The field of interventional cardiology has evolved since the introduction of balloon angioplasty by Andreas Gruntzig,1 with current high procedural success rates and low attendant periprocedural complications.2 Percutaneous coronary intervention (PCI) and transcatheter aortic valve replacement (TAVR) are now preferred in many high-risk subgroups in whom they were previously contraindicated.3-7
Evaluation of risk-benefit ratios and risk stratification are important elements in optimizing care for an individual undergoing PCI or TAVR. Various models for prediction of risks can help physicians, patients, and their families better comprehend attendant risks and provide an objective basis for the most suitable treatment option. It is paramount for clinicians to become familiar with the available risk scores and apply them in clinical practice. This chapter focuses on the strengths and weaknesses, ease of applicability, and use of risk prediction models to estimate the risk of mortality and major adverse cardiac events (MACE) in the current environment of interventional cardiology.
A good predictive model should be accurate and able to discriminate between different levels of risk. An accurate model, on average, is not biased toward over- or underprediction. If the true average risk of an event in a population is 5%, one could achieve accuracy by predicting 5% risk for every patient. Such estimates would lack precision, however, because the same prognosis is given for both low- and high-risk patients. Discriminatory ability is related to precision (distinguishing high- from low-risk patients).
In the context of this chapter, a “prediction” is the probability that an event will occur. A high-risk patient may have a predicted risk of 0.50, but he or she will not suffer a half-event. This may be why assessment of these models tends to focus more on discriminatory ability than accuracy.
Logistic regression is the standard statistical analysis employed for binary outcomes.8 Harrell and colleagues9 recommend that the number of explanatory variables considered should not exceed one-tenth of the number of events. This set of variables should be determined without knowledge of their relationship to the outcome in the modeling data set. Clinical expertise is essential at this stage of model development. After candidate explanatory variables are chosen, the final model may be determined using automatic selection9 or bootstrap10 methods. These methods are intended not only to simplify the model, but also to avoid overfitting. Overfitting occurs when a model reflects anomalous associations specific only to the model-building data, resulting in suboptimal performance in other data sets.
Once a final model is chosen, the Hosmer-Lemeshow goodness-of-fit test may be used to determine if the model adequately reflects the observed data.11 A significant test result indicates an inadequate fit. Accuracy may be internally validated using data-splitting, cross-validation, or bootstrap methods.12 However, external validation is more valuable than these methods. Comparison of the observed number of events in the external data set against the events predicted by the model determines the model accuracy.
The discriminatory ability of a model may be quantified by the c-statistic (c stands for concordance).13 The c-statistic, or area under the receiver operating characteristic (ROC) curve, is the proportion of times that the model correctly ranks the risks for a pair of subjects. That is, if 2 patients are selected, only 1 of whom will have an event, it is the rate at which the model assigns a higher level of risk to the patient with the event. Thus, a c-statistic of 0.50 indicates the model performs as well as assigning risk by the toss of a coin. Perfect discrimination results in a c-statistic of 1.00.
The simplification of the statistical model to an additive integer-scoring tool may be useful for patient counseling. The goal is to assign integer coefficients to risk factors significantly associated with an event so that the physician may quickly sum the coefficients for a numerical ranking of the patient’s risk. Once the logistic regression model is finalized, the simplified scoring tool may be defined by selecting integer coefficients roughly proportional to the log-odds ratio coefficients (ie, parameter estimates) of the logistic regression model variables.
RECENT RISK MODELS PREDICTING COMPLICATIONS FOLLOWING PERCUTANEOUS CORONARY INTERVENTIONS
Multiple models have been developed that incorporate demographic features, existing comorbidities, and angiographic characteristics to predict in-hospital and long-term outcomes of patients undergoing PCI. Of these, the Mayo Clinic Risk Score, American College of Cardiology National Cardiovascular Data Registry (ACC-NCDR) CathPCI risk score, and SYNTAX (Synergy Between Percutaneous Coronary Interventions with Taxus and Cardiac Surgery) score models are the most commonly applied in practice.14-16 These models are usually used in synergy and complement prognostic information; the Mayo Clinic and NCDR CathPCI scores stratify patient risk based on bedside clinical variables and PCI urgency (Table 58-1),14,15,17 while the SYNTAX score can further refine the overall risk and success of PCI based on the angiographic complexity and extent of coronary artery disease (CAD).16,18 Nine models that predict the risk of in-hospital and 30-day mortality and MACE have been described in the recent literature. They are listed below and compared in Table 58-1 and Table 58-2.
ACC-NCDR22 | NY State19,20 | NNE Model23 | Michigan24 | Beaumont Hospital25 | Cleveland Clinic26 | Mayo Clinic27 | Brigham and Women’s31 | |
---|---|---|---|---|---|---|---|---|
Risk(s) studied | Mortality | Mortality | Mortality | Mortality | Mortality | Mortality, Q-MI, emergent CABG | Mortality, Q-MI, emergent CABG, stroke | Mortality, MI, CABG |
Study time period | Jan 98-Sep 00 | Jan 91-Dec 94 | Jan 94-Dec 96 | Jul 97-Sep 99 | Jan 96-Dec 98 | Jul 94-Dec 94 | Jan 96-Dec 99 | Jan 97-Feb 99 |
Sample size (model development) | 50,123 | 62,670 | 15,331 | 10,729 | 9954 | 12,985 | 5463 | 1877 |
Event rate (%) | 1.4 | 0.9 | 1.1 | 1.6 | 1.4 | 4.5 | 4.0 | 6.4 |
Area under ROC (c) (modeling data set) | 0.89 | 0.892 | 0.88 | 0.90 | 0.87 | 0.648 | 0.782 | 0.767 |
Internal validation | c = 0.89 | NA | c = 0.88 ± 0.02 | c = 0.92 | c = 0.87 | c = 0.635 | c = 0.755 | 0.742 |
Validation set (n = 50,130) | Bootstrapping | Validation set (n = 5863) | Validation set (n = 12,005) | Cross-validation corrected | Validation set (n = 1781) | Validation set (n = 1460) | ||
Simplified scoring tool | No | No | No | Yes | Yes | No | Yes | Yes |
Long-term mortality assessment | No | Yes, on external data7 | No | No | No | No | No | No |
Operator volume included in the risk score | No | No | No | No | No | No | No | No |
Mortality | Complications | |||||||
---|---|---|---|---|---|---|---|---|
Event | ACC-NCDR22 | NY State19,20 | NNE23 | Michigan24 | Beaumont25 | Cleveland Clinic26 | Mayo Clinic27 | Brigham and Women’s31 |
Age | ||||||||
By decade | 1.83 | 1.37 | ||||||
Logarithm | 24.9 | |||||||
≥75 y | 1.35 | |||||||
>65 y | 1.95 | |||||||
50-59 y | 2.61 | 0.93 | 1.00 | |||||
60-69 y | 3.75 | 1.63 | 1.00 | |||||
70-79 y | 6.44 | 3.32 | 2.24 | |||||
≥80 y | 11.3 | 3.72 | 2.65 | |||||
LV function | ||||||||
50%-59% (EF) | 1.00 | 1.00 | 2.53 | 1.00 | ||||
40%-49% | 0.87 | 1.00 | 3.32 | 1.66 | ||||
30%-39% | 0.99 | 1.49 | 5.16 | 1.66 | ||||
20%-29% | 2.04 | 1.49 | 5.16 | 1.66 | ||||
10%-19% | 3.43 | 3.68 | 5.16 | 1.66 | ||||
<10% | 3.93 | 3.68 | 5.16 | 1.66 | ||||
CHF | 2.38 | 3.01 | 2.11 | 3.76 | ||||
(NYHA ≥III) | (NYHA ≥III) | |||||||
Acuity of presentation | ||||||||
Urgent PCI | 1.78 | 2.19 | 2.13 | |||||
Emergent PCI | 5.75 | 7.71 | 2.13 | 1.44 | ||||
AMI 1-7 d | 2.10 | 1.85 | 2.14 | 3.15 | ||||
AMI 6-23 h | 1.31 | 3.67 | (primary therapy) | 2.80 | (<14 d) | 4.75 | 3.15 | |
AMI <6 h | 1.31 | 5.22 | 2.80 | 4.75 | 3.15 | |||
Cardiogenic shock | 8.49 | 18.3 | 6.10 | 11.5 | 12.7 | 4.95 | 3.47 | |
IABP use | 1.68 | 2.39 | 3.91 | |||||
High-risk angiographic features | ||||||||
2-VD | 1.82 | 1.54 | 2.20 | 1.32 | 1.86 | |||
3-VD | (multivessel intervention) | 2.37 | 2.20 | 1.74 | 1.86 | |||
LM disease | 2.04 | 4.34 | 2.40 | |||||
(LM treated) | ||||||||
Thrombus | 1.67 | 1.90 | ||||||
ACC/AHA B2 | 1.63 | 2.58 | ||||||
ACC/AHA C | 1.94 | 2.66 | 2.58 | |||||
SCAI II | 1.64 | |||||||
SCAI III | 1.87 | |||||||
SCAI IV | 2.11 | |||||||
Other high-risk clinical features | ||||||||
Renal failure | 3.04 | 3.51 | 2.32 | 5.5 | 2.06 | 2.41 | 1.54 | |
PVD | 1.78 | 2.12 | 1.57 | 3.21 | ||||
Diabetes mellitus | 1.41 | 1.41 | 1.54 | |||||
Female sex | 1.31 | 1.82 | 3.57 | 1.82 |
New York (NY) State model19-21
American College of Cardiology-National Cardiovascular Data Registry (ACC-NCDR)15,22
Northern New England (NNE)23
Michigan Consortium24
William Beaumont Hospital25
Cleveland Clinic26
Brigham and Women’s Hospital21
Toronto Score28
In general, these risk models have good predictive capability (c-statistic, approximately 0.9). Their discrimination ability is reduced in high-risk populations. A recently described enhanced NCDR CathPCI model is effective in determining survival in patient undergoing urgent PCI with high-risk clinical features, such as cardiogenic shock or cardiac arrest. Based on the model, survival to hospital discharge after urgent PCI was 35% for patients with sustained shock and cardiac arrest, whereas patients who had transient shock without cardiac arrest had 85% chance of survival.17
All the models incorporate similar demographic features, existing comorbidities, and lesion variables (with the exception of NY State) (see Table 54-2). In general, the risk factors associated with in-hospital complications can be divided into the following broad categories: age, left ventricular function, acuity of presentation, high-risk angiographic features, and additional high-risk demographics, such as congestive heart failure, New York Heart Association class, renal failure, diabetes, chronic obstructive pulmonary disease, peripheral vascular disease, and other variables.
Advancing age is an important risk factor for PCI complication and is universally present in the risk models. Possible explanations for increased risk include reduced cardiac reserve, presence of multivessel disease, and increased prevalence of comorbidities.29,30 In all the risk scores, there was a graded increase in the mortality risk with advancing age. Thus, an octogenarian has 2.7 times the risk (Michigan model) as compared with patients between 50 and 59 years of age. The Mayo Clinic risk and NY state risk scores extend these observations to composite end points, with an unadjusted odds ratio for these complications of 6.03 and 4.7, respectively, in a person older than 80 years of age who undergoes PCI, as compared with a younger patient. Age was considered a binary variable in a recent simplified scoring system.25,31 This dichotomous cutoff of age might underestimate risks from PCI in the very elderly.
Assessment of left ventricular function is an important variable with prognostic implications during PCI. Various models have measured this risk variable differently. Cardiogenic shock resulting from severe left ventricular systolic dysfunction is the single most important predictor of PCI complications.3 The mortality in patients presenting with shock in various risk scores ranged from 28% (ACC-NCDR) to 33% (Michigan).22,24 In the Cleveland and Mayo Clinic models, the odds ratios of in-hospital complications in patients with shock were 12.7 and 4.95, respectively. Moreover, in the enhanced NCDR CathPCI model, sustained shock is associated with higher unadjusted in-hospital mortality than transient shock (63.3% vs 15.26%, respectively).17 In the NY State model, reduced ejection fraction and congestive heart failure were independent predictors of death during or after angioplasty. Increased risk of death was associated with patients presenting with New York Heart Association (NYHA) class greater than or equal to III in the Mayo model, in the NCDR CathPCI risk model, and in the NNE cardiovascular study group, with an ejection fraction of 40% of less. Similar conclusions were derived from other risk score models. Therefore, it is important to have clinical or objective assessment of left ventricular function at the time PCI is performed.
Acute myocardial infarction (especially within 24 hours), cardiac arrest, hemodynamic instability with cardiac arrhythmia, and cardiogenic shock are important clinical variables that influence the acuity of presentation and adversely affect the outcome of the procedure. In the NCDR CathPCI database, the overall in-hospital mortality after PCI was 1.27%, ranging from 0.65% in nonurgent PCI to 4.81% in ST-segment elevation myocardial infarction (STEMI).15 Use of intra-aortic balloon pump during an urgent procedure is an important marker for increased mortality risk as seen in the NY State, ACC-NCDR, and NNE models. The odds ratio estimate for complications in urgent or emergent procedures in the Mayo Clinic risk score was 2.13. Similarly, mortality with emergent PCI procedures was high in the other study populations, with rates between 5.8% and 6.35% in the ACC-NCDR and NNE groups, respectively.
Multivessel disease had a higher odds ratio for complications during PCI: 1.54 per extra vessel in the Michigan model, 1.86 in the Mayo Clinic model, and 1.32 per extra vessel in the Cleveland Clinic model. Multivessel angioplasty was a risk factor in the NY State model (odds ratio, 1.82) and can influence the prognosis of the patient.32 Other angiographic risk factors of importance are presence of thrombus (Mayo Clinic and Michigan models), American College of Cardiology/American Heart Association (ACC/AHA) type C lesion classification (ACC-NCDR, NNE, and Cleveland Clinic models), and left main coronary artery disease (ACC-NCDR and Mayo Clinic models) or left main intervention (Brigham and Women’s model).
Renal failure has been found to be an important predictor of in-hospital complications following PCI.33,34 The Michigan model had an odds ratio of 5.5 for mortality in patients undergoing PCI who have a creatinine level of more than 1.5 mg/dL. Similar findings were noted in all other models except the Cleveland Clinic model. Despite differences in the definition of renal failure, the presence of renal insufficiency clearly increases the risk of complications during PCI. Assessment of renal function before PCI not only helps in prevention and management of contrast-associated acute kidney injury, but also adds this variable to the profile of the patient.
Peripheral vascular disease is linked to higher mortality with the NNE, Michigan, and Beaumont models. Similar observations were noted in the NY State model (the odds ratio for mortality in patients with femoral popliteal disease was 1.775). This is an important diagnosis and is readily available from history and clinical examination. Peripheral vascular disease is not clearly defined in most of the risk scores; however, femoral popliteal disease (NY State model), claudication, amputation, peripheral bypass surgery, angioplasty, aortic aneurysm, and history of transient ischemic attacks, stroke, or carotid stenosis (Michigan model) add to the risk of the patient undergoing PCI.
Although not included in the previously mentioned risk models, the role of frailty has recently been recognized because frail patients have poor in-hospital and long-term outcomes. Frailty can help estimate the biological age and clinical vulnerability of elderly patients. Frail patients have an odds ratio for in-hospital mortality of 4.6 after urgent PCI.35 Adding frailty to the Mayo Clinic Risk Score improved the discriminability for adverse long-term outcomes (mortality and myocardial infarction) following PCI.36 Frailty is increasing recognized as an important variable that determines not only eligibility but also prognosis of elderly subjects evaluated for high-risk percutaneous procedures, such as TAVR. There is still no consensus on the definition; however, uniform evaluation with existing definitions is strongly encouraged, especially as the age of the patients referred to cardiac catheterization laboratory continues to increase. In the PARTNER trial, for example, frailty was assessed using a composite of 4 markers (serum albumin, dominant handgrip strength, gait speed, and Katz activity of daily living survey), which were combined into a frailty score.37
Other predictors (eg, diabetes mellitus [NY State and Beaumont models] and female sex [NY State, Cleveland Clinic, Michigan, and Beaumont models]) are not consistent across all the risk models and have a generally lower, weaker relationship with procedural complications.
AMERICAN COLLEGE OF CARDIOLOGY/AMERICAN HEART ASSOCIATION LESION AND SYNTAX CLASSIFICATIONS
Percutaneous angioplasty techniques have evolved rapidly since the original proposal of this classification in 1986 and its subsequent modification in 1990.38,39 It is still the most widely used system to scorecard the individual operator and the institution. More recently, in a new classification system, 2 variables (nonchronic total occlusion and degenerated saphenous vein grafts) were significantly correlated with death, non–Q-wave myocardial infarction, and the need for emergency coronary artery bypass grafting (CABG).40 The Society for Cardiac Angiography and Interventions (SCAI) proposed a simpler classification, collapsing ACC/AHA lesions A, B1, and B2 lesions into non-C category, and then stratifying the lesion by patency (SCAI I = non-C/patent; SCAI II = C/patent; SCAI III = non-C/occluded; SCAI IV = C/occluded), resulting in better, but modest, discrimination (c-statistic, 0.665) for prediction of major in-hospital complications as compared with ACC/AHA lesion classification (c-statistic, 0.624).41 ACC/AHA type A is associated with a 2% risk of complications, whereas the risk of complications increases to 21% with type C lesions.
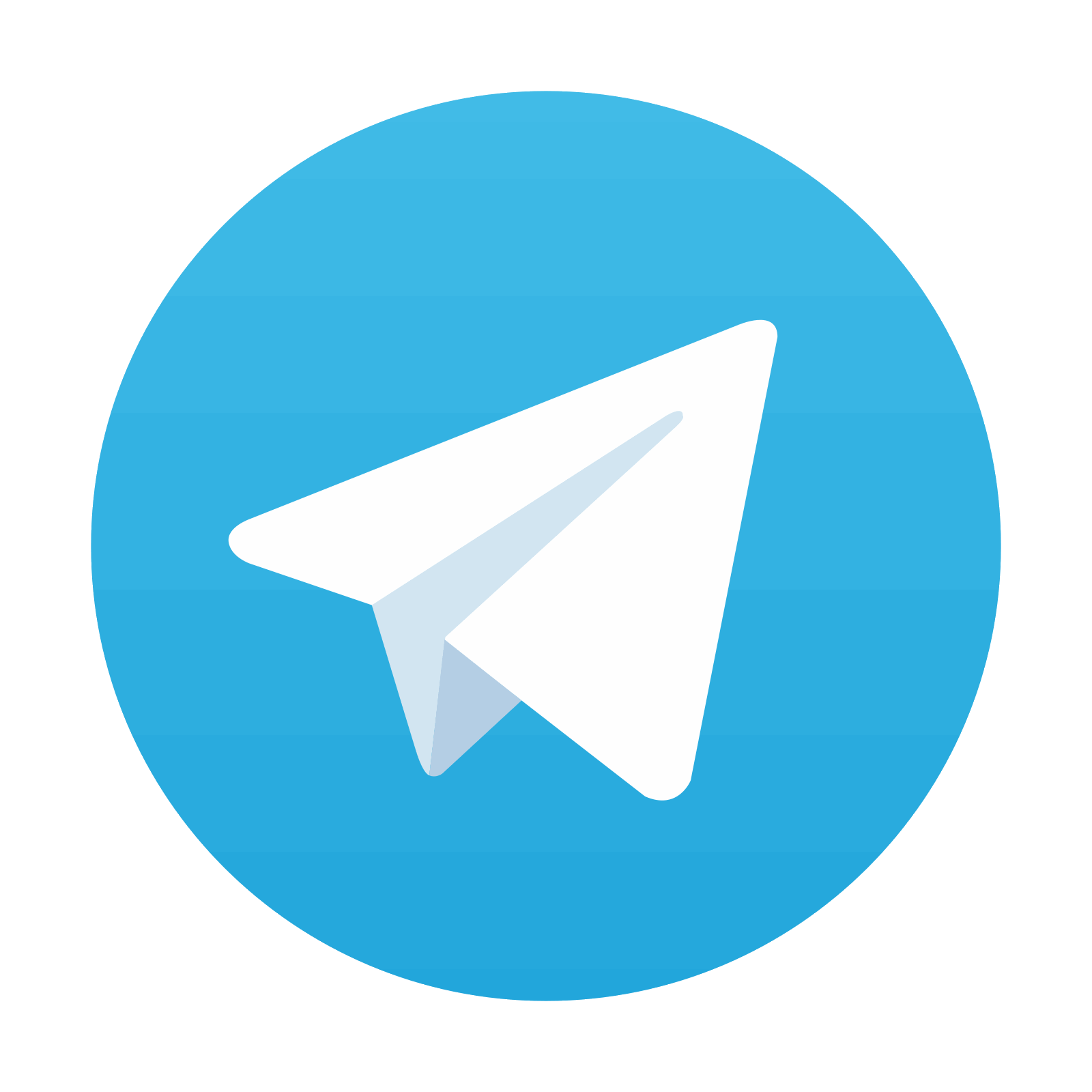
Stay updated, free articles. Join our Telegram channel

Full access? Get Clinical Tree
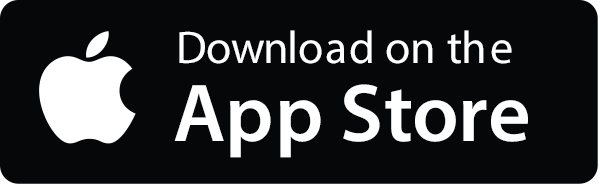
