CT Assessment of Lung Nodules—CT Versus Projection Radiography (PR)
This chapter will describe the importance of detecting and assessing the risk of lung cancer in a lung nodule by lung CT AI. The diameter of the pulmonary nodule was the first widely used research and clinical quantitative CT (QCT) metric derived from chest CT scans and predated the use of clinical QCT metrics of diffuse lung disease by several decades. This chapter introduces the topic of the solitary pulmonary nodule and the importance of assessing these nodules using multiple QCT metrics that help in determining the malignant potential of a lung nodule.
A lung nodule is a spherical structure of soft tissue density, approximately 40 HU, replacing the normal lung tissue with a diameter between 3 and 30 mm ( Fig. 4.1 ). The important features of a lung nodule include size, shape, density, contour, texture, and also assessing tissue features of the lung tissue adjacent to the lung nodule ( Box 4.1 ). It is very common to detect a nodule in screening populations that are at high risk for lung cancer. At least one nodule is detected in up to 51% of these patients, and greater than 95% of these nodules are benign. For this reason, there has been much research into determining what specific CT features of a lung nodule determine if it is benign or malignant. The size of the solitary pulmonary nodule is a simple feature that is a very strong predictor of the risk that the etiology of the nodule is due to lung cancer.

- ■
Shape
- ■
Size
- ■
Density in HU
- ■
Contour
- ■
Texture
- ■
Adjacent lung parenchymal density and texture
The detection and assessment of pulmonary nodules were an early strength of x-ray CT due to the true 3D assessment of the lung anatomy and increased contrast resolution compared to 2D x-ray projection radiographic imaging. The chest CT scan provides a 3D visualization of the lungs compared to the 2D representation that projection imaging generates, such as digital chest radiography. When a frontal and lateral 2D digital projection image of the chest is obtained on a person with suspected lung disease, the visual interpretation relies on the expert imaging physician being able to generate a 3D image of the lung anatomy in their brain, and this has its limitations. The chest CT is a more capable imaging method in the detection, localization, and assessment of nodule characteristics compared to projection radiography. This is due to chest CT’s accurate 3D depiction of the lung anatomy, high tissue contrast, compared to projection radiography, and the quantitative assessment of nodule features that are determined by the CT voxel size and HU value assigned to each voxel.
There are multiple clinical situations where one or more lung nodules may be detected on chest CT scans. The etiology of the lung nodule is most commonly due to prior infection, current infection, lung cancer, or metastatic cancer. There are multiple clinical scenarios, each with important considerations in the assessment of lung nodule(s). We will focus on two of these clinical scenarios: incidental lung nodules detected on noncontrast chest CT scans, and lung nodules detected on noncontrast low-dose chest CT scans as part of an approved ACR lung cancer screening program for high-risk smokers.
CT Protocol to Assess Lung Nodules
Nodule detection and the assessment of nodule size, shape, contour, density, and texture are important because lung cancer commonly presents as a solitary lung nodule, and the size and other CT features of the nodule can help in determining if the nodule is cancer or due to a benign tumor or infection. The best treatment approach is to detect a cancerous lung nodule when it is small and have it removed before it has spread to other structures within and/or outside of the thorax. Accurately detecting and following the size and other important features of a lung nodule require adherence to recommended CT scanning protocols, CT scanner quality assurance, and routine use of CT scanner lung nodule phantoms or test objects. A CT slice thickness of 2 mm or less is required to assess important features of the lung nodule. The size of a lung nodule increases on expiration and decreases on inspiration, so consistent inspiratory effort is necessary for the initial assessment of lung nodules and to accurately follow the size of the lung nodule over time. It has been reported that the mean change in nodule volume increases 23% between inspiration and expiration. The size, density, contour, and textural pattern of lung nodules are affected by slice thickness and reconstruction kernel. A neutral reconstruction kernel and narrow slice thickness represent the most accurate way of assessing lung nodule size, density, contour, and texture. A larger slice thickness (>2 mm) will reduce the size of the nodule due to partial volume effects at the surface of the nodule. The smaller the nodule, the greater this effect. The smooth kernel and large slice thickness (>2 mm) will average out important density, contour, and textural features. A sharp kernel will introduce artifacts within the nodule that will affect the accuracy of density and texture measurements. There are well-established quantitative criteria for the assessment of lung nodule size, growth, density, contour, and morphology.
CT Determination of Lung Nodule Size
The size of a lung nodule is a powerful predictor of whether a lung nodule is benign or due to a malignant cancer. The larger the nodule size, the greater the risk of lung cancer ( Fig. 4.2 ). Multiple research studies have shown that the risk of lung cancer in a small lung nodule (<5 mm in diameter) is negligible, even in high-risk patients, or negligible for a new nodule. The odds of a lung nodule being malignant increase between 1.1 and 1.15 times for every 1 mm increase in diameter above 4 mm. The prevalence of malignancy in nodules equal to or greater than 20 mm is greater than 64%. Currently, the QCT determination of lung nodule size is most commonly assessed by measuring the diameter of the nodule, and this is most commonly performed by the expert imaging physician visually interpreting the chest CT scan. The recommended interactive quantitative method to determine the average diameter of a lung nodule that is less than 10 mm in diameter is first to determine the longest diameter of the nodule on an axial, coronal, or sagittal lung CT image and then use the same imaging plane to obtain the shortest one-dimensional diameter of the nodule. Then the longest and shortest diameters of the nodule are used to calculate the average diameter of the nodule. Accurate linear measurements of anatomic structures on chest CT images, including nodule diameters, can be done using the interactive AI measuring tools available in the picture archiving and communication system (PACS) software. PACS used by expert imaging physicians to quantitatively assess the size of lung nodules detected on chest CT scans. This is the method recommended in the American College of Radiology Lung Imaging Reporting and Data System, Lung-RADS. There are AI agent software programs that can automatically calculate the diameter and volume of a lung nodule, but these AI agents require very specific CT scanning protocols to accurately determine the average diameter and volume of the lung nodule. The size of a lung nodule can change by simply using a different AI software program; by using expiratory instead of an inspiratory CT scan; or by using a thicker slice thickness instead of a thin slice thickness, and between standard and reduced dose CT scanning protocols. The volumetric approach is the best approach if the technical issues are all addressed properly.

CT Determination of Nodule Growth
The growth of a solid soft tissue lung nodule on sequential chest CT scans indicates that this is an active lesion, and the two most common etiologies are active infection or cancer. A solid soft tissue lung nodule that remains stable in size for over 2 years is presumed to be benign. There are also ground-glass and part-solid type nodules. A ground-glass nodule has increased density compared to a normal lung, but the normal lung vessels and outer airway wall can still be seen within the confines of the ground-glass nodule. The part-solid nodule has both ground-glass and solid soft tissue components. The solid soft tissue component obscures the normal lung vessels and the outer wall of airways. Ground-glass nodules and part-solid nodules equal to or greater than 6 mm need to be followed for a longer period of time, typically up to 5 years, to confirm they are benign. The growth of the nodule can be determined by assessing the interval increase in the nodule’s diameter, area, or volume. Lung CT AI software that assesses the volume of the nodule at multiple time points improves the accuracy of assessing nodule growth when compared to manual measurements of nodule diameter, area.
The time it takes a nodule to double in volume, volume doubling time (VDT), is helpful in determining if a solid soft tissue lung nodule is benign or malignant. Most malignant solid soft tissue lung nodules have a VDT between 20 and 400 days. Most nonmalignant lung nodules will have a doubling time less than 20 days or more than 400 days. The VDT of ground-glass nodules has been reported to be 628.5 days, and the VDT for part-solid nodules has been reported as 276.9 days. It is important to recall from the above discussion on the assessment of lung nodule size that nodule volumetric software using specific CT scanning protocols will produce the best results in assessing the VDT of lung nodules over time, but the same software and rigorous CT scanning protocol need to be used at each time point.
Milanese et al. (2018) reported the results of using the ClearRead lung CT AI nodule volume program (ClearRead CT, Riverain Technologies, Miamisburg, OH, USA) on nodules detected using chest CT. The chest CT scan protocol used the Siemens SOMATOM Force third-generation dual-source MDCT scanner is 100 kVp with tin filtration, reference tube-current product of 45 mAs, pitch of 1.2, collimation 96 × 0.6 mm, and a gantry rotation time of 0.5 seconds. The reconstructed image matrix was the standard 512 × 512. The reconstructed images used a slice thickness of 2 mm, slice increment of 1.6 mm, and the B64 sharp reconstruction kernel with the advanced modeled iterative reconstruction (ADMIRE) strength of 3. The ClearRead AI program removes the vessels from the lung CT images to enhance the detection and increase the accuracy of the nodule size measurements made interactively by expert imaging physicians, and by software programs designed to determine the volume of lung nodules seen on chest CT images. Nodules that are ill defined or adjacent to vessels are more difficult for AI nodule segmentation programs to characterize accurately. The standard chest CT images are referred to as SCT. The ClearRead processed lung CT images, which have the vessels and airways removed, are referred to as vessel suppressed CT (VSCT). The study included 93 patients that ranged in age from 20 to 80 years with a median age of 56 years. There were 24 females and 59 males. Seventy-seven solid soft tissue nodules were detected in 43 of the 93 subjects. Semiautomated measurements of nodule volume were done on 77 nodules. Manual correction of nodule volume segmentation was necessary in 49 of the 77 nodules (75%). Twelve nodules were excluded from the analysis because their volume exceeded 400 mm 3 . The 65 remaining nodules varied in volume from 13 to 366 mm 3 .
Each SCT and VSCT set of images were transferred to a workstation that was also running a dedicated computer-aided nodule detection software program, MM Oncology. One radiologist and the MM Oncology software program were both used to identify the nodules on the SCT images. The MM Oncology program was also used to semiautomatically calculate nodule maximum diameter, corresponding maximum orthogonal diameter, and volume for the SCT dataset. The radiologist used a five-point scale to assess the likelihood that each of the nodules detected by either the radiologist viewing the SCT images or the MM Oncology software program processing the SCT images were, in fact, lung nodules. The nodule scores assigned by the radiologist were: (1) no nodule, (2) no confident nodule, (3) probable nodule, (4) more definite nodule, and (5) definite nodule. Those nodules with a score of 3 or more were included in the study. The radiologist also indicated the location of the nodule: subpleural, adjacent to fissures, within 2 cm of the pleura, and central nodules. Contact between the nodule and a pulmonary vessel was also noted. The spatial coordinates of the included nodules were also included to allow for the matching of nodules on the VSCT-processed images.
Then, the radiologist and a radiologist resident independently performed semiautomatic segmentations of the 65 solid soft tissue nodules in the study using both SCT with MM Oncology software and VSCT. The volumes and longest diameters obtained using SCT and MM Oncology software by each reader were averaged, and these QCT metrics formed the reference standard to evaluate how well the ClearRead VSCT AI software performed.
The semiautomated volumetric lung nodule measurements on VSCT for the radiologist and the radiology resident showed substantial agreement when compared to the SCT/MM Oncology reference standard. There was nearly perfect agreement for the volumetric assessment of central nodules and nodules adjacent to a pulmonary vessel between the two readers using VSCT, compared to SCT/MM Oncology. There was substantial agreement for the volumetric assessment between the two readers for peripheral nodules and subpleural/perifissural nodules, compared to the SCT/MM Oncology reference standard. The longest diameter of each nodule showed substantial agreement between VSCT and SCT/MM Oncology for both the radiologist and the radiology resident.
The results of this study show that two independent methods of assessing nodule volume are in close agreement for both a radiologist and a radiology resident. The remaining challenge is to develop accurate, fully automatic segmentation of lung nodules to assess their volume and other desired size metrics over time, to help determine if the nodule is benign or malignant.
CT Determination of Nodule Density
The density of a soft tissue lung nodule is about 40 HU, which is similar to the CT density of muscle or solid organs. The visual or quantitative density assessment of a lung nodule is most reliable when a neutral reconstruction kernel is used, the slice thickness needs to be equal to or less than 2.5 mm but a slice thickness of 1.0 mm or less is preferred, if possible. The density features of a lung nodule can be assessed visually or quantitatively. The presence of visible fat in the lung nodule, Hounsfield number range −140 HU to −30 HU, indicates that the nodule is most likely a benign hamartoma or, rarely, a lipoma. The presence of certain patterns of calcium in the lung nodule can indicate that it is not a malignant tumor but due to prior infection, or it is a benign tumor of the lung. Calcium has a CT density number greater than 175 HU. There are four patterns of calcification that suggest the nodule is benign diffuse, central (<10% calcified), symmetric lamellar, and symmetric popcorn ( Fig. 4.3 ). The first three patterns suggest the nodule is due to prior granulomatous disease infection of the lung, such as mycobacterium tuberculosis or fungal infection. The fourth configuration, popcorn, can be seen most commonly in patients with a benign hamartoma-type tumor of the lung. There are occasions when other patterns of calcification occur in a lung nodule, but these can be seen in both malignant tumors and benign causes of lung nodules.
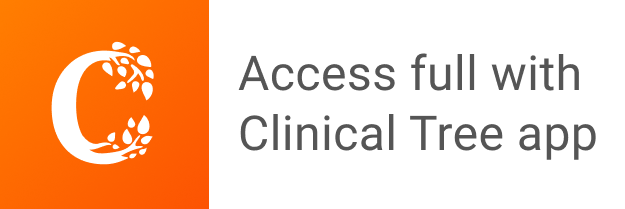