Although hypochloremia is strongly associated with adverse prognosis in acute heart failure (AHF), it is unknown whether incorporating hypochloremia into the preexisting risk model improves the model performance. We calculated the Get With The Guidelines–Heart Failure (GWTG-HF) risk score in 1,428 patients with AHF (derivation cohort) and developed 2 risk scores incorporating brain natriuretic peptide (BNP) into the GWTG-HF risk score (GWTG-BNP risk score) and incorporating both BNP and hypochloremia (GWTG-BNP-Cl risk score). Hypochloremia was defined as <98 mmol/L. The external validation and comparison of model performance were performed in an independent group of 1,256 patients with AHF (validation cohort). All models were tested for in-hospital mortality. Hypochloremia was observed in 9.4% and 12.2% of the derivation and validation cohorts, respectively. Hypochloremia was an independent predictor of in-hospital mortality in the derivation cohort (odds ratio 2.02; p = 0.028). In the validation cohort, the GWTG-HF, GWTG-BNP, and GWTG-BNP-Cl risk scores demonstrated good discrimination (area under the curve: 0.742, 0.749, and 0.763, respectively). However, the GWTG-BNP-Cl risk score was more reliable than the GWTG-HF and GWTG-BNP risk scores in risk reclassification (net reclassification improvement: 0.491 and 0.408, respectively; p <0.01 for both). Moreover, this score demonstrated a good calibration of the GWTG-BNP-Cl model (Hosmer-Lemeshow test: p = 0.479). In conclusion, incorporating hypochloremia into the preexisting risk model improves the model performance.
As the number of patients with heart failure is increasing because of the aging society, , acute decompensation of heart failure is one of the main reasons for the increase in hospital resource use and healthcare costs. Appropriate acute heart failure (AHF) risk stratification models are important, as they may improve patient triage and reduce healthcare costs. The Get With The Guidelines–Heart Failure (GWTG-HF) risk model is one of the most sophisticated and well-validated risk models for predicting in-hospital mortality. Moreover, adding the brain natriuretic peptide (BNP) to the GWTG-HF model has been shown to improve the model performance. Although recent studies reported that hypochloremia is strongly associated with mortality in patients with AHF, , it is unclear if hypochloremia increases the prognostic predictability and if incorporating this factor into the preexisting risk model can improve its performance. Therefore, we aimed to create a novel risk model and examine its performance.
Methods
We used 2 data sets of patients with AHF to examine whether incorporating hypochloremia, defined as a serum chloride level<98 mmol/L, can improve the model performance. First, we developed a new risk model that incorporates hypochloremia using a derivation cohort (Registry Focused on Very Early Presentation and Treatment in Emergency Department of Acute Heart Failure [REALITY-AHF] cohort). We then evaluated the model performance in an independent external validation cohort (Nara Registry and Analyses for Heart Failure [NARA-HF] cohort). The primary end point of this study was in-hospital mortality. We excluded patients with missing data on chloride and BNP levels at admission and those with missing GWTG-HF risk scores due to missing data on any of the components required to calculate it.
The REALITY-AHF was a prospective multicenter study (20 Japanese hospitals, including 9 university and 11 community hospitals), designed to evaluate the association between time to treatment and clinical outcome in patients with AHF presenting at the emergency department (ED). The study design has been reported elsewhere in detail. The AHF diagnosis was made based on the Framingham criteria. The exclusion criteria were treatment with an intravenous drug performed before ED arrival, previous heart transplantation, undergoing either chronic peritoneal dialysis or hemodialysis, acute myocarditis, and acute coronary syndrome requiring emergency/urgent revascularization. Patients with missing BNP or N-terminal-proBNP data were also excluded, as well as those with a BNP level <100 pg/ml or N-terminal-proBNP level <300 pg/ml at baseline. Patients were recruited from August 2014 to December 2015. At the ED phase, baseline physical findings and blood samples were evaluated for all patients as baseline data. The study information, including objectives, inclusion and exclusion criteria, and the names of participating hospitals, was published in the publicly available University Hospital Information Network (unique identifier: UMIN000014105) before the first patient was enrolled. The REALITY-AHF complied with the Declaration of Helsinki, and an institutional review board approval was obtained in each participating center.
NARA-HF retrospectively enrolled consecutive patients with AHF admitted to Nara Medical University Hospital from January 2007 to March 2011 and prospectively enrolled patients from April 2011 to December 2018. Diagnosis of HF was also made based on the Framingham study criteria. Patients with acute myocardial infarction, acute myocarditis, or AHF with acute pulmonary embolism were excluded. NARA-HF did not exclude patients with hemodialysis and BNP <100 pg/dL at the time of admission, based on its inclusion/exclusion criteria; however, we excluded them from the present analyses for consistency with the REALITY-AHF cohort. The present study was approved by the Nara Medical University Institutional Ethics Committee and performed according to the 1975 Declaration of Helsinki guidelines for clinical research protocols. Informed consent was obtained from all patients.
Continuous variables are expressed as means and SDs or as medians with interquartile ranges. The categorical variables are expressed as numbers and percentages. Associations between the variables and in-hospital mortality were evaluated using univariate and multivariate logistic regression models and generalized linear regression models. Unadjusted and adjusted odds ratios were estimated with their 95% confidence intervals; we also estimated the predicted in-hospital mortality.
For the derivation, we confirmed if hypochloremia was an independent predictor of in-hospital mortality independently of the GWTG-HF risk score and log-transformed BNP at admission (log BNP), as adding log BNP to GWTG-HF has already been shown to improve the model prediction performance. , In further analysis, log BNP levels were dichotomized to high/low levels according to the most appropriate cutoff derived from the Classification and Regression Trees analysis. Next, we performed the multivariate logistic regression analysis again to determine if hypochloremia remains independently associated with the in-hospital mortality after adjusting for the GWTG-HF risk score and high/low BNP level at admission. Based on the results of this multivariate logistic regression, we assigned an integer weight proportional to the regression beta coefficient for the GWTG-HF risk score, high/low BNP, and hypochloremia to create a new risk model. We defined the GWTG-BNP and GWTG-BNP-Cl risk scores, which comprise the GWTG-HF risk score and high/low BNP, and the GWTG-HF risk score, high/low BNP, and presence/absence of hypochloremia, respectively.
For the validation, we examined whether hypochloremia was an independent predictor of in-hospital mortality even after adjusting for the GWTG-HF risk score and log-transformed BNP in the NARA-HF cohort. Next, we calculated the GWTG-HF, GWTG-BNP, and GWTG-BNP-Cl risk scores to compare these 3 models. The discrimination and calibration performance of the GWTG-HF, GWTG-BNP, and GWTG-BNP-Cl risk scores was assessed using the Hosmer-Lemeshow test and the area under the receiver operating characteristic curve, respectively. The net reclassification improvement was calculated between the risk scores to compare the performance of the 3 models.
Results
Among the 1,682 patients registered to REALITY-AHF and the 1,391 patients registered to NARA-HF, 1,428 patients from REALITY-AHF were finally included and analyzed (201 patients were excluded because of the absence of BNP, 44 patients for no chloride levels, and 9 patients for no GWTG-HF risk score) and 1,256 patients from NARA-HF (79 patients were excluded for undergoing hemodialysis, 37 patients for BNP level <100 pg/dL, and 19 patients for missing data on BNP levels). The characteristics of the patients with and without hypochloremia are shown in Table 1 . The prevalence of patients with hypochloremia was 9.4% in the REALITY-AHF cohort and 12.2% in the NARA-HF cohort. Overall, the patient characteristics associated with hypochloremia were similar between the 2 cohorts and included lower blood pressure, lower prevalence of hypertension, lower rates of mineralocorticoid receptor-antagonist intake, lower sodium level, and higher C-reactive protein. In-hospital mortality was significantly higher in subjects with hypochloremia than in those without hypochloremia ( Figure 1 ).
Variable | REALITY-AHF | Nara-HF | ||||
---|---|---|---|---|---|---|
non-hypochloremia | hypochloremia | p value | non-hypochloremia | hypochloremia | p value | |
(n = 1,294) | (n = 134) | (n = 1,103) | (n = 153) | |||
Age (years) | 80 [71, 86] | 78 [67, 86] | 0.133 | 77 [67, 83] | 77 [68, 85] | 0.346 |
Men (%) | 735 (56.8%) | 68 (50.7%) | 0.210 | 638 (57.8%) | 76 (49.7%) | 0.068 |
Systolic blood pressure (mm Hg) | 151 ± 36 | 133 ± 36 | <0.001 | 147 ± 36 | 130 ± 36 | <0.001 |
Diastolic blood pressure (mm Hg) | 85 ± 25 | 76 ± 24 | <0.001 | 85 ± 25 | 76 ± 23 | <0.001 |
Heart rate (bpm) | 98 ± 29 | 93 ± 27 | 0.099 | 98 ± 28 | 91 ± 24 | 0.004 |
Left ventricular ejection fraction (%) | 46 [33, 69] | 48 [36, 63] | 0.165 | 44 [33, 60] | 46 [38, 63] | 0.141 |
NYHA Class III/IV at admission (%) | 1,098 (84.9%) | 115 (86.5%) | 0.712 | 1,009 (91.5%) | 144 (94.1%) | 0.338 |
Heart failure (%) | 661 (51.1%) | 71 (53.0%) | 0.742 | 216 (19.7%) | 40 (26.1%) | 0.079 |
Hypertension (%) | 881 (68.1%) | 74 (55.2%) | 0.004 | 844 (76.5%) | 103 (67.3%) | 0.018 |
Diabetes mellitus (%) | 470 (36.4%) | 49 (36.6%) | >0.999 | 432 (39.2%) | 72 (47.1%) | 0.075 |
Chronic Obstructive Pulmonary Disease (%) | 118 (9.1%) | 19 (14.2%) | 0.082 | 88 (8.0%) | 18 (11.8%) | 0.155 |
Coronary artery disease (%) | 390 (30.2%) | 36 (26.9%) | 0.487 | 286 (25.9%) | 31 (20.3%) | 0.158 |
Medication at admission (%) | ||||||
Loop diuretics | 661 (51.5%) | 87 (64.9%) | 0.004 | 507 (46.0%) | 81 (52.9%) | 0.120 |
Thiazide diuretics | 61 (4.8%) | 14 (10.4%) | 0.013 | 66 (6.0%) | 24 (15.4%) | <0.001 |
ACE-I | 215 (16.6%) | 25 (18.7%) | 0.637 | 258 (23.4%) | 38 (24.8%) | 0.769 |
ARB | 414 (32.1%) | 27 (20.1%) | 0.006 | 411 (37.3%) | 39 (25.5%) | 0.006 |
Beta blockers | 570 (44.3%) | 53 (39.6%) | 0.333 | 346 (31.4%) | 55 (35.9%) | 0.296 |
Aldosterone blockers | 289 (22.3%) | 38 (28.4%) | 0.141 | 173 (15.7%) | 44 (28.8%) | <0.001 |
Laboratory data at admission | ||||||
Albumin (g/dL) | 3.50 ± 0.56 | 3.37 ± 0.50 | 0.031 | 3.65 ± 0.49 | 3.57 ± 0.44 | 0.061 |
Hemoglobin (g/dL) | 11.78 ± 2.30 | 11.64 ± 2.09 | 0.519 | 11.60 ± 2.42 | 11.28 ± 2.20 | 0.122 |
Creatinine (mg/dL) | 1.12 [0.85, 1.62] | 0.98 [0.69, 1.53] | 0.006 | 1.11 [0.82, 1.70] | 1.17 [0.91, 1.95] | 0.064 |
BUN (mg/dL) | 25 [18, 35] | 24 [17, 37] | 0.810 | 23 [17, 35] | 28 [21, 47] | <0.001 |
Sodium (mmol/L) | 140 [137, 142] | 131 [128, 135] | <0.001 | 140 [138, 142] | 131 [127, 135] | <0.001 |
Chloride (mmol/L) | 105 [102, 108] | 95 [92, 96] | <0.001 | 105 [102, 107] | 94 [91, 96] | <0.001 |
Potassium (mmol/L) | 4.2 ± 0.7 | 4.3 ± 0.9 | 0.387 | 4.2 ± 0.7 | 4.3 ± 0.9 | 0.033 |
CRP (mg/dL) | 0.59 [0.20, 1.93] | 1.45 [0.42, 4.50] | <0.001 | 0.50 [0.20, 1.70] | 1.80 [0.30, 5.40] | <0.001 |
BNP (pg/ml) | 753 [451, 1348] | 668 [353, 1332] | 0.306 | 897 [513, 1607] | 1,097 [593, 1773] | 0.048 |
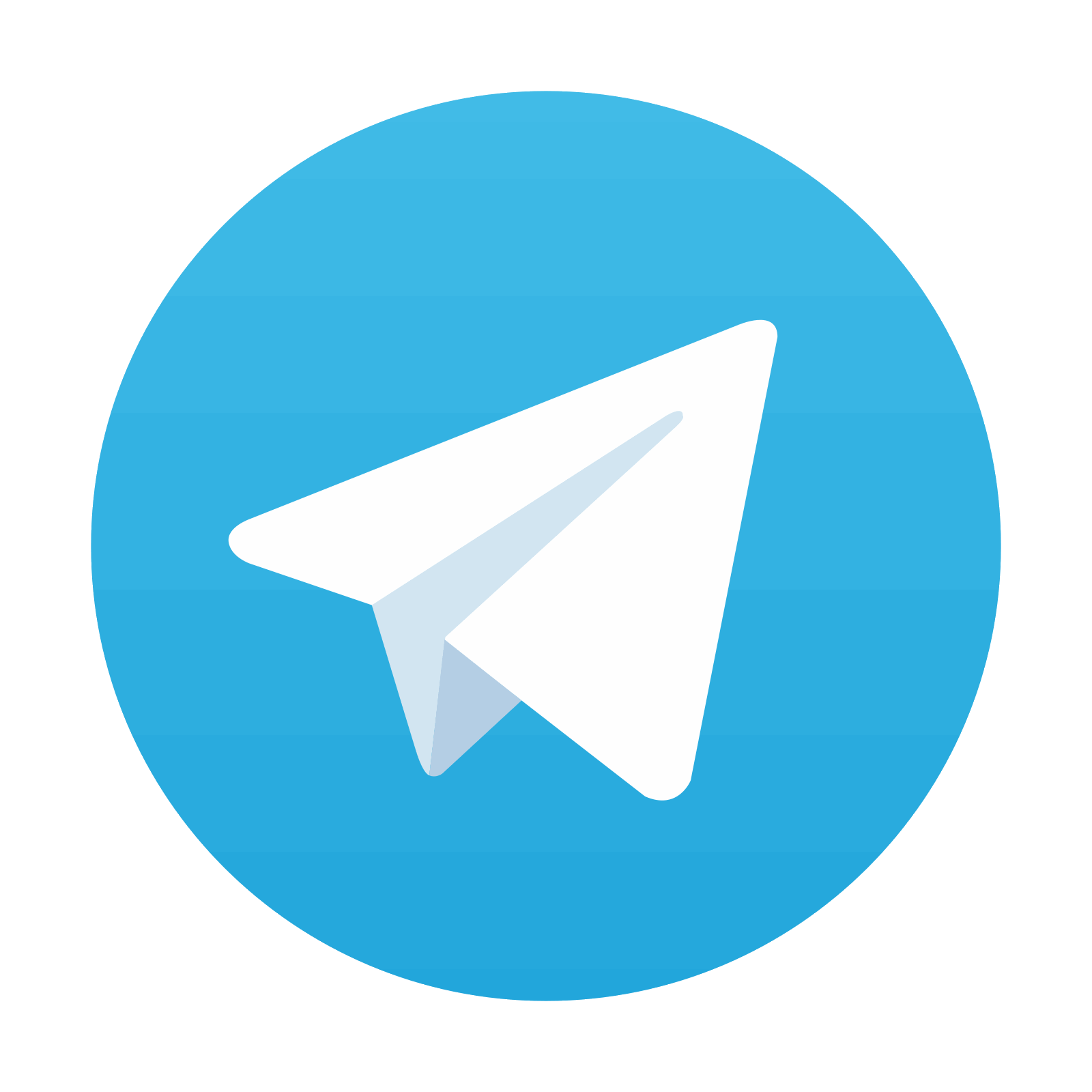
Stay updated, free articles. Join our Telegram channel

Full access? Get Clinical Tree
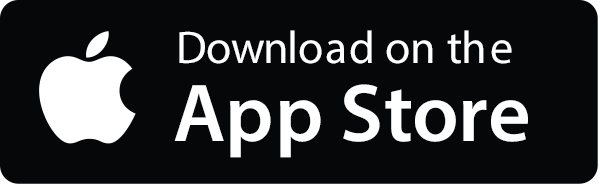

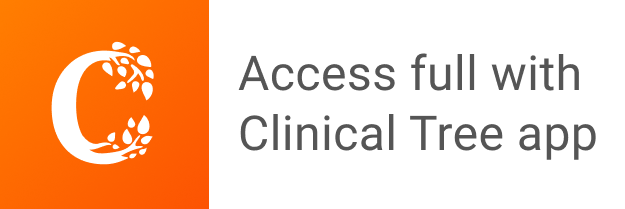