Highlights
- •
A new integrated risk tool combines clinical and polygenic risk scores.
- •
Prediction is significantly enhanced in multiple ethnicities and ancestries.
- •
Ethnicities include Black and White, ancestries include African and European.
- •
This is the first positive cross-ethnicity cross-ancestry validation of such a tool.
The American College of Cardiology / American Heart Association pooled cohort equations tool (ASCVD-PCE) is currently recommended to assess 10-year risk for atherosclerotic cardiovascular disease (ASCVD). ASCVD-PCE does not currently include genetic risk factors. Polygenic risk scores (PRSs) have been shown to offer a powerful new approach to measuring genetic risk for common diseases, including ASCVD, and to enhance risk prediction when combined with ASCVD-PCE. Most work to date, including the assessment of tools, has focused on performance in individuals of European ancestries. Here we present evidence for the clinical validation of a new integrated risk tool (IRT), ASCVD-IRT, which combines ASCVD-PCE with PRS to predict 10-year risk of ASCVD across diverse ethnicity and ancestry groups. We demonstrate improved predictive performance of ASCVD-IRT over ASCVD-PCE, not only in individuals of self-reported White ethnicities (net reclassification improvement [NRI]; with 95% confidence interval = 2.7% [1.1 to 4.2]) but also Black / African American / Black Caribbean / Black African (NRI = 2.5% [0.6–4.3]) and South Asian (Indian, Bangladeshi or Pakistani) ethnicities (NRI = 8.7% [3.1 to 14.4]). NRI confidence intervals were wider and included zero for ethnicities with smaller sample sizes, including Hispanic (NRI = 7.5% [−1.4 to 16.5]), but PRS effect sizes in these ethnicities were significant and of comparable size to those seen in individuals of White ethnicities. Comparable results were obtained when individuals were analyzed by genetically inferred ancestry. Together, these results validate the performance of ASCVD-IRT in multiple ethnicities and ancestries, and favor their generalization to all ethnicities and ancestries.
Introduction
Current US guidelines for the primary prevention of cardiovascular disease are based on the quantification of an individual’s predicted risk of atherosclerotic cardiovascular disease (ASCVD) over the following 10 years using the ASCVD pooled cohort equations tool (ASCVD-PCE). The tool combines information from multiple clinical risk factors including age, sex, blood lipid levels, blood pressure, history of diabetes, smoking or anti-hypertensive treatment, and racial identity (entered as “White,” “African American” or “Other,” where “Other” is treated algorithmically as “White”). Although genetics is a known risk factor, it is not directly included in ASCVD-PCE (family history of ASCVD is assessed separately, outside of the tool). Polygenic risk scores (PRSs), which combine information across thousands of common genetic variants in the human genome, can be added to the ASCVD-PCE tool, but previous studies have reported variable predictive performance. Additionally, these studies focused primarily on individuals with European ancestries, but it is known that the predictive accuracy of a PRS tends to attenuate in individuals with non-European ancestries. We therefore undertook a clinical validation study of a new 10-year ASCVD risk prediction tool (ASCVD-IRT) that integrates a PRS for ASCVD with the established ASCVD-PCE tool, paying particular attention to its predictive performance in non-European ancestries and non-White ethnicities.
Methods
Following recent guidelines on the use and reporting of race, ethnicity and ancestry, we clarify that in this study we use the term “ethnicity” to refer to social categories including both race and ethnicity, and we do not distinguish “race” from “ethnicity.” In the testing cohorts described below, data on ethnicity were collected from questionnaire, census and other self-identification data, allowing us to infer that ethnicity was self-reported. We use the term “ancestry” or “genetic ancestry” to refer to inferences from genetic data. We note that the concepts of ethnicity and ancestry are correlated but not synonymous. To infer ancestry, we used a method based on principal components derived from genetic data to infer membership to one of 5 high-level ancestry groups conforming to those used by the 1000 Genomes Project (Sub-Saharan African [AFR], Native/Indigenous American [AMR], East Asian [EAS], European [EUR], and South Asian [SAS]; see Supplementary Materials for details, OTHER indicates mixed inferred ancestry). We note that this method is more accurately described as producing “ancestry-like” genetic similarity relationships. We present performance evaluations for both ethnicity and ancestry groups, as the former relate to important social categories while the latter allow us to examine the ancestry-specific PRS attenuation issue and its effect on IRT performance.
We tested the performance of the ASCVD-IRT using data from the Atherosclerosis Risk in Communities (ARIC) cohort, the Multi-Ethnic Study of Atherosclerosis (MESA), and UK Biobank (UKB). All individuals in these studies gave informed consent. Legal and ethical approval for our use of ARIC and MESA data is provided by the Western Institutional Review Board (Study Number 1264897, IRB Tracking Number 20192201). See Supplementary Materials for UKB approval information.
All 3 cohorts are prospective, contain participants that were extensively examined at baseline, and have continuing follow-up (via annual phone calls for ARIC and MESA, via linkage to national electronic healthcare and death records for UKB). ARIC comprises over 15,000 adults from predominantly 2 study-defined racial/ethnic groups (“Black” and “White”), from defined populations in 4 sites in the USA, aged 45 to 64 years when recruited between 1987 and 1989. MESA comprises over 6,000 adults from 4 study-defined racial/ethnic groups (“African American,” “Chinese American,” “Hispanic,” and “White/Caucasian”), recruited primarily via phone call invitation to 6 sites in the USA, aged 45 to 84 years and free of cardiovascular disease when recruited between 2000 and 2002. , UKB comprises over 500,000 adults, recruited via postal invitation to 22 sites in the UK, aged 40 to 69 when recruited between 2006 and 2010. , We carefully selected 88,666 UKB individuals from multiple ethnicities (labels defined from questionnaire data) for the “IRT testing” subgroup, in order to ensure that the IRT testing subgroup was maximally enriched for incident ASCVD cases from non-European ethnicities and ancestries, and to ensure the independence of these individuals from other UKB subgroups that were used to construct and train the IRT (see Supplementary Materials for further details).
We excluded individuals with cardiovascular disease or on cholesterol lowering medication at baseline, and also those related to others in the cohort at greater than third degree relative level according to the genetic inference method described in Bycroft et al. A separate bridging analysis was performed on individuals free of cardiovascular disease who were on cholesterol lowering medication at baseline, which indicated similar performance for this subgroup ( Supplementary Figure 1 ). White participants in MESA and ARIC were excluded from testing due to sample overlap with GWASs used to construct the ASCVD PRS (MEGASTROKE_EUR and CARDIoGRAMplusC4D respectively – see Supplementary Table 1 ). Black ARIC individuals were included, but it should be noted that they also formed part of the cohort data used to train the ASCVD-PCE model (outside of this study). It may therefore be expected that the absolute prediction performance of both ASCVD-PCE and ASCVD-IRT is somewhat elevated in this group. However, our validation focused on the comparative performance of these 2 tools, which is not expected to be biased. All individuals in our testing cohorts were selected so as to be independent and unrelated to any individuals used in the training of the PRS or the IRT model.
To define ASCVD cases, we used outcomes that closely matched the ASCVD-PCE tool. In MESA we used “CARDIOVASCULAR DISEASE (CVD), HARD,” and in ARIC we used a union of “MI (myocardial infarction), heart attack, or fatal CHD (coronary heart disease) by December 31, 2004” and “Definite or probable ischemic incident stroke by December 31, 2004.” The ASCVD definition for UK Biobank is described in Supplementary Materials .
The ASCVD-IRT tool is a function of the score obtained from the currently established ASCVD-PCE tool and a PRS for ASCVD, trained from multiple datasets that each represent individuals from multiple ancestry groups and from different geographies. Ten GWAS datasets for different ASCVD subtraits were meta-analyzed to derive the PRS, and an additional 4 cohorts were used to train the PRS effect size. Further details regarding the construction of ASCVD-IRT are provided in Supplementary Materials .
We assessed predictive performance of ASCVD-IRT via relative performance comparisons to ASCVD-PCE, focusing in particular on differences in sensitivity and specificity and on the sum of these differences, also known as the Net Reclassification Improvement. We used ASCVD events in the following 10 years to define cases and we used scores above and below a certain risk threshold to define positive and negative results. This relative performance approach is justified because ASCVD-PCE provides a strong basis for comparison, being the currently established and recommended tool for 10-year ASCVD risk prediction in the US. We note that absolute values of sensitivity, specificity, positive predictive value (PPV) and negative predictive value (NPV) are difficult to interpret on their own. ASCVD-IRT and ASCVD-PCE are not diagnostic tests but risk predictors of an uncertain future event, where even under the best of conditions a “positive” result has a measured probability of being a noncase and a “negative” result has a measured probability of being a case. For example, we label an individual with a risk of 8% a “positive” result, but they only have an 8% chance of becoming an ASCVD case, assuming the model is correct. But while absolute values are difficult to interpret, relative improvements remain good indicators of performance improvements. In particular, simultaneous improvements in both sensitivity and specificity are strongly indicative of superior test performance.
There is also a logical choice for which threshold to use for binary compartmentalization into “positive” (high-risk) and “negative” (low-risk) results, as required for metrics such as sensitivity and specificity. The same guidelines recommending ASCVD-PCE for risk prediction also lay out recommendations for actionable risk thresholds above which intervention is advised. This threshold is 7.5% risk over 10 years for most individuals, but is reduced to 5% for individuals presenting with additional risk factors, one of which is South Asian ethnicity. , We therefore applied the same thresholds for our clinical performance assessment, applying the 5% threshold for individuals of South Asian ethnicities or ancestries and 7.5% for all others.
Further details on the statistical methods used to calculate performance metrics and their confidence intervals are provided in Supplementary Materials .
Results
Tables 1 and 2 show the subgroup sample sizes, after exclusions, in each IRT testing cohort. Figure 1 and Supplementary Table 2 summarize the relative performance improvements of ASCVD-IRT over the currently established tool (ASCVD-PCE). When meta-analyzed across all testing cohorts, ASCVD-IRT shows significantly improved performance (as measured by 95% CI) across NRI, sensitivity, and specificity (combined results [with 95% CI]: NRI = 3.0% [1.7 to 4.3]; delta-sensitivity [equivalent to NRI in cases] = 1.5% [0.2 to 2.8]; delta-specificity [equivalent to NRI in noncases] = 1.5% [1.3 to 1.7]). Positive predictive value (PPV) and negative predictive value (NPV) were also significantly improved ( Supplementary Table 2 ). The NRI is also significantly positive in all 3 cohorts, with the largest point estimates seen in the 2 US cohorts ( Figure 1 a, Supplementary Table 2 ). The within-cohort point estimates for changes in sensitivity, specificity, log(PPV), log(NPV) and Harrell’s C are also all positive, albeit in some cases with 95% confidence intervals that are large and overlap zero ( Figure 1 b-c, Supplementary Table 2 ).
Cohort | Self-reported ethnicity | Age at recruitment | Women | Men | ||
---|---|---|---|---|---|---|
Cases | Noncases | Cases | Noncases | |||
ARIC | Black | 40-54 | 47 (2.4%) | 762 (39%) | 38 (1.9%) | 422 (21%) |
55-69 | 40 (2.0%) | 383 (19%) | 40 (2.0%) | 233 (12%) | ||
MESA | Chinese American | 40-54 | 0 (0.0%) | 0 (0.0%) | 0 (0.0%) | 0 (0.0%) |
55-69 | 0 (0.0%) | 1 (0.1%) | 1 (0.1%) | 3 (0.2%) | ||
70-79 | 0 (0.0%) | 0 (0.0%) | 0 (0.0%) | 0 (0.0%) | ||
African American | 40-54 | 4 (0.2%) | 191 (9.9%) | 8 (0.4%) | 154 (8.0%) | |
55-69 | 10 (0.5%) | 252 (13%) | 20 (1.0%) | 216 (11%) | ||
70-79 | 15 (0.8%) | 102 (5.3%) | 16 (0.8%) | 109 (5.7%) | ||
Hispanic | 40-54 | 2 (0.1%) | 134 (7.0%) | 1 (0.1%) | 146 (7.6%) | |
55-69 | 10 (0.5%) | 159 (8.3%) | 25 (1.3%) | 186 (9.7%) | ||
70-79 | 8 (0.4%) | 59 (3.1%) | 9 (0.5%) | 84 (4.4%) | ||
UKB (IRT testing) | White | 40-54 | 135 (0.2%) | 18191 (21%) | 308 (0.4%) | 14167 (16%) |
55-69 | 588 (0.7%) | 25162 (28%) | 1048 (1.2%) | 18098 (20%) | ||
Indian, Bangladeshi or Pakistani | 40-54 | 12 (0.01%) | 1211 (1.4%) | 53 (0.1%) | 1136 (1.3%) | |
55-69 | 17 (0.02%) | 652 (0.7%) | 54 (0.1%) | 553 (0.6%) | ||
Black Caribbean or Black African | 40-54 | 14 (0.02%) | 1628 (1.8%) | 17 (0.02%) | 1194 (1.4%) | |
55-69 | 16 (0.02%) | 633 (0.7%) | 18 (0.02%) | 415 (0.5%) | ||
Chinese | 40-54 | 1 (0.001%) | 390 (0.4%) | 0 (0.0%) | 233 (0.3%) | |
55-69 | 4 (0.01%) | 238 (0.3%) | 1 (0.001%) | 112 (0.1%) | ||
Other | 40-54 | 10 (0.01%) | 948 (1.1%) | 8 (0.01%) | 624 (0.7%) | |
55-69 | 13 (0.01%) | 501 (0.6%) | 14 (0.02%) | 249 (0.3%) |
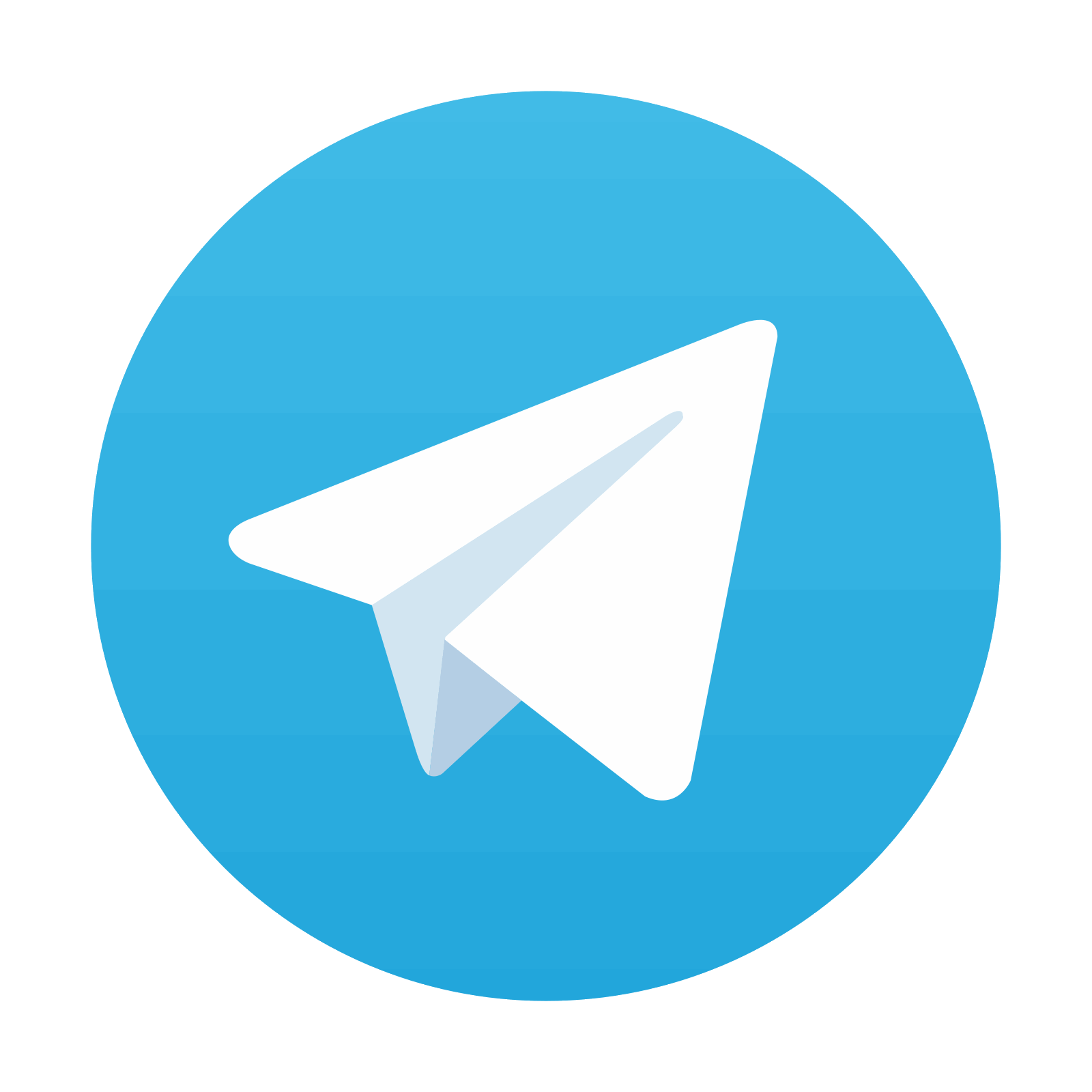
Stay updated, free articles. Join our Telegram channel

Full access? Get Clinical Tree
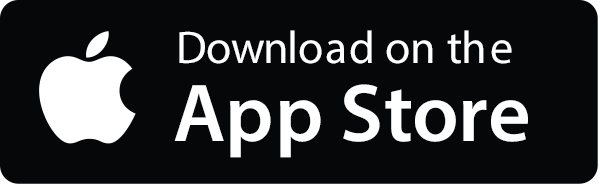

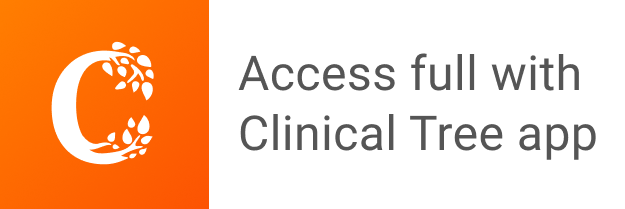