Although randomized trials and observational studies are used as the evidentiary basis of clinical practice guidelines, they are not always in agreement. Limitations in the process of randomization in the former and the selective referral of patients for treatment as a consequence of clinical “risk stratification” in the latter are underappreciated causes for these disagreements. As a result, neither is guaranteed to correctly quantify treatment benefit. This essay reviews the operational differences between these alternative evidentiary sources and shows how these differences can affect individual clinical decisions, population-based practice guidelines, and national health policy. In conclusion, the process of evidence-based medicine can be improved by independent agencies charged with the responsibility to identify and resolve these differences.
Life [is] like a box of chocolates. You never know what you’re gonna get.
The randomized clinical trial is the foundation of evidence-based medicine. Sometimes, however, such trials seem far removed from real-world clinical practice. Two particularly contrasting examples are worth noting. The explanatory trial seeks to verify the truth of a fastidious hypothesis: Treatment A is better than Treatment B . Thus, if we wish to compare the efficacy of alternative treatments relative to long-term survival rate, we might choose to restrict our study to older and sicker patients in whom differences in benefit and harm will be easier to demonstrate over a reasonably short period of follow-up. The disadvantage of this choice, however, is that we might define the population so narrowly as to limit its inferential power with respect to younger patients or those with lesser degrees of infirmity.
A pragmatic trial, in contrast, seeks to minimize the chance of administering the inferior treatment: should we recommend Treatment A or Treatment B ? The advantage of the pragmatic trial is that it more closely mirrors the actual decisions faced by real-world patients and practitioners. Its disadvantage is that it must be performed in a large population that is broadly representative with respect to these self-same decisions. Such trials are usually very inefficient in terms of resource allocation and cost. As a result, so-called observational registries (formalized compilations of uncontrolled data derived from routine clinical practice, individual unrandomized studies, or meta-analytic summaries of such studies) are increasingly seen as a more practical alternative.
Unfortunately, clinical trials and observational registries do not always agree. For example, Sen et al recently argued that allocation bias explains the disparity between randomized clinical trials and observational data relative to the comparison of primary percutaneous coronary intervention and fibrinolysis for the management of ST-elevation myocardial infarction. In positing their argument, the authors take it to be axiomatic that the process of randomization guarantees equal allocation of all recognized and unrecognized covariates and thereby serves to define the true effect of the alternative treatments. This true effect is “abolished” in the observational data by the “biased” allocation of “high-risk” (Killip class >1) patients to percutaneous coronary intervention. However, as we shall see, the observed disparity might just as well imply the failure of randomization to guarantee equal allocation. Although none of this is new to statisticians, epidemiologists, and trialists, it is largely underappreciated by clinicians. This has substantial consequences for evidence-based clinical practice.
The purpose of this essay, therefore, is to review the operational distinctions between randomized trials and unrandomized registries and to show how the attendant differences in allocation might impact on individual clinical decisions, population-based practice guidelines, and national health policy.
The Origins of Biased Allocation
The ideal clinical trial for deciding between alternative treatments would keep all conditions identical, except for the treatments themselves. Under these circumstances, any differences in outcome must be attributed to the differences in treatment and not to any extraneous factors. This, of course, is impossible. Patients and conditions will always differ in innumerable ways. Because this ideal trial cannot be performed in the real world, R.A. Fisher proposed a restrictive set of pragmatic standards.
- •
First, conditions are to be made as alike as possible with regard to those factors that are known to influence the outcome. These factors are thereby said to have been “controlled.”
- •
Second, to overcome the influence of other unknown factors (what we might call covariates, confounders, or nuisance variables), Fisher advocated the process of “randomization.” This requires that patients be allocated to alternative treatment groups according to some inherently random act (more on this below).
Over the ensuing decades, Fisher’s pragmatic standards have become the foundation of evidence-based medicine—despite some serious limitations. First, randomization does not in any way “guarantee” equal allocation of all covariates; it simply minimizes the chance of unequal allocation. As a result, measured covariates often exhibit statistically significant differences in magnitude or frequency in published randomized trials especially when sample size is large, and this is just as likely to be the case for the infinity of unmeasured covariates. In fact, Fisher’s justification for randomization was not as a guarantee of the equal allocation of these covariates, but “…of the validity of the [subsequent] test of significance” against corruption by any residual unequal allocation.
Second, we must assume that no clinically important exclusions occurred before the randomization step (such as the removal from consideration of a relevant group of potential treatment candidates). As an extreme example, imagine women were excluded from some trials because they were assumed to be at low risk relative to men. Can we then use the results of this “misogynistic” trial to justify the subsequent withholding of treatment in women? Obviously not.
Third, we must also assume that no meaningful differences occurred after the randomization step (such as more intensive adjunctive treatment in some group vs the other). This was a hotly debated issue in a recent trial comparing optimal medical therapy and percutaneous coronary intervention in patients with chronic stable angina. This requirement was well appreciated by Fisher who recommended that the randomization take place at “…the last in time of the stages in the physical history of the objects which might affect their experimental reaction.” The process of “double-blinding” can serve this purpose when the treatments are outwardly indistinguishable (drug vs placebo), but this is often not the case (medicine vs surgery).
Finally, we require the process of randomization to depend on a completely impartial act such as the flip of the proverbial “fair coin,” in which case we expect the heads (H) and tails (T) to be distributed (in the limit) in a 50-50 fashion in compliance with the binomial theorem and the law of large numbers. Consequently, if we examine a series of 2 successive tosses, we expect each of the 4 possible outcomes, HH, HT, TH, and TT, to occur (in the limit) with probability 1/4. Similarly, if we examine a series of 3 successive tosses, we expect each of the 8 possible outcomes, HHH, HHT, HTH, HTT, THH, THT, TTH, and TTT, to occur with probability 1/8. In general, then, for any number of consecutive tosses (n), we expect the 2 n possible outcomes to occur with probability 1/2 n . As reasonable as this seems, however, it has been mathematically proven that no sequences are truly random in this sense. As a result, the answer to even the most objective of questions can depend on seemingly innocent choices regarding the details of randomization ( Figure 1 ). Such choices can have a major influence on the measurement of treatment benefit in randomized trials and observational registries.
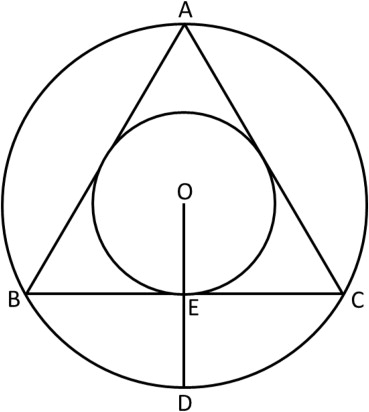
The Measurement of Treatment Benefit
Clinical trialists commonly measure treatment benefit in terms of the relative risk or risk ratio (RR)—the probability of an outcome event given exposure to some set of factors versus its probability given nonexposure
By expressing this simple ratio in the precise notation of conditional probability, the differences between its determination in randomized trials and observational registries become apparent. With respect to a randomized trial, p nonexposed is represented by p(O|r) and p exposed is represented by p(O|r, t), where O is the outcome of interest, r is the set of measured covariate factors under the control of randomization plus the set of unmeasured covariate factors assumed to be under the control of randomization, and t is the set of exposure factors (the qualitative and quantitative details of the treatment under investigation). Accordingly, the RR in a randomized trial is
In an observational registry, the RR is represented similarly as
Here, however, R, the set of measured and unmeasured covariates not controlled by randomization, replaces r. Accordingly, if we ignore any differences in t, the RR in both the randomized trial and the observational registry is conditioned on comparative differences in the set of covariates (many of which are unknown or unmatched), and when these sets differ (R ≠ r), the RRs will differ (RR trial ≠ RR registry ).
The Measurement of Treatment Benefit
Clinical trialists commonly measure treatment benefit in terms of the relative risk or risk ratio (RR)—the probability of an outcome event given exposure to some set of factors versus its probability given nonexposure
By expressing this simple ratio in the precise notation of conditional probability, the differences between its determination in randomized trials and observational registries become apparent. With respect to a randomized trial, p nonexposed is represented by p(O|r) and p exposed is represented by p(O|r, t), where O is the outcome of interest, r is the set of measured covariate factors under the control of randomization plus the set of unmeasured covariate factors assumed to be under the control of randomization, and t is the set of exposure factors (the qualitative and quantitative details of the treatment under investigation). Accordingly, the RR in a randomized trial is
In an observational registry, the RR is represented similarly as
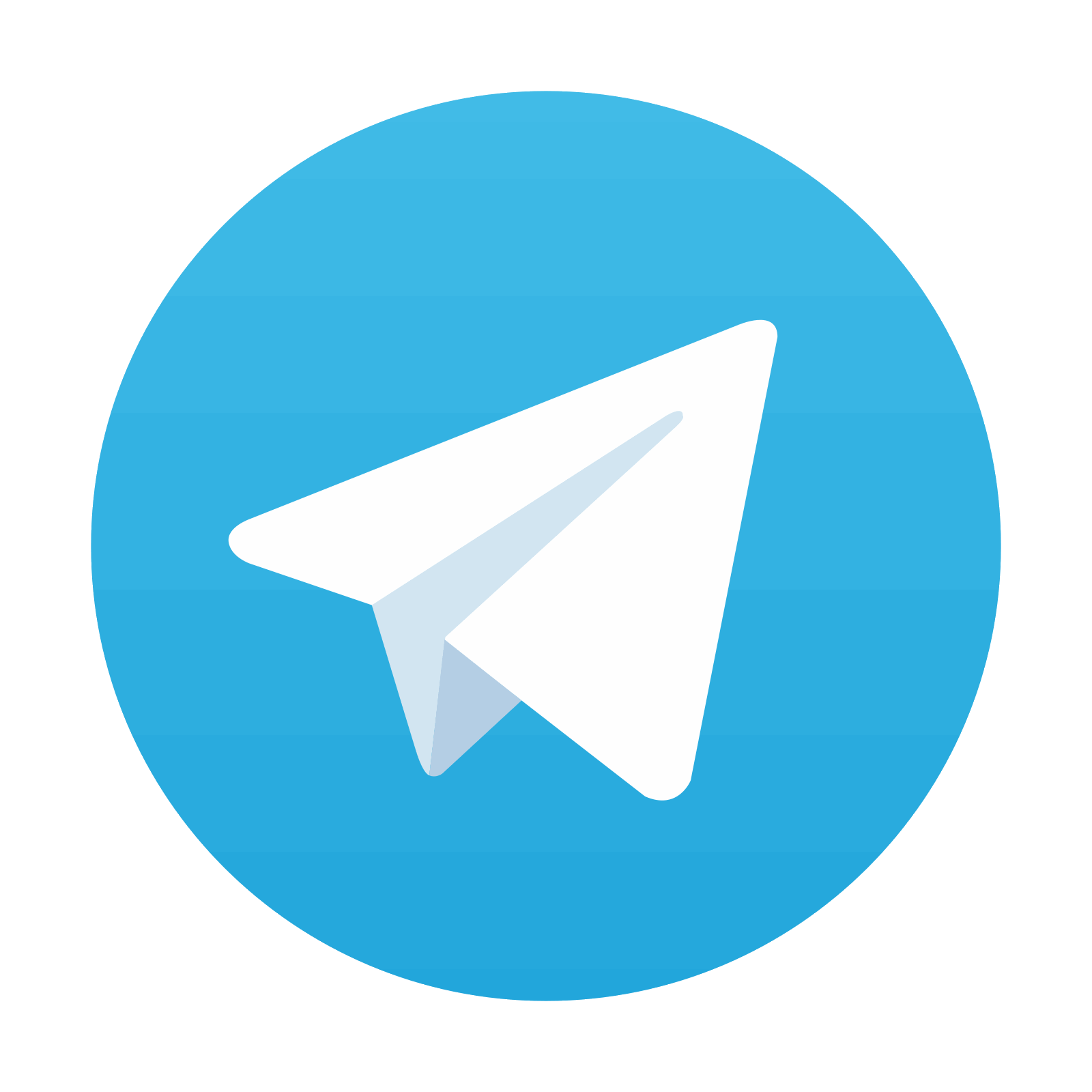
Stay updated, free articles. Join our Telegram channel

Full access? Get Clinical Tree
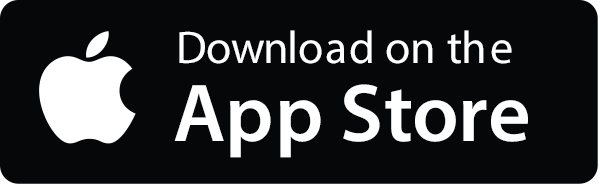
