Objective
Risk stratification of patients presenting to the emergency department (ED) with suspected cardiac chest pain (CP) and an undifferentiated electrocardiogram (ECG) is difficult. We hypothesized that in these patients a risk score incorporating clinical, ECG, and myocardial contrast echocardiography (MCE) variables would accurately predict adverse events occurring within the next 48 hours.
Methods
Patients with CP lasting for 30 minutes or more who did not have ST-segment elevation on the ECG, were enrolled. Regional function (RF) and myocardial perfusion (MP) were assessed by MCE. A risk model was developed in the initial 1166 patients (cohort 1) and validated in subsequent 720 patients (cohort 2). Any abnormality or ST changes on ECG (odds ratio [OR] 2.5; 95% confidence interval [CI], 1.4-4.5, P = .002, and OR 2.9, 95% CI, 1.7-4.8, P < . 001, respectively), abnormal RF with normal MP (OR 3.5, 95% CI, 1.8-6.5, P < . 001), and abnormal RF with abnormal MP (OR 9.6, 95% CI, 5.8-16.0, P < . 001) were found to be significant multivariate predictors of nonfatal myocardial infarction or cardiac death.
Results
The estimate of the probability of concordance for the risk model was 0.82 for cohort 1 and 0.83 for cohort 2. The risk score in both cohorts stratified patients into 5 distinct risk groups with event rates ranging from 0.3% to 58%.
Conclusion
A simple predictive instrument has been developed from clinical, ECG, and MCE findings obtained at the bedside that can accurately predict events occurring within 48 hours in patients presenting to the ED with suspected cardiac CP and an ECG that is not diagnostic for acute ischemic injury. Its application could enhance care of patients with CP in the ED. For instance, patients with a risk score of 0 could be discharged from the ED without further workup. However, this needs to be validated in a multicenter study.
Despite technologic advances over the past few decades, patients who present to the emergency department (ED) with chest pain (CP) and an electrocardiogram (ECG) nondiagnostic for acute ischemic injury continue to remain a diagnostic challenge. Because of antiplatelet and other therapy, even patients who are experiencing an acute myocardial infarction (AMI) are now less likely to present to the ED with a diagnostic ECG. Moreover, patients with CP today are more likely to have unstable angina pectoris rather than an AMI.
Current management of such patients involves either admission to the hospital or observation in a CP unit in the ED, where the ECG is periodically repeated and blood samples are acquired to measure biomarkers that indicate myocardial injury. In the United States the majority of these approximately 5 million patients seen in the ED annually are discharged after myocardial injury is ruled out, which costs the nation approximately $10 billion annually.
We and others have previously demonstrated the value of echocardiography (without and with contrast) in the detection of AMI and other acute coronary syndromes (ACSs) in the ED, as well as the prognostic utility of this tool to predict long-term outcome. In this study we hypothesized that a predictive instrument could be developed from clinical, ECG, and myocardial contrast echocardiography (MCE) findings obtained at the bedside that would accurately predict events occurring within 48 hours in patients presenting to the ED with suspected cardiac CP and a nondiagnostic ECG. We developed the instrument from variables derived from a cohort of patients undergoing these tests and then tested its accuracy in a subsequent cohort who also underwent the same tests in the same ED.
Materials and Methods
Study Sample and Protocol
This prospective study was approved by the Human Investigation Committee. Patients presenting to the ED with CP not easily attributable to a noncardiac cause (eg, chest wall pain or pulmonary pathology) and who did not have ST-segment elevation on the ECG were approached for enrollment. The inclusion criteria were age > 30 years and CP lasting for at least 30 minutes and occurring within 12 hours of ED admission. Patients with a history of MI were not excluded from the study. All patients provided written informed consent. After the history was obtained and physical examination was performed by a cardiologist, ECG, blood samples, and MCE were obtained sequentially. ECG and blood samples were repeated every 6 hours as needed. MCE results (RF or MP) were not shared with the ED physician, who admitted or discharged the patient on the basis of routine criteria (clinical, ECG, and cardiac serum biomarkers).
The predictive instrument was developed in an initial cohort (cohort 1) and prospectively tested in a subsequent cohort (cohort 2) that was sequentially recruited. A different contrast agent (Definity; Lantheus Imaging, North Billerica, MA) was used in cohort 2 because the agent used in cohort 1 (Optison; GE Healthcare, Milwaukee, WI) was no longer available at that time.
Electrocardiography
The initial 12-lead ECG was interpreted by an observer blinded to all other information. It was classified as normal or abnormal. For the latter, it was further characterized as having ST-segment abnormality or T-wave inversion, left ventricular hypertrophy (with or without strain), conduction abnormality, and arrhythmia. Pathologic Q waves in 2 or more contiguous leads indicated prior MI.
Echocardiography
Echocardiography was performed using a Sonos 5500 system (Philips Ultrasound, Andover, MA). In cohort 1, 3 mL of Optison was diluted in 60 mL of normal saline and infused intravenously at a rate of 3 mL/min −1 using a model AS40A pump (Baxter Healthcare, Deerfield, IL). In cohort 2, 1.5 mL of Definity was diluted in 28.5 mL of normal saline and infused at a rate of 1.5 mL/min −1 using the same pump.
The infusion rate was adjusted to obtain homogeneous left ventricular cavity opacification with shadowing limited only to the left atrium in the apical views. Images were first acquired for regional function (RF) analysis in the apical and parasternal views using low (<0.3) mechanical index (MI). Myocardial perfusion (MP) images were then obtained using high (1.0) MI intermittent imaging (ultrasound transmission gated to end systole) with transmit/receive frequencies of 1.3/3.6 MHz. Ultrasound compression was set at 75%. If tissue signal was seen despite optimization of imaging settings, high MI intermittent power Doppler imaging was performed to eliminate the tissue signal. All settings were optimized at the beginning of the study and then held constant. Image acquisition was completed in < 10 minutes.
MP images were acquired in apical 2-, 3-, and 4-chamber views at pulsing intervals of 1, 2, 3, 4, and 5 cardiac cycles. The transmit focus was initially set at the level of the mitral valve but was adjusted to the apex if an apical defect was seen in order to discriminate between a true defect and an artifact. RF and MP data were stored separately on magneto-optical disks.
Image Interpretation
For cohort 1, RF and MP images were read separately by experienced observers blinded to all other information. These were scored as normal or abnormal using a 14-segment model covering the 3 coronary territories ( Figure 1 ). MP was scored as abnormal if maximal myocardial opacification was not seen within a segment by 5 cardiac cycles. RF was scored as 0 = normal, 1 = hypokinesia, 2 = akinesia, or 3 = dyskinesia. Studies were classified as normal if RF or MP in the visualized segments within every perfusion territory were normal. Studies were classified as abnormal if RF or MP was abnormal in 1 or more territories (usually multiple segments), even if all segments were not visualized. If a study could not be classified as above, it was deemed not assessable. For cohort 2, the MP study was evaluated immediately after interpretation of RF, and each of the 14 myocardial segments was scored as normal or abnormal on the basis of the combination of RF and MP results.

Statistical Methods
We used the “hard” events of nonfatal AMI and cardiac-related death occurring within 48 hours of ED admission for analysis. AMI was defined by an abnormal troponin I level (≥0.6 ng/mL −1 ). All patients who were admitted to hospital after enrollment were followed up in person before discharge, and their medical records were reviewed. Follow-up of discharged patients was obtained with a direct telephone call to each patient or the patient’s primary physician. All reported events were confirmed by review of medical records or death certificate.
Logistic regression was used to model the relationship between events and predictor variables in cohort 1. We first found the best model excluding MCE and then examined the added contribution made by MCE. To define the best model excluding MCE, all variables that had P values < .20 on univariate analyses were entered in a backward selection routine. Variables sequentially selected for removal were based on a significance level of .05. After this initial selection of variables, original variables not selected were entered one at a time to the backward selection model to determine significance in the presence of the other variables. Any variable significant at P < . 05 was then included in the final model. We used the same technique to find the best overall model including MCE.
The Pearson goodness-of-fit statistic was obtained to assess the fit of the model. The c-index, a measure of concordance between the predicted probabilities from the final logistic regression model and the observed responses, was calculated as an assessment of the predictive ability of the model. A bootstrap estimate of the c-index for the final model corrected for over-optimism was obtained using 1000 bootstraps. A c-index was then obtained for cohort 2 using the final model derived from cohort 1. In addition, a simple risk score, created using the logistic regression model from cohort 1, was validated in cohort 2. The contribution of a risk factor to the risk score for an individual was based on the regression coefficient for that risk factor. These coefficients were rounded to the nearest integer, so that a risk factor would contribute 0, 1, or 2 points to the summary score. Risk estimates were obtained as simple proportions, and the exact (Clopper–Pearson) confidence intervals (CIs) were used for binomial data.
Results
Patient Cohorts
A total of 2045 patients were studied. The first 1282 patients receiving Optison were assigned to cohort 1. Of these patients, MP imaging was not performed in 34 because of lack of feasibility (usually a technical problem, eg, interstitial infiltration of the intravenous fluid) and it was not interpretable in another 78 (80% of cases because of attenuation over the mid and basal lateral segments). Three additional patients had missing ECGs, and 1 patients was omitted from analysis because of noncardiac death. Those excluded from analysis were more likely to be male (67% vs 54%), to smoke (84% vs 66%), and to have hypercholesterolemia (63% vs 52%), diabetes (40% vs 28%), and coronary artery disease (CAD, 41% vs 28%). The latter was based on a patient’s reported history and not on review of coronary angiography. Patients had prior MI confirmed by EKG.
The second 763 patients receiving Definity were assigned to cohort 2. Of these patients, MP imaging was not performed in 22 because of lack of feasibility and was non-interpretable in 19. Two additional patients had missing ECGs. There were more male patients among those with missing data (72% vs 57%). No patient in either cohort had uninterpretable RF studies because of excellent endocardial border delineation.
Thus, 1166 patients from cohort 1 and 720 patients from cohort 2 form the basis of this report, and their baseline characteristics are listed in Table 1 . Cohort 1 patients had a higher incidence of hypercholesterolemia, family history of CAD, history of smoking, and abnormal RF on MCE, whereas the cohort 2 patients had a higher incidence of diabetes mellitus and an abnormal ECG.
Variables | Cohort 1 (n = 1166) | Cohort 2 (n = 720) | P value |
---|---|---|---|
Age ∗ | 59.8 ± 13.6 | 57.9 ± 13.3 | .003 |
Male (%) | 624 (54%) | 409 (57%) | .163 |
African-American (%) | 267 (23%) | 168 (23%) | .828 |
History of hypertension (%) | 756 (65%) | 437 (61%) | .070 |
History of hypercholesterolemia † | 607 (52%) | 290 (40%) | <.001 |
History of diabetes mellitus | 324 (28%) | 280 (39%) | <.001 |
Family history of CAD † | 546 (47%) | 290 (40%) | .006 |
History of smoking (%) † | 768 (66%) | 327 (45%) | <.001 |
Documented CAD | 328 (28%) | 233 (32%) | .052 |
Prior myocardial infarction | 88 (10%) | 22 (7%) | .078 |
Abnormal ECG (%) | 716 (61%) | 501 (70%) | <.001 |
ST-T changes on ECG (%) | 99 (8%) | 69 (10%) | .420 |
RF and MP | <.001 | ||
Abnormal RF and abnormal MP | 315 (27%) | 128 (18%) | |
Abnormal RF and normal MP | 172 (15%) | 57 (8%) | |
Normal RF and normal MP | 679 (58%) | 535 (74%) |
∗ Expressed as mean ± standard deviation.
Patient Outcomes and Development of Risk Model
Of the 1166 cohort 1 patients, 142 (12%) had an event (138 had AMI, 4 had AMI followed by death). In these patients, the initial troponin was 0.8 ± 52 (<0.08-470) and the peak troponin was 34 ± 71 (0.61-470). Univariate predictors that were considered for incorporation into the risk model included age, gender, the presence of cardiac risk factors (hypertension, hypercholesterolemia, diabetes, family history of early CAD, current smoking, or history of smoking), race (African American vs all others), ST-T changes on ECG, any abnormality on ECG, abnormal RF, and abnormal MP. When excluding MCE, our selection procedure produced a model that included diabetes status ( P = .011), gender ( P = .004), ST-T changes on ECG ( P < .001), and any abnormality on ECG ( P < .001). We then included MCE to obtain its incremental utility. The Pearson goodness-of-fit statistic for this model yielded a P value of .48, suggesting a reasonable fit.
Table 2 shows the odds ratios (ORs) along with the regression coefficients associated with each variable in this logistic regression model for cohort 1. In particular, adding MCE to the model resulted in a significant incremental contribution ( P < . 001). Abnormal RF and MP were both significant predictors of outcome. When both were abnormal, the odds of having an event was 9.0 times greater than when both were normal (95% CI, 5.4-14.9; P < . 001). Other significant multivariate predictors of events included the presence of ST-T changes on ECG (not ST elevation, because patients with this finding were not included in the study), having any abnormality on the ECG, and the presence of abnormal RF even when MP was normal. The c-index for prediction of adverse outcome based on gender and diabetes was 0.61. When including gender, diabetes, ST-T changes, and any abnormality on ECG, the c-index was significantly higher (0.74, P < . 001). With the addition of MCE, the c-index increased further (0.83, P < . 001). The optimism of this estimator based on results from 1000 bootstrap samples using this final model was only 0.006, and thus the bootstrap optimism-corrected estimate of the measure of concordance was also 0.82. When the nonsignificant variables, diabetes and gender, were removed from this model, the c-index remained at 0.82 and the remaining ORs changed only slightly and remained highly significant ( Table 3 ). This simpler model can also be obtained via backward selection with all candidate variables (including MCE) used in the first step. We focused on this more parsimonious model for risk prediction and validation.
Predictors | OR | 95% CI | Regression coefficient | P value |
---|---|---|---|---|
Abnormal ECG relative to normal ECG | 2.4 | (1.4-4.3) | 0.9 | .003 |
ST-T changes relative to no ST-T changes | 2.9 | (1.7-4.8) | 1.1 | <.001 |
RF abnormal but MP normal relative to both normal | 3.4 | (1.8-6.4) | 1.2 | <.001 |
RF and MP abnormal relative to both normal | 9.0 | (5.4-14.9) | 2.2 | <.001 |
Diabetic relative to nondiabetic | 1.31 | (0.9-2.0) | 0.1 | .190 |
Female relative to male | 0.72 | (0.5-1.1) | -0.2 | .119 |
Predictors | OR | 95% CI | Regression coefficient | P value |
---|---|---|---|---|
Abnormal ECG relative to normal ECG | 2.5 | (1.4–4.4) | 0.9 | .002 |
ST-T changes relative to no ST-T changes | 2.9 | (1.7–4.8) | 1.1 | <.001 |
RF abnormal but MP normal relative to both normal | 3.5 | (1.8–6.6) | 1.2 | <.001 |
RF and MP abnormal relative to both normal | 9.7 | (5.8–16.0) | 2.3 | <.001 |
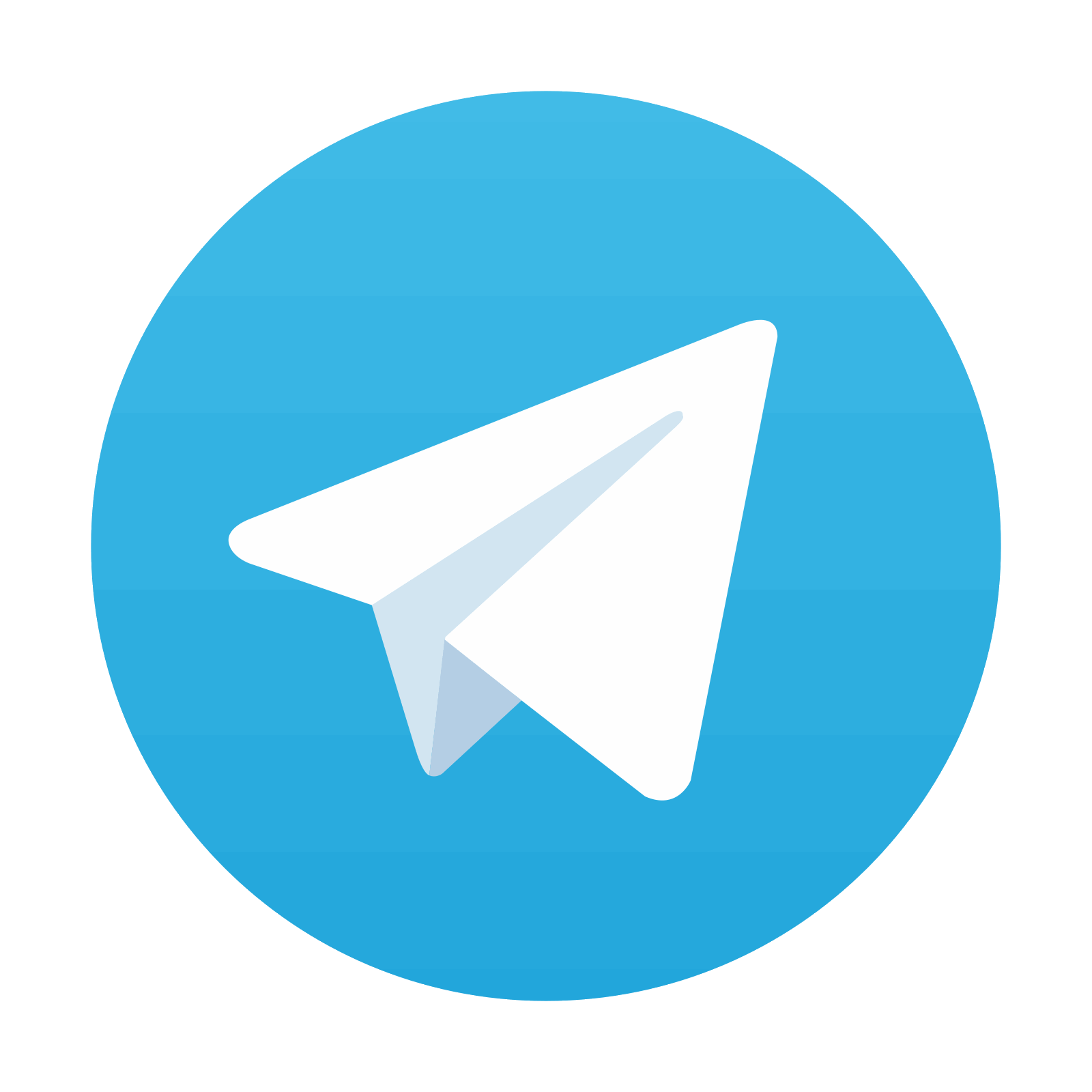
Stay updated, free articles. Join our Telegram channel

Full access? Get Clinical Tree
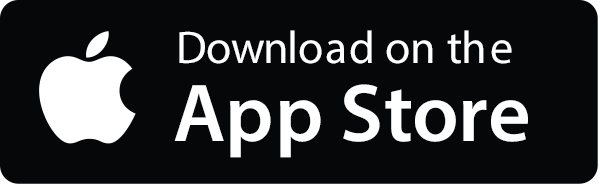

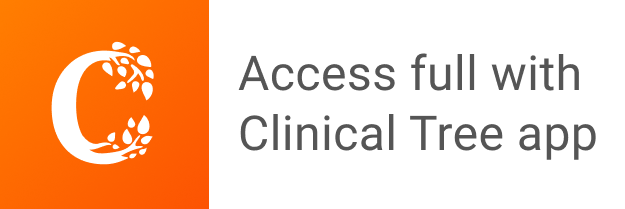