Assessing risk to improve outcomes is not a new principle. Formal assessment of patient care to improve outcomes dates back millennia. While it may be hard to establish an exact beginning, there are historical times and iconoclastic health-care providers who stand out as important contributors to the modern concepts of quality assessment and performance improvement. At least six individuals stand out as iconoclasts in the field of performance improvement in surgery (Table 7-1). From the practice-changing observational studies of medieval surgeons like Albucasis and Trotula to the twentieth century insistence on evidence-based studies and randomized controlled trials (RCTs) by Archie Cochrane, many individuals served as champions of performance improvement using the tools of their times. The six people outlined in Table 7-1 had exceptional effects on differentiating good from bad outcomes and on implementing process improvements that benefit our patients today. Each of these six shared a common life experience. At some time in their careers, they were outcasts among their peers. Their peers, and in some cases the public, chastised them for stating the obvious. Thankfully, they persisted despite these unpopular reactions. Reading the historical accounts of these six individuals reminds one that today’s public resistance to straight-forward observations is a long-standing perpetual obstacle that surgical leaders overcame.
Health-care Provider (Surgeon, Midwife, or Nurse) | Time Period | Contributions |
---|---|---|
Albucasis of Andalusia | Around 900 A.D. |
|
Trota of Salerno | Around 1200 A.D. |
|
Angelique du Coudrey of France | Mid 1700s |
|
John Hunter (England) | Late 1700s |
|
Florence Nightingale (England) | Early 1800s |
|
Ernest Amory Codman (United States) | Early 1900s |
|
Archie Cochrane (Scotland) | Mid-twentieth Century |
|
In the early 1960s, Donabedian suggested that quality in health care is defined as improvement in patient status after accounting for the patient’s severity of illness, presence of comorbidity, and the medical services received.1 He further proposed that quality could best be measured by considering three domains: structure, process, and outcome. Only recently has the notion of measuring health-care quality using this Donabedian framework been accepted and implemented. In 2000, the Institute of Medicine (IOM) issued a report that was highly critical of the US health-care system, suggesting that between 50,000 and 90,000 unnecessary deaths occur yearly because of errors in the health-care system.2 The IOM reports created a heightened awareness of more global aspects of quality. For most of the history of cardiac surgery an outcome measure, operative mortality, defined surgical quality. After the IOM report appeared, change occurred, and other aspects of Donabedian’s framework surfaced to measure quality. In addition to operative mortality, health-care quality measurement gave way to a broader analysis that included additional performance measures including operative morbidity, processes of care, and structural measures of care. The Joint Commission on Accreditation of Healthcare Organizations (formerly JCAHO now officially called The Joint Commission) proposed the following definitions of important quality measures:
Performance measure—A quantitative entity that provides an indication of an organization’s or surgeon’s performance in relation to a specified process or outcome.
Outcome measure—A measure that indicates the results of process measures. Examples are operative mortality, the frequency of postoperative mediastinitis, renal failure, and myocardial infarction.
Process measure—A measure that focuses on a process leading to a certain outcome. Intrinsic in this definition is a scientific basis for believing that the process will increase the probability of achieving a desired outcome. Examples include the rate of internal mammary artery (IMA) use in coronary artery bypass graft (CABG) patients or the fraction of CABG patients placed on anti-platelet agents postoperatively.
Structural measure—A measure that assesses whether an appropriate number, type, and distribution of medical personnel, equipment, and/or facilities are in place to deliver optimal health care. Examples include enrollment in a national database, adequate intensive care unit (ICU) facilities, or procedural volume.
Birkmeyer and coworkers outlined advantages and disadvantages associated with each of these three specific types of performance measures.3 The fact that structural measures can be readily tabulated in an inexpensive manner using administrative data is a distinct advantage. On the other hand, many structural measures do not lend themselves to alteration. Particularly in smaller hospitals, there may be no way to increase procedural volume or to introduce costly ICU design changes in an attempt to improve their performance on structural measures. Attempts to alter structure might even have adverse consequences (eg, unnecessary operations, costly and unnecessary new beds). Links between health-care quality and process measures exist and they are usually actionable on a practical level. Their major disadvantage lies in the fact that their linkage to outcomes may be weak. Although outcome measures are the most important endpoint for patients, inadequate sample size and lack of appropriate risk-adjustment limit accurate assessment of these outcomes.
Several national organizations develop and evaluate performance measures. Perhaps the most visible of these is the National Quality Forum (NQF), a public-private collaborative organization that uses a process of exhaustive, evidence-based scrutiny of candidate measures to determine their relevance to both patients and healthcare providers. The NQF considers whether candidate measures can be assessed accurately and whether actionable interventions can improve performance for these measures. Because of this scrutiny, NQF-endorsed measures have a high level of national credibility (Table 7-2).
NQF endorsed adult cardiac surgery measures |
Composite Measure |
1. STS CABG Composite Score composed of six outcome measures and five process measures. |
Outcome Measures |
2. Risk-Adjusted Deep Sternal Wound Infection Rate. |
3. Risk-Adjusted Operative Mortality for Aortic Valve Replacement (AVR). |
4. Risk-Adjusted Operative Mortality for AVR + CABG Surgery. |
5. Risk-Adjusted Operative Mortality for CABG. |
6. Risk-Adjusted Operative Mortality for Mitral Valve (MV) Repair. |
7. Risk-Adjusted Operative Mortality for MV Repair + CABG Surgery. |
8. Risk-Adjusted Operative Mortality for MV Replacement. |
9. Risk-Adjusted Operative Mortality for MV Replacement + CABG. |
10. Risk-Adjusted Postoperative Renal Failure. |
11. Risk-Adjusted Prolonged Intubation (Ventilation). |
12. Risk-Adjusted Stroke/Cerebrovascular Accident. |
13. Risk-Adjusted Surgical Re-exploration. |
Process Measures |
14. Anti-Lipid Treatment at Discharge. |
15. Anti-Platelet Medication at Discharge. |
16. Beta Blockade at Discharge. |
17. Duration of Antibiotic Prophylaxis for Cardiac Surgery Patients. |
18. Preoperative Beta Blockade. |
19. Selection of Antibiotic Prophylaxis for Cardiac Surgery Patients. |
20. Use of Internal Mammary Artery in CABG. |
Structural Measure |
21. Participation in a Systematic Database for Cardiac Surgery. |
*http://www.sts.org/quality-research-patient-safety/quality/quality-performance-measures. |
Other outcomes following cardiac procedures, such as patient satisfaction and health-related quality of life, are less well studied but extremely important in the assessment of performance. Meeting or exceeding patients’ expectations is a major goal of the health-care system. While all recognize the importance of performing safe surgery, one must also acknowledge that a safe operation with minimal patient benefit is to be avoided. The growing importance of patient-reported outcomes (PRO) also reflects the increasing prevalence of chronic disease in our aging population. The goal of therapeutic interventions is often to relieve symptoms and to improve quality of life, rather than cure a disease and prolong survival. This is especially important in selecting elderly patients for cardiac operations. Recent studies suggest that CABG results in excellent health-related quality of life 10 to 15 years following operation in most patients,4 and that this benefit extends to those patients older than 80 years of age.5 Future research into patient-perceived performance assessment is inevitable given the aging of populations in the developed countries.
Statistical models to adjust for patient risk are essential to determine the probability of procedural outcomes. Traditionally, risk models predict the probability of death or other postprocedural complications such as stroke, infection, or renal failure. These traditional models are clearly important to assess procedural risk, but the safety of an operation is only part of the decision-making process as to whether to recommend a given procedure. Patient benefit must be considered as well. Just because one can do a procedure safely does not mean it should be done—if it affords the patient only minimal benefit, then the patient has received poor treatment.
Patient benefit can be represented by using appropriate metrics other than procedural mortality and morbidity. PRO can be objectively determined from a variety of published scoring protocols. These scores can be considered to be an objective measure of patient benefit. In turn, the scores provide information necessary to develop statistical models that predict the probability of PRO scores, in much the same way that traditional models predict the probability of procedural complications. The results of these models should serve as a meaningful measure of predicted patient benefit. Clinical registries are now collecting data that will permit an objective estimate of both patient risk and patient benefit. Statistical risk models should soon be available to predict not only procedural mortality and major non-fatal complications, but also the probability of patient benefit.
The best approach to assessing surgical quality is uncertain. Operative mortality for most cardiac procedures is low and performance assessment requires large patient numbers to discriminate between hospitals and providers. For this reason other measures surface for performance assessment. There is growing interest in the use of composite measures to assess provider and hospital performance. Composite measures combine multiple quality indicators into a single score and can include outcome, structural, and process measures in various combinations. Evidence suggests that composite variables that incorporate multiple outcome measures as well as structural and process performance measures are better reflections of quality than are individual outcomes alone.6 Examples of composite performance measures used for quality assessment include indices that combine ICU care variables with outcome measures,7 and those that combine process of care variables with outcome variables and structural variables.8,9
Certain outcome variables may reflect multiple domains of performance. One such measure is failure-to-rescue, usually defined as the mortality rate for a subset of patients who experience postoperative complications. Studies suggest that failure-to-rescue rates depend on structural measures (eg, advanced ICU capabilities and presence of residency training), processes of care, and traditional morbidity outcome measures.10,11 While traditional outcome measures such as operative mortality are thought to be mostly dependent on patient-related risk factors, it appears that failure-to-rescue rates reflect a different part of the care continuum, specifically the intensive care arena. Some consider failure-to-rescue rates to be primarily a structural measure focused on nursing care and ICU staffing. There are multiple contributions to failure-to-rescue rates, thereby qualifying this variable as a composite performance measure. Several studies are underway to determine the ability of this variable to identify and stratify quality performers.
The development of composite measures is statistically complex. The composite metric is very dependent on the statistical approach used to combine the component variables.8 Despite the complexities of creating composite measures, increased reliance on composite measures of quality is inevitable, and efforts at improving the reliability and predictive accuracy are underway.
Analysis of cardiac surgical results is purpose-driven. Use of statistics follows the purpose for which data were gathered. There are three broad uses of statistics for analysis of cardiac surgical outcomes. Perhaps the most basic use of statistics is to recap or summarize information about a patient group. Certain reports can only document surgical outcomes in a few patients because of the rarity of disease or limited ability to sample patient populations. A single estimate of an outcome in a limited population may be misleading. In order to acknowledge the inaccuracy of a single estimate of a limited population, statistical measures describe this imprecision. Multiple statistical terms describe this uncertainty, including sample, mean, standard deviation, interquartile range, confidence interval, and standard error.
More commonly, statistics serve to relate or compare attributes of two or more groups. Comparisons between groups require comparisons between known reference distributions (eg, chi-square and t-distribution) and the data sample distribution. Differences between these two distributions allow calculation of a probability that there really is a difference between the sample and the reference distribution. This probability has different names including p-value or alpha (α) level. The most commonly used test for comparisons of two numeric variables is the Student’s t-test. The t-test makes use of the symmetry of the t–distribution of a sample and compares the t statistic calculated from each of the two group’s t–distributions with critical values. The astute reader quickly realizes that the statistical formulation of the t-test requires computer support as the workhorse that does the calculations. In fact, very few statistical tests do not rely on computer-intensive calculations. Reliance on computers to calculate p-values, and to perform statistical tests in general, provides opportunity for misuse or inaccurate use of both simple and complex statistics.12 Misuse of the simplest statistical tests is common, and has been for many years.13–15 It behooves the surgeon who evaluates the literature or who performs statistical analyses to understand the principles and application of various statistical tests used for comparing two or more groups.12
Comparing the outcomes between two groups based on several characteristics of each group considered simultaneously requires multivariable analysis. Multivariate regression analysis provides a means of accounting for multiple independent variables (also called risk factors) that predict the dependent (also called outcome) variable. The result of multivariate regression is called a “model,” a slightly counter-intuitive term. Regression models allow assignment of a regression coefficient to each predictor variable that roughly corresponds to the variable contribution to outcome prediction. Again, computer software does the work; investigators do the interpretation.
There is a multitude of regression models available for analysis of cardiac surgical data. Perhaps the most common is logistic regression. Logistic regression models are a means of analyzing independent variables that predict a dichotomous outcome (eg, death, renal failure, and mediastinitis).
Regression models provide risk-adjusted assessment of outcomes that is often used for performance assessment across groups of providers. For example, logistic regression models provide a population probability (value between zero and one) of an outcome based on multiple independent predictor variables. These probabilities are called risk-adjusted probabilities and can be defined as the predicted population outcome or expected outcome. Individual members of the population have an observed outcome. The ratio of observed outcome to predicted outcome is called O/E ratio, and reflects a measure of individual provider performance. There are multiple ways to assess adequacy of regression models, especially logistic regression. Hosmer and coauthors provide a particularly comprehensive, yet practical, description of regression model assessment.16 The O/E ratio for an individual provider may give a rough estimate of risk-adjusted performance, but this measure alone does not strictly determine clinical competence or quality of care. Cumulative sum (CUSUM) analysis calculates provider O/E ratios over time and allows a graphical picture of performance that avoids point estimates.
Cardiac surgeons are frequently concerned about events that occur late following operations. Special statistical methods are used to determine the long-term status of patients following operative procedures.
The most common way to estimate time-related benefit employs the Kaplan-Meier method. This method provides an estimate of survival (or some other later time-dependent event) probability before all patients in the cohort experience the late event. This method assumes that patients who are alive at the time of analysis have the same risk of future death as those who have already died. The graphical representation of the Kaplan-Meier model gives a survival curve and allows comparisons between survival rates associated with different interventions (eg, two different valve types). The log-rank statistic is used most often to compare Kaplan-Meier survival curves.
At any point in time, an individual patient has a risk of experiencing the designated endpoint. This risk of reaching the endpoint is known as the hazard. If death is the selected endpoint, then the hazard is the risk of dying at any point in time. The cumulative hazard function is the negative logarithm of the Kaplan-Meier estimated survival obtained from the survival curve.
Surgeons are often interested in the multiple factors that predict long-term survival. These factors are typically the clinical risk factors for a patient population or the type of intervention performed. Cox regression models provide a multivariable analysis of predictors of survival using the hazard function. Using the results of Cox models, one can calculate a hazard ratio (HR), which adjusts for clinical factors in the process of providing a comparison of survival for two interventions at a given time. For example, to compare the survival of CABG against the survival of percutaneous coronary intervention (PCI) at a specified time after the procedure, one could determine the HR comparing the two procedures. The HR provides the relative risk of death of CABG versus PCI for risk-adjusted populations at a given point in time. This method assumes that the hazard function is constant over time; hence the proper name Cox proportional hazards regression. When survival curves intersect at some point in time, the proportional hazards assumption is not met and one should then use other metrics such as the risk ratio.
Essential for the assessment of the success of cardiac operations is the ability to arrange patients according to their severity of illness. Comorbid illness in patients with cardiac disease is common. Various measures of comorbidity compile patients’ risk factors into a single variable that reflects global comorbidity (Table 7-3). The comorbidity indices in Table 7-3 adjust for the incremental risk associated with specific preoperative factors variously known as risk factors, risk predictors, comorbidities, or covariates. The comorbidity systems listed in Table 7-3 are in constant evolution and use of these indices often extends to populations that include cardiac surgical patients, despite the fact that none of the indices are derived from patients undergoing cardiac procedures.
Comorbidity Index | Variables in the Index | Weights Used to Compute Index | Final Index Score | Population Used to Derive Index |
---|---|---|---|---|
Charlson Index | 19 comorbid conditions | Relative risk for each comorbid condition derived from logistic regression of mortality | Sum of weights | Cancer patients, heart disease, pneumonia, elective noncardiac operations, amputees. |
CIRS | 13 body systems | Score from 0 to 4 for each body system | Sum of weights | Elderly patients many institutionalized for long-term care. |
ICED | 14 disease categories and 10 functional categories | Score of 1 to 5 for disease categories and 1 to 3 for functional categories | Scoring algorithm that sums up disease and functional scores to arrive at values from 1 to 4 | Total hip replacements and nursing home patients. |
Kaplan Index | Two categories—vascular or nonvascular disease | Graded 0 through 3 for each category | Most severe condition. Two grade 2 are ranked as grade 3 | Diabetes and breast cancer. |
BOD Index | 59 diseases | 0 through 4 for each disease | Sum of weights | Long-stay nursing home patients. |
Cornoni-Huntley Index | 3 categories | 1—No comorbidity 2—Impaired hearing or vision 3—Heart disease, stroke or diabetes 4—Both 2 and 3 | Graded 1 through 4 | Hypertensive population and age > 75 years. |
Disease Count | Number of diseases present based on ICD-9 codes | Sum number of diseases | Maximum score based on number of diseases | Breast cancer, MI, HIV, asthma, appendicitis, low back pain, pneumonia, diabetes, abdominal hernia. |
Shwartz Index | 21 comorbidities | Relative risks from model that predicts medical costs | Sum of relative risks for each comorbidity | Stroke, lung disease, heart disease, prostate cancer, hip fracture, and low back pain. |
Table 7-3 compares commonly used comorbidity measures. The Charlson Index, the CIRS, the ICED, and the Kaplan Index are valid and reliable measures of comorbidity as measured in certain specific patient populations, but not in patients undergoing cardiac operations.17 The other comorbidity measures in Table 7-3 do not have sufficient data to assess their validity and reliability and are probably less useful than the four validated measures. There are many limitations of comorbidity indices, and they are not applied widely in studies of efficacy or medical effectiveness for cardiac operations.
Comorbidity indices, and risk factors in general, make up the variables that generate regression models that are used for risk adjustment. Most risk-adjustment models share several common features. First, the risk factors or comorbidities in the model are associated with a specific outcome. Second, if the goal is to measure provider performance, the risk factors include only patient characteristics (not hospital, physician, or regional characteristics) present prior to surgery.18 Third, a sufficient number of patients must have the risk factor, and a sufficient number must experience the adverse outcome, in order to construct an accurate risk model. Finally, it is necessary to define the period of observation for the outcomes of interest (eg, in-hospital, 30-day mortality, or both). Table 7-4 lists risk-adjustment models used to define quality or performance based on clinical outcome measures (eg, risk of death or other adverse clinical outcomes). In addition, two of the risk adjustment models shown in Table 7-4 (the Pennsylvania Cardiac Surgery Reporting System and the Canadian Provincial Adult Cardiac Care Network of Ontario) assess risk based on resource utilization (eg, hospital length-of-stay and cost) as well as on clinical outcome measures.19,20 Of the risk models listed in Table 7-4, only one, the APACHE III system, computes a risk score independent of patient diagnosis.21 All of the others in the table are diagnosis-specific systems that use only patients with particular diagnoses in computing risk scores.
Severity System | Data Source | Classification Approach | Outcomes Measured |
---|---|---|---|
APACHE III | Values of 17 physiologic parameters and other clinical information | Integer scores from 0 to 299 measured within 24 hours of ICU admission | In-hospital death |
Pennsylvania | Clinical findings collected at time of admission | Probability of in-hospital death ranging from 0 to 1 based on logistic regression model and MediQual’s AtlasTM admission severity score | In-hospital death and cost of procedure |
New York | Condition specific clinical variables from discharge record | Probability of in-hospital death ranging from 0 to 1 based on logistic regression model | In-hospital death |
Society for Thoracic Surgeons | Condition-specific clinical variables from discharge record | Originally used Bayesian algorithm to assign patient to risk interval (percent mortality interval). More recently used logistic and hierarchical regression methods | In-hospital death and morbidity |
EuroSCORE | Condition specific clinical variables from discharge record | Additive logistic regression model with scores based on presence or absence of important risk factors | 30-day and in-hospital mortality |
Veterans Administration | Condition-specific clinical variables measured 30 days after operation | Logistic regression model used to assign patient to risk interval (percent mortality interval) | In-hospital death and morbidity |
Canadian | Condition specific clinical variables entered at time of referral for cardiac surgery | Range of scores from 0 to 16 based on logistic regression odds ratio for six key risk factors | In-hospital mortality, ICU stay and postoperative length of stay |
Northern New England | Condition specific clinical variables and comorbidity index entered from discharge record | Scoring system based on logistic regression coefficients used to calculate probability of operative mortality from 7 clinical variables and 1 comorbidity index | In-hospital mortality |
Once developed from a reference population, each of the risk stratification models shown in Table 7-4 is validated in some way. There are too many individual patient and procedural differences, many of them unknown or unmeasured, to allow completely accurate validated preoperative risk assessment. The most important reason that risk-adjustment methods fail to completely predict individual outcomes is that the data set used to derive the risk score comes from retrospective, observational data that contain inherent selection bias, that is patients were given a certain treatment that resulted in a particular outcome because a clinician had a selection bias about what treatment that particular patient should receive. In observational datasets, patients are not allocated to a given treatment in a randomized manner. In addition, clinician bias may not reflect evidence-based data. Methods are available that attempt to overcome some of these limitations of observational data. These methods include use of propensity matching and “bootstrap” variable selection.22,23 Observational datasets are much more readily available and represent “real-world” treatment and outcomes compared to RCTs. An excellent review of the subtleties of evaluating the quality of risk-adjustment methods is given in the book by Iezzoni and this reference is recommended to the interested reader.24
Within the last decade the Society of Thoracic Surgeons (STS) published the most comprehensive set of cardiac surgery risk models yet available. Twenty-seven risk models encompassed nine endpoints for each of three major groups of cardiac procedures (isolated CABG, isolated valve, and valve + CABG).25–27 These risk models provide the framework for measurement of risk-adjusted outcomes for cardiac operations and, ultimately, for performance assessment of individual cardiac programs or for individual providers. The NQF has endorsed most of the STS risk adjustment models for use in determining cardiac surgery quality measures (see Table 7-2).
Ideally, differences in risk-adjusted outcomes are due to differences in quality of care, but caution is necessary in the interpretation of provider differences based on differences derived from risk adjustment models. One study simulated the mortality experience for a hypothetical set of hospitals assuming perfect risk adjustment and with prior perfect knowledge of poor quality providers.28 These authors used various simulation models, including Monte Carlo simulation, and found that under all reasonable assumptions, sensitivity for determining poor provider quality was less than 20% and the predictive error for determining high outliers was greater than 50%. Much of the observed mortality rate differences between high outliers and nonoutliers were attributable to random variation. Park and coauthors suggest that providers identified as high outliers using conventional risk adjustment methods do not provide lower quality care than do nonoutliers, and that most of the outcome differences are due to random variations.29
Many risk stratification models for cardiac operations are used to assess surgical performance. Before a risk model and its component risk factors are used to evaluate provider performance, these models are tested for accuracy. Many patient variables are candidate risk factors for operative mortality following coronary revascularization. Examples include serum blood urea nitrogen (BUN), cachexia, oxygen delivery, HIV, case volume, low hematocrit on bypass, the diameter of the coronary artery, and resident involvement in the operation. On the surface, these variables seem like valid risk factors, but many are not. All putative risk factors should be subjected to rigorous scrutiny. Tests of risk model prediction including regression diagnostics (eg, receiver operating characteristics (ROC) curves and cross-validation studies) performed on the models included in Tables 7-4 suggest that the models are good, but not perfect, at predicting outcomes. In statistical terms this may mean that all of the variability in outcome measurement is not explained by the set of risk factors included in the regression models. Hence, it is possible that inclusion of new putative risk factors in the regression equations may improve the validity and precision of the models. New regression models, and new risk factors, must be scrutinized and tested with regression diagnostics before acceptance. However, it is uncertain whether inclusion of many more risk factors will significantly improve the quality and predictive ability of regression models, and there is an ongoing tension between parsimonious models and robust models that contain many variables. For example, the STS risk stratification model described in Table 7-4 includes many predictor variables, while the Toronto risk adjustment model includes only five predictor variables. Yet the regression diagnostics for these two models are similar, suggesting that both models have equal precision and predictive capabilities for identifying outcome measures. Studies show that much of the predictive ability of risk models is contained in a relatively small number of risk factors.30,31 Other studies suggest that the limiting factor in the accuracy of current risk models may be a failure to understand and account for all the important factors related to risk.32 Additionally, risk models are useful for predicting the average outcome for a population of patients with specific risk factors, but not necessarily accurate for predicting the outcome for a specific patient. Further work needs to be done, both to explain the differences in risk factors seen between the various risk models and to determine which models are best suited for studies of quality improvement and performance assessment.
After World War II, there was a rapid expansion of therapeutic options, especially drugs, for treatment of previously untreatable acute and chronic diseases. With the advent of this new drug therapy came the introduction of RCTs to define the efficacy of various drug regimens. Archie Cochrane, a Scottish pulmonary medicine specialist, championed RCTs as the most reliable means of deciding on optimal treatment (Fig. 7-1). He suggested that RCTs were the best means of identifying interventions that have a causal influence on outcomes. His efforts eventually led to the creation of the Cochrane Collaboration, a repository for works of evidence-based medicine (EBM). He is arguably the father of EBM and is largely responsible for the current preeminence of RCTs as the “holy grail” of decisions about treatment options. RCTs provide the best evidence to decide about surgical treatment options for cardiac diseases, as well as providing the best means of identifying cause and effect relationships. The impact of Cochrane’s insistence on wide dissemination of published high-quality evidence, including RCTs, and summaries of RCTs and observational data including meta-analyses and systematic reviews (collectively referred to as EBM publications), is felt today, even more than in previous decades. In 2014, a Pub Med search identified 27 EBM publications concerning various treatment options related to cardiac operations and associated diseases.
Not every question about cardiac operations can be answered by RCTs. For many reasons the majority of the published cardiac surgical studies are observational studies, not RCTs. In nearly all observational studies, there may be selection bias. Patients underwent a treatment for nonrandom reasons. The nonrandom nature of observational studies mandates statistical methods to account for variables (usually preoperative variables) that affect the outcome (typically operative mortality and/or morbidity). Shortly after the creation of the Cochrane Collaboration in the 1970s, publications suggested that observational studies may weight outcomes in favor of new therapies, much more so than RCTs that address the same comparisons.33 Almost 20 years later reassessment of the value of nonrandomized comparisons suggested that well-done observational studies using carefully selected controls (either cohort or case-control designs) do not overestimate treatment benefit.34
There are multiple ways to address bias in observational studies. Perhaps the most widely used technique involves careful matching of treatment and control groups using various statistical techniques, the most popular method being propensity score matching.35 The propensity score is constructed in as robust a manner as possible given the population variables. Typically logistic regression with all preoperative independent variables forced into the regression equation is used to compute the propensity score for comparison of two groups.36 Matching control and experimental groups based on their propensity scores (ie, probability of a given outcome based on the logistic regression-derived probability) provides a “pseudo-randomized” group from the total population. Comparisons using carefully matched groups approximate RCTs in most cases.34
There are clear advantages and disadvantages associated with both RCT and observational studies. Because of the absence of confounding and selection bias, the RCT remains the “gold standard” for comparing results. RCTs focus on relatively small, highly select populations that may have little in common with patients typically seen in practice. Small sample size often precludes meaningful subgroup analysis and statistical analyses may be underpowered. RCTs are also quite costly and so time-consuming that results may often be outdated by the time they are reported. On the other hand, observational studies usually involve large populations of “real-world” patients. Subgroup cohorts with adequately powered statistical analysis are commonly seen in observational studies. Since these studies often use registry data, they can be carried out in a timely fashion with minimal expense. Cohort balancing using propensity scoring techniques and other statistical approaches provides useful comparisons that can approximate RCTs, but careful matching techniques for group comparisons never completely rule out bias or confounding.
One goal of assessment of cardiac procedures is to define best practices. Wide dissemination of global assessment of cardiac operations can provide evidence that helps surgeons know the best alternative for surgical treatment of cardiovascular disorders. Recognizing the difficulties in defining “best practices” for a given illness, professional organizations opted to promote practice guidelines or “suggested therapy” for cardiac surgical diseases.37,38 These practice guidelines represent a compilation of available published evidence, including randomized trials and observational studies. For example, the practice guideline for CABG is available for both practitioners and the lay public on the Internet (http://circ.ahajournals.org/content/124/23/e652.full.pdf+html).
Guidelines are a list of recommendations that have varying support in the literature. The strength of a guideline recommendation is often graded by class and by level of evidence used to support the class of recommendation. A typical rating scheme used by the STS Workforce on Evidence Based Surgery and by the Joint American College of Cardiology/American Heart Association Task Force on Practice Guidelines has three classes of recommendations as follows:
Class I—Conditions for which there is evidence and/or general agreement that a given procedure or treatment is beneficial, useful, and effective.
Class II—Conditions for which there is conflicting evidence and/or a divergence of opinion about the usefulness/efficacy of a procedure or treatment.
Class IIa—Weight of evidence/opinion is in favor of usefulness/efficacy.
Class IIb—Usefulness/efficacy is less well established by evidence/opinion.
Class III—Conditions for which there is evidence and/or general agreement that a procedure/treatment is not useful/effective and in some cases may be harmful.
Evidence supporting the various classes of recommendations ranges from high-quality RCTs to consensus opinion of experts. Three categories describe the level of evidence used to arrive at the Class of Recommendation:
Level A—Data derived from multiple randomized clinical trials or meta-analyses.
Level B—Data derived from a single randomized trial or nonrandomized studies.
Level C—Consensus opinion of experts, case studies, or standard-of-care.
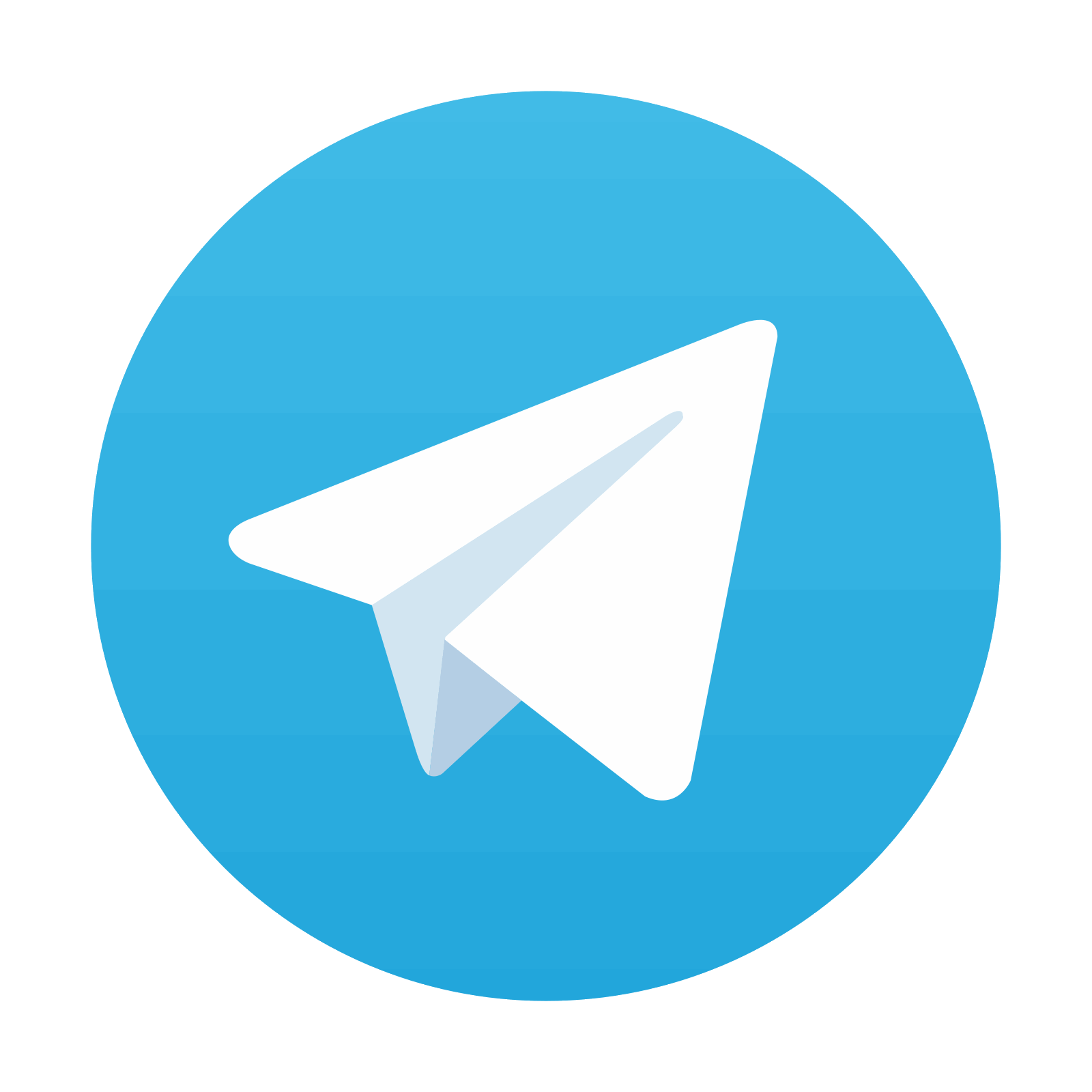
Stay updated, free articles. Join our Telegram channel

Full access? Get Clinical Tree
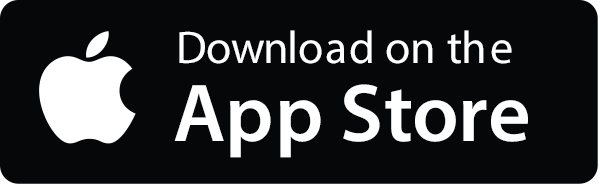
