Heart failure (HF) is a major cause of mortality and morbidity in the United States that carries substantial healthcare costs. Multiple risk prediction models and strategies have been developed over the past 30 years with the aim of identifying those at high risk of developing HF and of implementing preventive therapies effectively. This review highlights recent developments in HF risk prediction tools including emerging risk factors, innovative risk prediction models, and novel screening strategies from artificial intelligence to biomarkers. These developments allow more accurate prediction, but their impact on clinical outcomes remains to be investigated. Implementation of these risk models in clinical practice is a considerable challenge, but HF risk prediction tools offer a promising opportunity to improve outcomes while maintaining value.
In the United States, heart failure (HF) is a major cause of mortality and morbidity. Approximately 6.7 million Americans have HF, with the prevalence projected to increase to 8 million within the next 10 years. Similarly, the lifetime risk for developing HF has significantly increased over the past 50 years.
Targeted HF prevention efforts have been overshadowed by a great emphasis on atherosclerotic cardiovascular disease (ASCVD) risk estimation and prevention. Although related, reframing HF risk in the context of ASCVD and other associated risk factors will allow a greater appreciation of the magnitude and the need for therapeutic urgency. Cost-effective HF prevention efforts require targeting subjects and populations at high risk given recent therapies (sodium-glucose transport protein 2 [SGLT2] inhibitors and glucagon-like peptide 1 [GLP1] receptor agonists) have been effective in reducing risk of developing HF in specific populations. Therefore, improving identification of subjects at risk for HF is critical for timeously initiating preventive therapies and maximizing efforts in precision medicine.
Optimizing traditional risk factors (including blood pressure, smoking, obesity, and diabetes management) has been the mainstay for HF prevention. To further understand a subject’s personal risk to target prevention efforts, multiple prediction models using various variables have been developed over the past several decades. Risk prediction models aim to provide prognostic information to influence clinical decisions and improve outcomes.
Recent guidelines, including the American Heart Association (AHA)/American College of Cardiology (ACC)/Heart Failure Society of America (HFSA) 2022 Heart Failure Guideline, strongly emphasize HF prevention, including using SGLT2 inhibitors for the primary prevention of HF in subjects with type 2 diabetes (class 1, strong recommendation), but also notably highlight the usefulness of validated multivariable risk scores for estimating incident HF risk (class 2a, moderate recommendation). , Further research and evidence are required to launch the routine application of HF risk prediction models. This review focuses on previous achievements and recent advancements in incident HF prediction.
Through population-based observational studies, major clinical risk factors for HF have been established with recognition of the breadth of HF etiologies. The AHA reflects modifiable risk factors associated with HF in their Life’s Essential 8, an advisory aimed to improve overall cardiovascular health. These risk factors, when uncontrolled and present together, were linked to marked increases in HF risk. Uncontrolled blood pressure, diabetes, and/or obesity were linked to almost 1/4 of HF cases in the Atherosclerosis Risk in Communities (ARIC) study population. In the AHA/ACC/HFSA 2022 Heart Failure Guideline, there were 3 class I recommendations for prevention of HF including (1) controlling blood pressure, (2) controlling diabetes, and (3) obesity management and other lifestyle recommendations.
Risk prediction models leverage established risk factors and additional variables to compute a subject’s risk based on population data. A systematic review of HF risk prediction models identified that age, systolic blood pressure, sex, left ventricular hypertrophy, and coronary artery disease were the most common variables in these models. Emerging risk markers such as albuminuria also have a strong prognostic association with incident HF, highlighting the interrelatedness of cardiovascular-kidney-metabolic (CKM) health. ,
The Framingham HF Risk Score (published in 1999) aimed to develop a risk profile to identify subjects at high risk for HF as part of the prospective Framingham Study. The 4-year likelihood of developing HF was calculated on the basis of a weighted risk score using sex, age, forced vital capacity, systolic blood pressure, heart rate, left ventricular hypertrophy on electrocardiogram (ECG), coronary artery disease, valve disease, diabetes, and cardiomegaly. One limitation, however, was that the Framingham cohort lacked diversity, with mostly White subjects with prevalent cardiovascular disease (CVD), which may underestimate the risk of an ethnically and socioeconomic diverse healthy population. However, this model provided the foundation for subsequent efforts including the Health ABC (Aging and Body Composition) HF Score, MESA (Multi-Ethnic Study of Atherosclerosis) Risk Score, ARIC Risk Score, and Pooled Cohort equations to Prevent HF (PCP-HF) ( Table 1 ).
Framingham Heart Failure Risk Score | Health ABC Heart Failure Score | ARIC Risk Score | MESA Risk Score | PCP-HF | |
---|---|---|---|---|---|
Published | 1999 | 2008 | 2012 | 2015 | 2019 |
Population | Participants in FHS with history of CAD, HTN, or valvular disease but without HF (n=6,354 Men, n=8913 Women) | Participants in Health ABC Study without baseline HF (n=2,935) | ARIC Study (n=13,555) | MESA Study (n=6,814), individuals free of clinical CVD | Participants free of CVD in 5 community-based cohorts (n = 11,771; ARIC, CARDIA, CHS, FOF, MESA) with external validation from PREVEND (n=6,699) and JHS (n=2,770) cohorts |
Age Range (mean ± SD) | 45 to 94 years (Men: 62 years, Women: 63.8 years) | 70 to 79 years (73.6 ± 2.9 years) | 45 to 64 years (With incident HF 56.6 years (n=1,487), without 53.8 years (n=12,068)) | 45 to 84 years (62.1 ± 10 years) | 30 to 80 years old (52 ± 12 years) |
Risk Factors | Age, SBP, LVH on ECG, Vital Capacity by spirometry, HR, CAD, Murmurs signifying Valve Disease, Diabetes, Radiographic cardiomegaly, and BMI | Age, History of CAD, Smoking Status, SBP, HR, Fasting glucose, Creatinine, Albumin levels, and LVH | Age, Sex, Diabetes, HTN, SBP, HR, BMI, Smoking Status | Age, Sex, Smoking Status, BMI, SBP, HR, Diabetes status (Fasting Glucose), NT pro-BNP | Sex, Race, Age, SBP, anti-HTN medication usage, BMI, Total Cholesterol, HDL, Smoking Status, Fasting Glucose, Diabetic Medication usage, and QRS duration |
Outcome | 4-Year Risk of Developing Congestive Heart Failure | 5-year risk of incident HF (defined as hospitalization for new onset HF) | 10-year risk of hospitalized HF | 5-year risk of incident HF | 10-year risk of incident HF |
The Health ABC Heart Failure Score was based on older patients (70 to 79 years old), which limits generalizability to a middle-aged population. The ARIC Risk Score incorporates the use of N-terminal pro b-type natriuretic peptide, which is not typically acquired in subjects without symptoms and without preexisting HF. More recently, the PCP-HF model was developed using individual-level data from 7 community-based cohorts (age range 30 to 80 years) to provide a race- and gender-specific 10-year risk equation for incident HF. The variables in PCP-HF include age, systolic blood pressure, antihypertensive medication usage, body mass index (BMI), total and high-density cholesterol levels (HDL), current smoking status, fasting glucose, diabetic medication usage, and electrocardiogram measurement of QRS duration. Previous systematic reviews and meta-analyses of HF risk scores have shown that the ARIC and PCP-HF risk scores have the highest discrimination for incident HF in the general population.
Multivariable risk scores and models for HF risk have also been established for specific populations at risk. WATCH-DM(Weight [BMI], Age, hyperTension, Creatinine, HDL-C, Diabetes control [fasting plasma glucose], QRS Duration, MI, and CABG) is a risk score model derived from machine learning to predict 5-year incident HF risk using data from 8,765 patients without HF from the Action to Control Cardiovascular Risk in Diabetes (ACCORD) trial. , WATCH-DM incorporates BMI, age, hypertension, creatinine, HDL, diabetes control, QRS duration, and history of myocardial infarction or coronary artery bypass graft surgery (CABG) into a 34-point maximum risk score. Each 1-unit increment increase in WATCH-DM represents a 24% increase in relative risk of developing HF in 5 years, with >14 points designating the “very high” HF risk group. This score was externally validated in datasets from the Antihypertensive and Lipid-Lowering Treatment to Prevent Heart Attack Trial (ALLHAT) and pooled community cohorts. As data generation becomes more readily available, applications of advanced methods including machine learning may allow us to potentially gain more insight in identifying subjects at risk.
Current clinical practices including the AHA/ACC ASCVD Risk Score estimate 10-year ASCVD risk, defined as coronary artery death or nonfatal myocardial infarction, or nonfatal/fatal stroke. Despite many overlapping risk factors, including HF risk has been a challenge. The AHA-sponsored Cardiovascular-Kidney-Metabolic (CKM) Science Advisory Group recently developed and validated the Predicting Risk of CVD EVENTs (PREVENT) equations to estimate a 10- and 30-year comprehensive CVD risk in adults aged 30 to 79 years without history of CVD. Importantly, the PREVENT risk score includes estimates of HF. The PREVENT equations use traditional risk factors (smoking status, blood pressure, cholesterol, antihypertensive use, statin use, diabetes, and kidney function). Optional predictors (urine albumin-to-creatine ratio, hemoglobin A1c, and social deprivation index) were also used to develop equations to predict each CVD subtype (ASCVD, HF). The social deprivation index incorporates measures of income, education, employment, housing, household characteristics, and transportation that have all been shown to be associated with cardiovascular risk.
The AHA launched an online PREVENT calculator ( https://professional.heart.org/en/guidelines-and-statements/prevent-calculator ) to be used in the primary prevention setting ( Figure 1 ). Its implementation and uptake in primary and prevention care and whether updates to the blood pressure and cholesterol guidelines should routinely use this new calculator rather than the ASCVD risk estimator remain to determined.
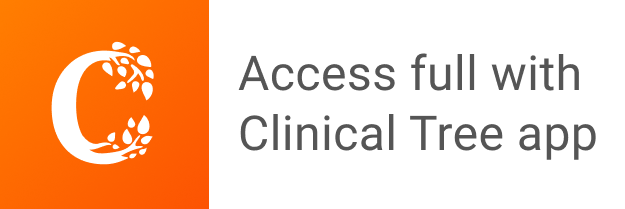