Fig. 32.1
Schematic of evolving patient data. Data that is observed only intermittently (a) may not reveal critical underlying clinical trends (b).
Variety
Data in critical care comes from multiple interrelated sources that can serve to illuminate or cloud the relevant underlying clinical state. As an example, consider the evaluation and monitoring of adequate oxygen supply/demand balance. Estimating this factor for a critically ill patient is arguably the most important aspect of pediatric critical care [7]. While the balance between oxygen demand and delivery can be estimated and calculated with intermittent data points, it is difficult to measure continuously. Moreover, there is no way to measure the effective utilization of oxygen for oxidative phosphorylation at the mitochondrial level. Instead, clinicians use a variety of signals that serve, to some degree, as indirect measurements. These proxy measurements include general hemodynamic parameters (e.g. heart rate, perfusion pressure), end-organ perfusion parameters (urine output and biochemical indices of function), metabolic byproducts (lactic acid, and mixed venous O2 level), and other hematologic parameters (hemoglobin). Although none of these individual proxies provide sufficient information to properly assess adequate oxygen delivery, taken as a group they can theoretically provide a more accurate physiologic picture.
In practice, clinicians have a limited capacity to interpret multiple dissimilar data sources [1, 8]. In a study by Tibby et al. [9] clinicians were asked to stratify the cardiac output of ventilated children (high, normal, low) when provided with all available hemodynamic, laboratory, and physical examination findings. When their responses were compared to the current gold-standard measurement derived from femoral artery thermodilution, researchers found low correlation (r = 0.24) independent of the level of training of the clinician, thus demonstrating the inherent challenge of complex clinical assessment.
Volume
The frequent and standardized repetition of specific circumscribed care will improve a clinician’s technical skills. This is much more difficult for complex and varying clinical situations in critical care, such as the management of multi-organ failure or postoperative cardiac surgical care. For a clinician treating such a patient, it is difficult to abstract the enormous volume of data into meaningful information at the moment of care, let alone to derive important and new clinical knowledge that will be relevant to the next patient.
Despite years of experience with ostensibly similar patients, clinicians often reach markedly divergent conclusions about patient care. In an unpublished study, the authors conducted a survey of attending-level physicians to assess the perceived critical bounds of physiologic variables for post-operative management of single-ventricle infants after stage 1 palliation. Physicians were asked to specify three regions for each variable: (1) Physiologically optimal (Green), (2) Physiologically non-optimal (Yellow), and (3) Imminent physiologic failure (Red). The bounds assigned by the clinicians were then compared with the actual physiologic histograms from 39 patients.
Figure 32.2 shows frequency data for Heart Rate (HR), Oxygen Saturation (SpO2) and Mean Arterial Pressure (ABPm) for this cohort, and demonstrates considerable inconsistencies between individual practitioners in the perceived bounds. Specifically, some practitioners set bounds implying imminent clinical failure more than 40 % of the time, and others assigned values that were >3 standard deviations from the mean as normal. Thus despite years of training and experience, it is challenging to integrate patient data into consistent and accurate clinical guidelines.
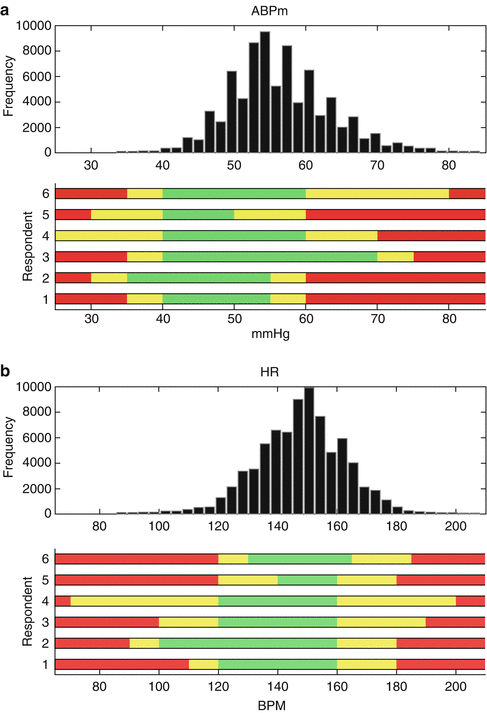
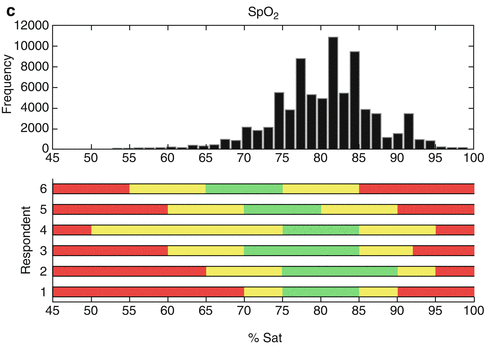
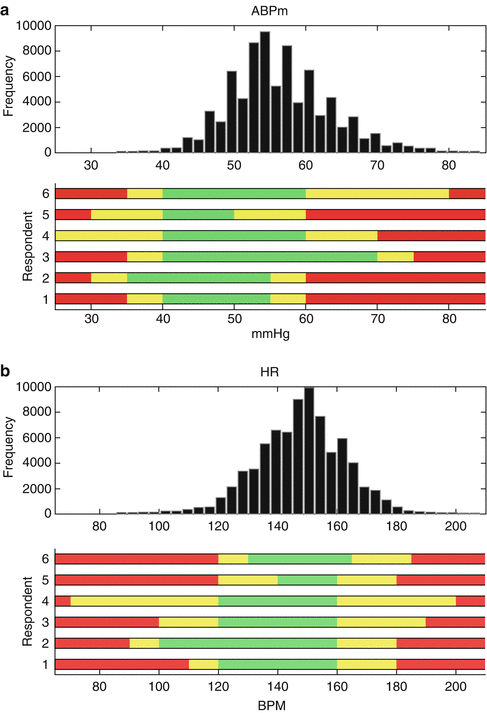
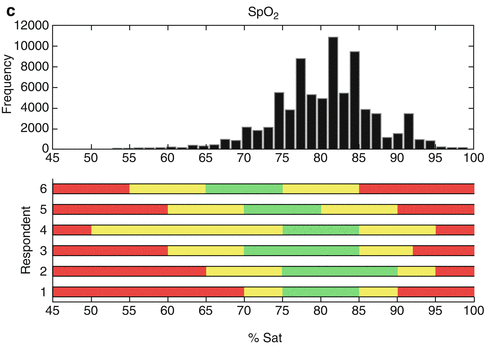
Fig. 32.2
Histograms of patient data frequency vs. clinician’s assignment of physiologically optimal (Green), physiologically non-optimal (Yellow), and imminent physiologic failure (Red) for Mean Arterial Blood Pressure (a), Heart Rate (b), and Oxygen Saturation (c).
Components of Effective Data Utilization
Technologies that seek to overcome the problems caused by data overload in critical care will inevitably need to address several common components of data analysis and utilization. First, data must be captured from multiple heterogeneous sources, formatted universally, and aggregated into a single cluster. Next, the data must be analyzed to extract information relevant to a specific patient’s state and trajectory. Finally, the resulting information must be presented to clinicians so as to effectively assist the care process. The following sections present an overview of the specific challenges presented by each of these components, and some technological solutions to these challenges.
Data Capture
Sources of patient information include those directly attached to patients, such as external sensors/devices, and external software systems, such as the electronic health records. Each of these may be locally self-contained, networked back to a local hub within a patient room, or networked centrally within the hospital.
Data may exist in one of many standardized formats, and exchanged using protocols that span multiple conceptual levels. The process of information exchange is often described as the connection between “stacks” of protocols, where each protocol acts on a higher conceptual level than the one preceding it. The conceptual levels can be summarized generically, in increasing conceptual level, as (a) the method of delivery, (b) the structure of the message, (c) the content of the message, and (d) the expected behavior of the recipient. Only when these rules are defined and understood by stakeholders will the exchange proceed in an efficient and expected manner. In order to successfully collect and collate data from multiple healthcare data sources it is imperative that the exchange mechanisms are compatible at each level of the protocol. The mechanisms of interoperation are beyond the scope of this document, but one could imagine that the challenges of information exchange depend largely on which protocols need to be adapted.
A deceptively challenging task is to associate data with its originating patient. While some devices can do this locally by collecting and displaying data only within a patient room, networked data requires a system to associate the correct source of data with other levels of information, such as from the admission, discharge, transfer and patient demographic systems, to ensure there is system-wide agreement. This type of challenge, known as the “multi-master problem” arises whenever two systems assert the same piece of information and a third system has to adjudicate to determine which it should accept. For example, if a monitor in room 123 is associated with Patient A, but the EMR associates room 123 with Patient B, a third system which integrates information from both systems has the option to either (a) assume that the monitor is correct, (b) assume that the EMR is correct, or (c) assume neither and disregard messages until the discrepancy is resolved. The correct resolution will depend on clinical workflows and site-specific requirements, such as ensuring that a nurse or clerk in the critical care unit correctly enters the unique medical record number for a patient to a specific bed space monitoring system, but might involve alerting a clinician to the discrepancy and making the data unavailable until changes are made to correct the ambiguity. A best practice is to reduce the chances of multi-master problems by providing as few masters as possible. When feasible, systems can be connected such that one upstream change (e.g. during the admission process) triggers updates to both the EMR and monitor systems, ensuring they stay synchronized.
< div class='tao-gold-member'>
Only gold members can continue reading. Log In or Register a > to continue
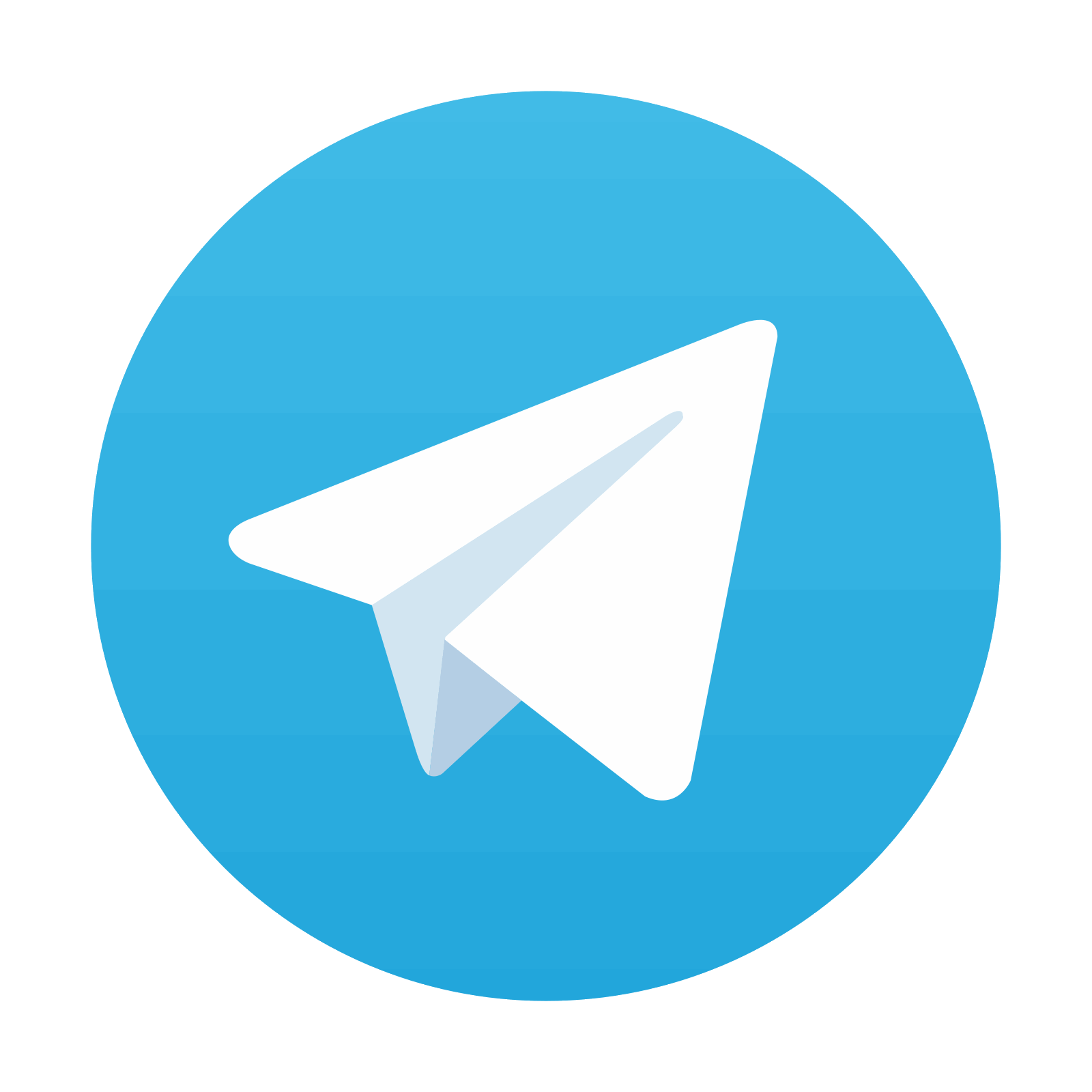
Stay updated, free articles. Join our Telegram channel

Full access? Get Clinical Tree
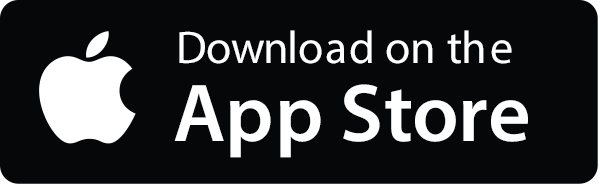
