Clinical trials have demonstrated cardiovascular benefits of sodium-glucose cotransporter 2 inhibitors (SGLT2i) and glucagon-like peptide-1 receptor agonists (GLP-1RA). However, their impact on all-cause and cause-specific hospitalization in real-world practice remains unclear. We identified patients with diabetes who initiated SGLT2i (n = 2,492), GLP-1RA (n = 1,982), or dipeptidyl peptidase-4 inhibitors (DPP4i, n = 2,492) between 2015 and 2018 in Geisinger Health System. We examined all-cause hospitalization (net benefit indicator) and cardiovascular disease (CVD) hospitalization (CV benefit indicator), as well as non-CVD hospitalization (harm indicator), using Cox proportional hazards regression. During a median follow-up of 16 months, SGLT2i and GLP-1RA were associated with lower risk of all-cause hospitalization (hazard ratio [HR] 0.85, 95% CI 0.75 to 0.95 for SGLT2i; HR 0.89, 95% CI 0.78 to 0.98 for GLP-1RA), as well as CVD hospitalization (HR 0.61, 95% CI 0.47 to 0.79) for SGLT2i; HR 0.77, 95% CI 0.60 to 0.99 for GLP-1RA) compared with DPP4i. The risks of all-cause and CVD hospitalization were similar between SGLT2i and GLP-1RA. SGLT2i was associated with substantially lower risk of myocardial infarction and heart failure hospitalization compared with DPP4i and lower risk of heart failure hospitalization compared with GLP-1RA. The risk of non-CVD hospitalization did not differ among the treatment groups. These results from real-world comparison further encourage SGLT2i and GLP-1RA use in routine diabetes care, particularly among patients at high risk of cardiovascular events.
Type 2 diabetes mellitus (T2DM) is a major public health concern around the world. Multiple clinical trials have shown cardiovascular protective effects of sodium-glucose cotransporter 2 inhibitors (SGLT2i) and glucagon-like peptide-1 receptor agonists (GLP-1RA). Hospitalization increases risk of death and other adverse outcomes and increases medical cost for patients with T2DM. , Although SGLT2i lowers the risk of hospitalization for heart failure (HF), , the impact of SGLT2i and GLP-1RA on all-cause hospitalization and cause-specific hospitalization is unclear. Potential adverse events of SGLT2i, such as diabetic ketoacidosis (DKA), lower extremity amputation, and Fournier’s gangrene, may result in increased risk of hospitalization. Better understanding of all-cause hospitalization, as well as cause-specific hospitalization by head-to-head comparison of commonly used antidiabetic medications will be informative to provide optimal therapies for patients with T2DM. In the present study, we examined the risk of all-cause hospitalization and cause-specific hospitalization by 3 newer classes of antidiabetic medications: SGLT2i, GLP-1RA, and dipeptidyl peptidase-4 inhibitors (DPP4i).
Methods
We used electronic health record data from the Geisinger Health System that serves 45 counties in central and northeastern Pennsylvania. The electronic health record data provide information on patient demographics, inpatient and outpatient encounters, outpatient prescriptions and laboratory results. Patients with diabetes who initiated SGLT2i, GLP-1RA, or DPP4i between 2015 and 2018, had at least 1 year of previous engagement with Geisinger system, were free of end-stage kidney disease and had at least 1 serum creatinine and hemoglobin A1c measurement 1 year before medication initiation ( t 0 ) were included. Diabetes was diagnosed using International Classification of Diseases diagnosis codes (250, E10, E11, or E13), prescription of antidiabetic medication (excluding conditions such as polycystic ovarian syndrome and gestational diabetes), hemoglobin A1c ≥7%, and at least 2 fasting glucose >7 mmol/L within 1 year. This study was approved by the Geisinger Medical Center Institutional Review Board and the Johns Hopkins University Institutional Review Board.
Prescriptions of SGLT2i, GLP-1RA, or DPP4i were identified from outpatient prescription records ( Supplementary Table 1 ). Patients were characterized by their initial prescription per an intention-to-treat approach. Patients who initiated more than 1 study medication were excluded. We did pair-wise comparisons among the 3 treatment groups.
The primary end point was all-cause hospitalization, an indicator of net benefit. Patients were followed from the first prescription date (T 0 ) until first hospitalization after T 0 , death, last encounter with Geisinger, or January 30, 2019, whichever came first. Secondary end points included cardiovascular disease (CVD) hospitalization and non-CVD hospitalization. CVD hospitalization, a CV benefit indicator, was defined as hospitalization due to myocardial infarction (MI), HF, or stroke. We also evaluated the risk of each individual component. Because severe adverse events of antidiabetic medications may lead to hospitalization, we treated non-CVD hospitalization as a potential harm indicator. Lastly, we explored the number of rare severe adverse events of SGLT2i including DKA, lower extremity amputation, and Fournier’s Gangrene during hospitalization. Cause of hospitalization was ascertained based on inpatient International Classification of Diseases codes ( Supplementary Table 2 ).
Covariates included were demographic characteristics, the calendar year of medication initiation, insurance status, smoking and drinking history, blood pressure, lab measurements, co-morbidities, concurrent use of medications, and number of outpatient encounters, and number of hospitalizations in the previous year (see Supplementary Methods for detailed definition). Approximately 35% of patients were missing at least 1 covariate, with urine albumin : creatinine ratio (25%) and body mass index (16%) being the most commonly missed. We used multiple imputation by chained equation to impute 40 datasets. Aforementioned patient characteristics, exposure, and outcomes were included in the imputation model.
Within each imputed dataset, we estimated the generalized propensity score of receiving each of the 3 treatments using multinomial logistic regression. Aforementioned patient characteristics were included in the model. Inverse probability of treatment weighting (IPTW) based on the propensity scores was applied to minimize differences in baseline characteristics. Estimated weights were truncated at 99% to prevent outliers from strongly affecting the analyses. Balance in covariates was evaluated using the standardized mean difference (SMD) before and after IPTW. A SMD below 0.1 indicates good balance. We further adjusted for covariates that did not achieve good balance in analyses. We randomly selected 1 imputed dataset to present characteristics of the study population.
Baseline characteristics of the study population were reported as number (percentage), mean ± standard deviation (SD), or median (interquartile interval), as appropriate. Before IPTW, we used the log rank test to compare unadjusted Kaplan-Meier curves between treatments. After multiple imputation and IPTW, we combined Kaplan-Meier estimators and hazard ratios (HRs) estimated from Cox proportional hazard regression in each imputed dataset using Rubin’s rules. We tested the proportional hazard assumption by checking Schoenfeld’s partial residuals.
We assessed whether the associations differed by prespecified patient characteristics, including baseline age (<60 vs ≥60 years), gender, baseline estimated glomerular filtration rate (eGFR) level (<60 vs ≥60 ml/min/1.73 m 2 ), albumin: creatinine ratio (<30, 30 to 300, or >300 mg/g), and history of CVD (CHD (coronary heart disease), stroke, or HF). We further stratified the analysis by history of CHD, stroke, and HF, separately in sensitivity analysis. To evaluate for potential residual confounding, we assessed risk of hospitalization due to severe bleeding as a negative control. We selected severe bleeding as an outcome thought to be unaffected by antidiabetic medication. A 2-sided p value <0.05 was considered statistically significant. Statistical analyses were performed with SAS 9.4 (SAS Institute, Cary, North Carolina) and R Foundation for Statistical Computing, Vienna, Austria ( http://www.R-project.org/ ).
Results
A total of 9,236 patients with diabetes from Geisinger met the inclusion criteria: 2,492 SGLT2i, 1982 GLP-1RA, and 4,762 DPP4i initiators between 2015 and 2018 ( Supplementary Figure 1 ). Before IPTW, SGLT2i users had, on average, a lower risk profile than DPP4i or GLP-1RA users ( Supplementary Table 3 ). After IPTW, the weighted mean ± standard deviation age was 58 ± 12 years in the SGLT2i group, 59 ± 14 years in the DPP4i group, and 58 ± 13 years in the GLP-1RA group ( Table 1 ). Good covariate balance was achieved for all covariates except the proportion of patients with eGFR <30 ml/min/1.73 m 2 in pair-wise comparison between SGLT2i versus DPP4i (SMD 0.1) and SGLT2i versus GLP-1RA (SMD 0.1) ( Supplementary Table 4 ). We further adjusted for eGFR categories in outcome analyses.
Variable | SGLT2i (n = 2,492) | GLP-1RA (n = 1,982) | DPP4i (n = 4,762) |
---|---|---|---|
Age (years) | 58 ± 12 | 58 ± 13 | 59 ± 14 |
Women | 1,167 (49%) | 957 (49%) | 2,320 (49%) |
White | 2,174 (91%) | 1,775 (91%) | 4,314 (91%) |
Body mass index (kg/m 2 ) | 36 ± 8 | 367 ± 8 | 36 ± 9 |
Systolic blood pressure (mm Hg) | 130 ± 15 | 129 ± 15 | 129 ± 15 |
HbA1c (%) | 8 ± 2 | 8 ± 2 | 8 ± 2 |
eGFR (ml/min/1.73 m 2 ) | 85 ± 23 | 84 ± 25 | 84 ± 25 |
eGFR categories (ml/min/1.73 m 2 ) | |||
≥90 | 1,150 (48%) | 923 (48%) | 2,205 (47%) |
60–89 | 840 (35%) | 667 (34%) | 1,621 (34%) |
45–59 | 262 (11%) | 205 (11%) | 507 (11%) |
30–44 | 122 (5%) | 112 (6%) | 306 (7%) |
<30 | 15 (1%) | 37 (2%) | 93 (2%) |
Urine albumin creatinine ratio (mg/g) | 12 [6, 40] | 12 [5, 42] | 12 [5, 46] |
ACR categories (mg/g) | |||
<30 | 1,674 (70%) | 1,347 (70%) | 3,227 (68%) |
30–300 | 559 (23%) | 462 (24%) | 1,191 (25%) |
>300 | 155 (7%) | 135 (6%) | 315 (7%) |
Atrial fibrillation | 182 (8%) | 149 (8%) | 382 (8%) |
Stroke | 169 (7%) | 155 (8%) | 382 (8%) |
Carotid atherosclerosis | 96 (4%) | 86 (4%) | 227 (5%) |
Peripheral artery disease | 81 (3%) | 71 (4%) | 159 (3%) |
Coronary heart disease | 519 (22%) | 441 (23%) | 1,059 (22%) |
Heart failure | 175 (7%) | 148 (8%) | 397 (8%) |
Acute kidney injury | 77 (3%) | 73 (4%) | 192 (4%) |
Charlson comorbidity index | 5 ± 3 | 5 ± 3 | 5 ± 3 |
Hospitalization in the prior year | |||
0 | 2,106 (88%) | 1,723 (89%) | 4,136 (87%) |
1 | 201 (8%) | 156 (8%) | 414 (9%) |
>1 | 83 (4%) | 65 (3%) | 182 (4%) |
Outpatient visits in the prior year, median [IQI] | 5 [3,9] | 5 [3,9] | 5 [3,9] |
Concurrent use of medication | |||
Insulin | 519 (22%) | 392 (20%) | 906 (19%) |
Sulfonylurea | 825 (35%) | 705 (36%) | 1,676 (35%) |
Metformin | 1,655 (69%) | 1,325 (68%) | 3,204 (68%) |
Thiazolidinediones | 75 (3%) | 54 (3%) | 138 (3%) |
Statins | 1,407 (59%) | 1,148 (59%) | 2,794 (59%) |
ACEI | 1,021 (43%) | 803 (41%) | 1,980 (42%) |
ARB | 377 (16%) | 307 (16%) | 751 (16%) |
Beta blocker | 734 (31%) | 614 (32%) | 1,500 (32%) |
Diuretics | 812 (34%) | 663 (34%) | 1,613 (34%) |
Antiplatelet medications | 972 (41%) | 773 (40%) | 1,929 (41%) |
Medication initiation year | |||
2015 | 461 (19%) | 387 (20%) | 933 (20%) |
2016 | 506 (21%) | 442 (23%) | 1,032 (22%) |
2017 | 674 (28%) | 519 (27%) | 1,314 (28%) |
2018 | 748 (32%) | 596 (31%) | 1,453 (30%) |
Insured | 2,072 (87%) | 1,685 (87%) | 4,115 (87%) |
Smoker | |||
Never | 1,039 (43%) | 845 (43%) | 2,124 (45%) |
Past | 946 (40%) | 772 (40%) | 1,833 (39%) |
Current | 405 (17%) | 327 (17%) | 776 (16%) |
Alcohol drinker | 994 (42%) | 800 (41%) | 1,921 (41%) |
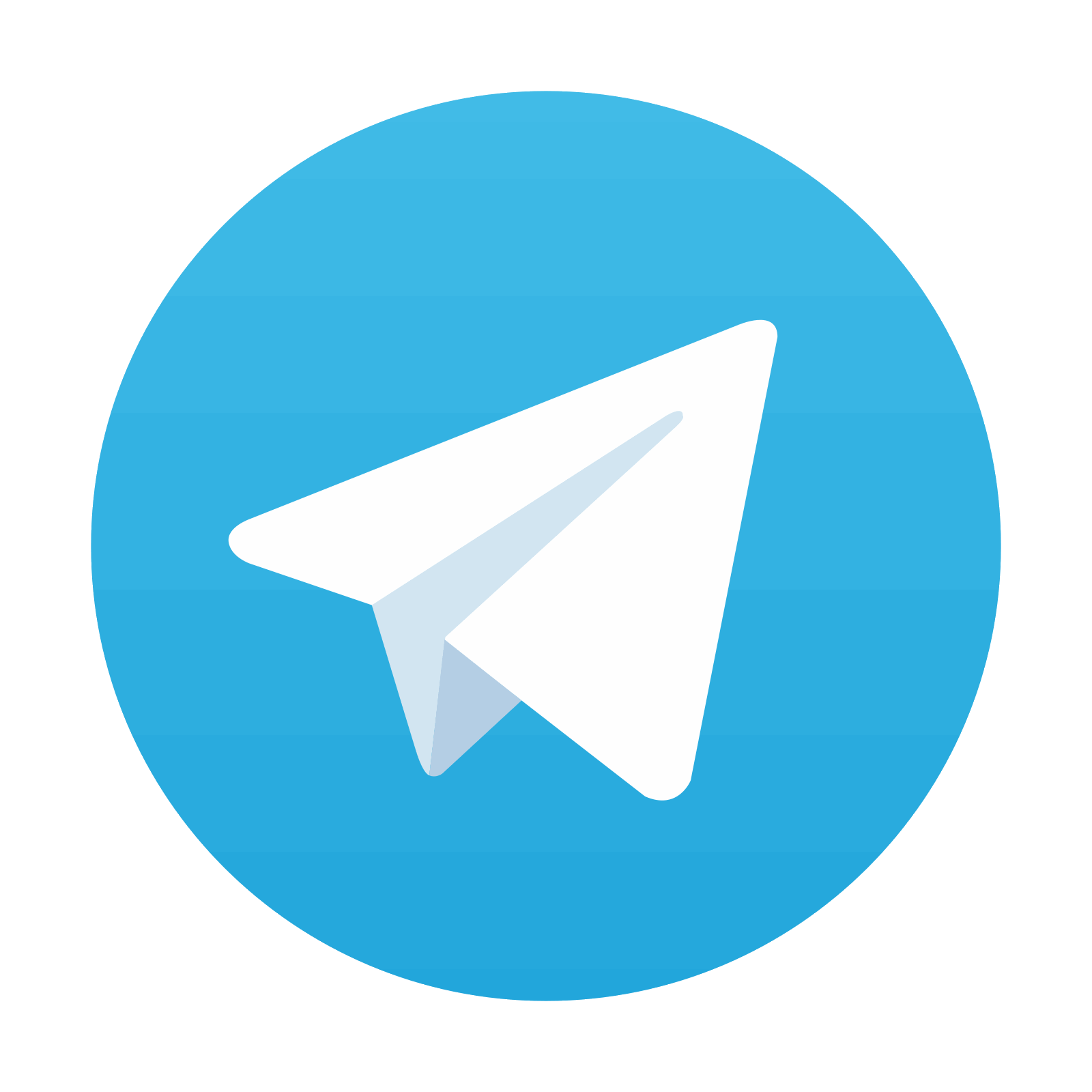
Stay updated, free articles. Join our Telegram channel

Full access? Get Clinical Tree
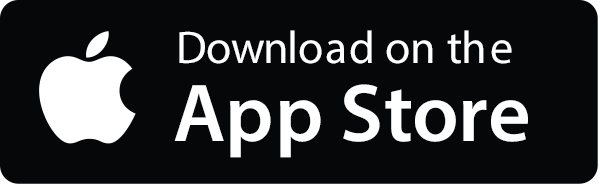

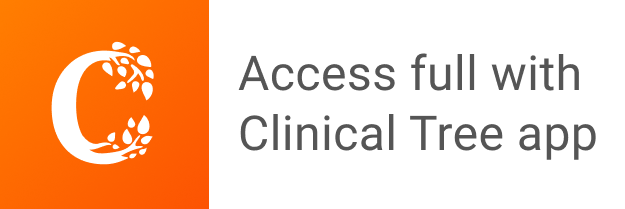