Summary
Background
Resistant hypertension is common, mainly idiopathic, but sometimes related to primary aldosteronism. Thus, most hypertension specialists recommend screening for primary aldosteronism.
Aims
To optimize the selection of patients whose aldosterone-to-renin ratio (ARR) is elevated from simple clinical and biological characteristics.
Methods
Data from consecutive patients referred between 1 June 2008 and 30 May 2009 were collected retrospectively from five French ‘European excellence hypertension centres’ institutional registers. Patients were included if they had at least one of: onset of hypertension before age 40 years, resistant hypertension, history of hypokalaemia, efficient treatment by spironolactone, and potassium supplementation. An ARR > 32 ng/L and aldosterone > 160 ng/L in patients treated without agents altering the renin–angiotensin system was considered as elevated. Bayesian network and stepwise logistic regression were used to predict an elevated ARR.
Results
Of 334 patients, 89 were excluded (31 for incomplete data, 32 for taking agents that alter the renin–angiotensin system and 26 for other reasons). Among 245 included patients, 110 had an elevated ARR. Sensitivity reached 100% or 63.3% using Bayesian network or logistic regression, respectively, and specificity reached 89.6% or 67.2%, respectively. The area under the receiver-operating-characteristic curve obtained with the Bayesian network was significantly higher than that obtained by stepwise regression (0.93 ± 0.02 vs. 0.70 ± 0.03; P < 0.001).
Conclusion
In hypertension centres, Bayesian network efficiently detected patients with an elevated ARR. An external validation study is required before use in primary clinical settings.
Résumé
Contexte
L’hypertension résistante est fréquente, principalement idiopathique mais parfois liée à un hyperaldostéronisme primaire. La plupart des spécialistes de l’hypertension recommandent le dépistage de l’hyperaldostéronisme primaire chez des hypertendus résistants afin d’en optimiser la prise en charge.
Objectifs
Prédire un rapport aldostérone sur rénine élevé à partir des caractéristiques cliniques et biologiques simple de patients hypertendus résistants dans le but d’optimiser la sélection des patients à risque d’hyperaldostéronisme primaire.
Méthodes
Nous avons, à partir de registres, colligé les données de patients vus consécutivement entre le premier juin 2008 et le 30 mai 2009 dans cinq centres français labélisés « centres d’excellence européens d’hypertension ». Les patients inclus avaient au moins un des critères suivants : apparition d’une hypertension avant 40 ans (55 %), hypertension résistante (44 %), antécédent d’hypokaliémie (38 %), traitement efficace par spironolactone (15 %) et/ou supplémentation en potassium (11 %). Un rapport aldostérone sur rénine (ARR) > 32 (ng/L)/(ng/L) (avec une aldostéronémie > 160 ng/L) chez les patients traités sans agents modifiant le système rénine–angiotensine a été considéré comme élevé. Un réseau bayésien et une régression logistique ont été comparés pour leurs performances dans la prédiction d’un ARR élevé.
Résultats
Trois cent trente-quatre patients adultes ont été inclus dans l’étude. Quatre-vingt-neuf ont été exclus pour données incomplètes ( n = 31), pour traitement avec des agents modifiant le système rénine–angiotensine ( n = 32) ou pour une autre cause connue d’hypertension secondaire ( n = 26). Parmi les 245 dossiers analysés, 110 sujets avaient un ARR élevé. Utilisant le réseau bayésien ou la régression logistique, la sensibilité de la prédiction d’un ARR élevé atteint respectivement (100 % vs 63,3 %) et la spécificité (89,6 % vs 67,2 %). L’aire sous la courbe ROC obtenue avec le réseau bayésien est significativement plus élevée que celle obtenue par la régression logistique (0,93 ± 0,015 vs 0,70 ± 0,03, p < 0,001).
Conclusion
Dans les centres d’hypertension, l’utilisation d’un réseau bayésien pourrait permettre de détecter efficacement les patients ayant un ARR élevé, patients à haut risque d’hyperaldostéronisme primaire. Une étude de validation externe sera néanmoins nécessaire avant l’utilisation du modèle en médecine générale.
Background
Biological primary aldosteronism is one of the most common causes of resistant hypertension , either because aldosterone secretion does not correspond to treatment or due to underlying primary aldosteronism. Because resistant hypertension is common and mainly idiopathic, screening for biological primary aldosteronism must be restricted to selected patients. Detection of primary aldosteronism is recommended in resistant hypertension and in patients with hypokalaemia by first determining the plasma aldosterone-to-renin ratio (ARR) under standard conditions .
Using screening models, selection of patients at higher risk of an elevated ARR could be improved. We therefore looked for clinical and readily available biochemical variables predictive of an elevated ARR. Usually, logistic regression analyses are used to build prediction models , but recently, Bayesian networks have been used to develop diagnostic and prognostic models . The aim of our work was to assess the best model to predict an elevated ARR from simple clinical and biological criteria collected during a medical consultation.
Methods
Patients
Using institutional registers, data from patients referred for primary aldosteronism screening between 1 June 2008 and 30 May 2009 were collected retrospectively. The inclusion criterion was the presence of one of the following: onset of hypertension before 40 years of age, history of hypokalaemia, drug-resistant hypertension (to three drugs), potassium supplementation or high effectiveness of spironolactone on arterial pressure. Previous diagnosis of any secondary forms of hypertension was an exclusion criterion.
Data collection
We recorded factors that are clearly linked to the risk of primary aldosteronism and discarded those that are still debated in the literature. Accordingly, the following data were collected:
- •
anthropomorphic variables and lifestyle: weight, height, smoking habit, alcohol consumption, liquorice consumption;
- •
hypertension history: duration, family history, effectiveness of spironolactone to normalize blood pressure and potassium supplementation;
- •
biological variables: natraemia, kalaemia, creatininaemia, plasma bicarbonate and 24-hour urinary excretion of creatinine, sodium, potassium, albumin and aldosterone.
Sitting blood pressure was measured three times at 2-minute intervals after a 5-minute rest. The average of the three blood pressure measurements was calculated. Validated automatic sphygmomanometers were used in all centres and cuffs were adapted to arm circumferences. Aldosterone and renin concentrations (both expressed in ng/L) were determined after 1 hour in the supine position and after ≥ 1 hour in the standing position. An ARR > 32 ng/L with a plasma aldosterone value > 160 ng/L was used to define elevated ARR .
Medications
Treatments that might interfere with the renin–angiotensin system were not allowed. A washout period before ARR screening was required, for 2 weeks for angiotensin converting enzyme inhibitors, angiotensin receptor blockers and beta-blockers, 4 weeks for diuretics, and 6 weeks for spironolactone. Calcium channel blockers (non-dihydropyridine or long-acting dihydropyridine), central-acting agents (rilmenidine), and alpha blockers (slow-release prazosin, urapidil) were allowed. Any sodium and potassium dietary supplementation were not modified; and any oral contraceptives and hormone replacement therapies were not stopped.
Statistical analysis
Patients with an elevated ARR were compared with the remaining patients. Data are expressed as mean ± standard deviation (SD) or number (percentage). Mean values of groups were compared using Student’s t -test. Categorical variables were compared using a Chi 2 test. Continuous variables included in the logistic regression were: age, body mass index (BMI), systolic blood pressure (SBP), diastolic blood pressure (DBP), heart rate, kalaemia, natraemia, hypertension duration, estimated glomerular filtration rate (eGFR), plasma bicarbonate and 24-hour urinary excretion of sodium and potassium. Smoking status, potassium supplementation and gender were entered as binary variables.
Bayesian networks belong to the family of graphical models. The network structure can be described as follows: each node in the graph represents a variable, while the edges between the nodes represent probabilistic dependencies among the corresponding variables. Netica ® 2.05 software (Norsys Corporation™, https://www.norsys.com ) was used for modelling. The Kullback–Leibler distance was used to assess the degree of dependence between two variables of the network .
A receiver-operating-characteristic (ROC) curve graphically displays the relationship between sensitivity ( y -axis) and 100–specificity ( x -axis) and permits the selection of the point that best represents the trade-off between the ability to detect the positives (sensitivity) against the failure to detect the negatives (specificity). Sensitivity and specificity for the best cut-off value, area under the ROC curve, positive predictive values (PPVs), and negative predictive values (NPVs) were calculated. Areas under the ROC curves obtained by both models were compared. All analyses were performed using Medcalc ® software (version 11.0.1).
Results
Among 334 potential patients, 89 were excluded (31 for incomplete data, 32 for taking agents that alter the renin–angiotensin system and 26 for other reasons [recent child birth, known nephropathy, known adrenal adenoma, known renal artery stenosis]). Thus, our analysis was based on 245 patients with complete data corresponding to our objective. The following percentages of patients met at least one inclusion criteria: onset of hypertension before 40 years of age (55%), resistant hypertension (44%), history of hypokalaemia (38%), efficient treatment by spironolactone (15%), and potassium supplementation (11%).
Among the 245 included patients, 110 had an elevated ARR and 135 were considered to have essential hypertension (EH). Patient characteristics are shown in Table 1 . SBP values at presentation were higher in the elevated ARR group ( P < 0.05) while DBP values were not different between groups. Kalaemia was significantly lower in the elevated ARR group ( P < 0.001) and hypokalaemia (kalaemia ≤ 3.5 mmol/L) was significantly more frequent in the elevated ARR group than in the EH group (50.9% vs. 27.4%; P < 0.001). eGFR (estimated by the Modification of Diet in Renal Disease formula) did not differ between the groups. Supine and standing renin levels were significantly lower in the elevated ARR group ( P < 0.001) whereas supine and standing aldosterone were significantly higher ( P < 0.001).
EH ( n = 135) | Elevated ARR ( n = 110) | P a | |
---|---|---|---|
Age (years) | 44.2 ± 16.3 | 50.3 ± 12.5 | < 0.001 |
Men | 70/65 | 56/54 | NS |
Onset of hypertension before age 40 years | 63.7 | 43.6 | < 0.01 |
Hypertension duration (years) | 8.8 ± 10.2 | 9.8 ± 10.2 | NS |
Supine aldosterone (ng/L) | 107 ± 74 | 206 ± 183 | < 0.001 |
Standing aldosterone (ng/L) | 314 ± 205 | 442 ± 257 | < 0.001 |
Supine renin (ng/L) | 11.3 ± 4.7 | 7.1 ± 4.3 | < 0.001 |
Standing renin (ng/L) | 22.6 ± 6.4 | 14.2 ± 5.3 | < 0.001 |
Supine ARR | 10.3 ± 6.3 | 36.6 ± 35.4 | < 0.001 |
Standing ARR | 15.5 ± 7.0 | 70.3 ± 53.1 | < 0.001 |
BMI (kg/m 2 ) | 27.7 ± 5.7 | 28.9 ± 6.4 | NS |
Kalaemia (mmol/L) | 3.95 ± 0.33 | 3.78 ± 0.43 | < 0.001 |
eGFR (mL/min/1.73 m 2 ) | 88 ± 19 | 87 ± 21 | NS |
Sodium (mmol/L) | 140.8 ± 2.3 | 142.0 ± 2.6 | < 0.01 |
SBP (mmHg) | 156 ± 23 | 161 ± 20 | < 0.05 |
DBP (mmHg) | 95 ± 14 | 96 ± 13 | NS |
Potassium supplementation | 2.9 | 19.1 | < 0.001 |
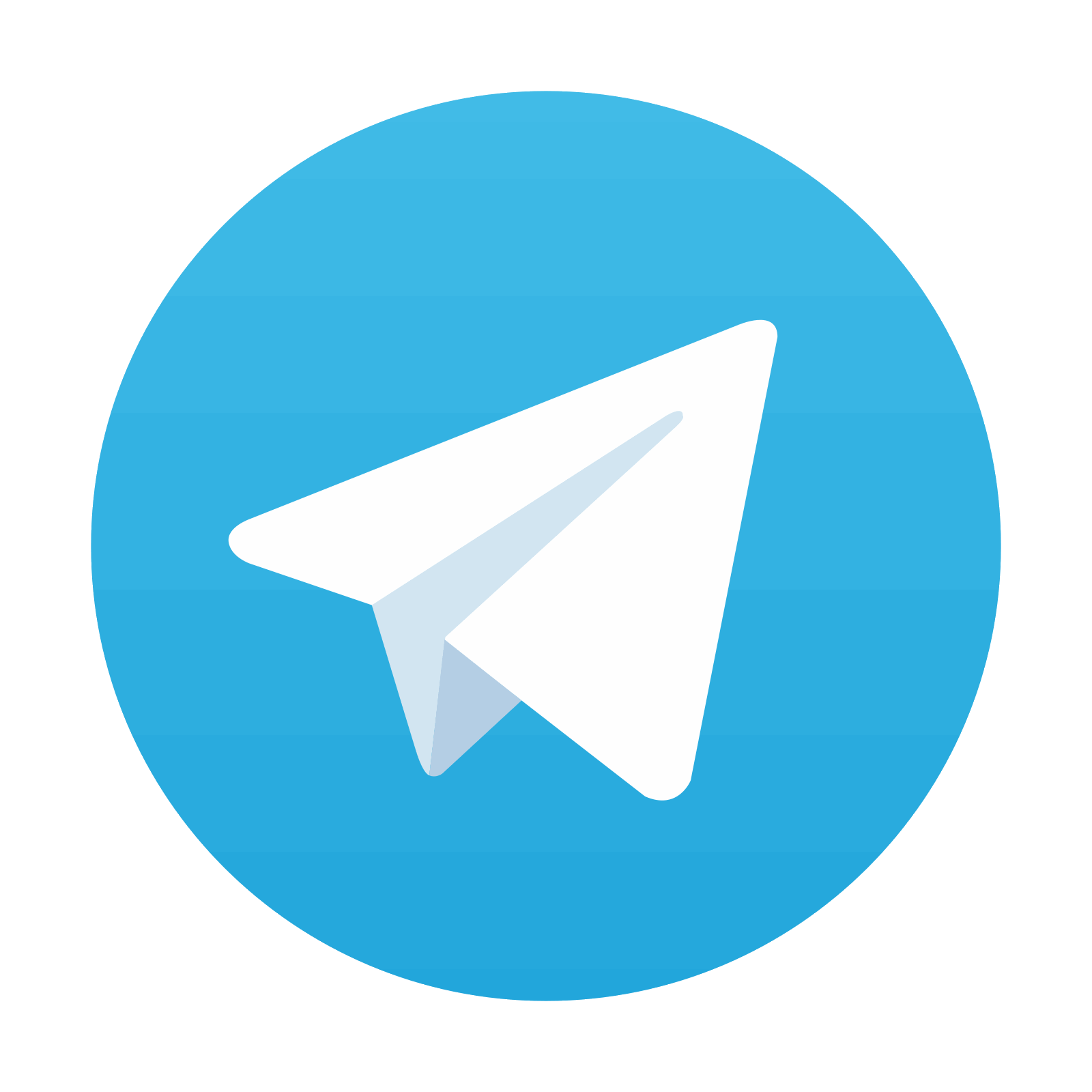
Stay updated, free articles. Join our Telegram channel

Full access? Get Clinical Tree
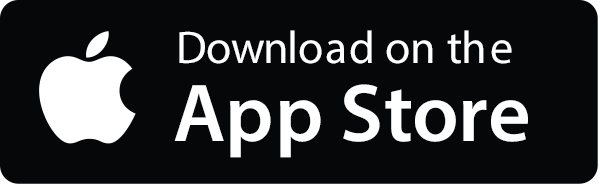

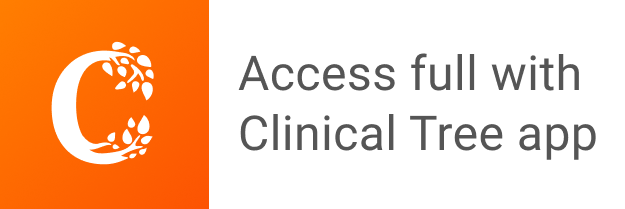