The novel coronavirus SARS-CoV-2 uses the angiotensin-converting enzyme 2 receptor as an entry point to the cell. Cardiovascular disease (CVD) is a risk factor for COVID-19 with poor outcomes. We tested the hypothesis that the rate of angiotensin-converting enzyme inhibitor (ACEI) and angiotensin receptor blocker (ARB) use is associated with the rate of COVID-19–confirmed cases and deaths. We conducted a geospatial, ecological study using publicly available county-level data. The Medicare ACEI and ARB prescription rate was exposure. The COVID-19–confirmed case and death rates were outcomes. Spatial autoregression models were adjusted for the rate of births and deaths; Group Quarters population; percentage of female; percentage of Native American, Pacific Islander, Hispanic, and Black; percentage of children and older (>65 years) adults; percentage of uninsured; percentage of those living in poverty; percentage of those who are obese, smoking, admitting insufficient sleep, and those with at least some college degree; median household income; air quality index; CVD hospitalization rate in Medicare beneficiaries; and CVD death rate in a total county population. After adjustment for confounders, the ACEI use rate did not associate with COVID-19–confirmed case rate (direct county-own effect + 0.027%; 95% confidence interval [CI] −1.080 to 1.134; p = 0.962; indirect spillover effect + 0.26%; 95% CI −70.0 to 70.5; p = 0.994). Similarly, the ARB use rate was not associated with COVID-19–confirmed case rate (direct effect + 0.029%; 95% CI −0.803 to 0.862; p = 0.945; indirect effect + 0.19%; 95% CI −52.8 to 53.2; p = 0.994). In both unadjusted and adjusted Bayesian zero inflation Poisson analysis, neither ACEI nor ARB use rates were associated with COVID-19 death rates. In conclusion, ACEI and ARB use rates were not associated with COVID-19 infectivity and death rate in this ecological study.
Despite available vaccines, the pandemic of the novel COVID-19 caused by the SARS-CoV-2 virus remains a threat. It has been shown that SARS-CoV-2 uses the angiotensin-converting enzyme (ACE) 2 receptor as an entry point into a cell. With the ACE2 receptor acting as a binding site for SARS-CoV-2, the renin-angiotensin-aldosterone system, and the medications affecting it become important points of discussion. ACE inhibitors (ACEIs) and angiotensin receptor blockers (ARBs) are 2 classes of medications widely used in patients with hypertension, diabetes mellitus, cardiovascular disease (CVD), and latent or manifest left ventricular dysfunction, who are at risk for severe COVID-19 cases and deaths. Previous experiments showed that ACE2 expression was associated with susceptibility to SARS-CoV-2 infection. The consensus is reached about the importance of the continuation of ACEI and ARB use in the COVID-19 pandemic. , However, controversy remains whether clinically indicated use of ACEI and ARB improves or worsens infectivity or the course of COVID-19. To address this need, we conducted an ecological study. We hypothesized that in the geospatial analysis, the rate of ACEI and ARB use is associated with the number of confirmed COVID-19 cases and deaths in the United States.
Methods
An individual county in the United States was an observation unit in this study. We used a Federal Information Processing Standard (FIPS) county code to link the data. Data with missing FIPS codes were excluded from the study. Geographic information about each county was obtained from the cartographic boundary files (shapefiles) provided by the United States Census Bureau’s MAF/TIGER (Master Address File/Topologically Integrated Geographic Encoding and Referencing) geographic database.
We used the 2018 Centers for Medicare & Medicaid Services public dataset, the Medicare Provider Utilization and Payment Data: Part D Prescriber Public Use File, with information on prescription drugs prescribed by individual physicians and other health care providers and paid for under the Medicare Part D Prescription Drug Program in 2018. The dataset included the total number of prescriptions that were dispensed (total day supply), which include original prescriptions and any refills, and therefore reflects ACEI and ARB usage.
The Medicare dataset only includes city and state information and not county information. To map the Medicare prescription data to their corresponding FIPS Code, we used the Google Geocoding application programming interface. We then loaded the Medicare data and geocoded data into the SQLite database to produce the final datasets with prescription counts per county. Prescriptions from county-equivalents (independent cities) were manually matched with their corresponding FIPS code. Medicare prescriptions with misspelled cities or prescriptions that lacked valid city and state descriptions, if unable to be assigned, were excluded. Excluded prescriptions accounted for <0.01% of the data.
We calculated a drug class use rate as a sum of the total day supply in a county for all drugs comprising a particular class ( Supplementary Table 1 ), normalized by the total county population estimate. We used the United States Census Annual Resident Population Estimates for July 1, 2019.
The primary outcome was confirmed COVID-19 cases. The secondary outcome was COVID-19 deaths. We imported the raw COVID-19 data from the Johns Hopkins GitHub repository. The number of confirmed COVID-19 cases and deaths in each county as reported for February 6, 2021, was divided by the total population in each county (2019 county population estimate) and multiplied by 100,000 to convert to cases and deaths per 100,000 population. We decided to use February 6, 2021, COVID-19 data because, by that date, the pandemic had sufficiently played out without the significant confounding effect of the vaccination, which began at the end of December 2020.
We used the United States Census County Population Estimates, released in March 2020, and included reported deaths and births from July 1, 2018, to June 30, 2019. Because of the known negative impact of COVID-19 on the population of nursing homes and prisons/jails, we included July 1, 2019, Group Quarters total population estimate. Group Quarters Facilities include correctional facilities for adults, nursing homes, college/university student housing, military quarters, and group homes. Group Quarters data were gathered from an estimated 20,000 randomly selected facilities. Data were then collected through resident interviews of these selected facilities using the American Community Survey conducted by the United States Census Bureau. The total 2019 county population estimate normalized all demographic characteristics.
To characterize socioeconomic characteristics, we used the 2018 median household income expressed as a percent of the state total and percent of the total population in poverty, as reported by the Economic Research Service of the United States Department of Agriculture. We also used the data compiled by the County Health Rankings & Roadmaps program, which is a collaboration between the Robert Wood Johnson Foundation and the University of Wisconsin Population Health Institute.
To characterize CVD prevalence and severity, we used the Centers for Disease Control and Prevention (CDC) estimates of total CVD death rate per 100,000 population (2016 to 2018), total CVD hospitalizations (2015 to 2017) per 1,000 Medicare beneficiaries, heart failure (HF) death rate per 100,000 population (2016 to 2018), HF hospitalization rate per 1,000 Medicare beneficiaries (2015 to 2017), coronary heart disease (CHD) death rate per 100,000 population (2016 to 2018), CHD hospitalization rate per 1,000 Medicare beneficiaries (2015 to 2017), and age-adjusted diabetes mellitus percentage in adults (age >20 years). These data were obtained from the Interactive Atlas of Heart Disease and Stroke, published by the CDC. Within this atlas, death rates were gathered from the National Vital Statistics Program (Deaths), hospitalization rates were gathered from the CMS Medicare Provider Analysis and Review File Part A, and diabetes mellitus percentages were collected from the Division of Diabetes Translation.
To characterize the use of cardiovascular (CV) medications, we calculated the rate of CV medications use, which included original prescriptions and any refills (total day supply), as reported in the 2018 Centers for Medicare & Medicaid Services Part D Medicare Prescriber Public Use File. We considered the total day supply data for 20 medication groups ( Supplementary Table 1 ): ACEI; ARB; beta blockers; alpha and beta blockers; alpha blockers; Class I, III, and V antiarrhythmic medications; dihydropyridine and nondihydropyridine calcium channel blockers; aldosterone antagonists; central acting antihypertensive medications; vasodilators; diuretics; lipid-lowering drugs; insulins; oral hypoglycemic agents; anticoagulants; and antiplatelet medications. We normalized the CV medications’ day supply for each county by the 2019 county population estimate.
A detailed description of statistical analysis methods is provided in the Supplementary Material. To model an ecological association of exposure with outcomes, we used different models for COVID-19–confirmed case rate and death rate to obtain the best fit and satisfy the assumptions. As COVID-19 is a contagious disease, confirmed cases and deaths in neighboring counties are spatially correlated. Therefore, we used the spatial autoregression model that allows modeling the spatial dependence in the outcomes, covariates, and unobserved errors. The spatial autoregression model used the generalized spatial 2-stage (method-of-moment), least-squares estimator. , As we confirmed ( Supplementary Figure 1 ) nearly normal distribution of standardized confirmed case rates, which had no 0 values, we used Gaussian likelihood. The model included spatial lags of the outcome variable, spatial lags of covariates, and spatially autoregressive errors. The lag operator was a spatial weighting (inverse-distance) matrix, which summarized spatial relations between counties based on the distance between county centroids. The weighting matrix was scaled so that its largest eigenvalue is 1, which guarantees nonsingularity in the model estimation. We constructed cross-sectional spatial autoregressive models. The estimator treated the errors as heteroskedastic, thus relaxing the assumption that errors represent identically distributed disturbance. We conducted the Moran test to determine whether exposure, outcome, and covariates are spatially dependent.
First, we constructed unadjusted spatial autoregression models, to investigate a geospatial association of the county population characteristics with the ACEI and ARB use rate. Next, we constructed unadjusted and bivariate spatial autoregression models to evaluate a geospatial association of the county population characteristics with the rate of COVID-19–confirmed cases. We constructed adjusted spatial autoregression models to answer the main study question: whether there is an independent association of ACEI or ARB use rates with COVID-19–confirmed case rate. The selection of covariates for adjustment was guided by confounders observed in this study and model fit. Models with COVID-19–confirmed case rate outcome were adjusted for the rate of births and deaths; Group Quarters population; percentage of female, Native American, Pacific Islander, Hispanic, and Black non-Hispanic county residents; percentage of county residents younger than 18 and older than 65 years of age; percentage of uninsured, living in poverty, residents with at least some college degree; median household income as a percent of the state total; percentage of obese, smoking, admitting insufficient sleep residents; air quality index; CDC-reported CVD hospitalization rate in Medicare beneficiaries; and CVD death rate in a total county population.
Because there were counties without documented COVID-19 deaths, to account for multimodal distribution (mixture of distributions) of COVID-19 death outcome, we constructed Bayesian zero-inflated Poisson models , with noninformative prior. Models were adjusted for the rate of births and deaths, Group Quarters population, percentage of Hispanic residents, living in poverty, with at least some college degree, and admitting insufficient sleep, as well as air quality index, CDC-reported CVD hospitalization rate in Medicare beneficiaries, and CVD death rate in a total county population.
Cross-sectional geospatial analysis is susceptible to reverse causality bias. It is well-documented that patients with CVD and CV risk factors have a higher rate of COVID-19–confirmed cases and deaths. The rate of ACEI and ARB use indirectly indicates CVD prevalence and severity. While we adjusted our models for the broad range of confounders, including CVD mortality in a total county population, and CVD hospitalization rate in Medicare beneficiaries, reverse causality remained a concern. To assess the possibility and extent of reverse causality bias, we constructed the models for the use rate of other CV medications for each class of drugs separately, one by one. Statistical analyses were performed using STATA MP 16.1 (Stata Statistical Software: Release 16. (College Station, Texas. StataCorp LLC.). The study dataset and STATA code are provided at https://github.com/Tereshchenkolab/geospatial .
Results
We analyzed the data of 3,141 counties and county-equivalents in the 50 states and the District of Columbia. The ACEI group was the second most ubiquitous medication, surpassed only by lipid-lowering drugs ( Supplementary Table 1 ). Average county characteristics are reported in Table 1 . Figure 1 shows the ACEI and ARB total day supply rates across the United States. On average, the total daily supply rate was higher for ACEI than for ARB The Moran test indicated that the rates of ACEI and ARB use were spatially dependent (p <0.0001).
Characteristic | Mean ± SD |
---|---|
Population in 2019 | 104,502 ± 333,504 |
Births in 2019 per 100,000 population | 1,098.7 ± 240.5 |
Deaths in 2019 per 100,000 population | 1,041.3 ± 269.8 |
Group Quarters population in 2019 per 100,000 population | 3,375.5 ± 4,411.8 |
% poverty | 15.2 ± 6.1 |
Median household income as % of state total | 89.4 ± 20.1 |
% Adults with self-reported poor or fair health | 17.9 ± 4.7 |
% Adult smoking | 17.5 ± 3.6 |
% Adult obesity | 32.9 ± 5.4 |
% Physical inactivity | 27.4 ± 5.7 |
% Excessive drinking | 17.5 ± 3.1 |
% uninsured (all) | 11.5 ± 5.1 |
% with some college education | 57.9 ± 11.8 |
Air pollution index | 8.98 ± 2.01 |
% of households with high housing costs | 11.1 ± 3.7 |
% Food insecurity | 13.2 ± 4.0 |
% insufficient sleep | 33.0 ± 4.2 |
% population age <18 years | 22.1 ± 3.5 |
% population age >65 years | 19.3 ± 4.7 |
% non-Hispanic Black | 9.0 ± 14.3 |
% Native Americans | 2.3 ± 7.7 |
% Asians | 1.6 ± 3.0 |
% Pacific Islanders | 0.1 ± 0.4 |
% Hispanics | 9.7 ± 13.8 |
% non-Hispanic White | 76.0 ± 20.2 |
% female | 49.9 ± 2.2 |
CVD hospitalization rate per 1,000 Medicare beneficiaries | 59.5 ± 16.7 |
CVD death rate per 100,000 population | 239.9 ± 51.5 |
Heart failure hospitalization rate per 1,000 Medicare beneficiaries | 15.2 ± 6.5 |
Heart failure death rate per 100,000 population | 107.9 ± 25.8 |
CHD hospitalization rate per 1,000 Medicare beneficiaries | 13.1 ± 4.0 |
CHD death rate per 100,000 population | 102.7 ± 32.1 |
Diabetes mellitus age-adjusted percentage (age > 20 years) | 10.4 ± 3.8 |

In unadjusted spatial autoregression analysis ( Supplementary Table 2 ), as expected, CVD prevalence, general demographic characteristics, uninsured rate, and air quality were associated with the use of both ACEI and ARB. A higher percentage of the total population in poverty and the lower Group Quarters population were associated with higher use of ACEI, but not ARB. A higher percentage of residents with at least some college degree was associated with the use of ARB, but not ACEI.
COVID-19–confirmed case and death rates ( Figure 2 ) had similar geographic distributions. As of February 6, 2021, in an average county, there was a median of 8,498.1 (interquartile range 6,742.6 to 10,201.6) confirmed cases per 100,000 population. Every county had confirmed cases. The lowest rate of confirmed cases was 250.4 per 100,000 population.
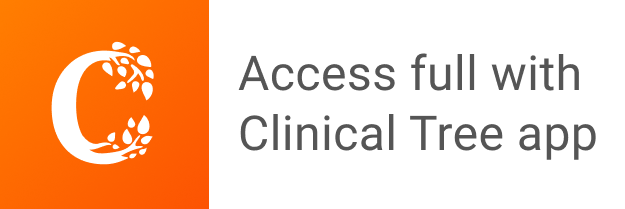