Fig. 5.1
In physiological conditions, different opposing positive and negative feedback mechanisms, such as baroreceptors and vagal and sympathetic afferents, in addition to central integration, promote neural control of the cardiovascular system
5.2 Methodologies
5.2.1 General Considerations
The appreciation that the technique for the assessment of autonomic cardiovascular control needs to be reliable, reproducible, and noninvasive has led to the development of several different tools during the last decades. Generally, we can classify three groups of techniques: (1) assessment of sympathetic activity by measuring plasmatic catecholamines, (2) direct recording of sympathetic nerve activity (e.g., muscle sympathetic nerve activity, MSNA, using a microneurographic techniques), and (3) the analysis of sympathetic and parasympathetic oscillations embedded in heart period and arterial pressure time series.
Plasmatic catecholamines are less reproducible even within the same patient and are an unreliable index of sympathetic activity as their levels are influenced by several factors, thus supporting the idea that this measure must not be considered a consistent and reproducible tool. The direct measure of sympathetic nerve activity is a reliable and reproducible tool to evaluate sympathetic nerve activity in health and diseases [6, 24]; however, its invasivity, although mild, could be a possible limiting factor for this tool specifically when large populations are involved. On the opposite, the analysis of cardiovascular oscillatory components related to sympathetic and parasympathetic controls to sinus node and vascular tree is able to assess autonomic regulation in health and pathologies being a noninvasive, reliable, and reproducible tool called heart rate variability (HRV) [24].
5.2.2 Muscle Sympathetic Nerve Activity (MSNA)
Neural pathways convey biological information coded simultaneously based on two different modalities, i.e., intensity, which represent the amplitude of the signal, or tonic activity, and oscillatory discharge pattern, i.e., modulation [7].
The direct recording of muscle sympathetic nerve activity (MSNA) allows the evaluation of both intensity and modulation of sympathetic neural discharge. In fact, absolute values of MSNA can provide information on sympathetic tonic activity when considering the dynamic responses to stressor stimuli (i.e., orthostatic challenge, pharmacological stimulation), because MSNA signal fluctuates depending on amplification and filters, electrode position, and the number of single units [23]. MSNA variability contains two main rhythmic oscillatory components, low-frequency (LF) and high-frequency (HF) rhythms [5], which are coherent with the corresponding heart period and blood spectral components.
Parasympathetic activation, induced by the pressure response to phenylephrine infusion, is characterized by a predominant HF component in heart period, blood pressure, and MSNA variability, while, on the contrary, sympathetic activation induced by nitroprusside infusion is associated with LF component [29], thus suggesting that these oscillations are index of neural excitation (LF) and inhibition (HF). Gravitational stimulus (i.e., tilt test maneuver) induced similar MSNA responses, showing that orthostatism was able to induce a significant increase of LF and a decrease of HF component of MSNA [6].
Interestingly, it has been shown that acute beta-blockade affected cardiovascular and MSNA spectral profile in healthy subjects: in fact, atenolol infusion caused an increase in R-R intervals, as expected, and an increased power of MSNA in the HF band and a higher coherence between HF component of MSNA and respiration, suggesting an increased cardiorespiratory coupling [4].
However, in addition to sympathetic neural recording, direct recording of renal sympathetic nerve activity in animals (RSNA) and skin (SSNA) has provided interesting information on autonomic reflexes in different situations (orthostatism, exercise, cardiovascular diseases) [26, 37].
In summary, MSNA can be considered a reliable tool able to provide important information on the sympathetic neural discharge in terms of tonic activity and rhythmic oscillations. However, MSNA provides information only on sympathetic neural discharge, while no information is provided on the vagal branch; in addition, MSNA is a relatively invasive technique, thus rendering this tool unsuited to researches on large scales [23, 24].
5.2.3 Heart Rate Variability
Five decades ago, pioneer studies reported that changes in RR intervals could have been used for an early identification of fetal distress before heart rate modifications were detected 16. The same was then reported for detection of diabetic neuropathy [25] and for the quantification of respiratory sinus arrhythmia (RSA) in post-myocardial infarction (i.e., reduced RSA associated with an increased mortality) [39].
These studies supported the hypothesis that heart rate and blood pressure rhythmically oscillate around their mean values and that these oscillations are due to the sympathetic and vagal modulation of sinus node function and vascular tree. Since each biological time series, including heart period and blood pressure time series, are the sum of several oscillatory components, characterized by a certain frequency and amplitude, the analysis of cardiovascular oscillations can provide reliable information on sympathetic and parasympathetic control of cardiovascular functions in physiological conditions as well as in cardiac and noncardiac diseases [1, 22, 23]. Thus, the analysis of these rhythmical components can be considered a window over cardiovascular autonomic control, i.e., heart rate and blood pressure variability (HRV and BPV) [28].
In the seventies, the idea was introduced that a computational analysis of heart rate and blood pressure variability (HRV and BPV, respectively) could have been a reliable method to investigate cardiovascular autonomic modulation. The important clinical relevance of this technique has later been demonstrated by the fact that HRV possesses a strong prognostic value in cardiac diseases. For instance, Kleiger et al. were the first to demonstrate that a reduction of HRV parameters following acute myocardial infarction was an independent predictor of mortality [15, 18]. In the last years, a growing interest has been focused on the complex interaction between sympathetic and parasympathetic afferent and efferent pathways, the sympatho-sympathetic excitatory reflexes [20], and the reciprocal and non-reciprocal changes of the sympathovagal balance in health and diseases.
5.2.3.1 Linear Analysis
Frequency domain analysis is based on the evaluation of different rhythmic oscillatory components embedded in cardiovascular time series. Several different techniques have been validated in the past years for HRV analysis, usually categorized into parametric and nonparametric methods. Parametric approaches, such as autoregressive models, can identify spectral components independently of preselected frequency bands and they can provide accurate evaluation of spectra on small samples. Nonparametric methods use a simple algorithm, such as Fast Fourier Transform, and are characterized by higher process speed (Task Force of the European Society of Cardiology the North American Society of Pacing Electrophysiology [32]).
Spectral analysis of HRV assesses rhythmical oscillations embedded in cardiovascular time series. By using an autoregressive model, the algorithm can identify three main oscillatory components which are embedded in heart period and blood pressure time series:
Very low-frequency component (VLF), frequency band below 0.04 Hz
Low-frequency component (LF), frequency band bounded between 0.04 and 0.15 Hz
High-frequency component (HF), frequency band bounded between 0.15 and 0.4 Hz, synchronous with respiration
Experimental studies showed that VLF can be considered a marker of very slow oscillations such as hormonal changes, circadian rhythms, etc.; LF component is a marker of sympathetic heart rate modulation, while HF component is a marker of vagal heart rate modulation. The sum of VLF, LF, and HF represents the area under the curve, also called al total power (TP), or total variability; TP is a global marker of autonomic cardiovascular variability, representing the capability of the autonomic control to respond to stressors stimuli. Aging and diseases are associated with gradual decrease of TP, suggesting a loss of autonomic control to reliably counteract exogenous and endogenous stimuli [24].
Each oscillation can be described in terms of both frequency, expressed in Hz, and amplitude, which can be expressed both in absolute units (ms2 and mmHg2 for heart period and blood pressure time series, respectively) and normalized units (nu). Nu represent the relative value of LF and HF components with respect to the total power, and they are calculated dividing the component by total power to which VLF have been subtracted, i.e., LF nu = [LF absolute units / (total power – VLF power)] and the HF nu = [HF absolute units / (total power-VLF)]. The ratio between LF and HF power can be calculated and it is considered marker of the sympathovagal balance (see Figs. 5.2).
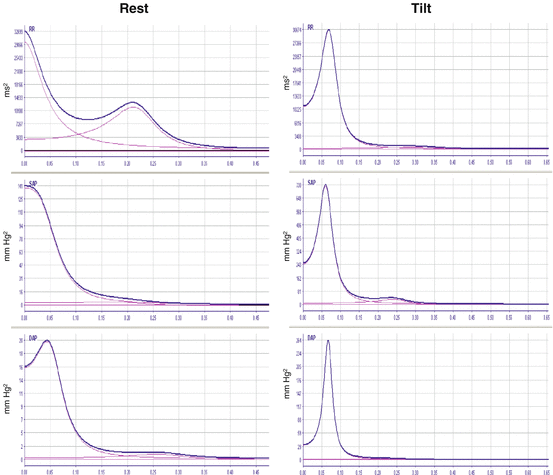
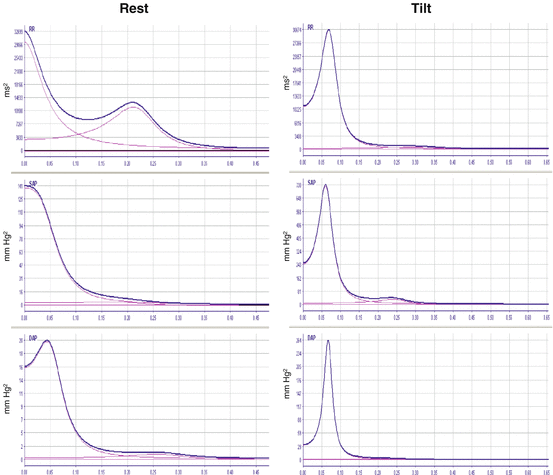
Fig. 5.2
Spectral analysis of heart rate and systolic and diastolic blood pressure during Rest (left column) and during Tilt (right column). During Tilt, LF component, marker of sympathetic modulation, significantly increases in both heart period and blood pressure time series compared to rest
5.2.3.2 Nonlinear Analysis
Nonlinear analysis of HRV can be considered complementary to spectral analysis for autonomic cardiovascular assessment. It has been hypothesized, and then demonstrated, that most of the biological system functions, including the cardiovascular one, are nonlinear, at least in specific situations. Therefore, the development of new techniques able to assess autonomic control in situations of nonlinearity became necessary for a reliable assessment of sympathetic and parasympathetic evaluation in health and diseases. In addition, we have to underline that ANS regulation is based on the interaction of subsystems at different time and space scales, acting in coordination and collaboration in order to control a certain variable, in the case that matters here, heart rate and blood pressure variations. This situation, which represents a physiological state, is characterized by a higher complexity, meaning that the system has good chances and flexibility to adapt itself to various stressors. On the contrary, when one of these systems becomes predominant on the others, meaning that this becomes the only system responsible for the regulation of that variable, the complexity of the system declines and the system becomes more rigid and less able to respond to stressor stimuli [8, 9, 14, 18, 31, 36].
It has been proposed that, among a biological signal, complexity and nonlinearity can be related to three main components: first, there are several subsystems which act, with feedback controls, in order to properly react and adapt to stressor stimuli; second, subsystems must adapt when conditions change, for instance, due to a pathological condition; third, in case one of the subsystem fails in control, other subsystems should act in order to compensate it.
Due to this conceptual important background, several different methods have been developed in order to assess nonlinear autonomic control in health and diseases and to evaluate the complexity of autonomic cardiovascular control using ad hoc algorithms. Namely, nonlinear analysis can be grouped into three families: fractal measure, such as power-law correlation and detrended fluctuation analysis, symbolic analysis, and, finally, complexity measures (such as Sample Entropy, Approximate Entropy, Multiscale Entropy, Shannon Entropy, Conditional Entropy, and Corrected Conditional Entropy). Although a mathematical description of these methods is beyond the scope of this chapter, we will briefly describe symbolic analysis, which can now be considered a complementary tool to linear spectral analysis in assessing autonomic cardiovascular control in health and diseases.
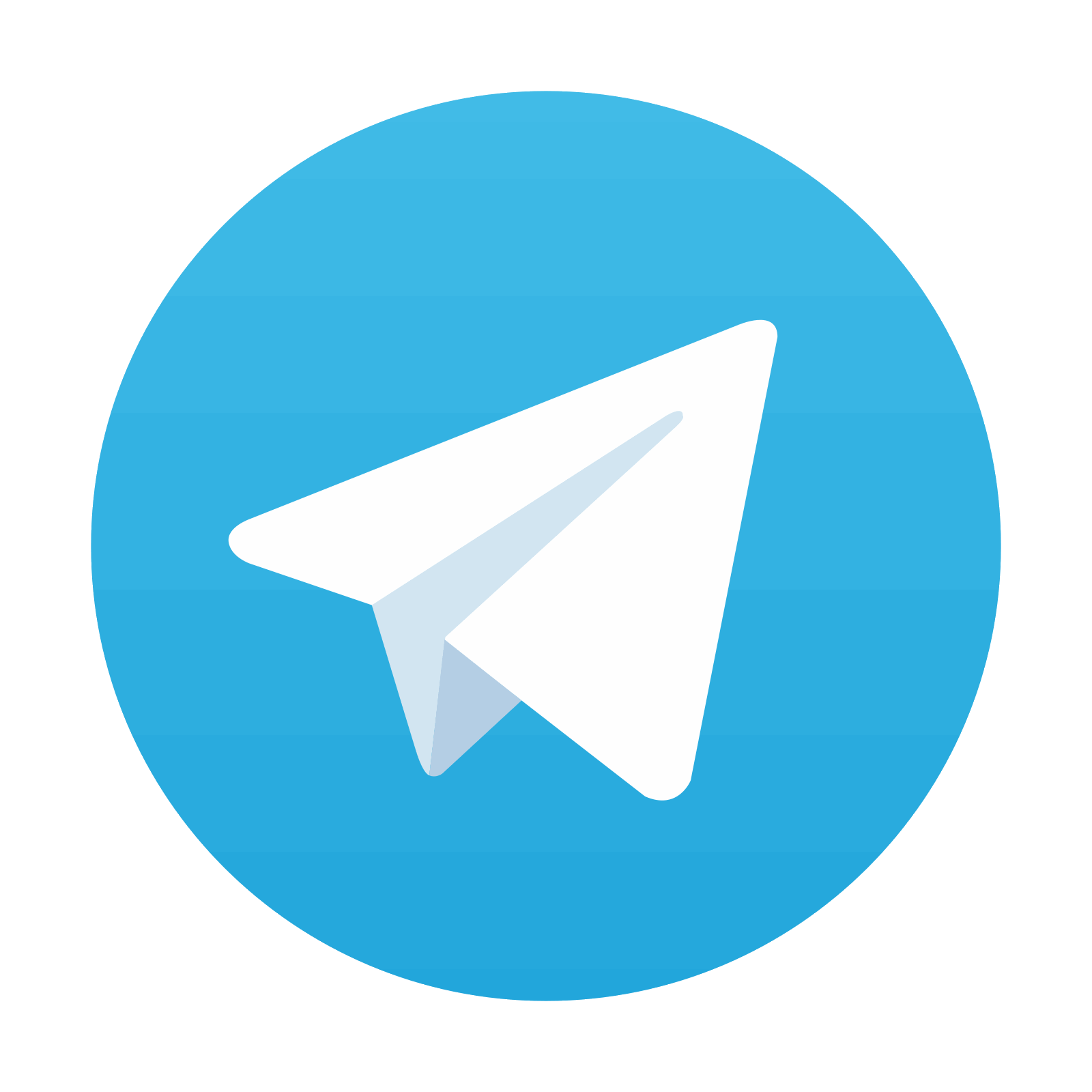
Stay updated, free articles. Join our Telegram channel

Full access? Get Clinical Tree
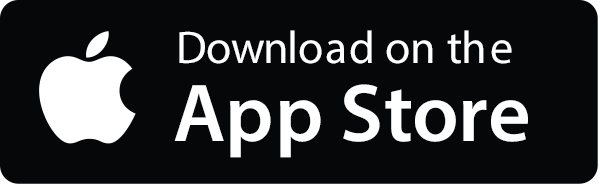
