HIGHLIGHTS
- •
The rate of cardiac rhythm monitoring at 6 months after stroke is just over one-third.
- •
Only 30% of patients with cryptogenic strokes receive implantable loop recorders.
- •
Monitor utilization after stroke is not related to estimated atrial fibrillation risk.
GRAPHICS ABSTRACT

We characterized monitor utilization in stroke survivors and assessed associations with underlying clinical atrial fibrillation (AF) risk. We retrospectively analyzed consecutive patients with acute ischemic stroke 10/2018-6/2019 without prevalent AF and assessed the 6-month incidence of monitor utilization (Holter/ECG, event/patch, implantable loop recorder [ILR]) using Fine-Gray models accounting for the competing risk of death. We assessed for predictors of monitor utilization using cause-specific hazards regression adjusted for the Cohorts for Heart and Aging Research in Genomic Epidemiology AF (CHARGE-AF) score, stroke subtype, and discharge disposition. Of 493 patients with acute ischemic stroke (age 65±16; 47% women), the 6-month incidence of monitor utilization was 36.5% (95% CI 31.7, 41.3), and 6-month mortality was 13.6% (10.4, 16.8). Monitoring was performed with Holter/event (n = 107; 72.3%), ILR (n = 34; 23.0%) or both (n = 7; 4.7%). Monitoring was more likely after cryptogenic (hazard ratio [HR] 4.53 [3.22, 6.39]; 6-month monitor incidence 70.6%) and cardioembolic (HR 2.43 [1.28, 4.62]; incidence 47.7%) stroke, versus other/undocumented (incidence 22.7%). Among patients with cryptogenic stroke, the 6-month incidence of ILR was 27.5% [18.5, 36.5]. Monitoring was more likely after discharge home (HR 1.80 [1.29, 2.52]; incidence 46.1%) versus facility (incidence 24.9%). Monitoring was not associated with CHARGE-AF score (HR 1.08 per 1-SD increase [0.91, 1.27]), even though CHARGE-AF was associated with incident AF (HR 1.56 [1.03, 2.35]). In conclusion, rhythm monitors are utilized after one-third of ischemic strokes. Monitoring is more frequent after cryptogenic strokes, though ILR use is low. Monitor utilization is not associated with AF risk.
Atrial fibrillation (AF) is a well-recognized risk factor for ischemic stroke, and AF-related strokes are typically severe. , Recognizing AF as the underlying mechanism of stroke is important, as oral anticoagulation substantially reduces risk of recurrent stroke. AF is frequently subclinical, however, and detected only after extended rhythm monitoring. As a result, current guidelines provide a Class IIa recommendation (moderate strength and good quality evidence) for extended rhythm monitoring (“approximately 30 days”) within 6 months following cryptogenic stroke (i.e., strokes with no apparent mechanism). However, rhythm monitoring is costly, can be invasive, and most patients who receive monitoring ultimately do not have AF. Furthermore, limited data suggest that ambulatory monitors are utilized in only a minority of patients after stroke, , and the effect of factors such as AF-related co-morbidity or stroke subtype on monitor use remain unclear. A better understanding of after-stroke monitor utilization and its determinants may therefore identify opportunities to optimize monitoring yield and appropriateness. In this study, we retrospectively assessed monitor utilization within consecutive patients presenting to a single large tertiary care hospital with acute ischemic stroke and no previous history of AF. We quantified overall monitor utilization and predictors of monitor deployment, including stroke subtype and co-morbidities predictive of AF.
Methods
The data supporting the current study contains protected health information and cannot be shared publicly. Relevant data processing scripts will be made available upon reasonable request to the corresponding author.
We retrospectively analyzed consecutive patients discharged from the stroke service of Massachusetts General Hospital, a tertiary referral center serving the New England region of the United States, following hospitalization for acute ischemic stroke between October 2018 and June 2019. Given our intent to characterize monitor utilization to detect undiagnosed AF, we excluded patients with prevalent AF or AF first detected during their hospitalization. To assess for heterogeneity in monitor utilization by provider, as well as the association between clinical risk of AF and rhythm monitoring, we also excluded patients whose hospitalizations spanned transitions between stroke service attending physicians, and patients with incomplete data for calculation of the CHARGE-AF score. Patient flow through the study is depicted in Figure 1 . This study was approved the local Mass General Brigham Institutional Review Board.

AF-related clinical factors were ascertained after discharge from the index stroke admission by clinical abstractors. Age, gender, and self-reported race were obtained from the electronic health record (EHR) demographic data. Self-reported ethnicity was not collected. Height, weight, systolic and diastolic blood pressure were obtained from initial neurological exam documentation. If weight was not documented, we accepted any weight in the EHR within 1 year of stroke. If height was not documented, we accepted any height in the EHR. The presence of smoking (current or not current), chronic hypertension, diabetes, history of myocardial infarction, and history of heart failure were obtained from the initial neurological history and physical exam. Discharge disposition (home vs rehabilitation facility) was obtained from the index discharge summary.
We estimated AF risk using the linear predictor of the Cohorts for Heart and Aging Research in Genomic Epidemiology AF (CHARGE-AF) score, a well-validated AF prediction instrument. For the purposes of calculating CHARGE-AF, the coefficient associated with white race was applied to patients of self-reported white race and not to patients of other races. , Score components and weights are shown in Supplementary Table 1 . Since many anti-hypertensive agents have multiple indications, we substituted the diagnosis of hypertension for anti-hypertensive medication use in the CHARGE-AF score, as performed previously. Given that the calibration of predicted AF risk estimates obtained using CHARGE-AF has not been assessed in an after-stroke setting, we did not attempt to convert CHARGE-AF to predicted absolute AF risk.
All patients were admitted to a dedicated stroke unit and underwent routine assessment for ischemic stroke etiology including continuous telemetry monitoring and electrocardiograms. Stroke subtypes were assigned in accordance with the classification schema utilized by the American Heart Association Get with the Guidelines Stroke initiative by clinical abstractors who manually reviewed physician notes available at the time of discharge from the index stroke hospitalization to extract the treating neurologist’s clinical impression of stroke subtype (see the Supplementary Appendix ). We collapsed the outlined ischemic stroke classification schema into 3 parent categories: cardioembolic, cryptogenic, and other/undocumented.
The primary outcome was cardiac monitor utilization at 6 months from stroke admission, defined as any of the following: (1) 24-48 hour Holter/ECG monitor, (2) 2-4 week event/patch monitor, or (3) implantable loop recorder (ILR). We utilized a 6-month timeframe for the primary outcome given current guidelines recommending extended monitoring within 6 months for patients with cryptogenic stroke, and assessed other timeframes in secondary analyses. We accounted for death as a competing risk given the mortality associated with acute ischemic stroke. Secondary outcomes included monitor utilization at 3 months and 12 months. In exploratory analyses, we also assessed incident AF at 12 months and recurrent stroke at 12 months. Monitor utilization, mortality, incident AF, and recurrent stroke were adjudicated by manual review of EHR data spanning from stroke admission to death or last encounter (defined as any inpatient encounter or outpatient office visit) by 3 clinical adjudicators blinded to stroke subtype and clinical AF risk. The median follow-up time in the EHR from stroke admission to death or last encounter was 5.1 months (quartile 1: 0.53, quartile 3: 8.7).
In all survival analyses, follow-up time began at stroke admission and ended at a monitoring event, death, or last encounter. To assess for associations between clinical factors and 6-month monitor utilization, we fit multivariable Cox proportional hazards models with death treated as a censoring event and CHARGE-AF score, stroke subtype, and discharge disposition as covariates. We assessed for potential non-linearity in the association between the CHARGE-AF score and monitor utilization using fractional polynomials. Given no evidence of non-linearity, we utilized the CHARGE-AF score as a linear variable in all models. The proportional hazards assumption was verified by inspecting scaled Schoenfeld residuals. We assessed heterogeneity in monitor utilization across providers by calculating the intra-class correlation coefficient and standard deviation (SD) of monitoring incidence across providers.
We estimated the cumulative incidence of any monitoring with treatment of death as a competing risk. We plotted the 6-month crude cumulative incidence of monitoring stratified by discharge disposition and stroke subtype, and the 6-month crude cumulative incidence of monitoring stratified by tertile of CHARGE-AF score. To estimate monitoring incidence across strata of interest while accounting for the effects of clinical factors, we used Fine-Gray models to predict stratum-specific incidence estimates for an average profile of other covariates included in our multivariable model.
In secondary analyses, we plotted the cumulative incidence of ILR use and fit additional Cox proportional hazards models with ILR utilization as the outcome of interest. To assess the association between covariates and the cumulative incidence of monitoring, we also conducted a subdistribution hazards analysis by fitting a Fine-Gray model with the same predictors. Temporal trends of monitoring were evaluated by fitting additional Cox proportional hazards models including (1) month of discharge, and (2) quarter of discharge, and assessing whether models including calendar time had improved model fit as compared with models not including calendar time, using the likelihood ratio test. To assess whether AF risk factors were associated with incident AF or recurrent stroke, we fit Cox proportional hazards models with (1) incident AF, and (2) recurrent stroke, as outcomes of interest and CHARGE-AF score as the sole predictor.
We considered a 2-sided p value <0.05 to indicate statistical significance. All analyses were performed using R v3.6 and SAS v9.4 (SAS Institute, Cary North Carolina).
Results
Of 710 patients discharged after acute ischemic stroke during the study period, 149 (21.0%) had a previous diagnosis of AF, 39 (5.5%) developed incident AF prior to discharge, 19 (4.1%) were seen by multiple providers and 10 (1.4%) had inadequate data for AF risk estimation, resulting in 493 patients in the primary analysis ( Figure 1 ). The mean age (±SD) was 65±16 years and 47.5% were female. Other baseline characteristics are listed in Table 1 . Characteristics of patients meeting exclusion criteria are shown in Supplementary Table 2 .
Variable (N = 493) | Mean ± standard deviation or N (%) |
---|---|
Age (years) | 64.5 ± 16 |
Female | 234 (48%) |
White | 354 (72%) |
Black | 119 (24%) |
Asian | 20 (4%) |
Active smoker | 86 (17%) |
Height (cm) | 167 ± 12 |
Weight (kg) | 80 ± 39 |
Systolic blood pressure (mmHg) | 157 ± 32 |
Diastolic blood pressure (mmHg) | 83 ± 17 |
Diabetes mellitus | 115 (23%) |
Heart failure | 23 (5%) |
CHARGE-AF | 12.8 ± 1.9 |
Stroke mechanism | |
Cardioembolic | 29 (6%) |
Cryptogenic | 130 (26%) |
Other/undocumented | 334 (68%) |
At 6 months, the cumulative incidence of any monitoring was 36.5% (95% CI 31.7, 41.3) and the cumulative incidence of death was 13.6% (95% CI 10.4, 16.8) over median follow-up 1 month (interquartile range 0.2-5.9). Of the monitors utilized, the vast majority were Holter/patch/event monitor (n = 107; 72%), followed by ILR (n = 34; 23%) or both (n = 7; 5%). In multivariable Cox regression, monitor utilization was higher for cryptogenic and cardioembolic stroke subtypes (vs other/undocumented), and higher among patients discharged home (vs facility). There was no significant association between CHARGE-AF score and monitor utilization ( Table 2 ). Association results were similar using the Fine-Gray model ( Supplementary Table 3 ). Across the 11 stroke providers, the variation in monitor utilization was not explained by variation between providers as opposed to variation between patients within providers (intra-class correlation coefficient 0.01; SD of monitoring incidence across providers 6%).
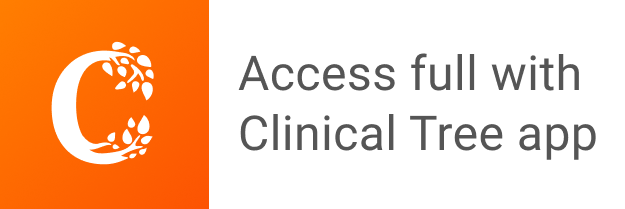