Systems biology to integrate complex data.
Lung Ventilation at Birth Is Dependent on Its Structure
How do the alveoli form? What are the cellular and molecular processes mediating alveolarization and lung maturation? How can static measurements of the cellular and molecular components of the lung be used to predict the dynamic nature of organ function? How important are the dynamic physical forces generated by lung fluid in utero and by the process of ventilation and perfusion to the structures and function of the lung? Although the size and tissue diversity of the conducting airways and large blood vessels have been amenable to quantitative visualization via casting and stereology and more recently by magnetic resonance imaging (MRI) and computerized tomography (CT) scanning, the fine structure of the alveoli have been more challenging to image because of their small size, diverse shapes, and low tissue density (12,13). Recent applications using computer assisted stereology of the developing lung (for example, with STEPanizer) enables rapid quantitation of volumes, lengths, and surfaces of tissue structure (14). X-ray defraction and MRI are unable to resolve alveolar structures at less than 2–3 microns. Imaging technologies, using finite element analysis of high resolution images made by using synchrotron radiation-based X-ray tomograms has furthered the study of the process of alveolarization at a level of resolution of 1.4 μm3. This technology is providing insights into the timing and processes involved in prenatal and postnatal growth of the pulmonary acinus and alveoli (13,15,16). With advances in confocal microscopy, immunochemistry, and new procedures to clarify tissue prior to fluorescence imaging, it is now possible to identify specific cell types and their precise anatomic positions during lung maturation (17–21). Changes in lung architecture accompanying the saccular–alveolar period of development in the primate (15,22), rat (23), and mouse (24) all support the concept that alveolar formation begins perinatally in processes that are shared among mammalian species. However, the timing of lung “maturation” proceeds according to species-specific time lines that are tightly regulated spatially and temporally. Although detailed studies in human alveolarization are limited (25), alveolar growth of the primate lung begins near the time of birth and continues into adulthood (24). In the rat and mouse, alveolar septae are formed primarily after birth within peripheral saccules that were formed by branching morphogenesis (15,26). Septa develop from preformed ridges by lifting off of new septa that further subdivide the saccules into alveoli. In the mouse, the process of alveolarization is most active from postnatal day 4 and continues through day 36. Approximately 5% of the surface area of the peripheral lung is formed prenatally via branching morphogenesis, while 50% of the surface area is created by the process of septation after birth (15). Continued growth of the lung parenchyma occurs after termination of septation, which in the mouse is completed at approximately 36 days postnatally. Thus, there are three major phases in the saccular–alveolar lung maturation in the mouse: (1) the saccular period from E17.5 to PN4 during which branching morphogenesis is completed and epithelial differentiation is induced; (2) the early alveolar period, during which new septa are produced (PN4-21); and (3) a period of isometric lung growth associated with the lengthening of alveolar septa, which continues until maturity. In the early postnatal period of mouse development, septation exceeds lung growth resulting in smaller alveoli. Alveolar size increases thereafter by the isometric growth of tissue that is achieved primarily by elongation, thus increasing the size of the alveoli, a process that continues until completion of lung growth in the mouse. Formation of alveoli is accompanied by the growth and maturation of the pulmonary capillary bed from a double lumen to single lumen alveolar capillary structure that is completed at approximately 21 days of age in the mouse (27). Both vasculogenesis and angiogenesis contribute to the formation of the extensive pulmonary microcirculation characteristic of the mature lung (28–30). Electron micrographic analyses have been a powerful approach to identify the cells contributing to the remarkable architecture during development and at maturity (1,31,32). More recently, use of cell-specific antisera, fluorescence probes, and transgenic mice used for genetic labeling of cells and cell lineages are providing detailed information regarding the cellular and molecular processes mediating branching morphogenesis and alveolarization (Figure 4-2)
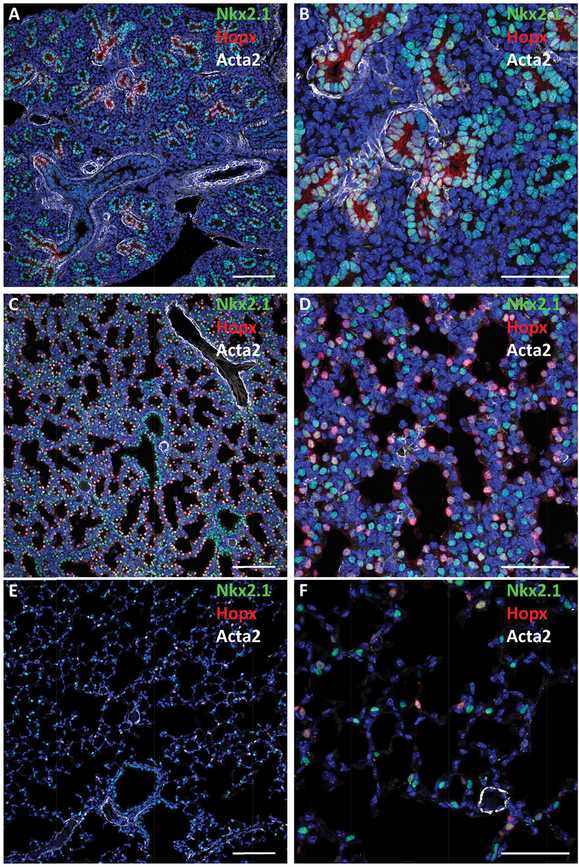
Maturation of the lung saccules before birth. Confocal immunofluorescence microscopy was used to image the fetal mouse lung at E16.5 (A, B), E18.5 (C, D), and postnatal lung at PND28 (E, F) demonstrating increasing dilation of the peripheral saccules with advancing age. Nkx2.1 (TTF-1) (green) expressed in epithelial progenitors at E16.5 and 18.5. At PND28, TTF-1 is selectively expressed in cuboidal type II epithelial cells; Hopx (red) is seen in squamous type I cells. As gestation advances, the tubules dilate and the walls of the saccules thin. At PND28, air spaces are prominent and alveolar walls are remarkably thin, enabling efficient gas exchange. αSMA (Acta2) staining of myofibroblasts is shown in white. Bars represent 100 microns (A, C, E) and 40 microns (B, D, F).
Physiological Adaptation to Air-Breathing Depends on Maturation of the Alveolar Epithelium
Perinatal survival is dependent on lung function once the placental circulation ceases at birth. Vital changes in lung and cardiovascular physiology accompany the transition to air-breathing: (1) clearance of lung liquid, (2) enhancement of pulmonary blood flow during the transition from fetal to postnatal cardiovascular circulation with closure of the foramen ovale and ductus arteriosus, and (3) production and secretion of pulmonary surfactant necessary to reduce surface tension at the gas–liquid interface at the alveolar surfaces. These changes are all required for the perinatal transition. At the cellular level, perinatal lung maturation is linked to the differentiation of the cuboidal alveolar type II epithelial cells that produce and secrete the four surfactant proteins (A, B, C, and D) and lipids (importantly, phosphatidylcholine, the major component of pulmonary surfactant) and the differentiation of more squamous, type I epithelial cells, whose close contact with the alveolar capillary endothelial cells enables efficient diffusion of oxygen and carbon dioxide (33, for review) (Figures 4-3 and 4-4). During the latter third of gestation, saccules dilate, mesenchymal components of the lung thin as the pulmonary microvasculature becomes more extensive and is increasingly in close contact with squamous type I epithelial cells lining the peripheral saccules. Dramatic changes in tissue architecture associated with sacculation and alveolarization of the mammalian lung before birth are accompanied by concomitant changes in the differentiation and metabolic functions of the epithelial cells lining the peripheral saccules. These events can be imaged using immunofluorescence confocal microscopy.
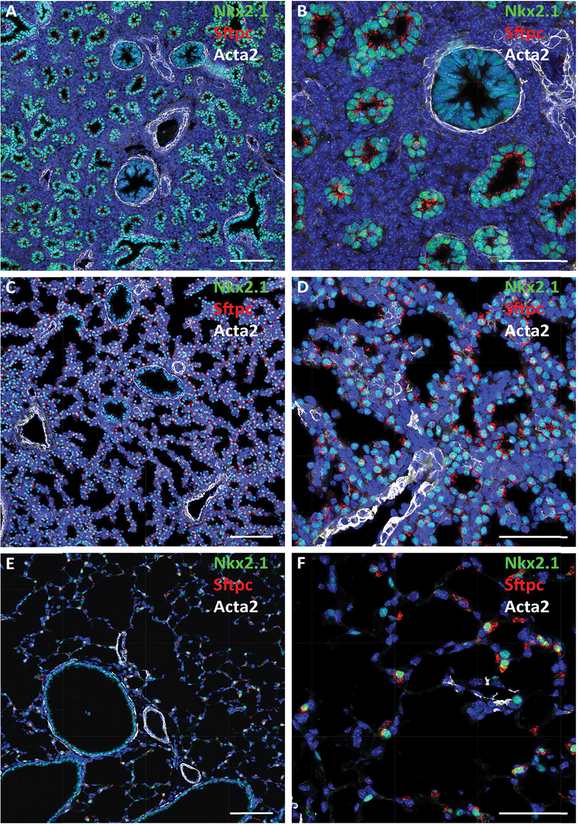
Confocal immunofluorescence microscopy shows developmental changes in lung architecture. Staining for proSP-C (red), Nkx2.1 (TTF-1; green), and αSMA (Acta2; white) are shown at E16.5 (A, B), 18.5 (C, D), and adult PND28 (E, F). Dilation of peripheral saccules occurs during maturation in association with increasing expression of the surfactant protein C (Sftpc) in Nkx2.1 stained cells. Bars represent 100 microns (A, C, E) and 40 microns (B, D, F).
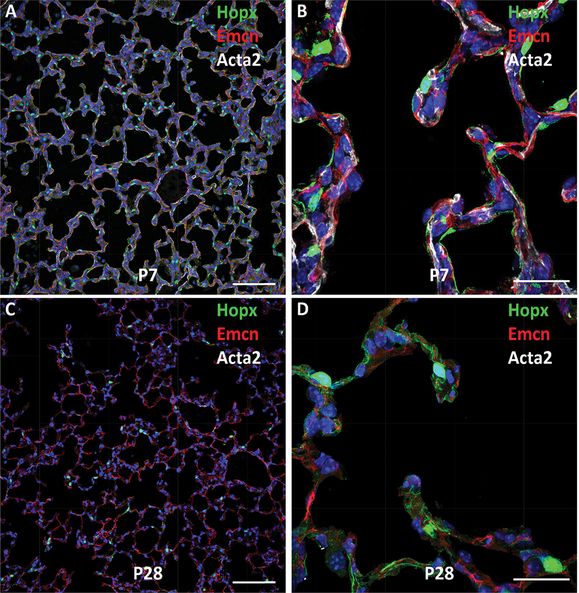
Shown are confocal images of mouse lung at PND7 (A, B) and PND28 (C, D). Type I epithelial cells express Hopx (green), alveolar capillary endothelial cells stain for endomucin (Emcn) (red), and myofibroblasts stain for Acta2 (αSMA) in white. Note prominent staining of αSMA during active alveolarization at PND7. Dual capillaries staining for Emcn (red) are seen within the septa. The surfaces of septal and alveolar walls are lined primarily by type I cells that stain for Hopx (green). Bars represent 100 microns (A, C) and 25 microns (B, D).
The architecture of epithelial and mesenchymal cells contributing to the alveoli and alveolar septa have been studied in detail using electron microscopy (1) and more recently using fluorescence confocal microscopy. In late gestation, the thickness of the lung saccules decreases in association with increasingly prominent microvasculature and the differentiation of type II and type I cells that line the surface of the peripheral lung. In the rodent, alveolarization is marked by the extension of αSMA stained myofibroblasts and pericytes toward the tips of the septa and the invasion of double lumen and capillaries that are covered by squamous type I epithelial cells. The alveolar walls of rodent lungs contain prominent lipofibroblasts and fibroblasts that stain for neutral lipids. Confocal fluorescence microscopy of some of the distinct cell types comprising alveolar septa in the developing mouse are shown in Figure 4-4.
Integrating Gene Expression Data with Lung Structure During Lung Maturation
A great challenge in lung biology is to integrate structural and genetic data with the cellular processes that determine lung maturation in the perinatal period. Formation of the alveoli requires complex interactions occurring among multiple cell types populating the peripheral lung, including diverse fibroblasts, pericytes, smooth muscle cells, epithelial and endothelial cells that mediate efficient ventilation and perfusion after birth. Proliferation, differentiation, migration, and appropriate placement of cells within the architecture of the peripheral lung saccules are dependent on myriad transcriptional and signaling networks active in each cell that are integrated by interactions with neighboring cells. Precise coordination of cell signaling via direct cell–cell contact, cell–matrix interactions, and those regulating both paracrine and autocrine cell communication direct the morphogenesis of the peripheral saccules during alveolarization. Ultimately, the spatial organization of the lung is dependent on complex interactions of multiple cell types that function within “ecological” networks. Although analysis of the complex, multicellular interactions is challenging, the application of single-cell transcriptomic analysis, lineage tracing, and cell sorting has begun to shed light on some of the regulatory processes involved in formation of the peripheral lung and maturation of the respiratory epithelium in the perinatal period.
RNA Expression Profiling and Functional Genomics Provide Insights into the Control of Lung Maturation
RNA analysis using RT-PCR, RNA microarray, RNA-sequence analyses (RNA-Seq), and chromatin immunoprecipitation (CHiP) are being used to identify genetic and molecular programs mediating lung maturation, the majority of the data being derived from the study of mouse lung development (34, 35). These and other experiments provide a wealth of expression data whose interpretation and integration with biological functions presents great opportunities for the computational and bioinformatic approaches needed to understand these complex biological systems. Study of gene expression in the lung include the expression profiling of RNAs from whole lung tissue, purified subsets of cells sampled by fluorescence activated cell sorting (FACS) or antibody mediated “panning,” and tissues sampled by laser capture microdissection. Recent advances include single-cell, whole genome analyses using RNA-sequencing (36). Together, these measurements provide rich data resources for the generation of new knowledge and hypotheses regarding lung maturation. A general workflow used in analysis of RNA expression data is given in Table 4-1, the study of which begins with an unbiased assessment of RNA expression data. The workflow consists of four major steps: data access, data analysis, data/knowledge integration, and data output.
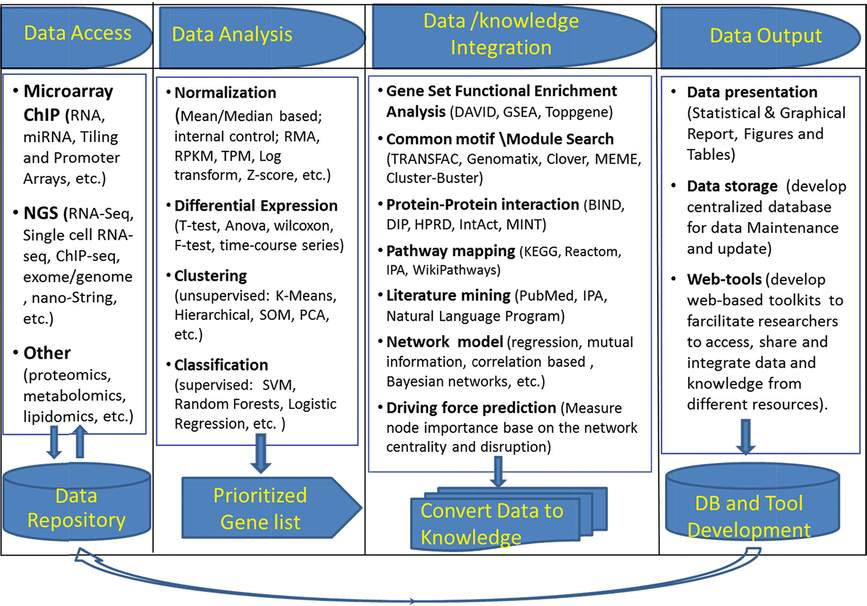
Schema of bioinformatics/systems biology workflow to process high-throughput data. The workflow consists of four major components: data access, data analysis, data/knowledge integration, and data output.
Data Access: Data are generated from specifically designed experiments from traditional research, and much of the data are already available in data warehouses. In a systems biology approach, extensive data resources that store and integrate data are available from different technological platforms that can be used to develop a comprehensive model.
Data Analysis: Transcriptomics has evolved rapidly with the advent of microarray and next-generation DNA sequencing technologies for large-scale DNA and RNA profiling. A wide range of statistical approaches have been developed for genome-wide expression data analysis and are readily available. Appropriate choices for these applications depend on the nature of data and experimental design. An understanding of both biology and computational approaches is essential for choosing an analytic pipeline and applying appropriate data mining tools. Some useful approaches for analysis of gene expression profiling include identifying differential expression, “clustering,” and predicting sample classifications using statistical approaches.
Differentially Expressed Genes: A common approach for transcriptional profiling experiments is to compare gene expression in two different conditions. In experiments without biological replicates, fold change is the simplest way to define differentially expressed genes (i.e., genes with ratios above a fixed cutoff; typically a difference of ±2 is considered to be significant). False discovery associated with this approach is significant. Replication is essential to enable estimation of variability, which is needed to identify reproducibility of changes among distinct samples. Standard t-tests are useful for detecting significant changes between two groups. Among multiple groups, the ANOVA F-test statistic is appropriate. For data failing to satisfy a normal distribution, nonparametric, rank-based statistics or permutation-based tests are preferred (37,38).
Pattern Recognition and Clustering Analysis: Pattern discovery is widely used to simplify data involving multivariate comparisons; related groups are identified based on expression similarity, which provides an “overview” of the data. Useful dimension-reduction methods include principal components analysis (PCA), independent components analysis (ICA), singular value decomposition (39), and other clustering algorithms. These methods are considered “unsupervised,” meaning that the analysis is derived solely from data and is independent of previous knowledge or classifications. PCA assumes that large variations in the data can be explained by a smaller number of transformed variables. PCA explains the variance-covariance structure of the original data through a few linear combinations of the variables and projects the original data into a new space, for example, in two- or three-dimensional visual representations.
Clustering is a powerful way to explore complex gene expression data and is useful for identifying similarity in expression patterns (40–42). Classical clustering algorithms including K-means, SOM, and “hierarchical” clustering generally emphasize clear group separations; any given entity is assigned to only one cluster. However, many genes have multiple cellular roles and function in cooperation with other interacting partners. Therefore, each gene/RNA/protein can belong to more than one functional class governed by distinct regulatory mechanisms in response to various conditions. Fuzzy heuristic partition (43) considers each gene to be a member of every cluster, each with variable degrees of membership. In these analyses, “genes” can be assigned to more than one cluster with variable but significant membership, enabling identification of context-dependent regulation. For example, we applied fuzzy clustering by a local approximation of membership algorithm (44) to 194 mRNA microarray samples from 27 distinct mouse models related to lung development and disease. We identified three coexpressed gene clusters that were highly enriched for lung RNAs that influence lipid synthesis, transport, and surfactant homeostasis (45).
Clustering methods are useful in grouping coexpressed genes and provide a basis for the identification of functionally related genes and shared mechanisms by which they are regulated. Alternatively, one can identify their relationship to specific phenotypes in a process used to identify disease-related pathways. Published expression studies of lung RNAs derived after conditional deletion or mutation of specific genes led to the identification a number of transcription factors and signaling molecules that are critical for respiratory adaptation at birth, including NKX2-1 (also termed TTF-1 or thyroid transcription factor-1), FOXA2, C/EBPα, and CNB1. Conditional deletion or mutation of these genes caused phenotypic and biochemical changes similar to those associated with respiratory distress syndrome (RDS), for example, decreased expression of pulmonary surfactant-related lipids and proteins, failure of differentiation of type I and II cells, lack of lamellar bodies, and delayed sacculation (46–50). Meta-analysis of RNA microarray data from these “phenoclusters” showed that although these transcription factors and signaling molecules act via different signaling pathways and bind to distinct cis-elements on transcriptional targets, each is required for normal expression of common transcriptional targets involved in surfactant protein and lipid biosynthesis (e.g., Abca3, Scd1, Pon1, Sftpa, Sftpb, Sftpc, and Sftpd), fluid and solute transport (e.g., Aqp5, Scnn1g, and Slc34a2), and innate host defense (e.g., Lys, Sftpa, Sftpd, and Scgb1a1), suggesting that FOXA2, CEBPα, CNB1, and TTF-1 (NKX2.1) function in a common transcriptional network regulating genes for perinatal lung maturation and postnatal respiratory adaptation (Figure 4-5A, B).
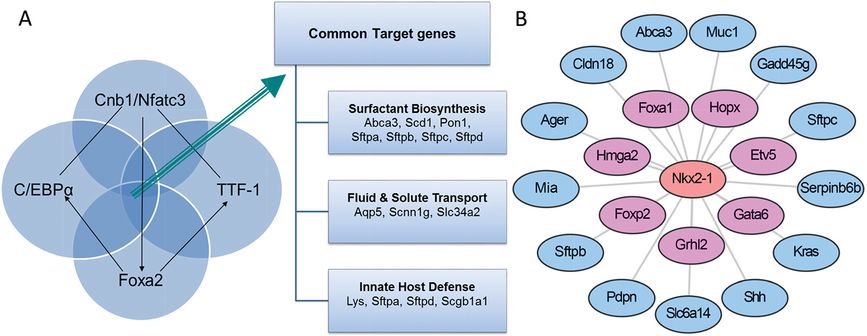
NKX2-1 (TTF-1) plays a central role in lung formation and maturation. (A) Meta-analysis of microarrays from mouse models sharing common respiratory distress phenotypes at birth (“phenocluster”). Lung epithelial deletion of Foxa2, Cebpa, Cnb1, and mutation of the mouse Nkx2-1 gene (encodes TTF-1) identified common transcriptional targets involved in surfactant biosynthesis, fluid and solute transport, and innate defense. (B) An Nkx2-1 local transcription network was identified as an important transcription factor by statistical “driving force analysis.” The NKX2-1 gene influences expression of numerous genes important for lung epithelial growth, differentiation, and function.
Data/Knowledge Integration: After identifying differentially expressed genes and coexpressed gene groups, general questions to address are: “What is unique about the differentially expressed genes or gene set? Do these genes instruct our understanding of the mechanisms underlying the complex process of lung maturation?” Statistical significance does not directly lead to “biological discovery.” Data integration, via systems biology approaches represents an unbiased way to identify the biological themes that are present in complex data sets.
Gene Set Functional Enrichment Analysis: As each gene is associated with multiple biological annotations from various resources (e.g., gene ontologies, pathways, protein–protein interactions, mouse and human phenotypes, promoter cis-elements), the enrichment of genes in functional categories can be estimated using Fisher’s exact test to compare the occurrence of a given term in the gene set of interest to all available data. Multiple precompiled Web-based functional annotation tools, including Onto-Express (51), GoMiner (52), DAVID (53), GSEA (54), and ToppGene (55), have been developed to identify potential functions of groups of genes.
Biological Knowledge Incorporation: Genome-wide biological knowledge data have been assembled via a number of public/private efforts that include functional annotations and curations of genes/proteins, for example, NCBI Entrez Gene database (http://www.ncbi.nlm.nih.gov/), Ensembl (http://www.ensembl.org/index.html), GeneCards (http://www.genecards.org/), ENCODE (http://www.genome.gov/10005107), Protein Information Resource (PIR: http://pir.georgetown.edu/), Kyoto Encyclopedia of Genes and Genomes (KEGG: http://www.genome.jp/kegg/), Online Mendelian Inheritance in Man (OMIM: http://omim.org/), Human Gene Mutation Database (HGMD, http://www.hgmd.org), and Ingenuity knowledge base (IPA: http://www.ingenuity.com/science/knowledge-base). These resources provide exceptional depth of present functional knowledge for genes and proteins. Incorporation of knowledge from different genomic and biomedical information resources is useful for “omics” data interpretation. Algorithms for integrating different types of data show promise in combining high-throughput data with knowledge from other clinical or experimental observations.
Network Modeling: A wide range of methods are useful for inferring genome-scale regulatory networks from gene expression RNA datasets (56). A comprehensive assessment of over 30 network inference methods used to analyze microarray datasets from multiple resources was provided by Marbach et al. (57), who concluded that no single method performs optimally across all data sets. In contrast, integrative predictions from multiple inference methods were most useful. Different methods for integration provide complementary advantages and are powerful for optimizing identification of regulatory networks. Such network development and optimization can be achieved via multilevel integration (i.e., data, knowledge, and method integration, as shown in Table 4-1).
Driving Force Prediction: The identification of essential regulators controlling cell fate and associated biological processes during lung development and maturation is fundamentally important to lung biology and identifies genes to prioritize for experimental validation. An algorithm to quantitatively measure node importance in networks based on node centrality (determine a node connectivity with all other nodes in the network) and/or disruption (determine how the removal of a node in the network affects the network structure) (58, 59) was developed to model regulatory networks important for lung maturation (Guo et al., submitted for publication). Figure 4-5B shows an example of an NKX2-1 subnetwork model based on the single-cell RNA-seq data from E16.5 mouse lung. In that model, TTF-1 (Nkx2-1) is predicted to be an important lung epithelial cell specific driving force functioning via its interactions with other transcription factors, including Gata6, Etv5, Foxp2, Grhl2, and Hopx to regulate target genes, including surfactant proteins, Abca3, Cldn18, Shh, Kras, and Muc1 (Figure 4-5B).
Data Output: Data related to “lung development and disease” has expanded dramatically in the past decade, providing a wealth of data resources useful for both basic science and clinical applications. Given the high volume and complex nature of these data, data storage, maintenance, and utilization becomes a major challenge. A LungMAP consortium (http://www.lungmap.net/) provides a freely accessible repository of comprehensive lung development data from different species and developmental time points. This LungMAP is linked to Web-based resources supporting investigations into the processes that regulate lung development. An analytic pipeline (https://research.cchmc.org/pbge/sincera.html), relational database, and associated web-tool, termed “LungGENS,” was developed to assist users to query gene expression patterns and cell type RNA signatures in specific pulmonary cells at the single-cell level (https://research.cchmc.org/pbge/lunggens/default.html).
Transcriptional Regulatory Network Regulating Lung Surfactant Homeostasis (Static Model): Pulmonary surfactant is required for lung function at birth and throughout life. Lung lipid and surfactant homeostasis requires regulation among multitiered processes, coordinating the synthesis of surfactant proteins and lipids, their assembly, trafficking, and storage in type II alveolar cells. To generate a transcriptional regulatory network model controlling surfactant homeostasis, we retrieved mRNA microarray samples from a number of distinct experiments in which mouse models were utilized to study lung maturation and function. An algorithm integrating expression profiling with expression-independent knowledge using Gene Ontology (GO) similarity analysis, promoter (gene regulatory sequences) motif, searching, protein–protein interactions, and literature mining were developed to model genetic networks regulating surfactant lipid–related biological processes in lung. A transcription factor (TF)-target gene (TG) similarity matrix was generated by integrating data from different analytic methods. A scoring function was built to rank likely transcription factor–transcription factor regulated targets or TF–TG pairs. Using this strategy, critical components of transcriptional networks directing lipogenesis, lipid trafficking, and surfactant homeostasis in the mouse lung were identified. Within the transcriptional network, SREBP, CEBPA, FOXA2, ETSF, GATA6, and IRF1 were identified as regulatory hubs displaying high connectivity (Figure 4-6). SREBP, FOXA2, and CEBPA were identified as transcription factors forming a regulatory module that controls expression of pulmonary surfactant lipid homeostasis. In turn, this core module comprised of critical transcriptional regulators and their target genes cooperates with other factors to regulate perinatal lung and lipid homeostasis, cell growth, survival, and immune responses (45).
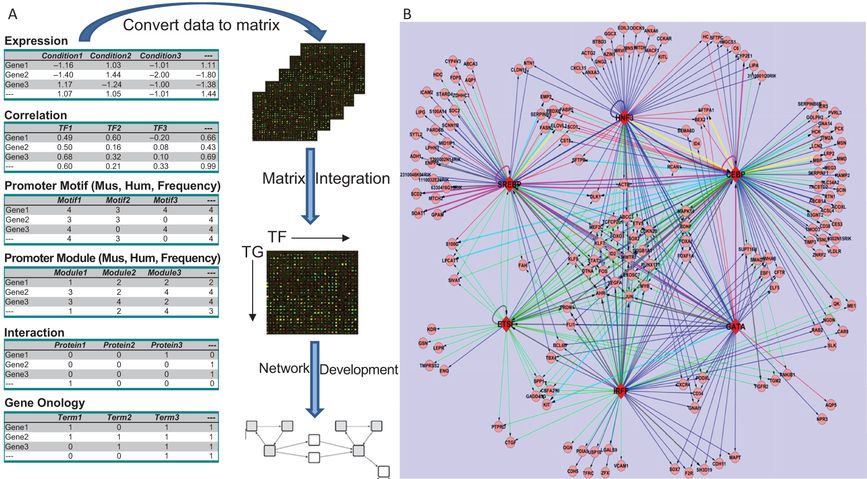
Development of a transcriptional regulatory network (TRN) regulating surfactant lipid homeostasis. (A) Work flow for construction of a TRN via data and knowledge integration from multiple independent gene expression profiling studies and expression-independent data (protein interactions, functional annotation, promoter analyses, and literature mining) is shown. We calculated the relative confidence score of TF–TG associations by combining the data. (B) A graphical representation of a subnetwork consisting of predicted TF–TG pairs with confidence cutoff as 0.60 (top 4.5%) and the top 6 TFs with the highest connectivity is shown. The network has 183 nodes and 386 links. Round nodes represent TGs; red diamond nodes represent TFs
Dynamic Modeling of the Transcriptional Programs Controlling Perinatal Lung Maturation: Genetic, genomic, and bioinformatics methods were used to analyze relationships between the length of gestation and lung maturation in two inbred mouse strains (C57BL/6J and A/J) whose gestational length differed by approximately 30 hr. We were able to identify the genetic regulators that link lung maturation to the timing of birth (60–62). A functional Bayesian statistical approach was used to analyze time-dependent changes in lung mRNAs from each mouse strain from E15.5 to postnatal day 0 (PN0) (63). Dynamic profiling of transcription factors and their targets that changed during lung maturation were matched using STEM (Short Time-series Expression Miner), a clustering algorithm designed for the analysis of a series of gene expression data sets (64). Through these analyses, we identified both temporal and strain-dependent gene expression patterns during lung maturation, identified key regulators, bioprocesses, and transcriptional networks controlling lung maturation. Cell adhesion, vasculature development, and lipid metabolism/transport were major bioprocesses induced during the saccular stage of lung development at E16.5–E17.5 (Figure 4-7). CEBPA, PPARG, VEGFA, CAV1, and CDH1 were found to be key signaling and transcriptional regulators of these processes. Innate defense/immune responses were induced at later gestational ages (E18.5–20.5), and STAT1, AP1, and EGFR were important regulators of these responses. Expression of RNAs associated with the cell cycle and chromatin assembly was repressed with advancing prenatal lung maturation, with predicted regulation by FOXM1, PLK1, chromobox, and high mobility group families of transcription factors. Mouse strain-dependent differences in RNA expression patterns were most apparent at E18.5, indicating the earlier maturation of the lung in mice of shorter gestation. At this time, mRNAs regulating surfactant and innate immunity were controlled by the genotype of the dam. These more abundantly expressed genes were in lungs of C58BL6 mice (short gestation) relative to the A/J strain (long gestation), supporting the notion that prior to birth, innate immune responses and surfactant production are critical and “connected” processes that influence cellular behaviors required for respiration and survival after birth (35, 65). Timing of lung maturation and gestational length were determined by the maternal, not paternal, genome in these two mouse strains.
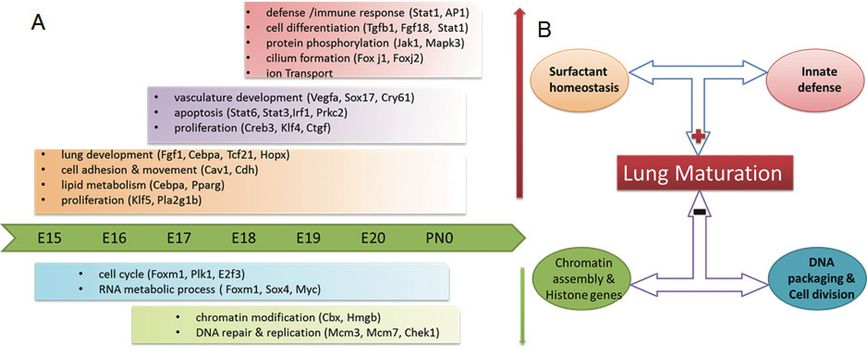
Schematized depiction of gestational length and strain dependent effects on lung maturation. (A) Key bioprocesses and regulators controlling lung maturation. (B) Strain effect underlying lung maturation
Transcriptional Control of the Genetic Circuits That Regulate Maturation of the Respiratory Epithelium
Changes in lung architecture and cardiopulmonary physiology that accompany the transition to air breathing are mediated by dramatic changes in gene expression that controls cell proliferation and differentiation in pulmonary epithelial cells (35, 36). The identification of the surfactant proteins and genes controlling surfactant lipid homeostasis provided molecular tools (antibodies, cDNAs, and gene promoters) useful for a further exploration of the genetic basis of lung epithelial maturation (33). The precise orchestration of gene expression controlling cell proliferation and differentiation directs the behavior of cells critical for lung function. RNA microarray and RNA-sequence studies utilized in concert with cell lineage tracing, gene deletion, and gene addition experiments have helped elucidate the processes regulating lung maturation. High rates of cell proliferation occur during the period of embryonic lung formation as peripheral lung structures are formed by the process of branching morphogenesis occurring from E9.5 to approximately E17.5 in the developing mouse. During the saccular period of perinatal lung maturation before birth, cell proliferation decreases in concert with increasing expression of a multiplicity of genes associated with epithelial cell differentiation (35). In the mouse lung, proliferation of peripheral lung cells again increases in the early postnatal period, when the processes of septation and lung growth begin to form the alveolar structures characteristic of the more mature lung. Expression of genes associated with pulmonary maturation, including those encoding the surfactant-associated proteins, particularly those related to host defense, fluid and electrolyte transport, and surfactant homeostasis are induced during this late saccular period in lung development prior to birth (E17.5 to birth) (35, 66). Bioinformatic analyses of the changes in expression of RNAs accompanying the perinatal period provides insight into the regulatory network controlling lung function at birth (35, 45) (Figures 4-6 and 4-7). Although relatively limited data are available at the level of single cells, the ability to identify the genetic processes in specific lung cells provided by single-cell analysis have been integrated with dynamic changes in RNAs that occur during whole tissue development that, together, begin to identify the genetic programs in respiratory epithelial cells, during perinatal lung function (36).
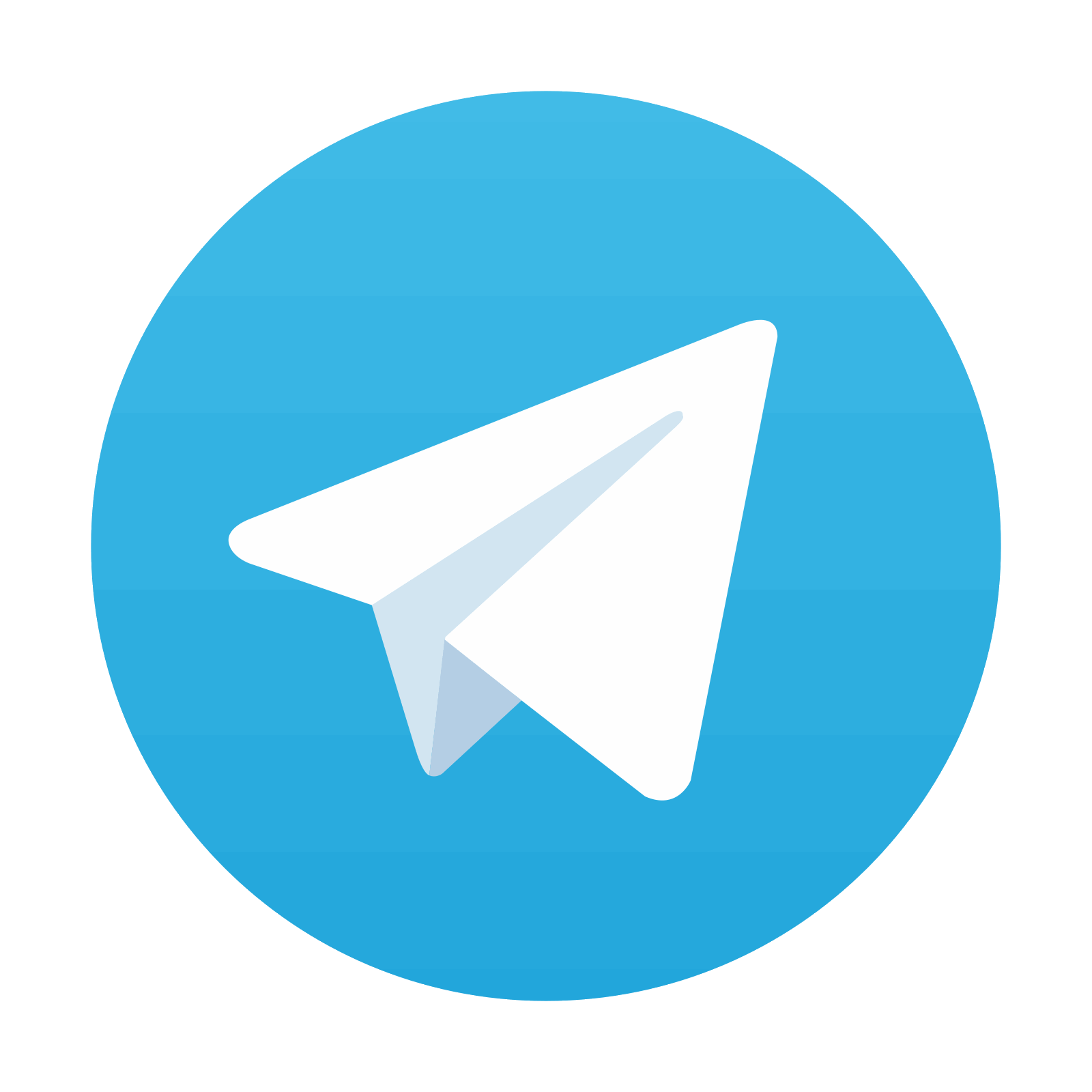
Stay updated, free articles. Join our Telegram channel

Full access? Get Clinical Tree
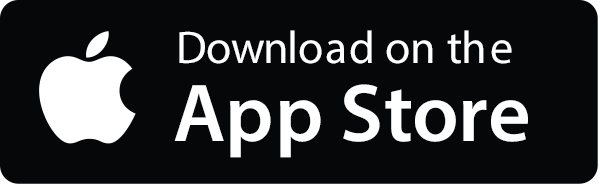
