 148 ± 15 | 107 ± 12 | 88 ± 12 | 71 ± 12 | 71 ± 12 |
Body mass index (kg/m 2 ) | 16 ± 2 | 16 ± 1 | 16 ± 2 | 19 ± 3 | 22 ± 2 |
Body surface area (m 2 ) | 0.3 ± 0.1 | 0.6 ± 0.1 | 0.9 ± 0.2 | 1.4 ± 0.2 | 1.8 ± 0.2 |
LV ejection fraction (%) | 58 ± 4 | 59 ± 4 | 61 ± 4 | 60 ± 5 | 60 ± 5 |
QRS duration (msec) | 67 ± 8 | 75 ± 8 | 81 ± 10 | 85 ± 9 | 87 ± 12 |
QTc (msec) | 406 ± 25 | 416 ± 32 | 407 ± 22 | 395 ± 33 | 409 ± 27 |
Frame rates ranged from 44 to 155 frames/sec. The median frame rate in the short-axis view was 77 frames/sec and the median frame rates in the two-chamber, three-chamber, and four-chamber views were 76, 76, and 77 frames/sec, respectively. Acquisition and analysis of good-quality longitudinal four-chamber view time-strain curves succeeded in 94.8% of the subjects ( n = 163) and radial and circumferential time-strain curves in 89.5% of the subjects ( n = 154). Of the 111 subjects with good-quality two-chamber views, analysis succeeded in 85.6% of the subjects ( n = 95). Finally, of the 104 subjects with good-quality three-chamber views, analysis succeeded in 86.5% of the subjects ( n = 90). The results of the intraobserver and interobserver variability analyses are depicted in Table 2 and Figure 2 .
Variable | Interobserver | |||
---|---|---|---|---|
Intraobserver | Bland-Altman analysis | |||
ICC | Bias | 95% limits of agreement | CV | |
Global peak strain | ||||
Longitudinal six-segment model | 0.79 | 0.0 | −4.1 to 4.1 | 10 |
Radial | 0.72 | −3.8 | −27.9 to 20.3 | 24 |
Circumferential | 0.67 | 1.2 | −4.5 to 6.9 | 13 |
Time to global peak strain | ||||
Longitudinal six-segment model | 0.96 | 8.6 | −29.6 to 46.8 | 6 |
Radial | 0.93 | 12.7 | −37.1 to 62.5 | 8 |
Circumferential | 0.96 | 2.0 | −35.0 to 39.0 | 6 |
Intraventricular time-differences | ||||
Longitudinal | ||||
S-L delay | 0.55 | −4.4 | −55.9 to 47.1 | 86 |
SDt-6 | 0.66 | −0.04 | −21.4 to 21.3 | 38 |
Radial | ||||
AS-P delay | 0.36 | −9.1 | −66.7 to 48.5 | 93 |
SDt-6 | 0.57 | −1.8 | −27.7 to 24.1 | 70 |
Circumferential | ||||
AS-P delay | 0.37 | −9.4 | −99.2 to 80.4 | 107 |
SDt-6 | 0.45 | −9.0 | −53.7 to 35.7 | 74 |

Global Peak Strain
Global peak longitudinal, radial, and circumferential strain data as assessed in the left ventricles of our normal population are summarized in Table 3 . Using linear regression, no linear relation was observed between age and most global peak strain parameters, except for a weak correlation between global peak longitudinal six-segment strain and age ( P = .013, R 2 = 0.04; Figure 3 ).
Variable | Age (y) | ||||
---|---|---|---|---|---|
<1 | 1–4 | 5–9 | 10–14 | 15–19 | |
Global peak strain (%) | |||||
Longitudinal six-segment model | −20.3 ± 3.1 | −22.8 ± 2.4 | −21.9 ± 2.1 | −20.5 ± 2.2 | −19.5 ± 1.7 |
Longitudinal 12-segment model | −20.8 ± 3.2 | −23.5 ± 1.4 | −23.3 ± 2.3 | −21.8 ± 2.0 | −21.0 ± 1.8 |
Longitudinal 18-segment model | −20.6 ± 3.1 | −23.6 ± 1.5 | −23.1 ± 2.2 | −21.8 ± 2.1 | −20.8 ± 1.8 |
Radial | 40.5 ± 13.5 | 49.8 ± 13.7 | 55.0 ± 11.2 | 49.6 ± 14.8 | 38.1 ± 8.8 |
Circumferential | −21.7 ± 4.7 | −22.1 ± 3.6 | −23.1 ± 2.7 | −22.8 ± 3.6 | −21.6 ± 1.3 |
Time to global peak strain (msec) | |||||
Longitudinal six-segment model | 260 ± 23 | 325 ± 28 | 365 ± 28 | 396 ± 33 | 376 ± 35 |
Longitudinal 12-segment model | 262 ± 16 | 325 ± 26 | 358 ± 28 | 386 ± 27 | 368 ± 24 |
Longitudinal 18-segment model | 262 ± 13 | 326 ± 23 | 357 ± 27 | 387 ± 21 | 364 ± 16 |
Radial | 260 ± 37 | 323 ± 30 | 355 ± 24 | 382 ± 42 | 373 ± 46 |
Circumferential | 242 ± 23 | 311 ± 25 | 340 ± 26 | 364 ± 36 | 359 ± 41 |
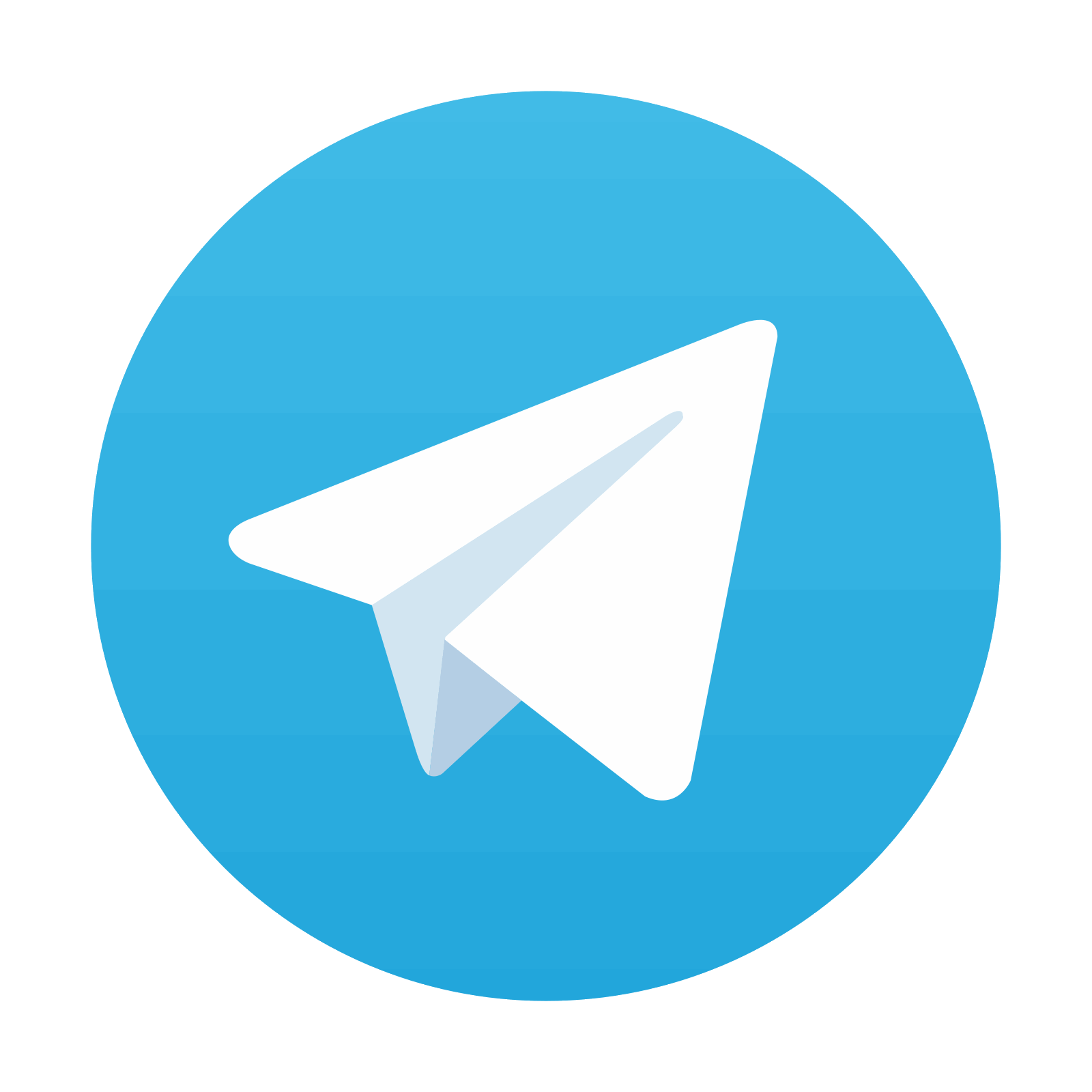
Stay updated, free articles. Join our Telegram channel

Full access? Get Clinical Tree
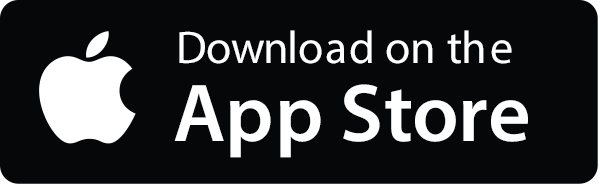

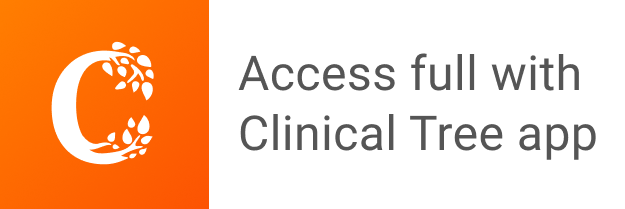