, Dilip R. Karnad2 and Snehal Kothari3
(1)
Cardiac Safety Services Quintiles, Durham, North Carolina, USA
(2)
Research Team, Cardiac Safety Services Quintiles, Mumbai, India
(3)
Cardiac Safety Services Global Head, Cardiac Safety Center of Excellence Quintiles, Mumbai, India
The modifications of the proarrhythmic cardiac safety paradigm discussed in this chapter facilitate a move away from an environment in which a dedicated TQT study is conducted in later phases of clinical development towards one in which intensive ECG evaluation is conducted in the early phase of drug development during first-in-human studies.
8.1 Introduction
As has been noted several times, the third revision to the ICH E14 “Questions & Answers” document (ICH E14 Q&A R3) was released in December 2015. While QTc-concentration relationship analysis had assisted regulatory decision-making on a case-by-case basis for several years by that time (Garnett et al. 2008; Zhang et al. 2015), the release of ICH E14 Q&A R3 was a milestone in the domain of proarrhythmic cardiac safety. Its release validated widespread acknowledgment of the benefits of this methodology and created an alternative primary methodology for the evaluation of a drug’s clinical QTc prolongation liability that can be employed much earlier in its clinical development program. Events occurring in the last few years leading to ICH E14 Q&A R3’s release, which we collectively refer to as the Early QTc Assessment Initiative, are described in this chapter by discussing several publications that preceded its release: ICH E14 Q&A R3 itself is then addressed in Sect. 8.7.
8.2 Advantages of Exposure–Response Modeling as a Primary Methodology in Clinical Proarrhythmic Cardiac Safety Assessment
Exposure–response modeling, which is already regularly employed in other aspects of drug development (e.g., modeling the impact of drug–drug interactions and other intrinsic and extrinsic factors that can impact exposure, evaluating new formulations), allows data to be analyzed across multiple cohorts and potentially multiple studies, in a single model. This is an important feature of the methodology since most Phase I studies use small cohorts for a given dose level and pool placebo data across cohorts (Bloomfield 2015). As an example, consider a sponsor who wishes to examine whether any of three doses of a drug, Dose A, Dose B, and Dose C, have an effect on QTc. Three cohorts of eight participants might be selected, one for each dose. In each case, a 3:1 randomization ratio would be employed to randomize six participants to the drug and two to placebo. This ratio maintains randomization while also permitting drug-related QTc data to be gathered from 75 % of those participating in the trial. Once all participants have completed the trial, six will have received each dose, and six will have received placebo. Exposure–response modeling using all the data collected would then permit estimation of the relationship between drug exposure and its effect (if any) on QTc over a wide range of concentrations.
This approach contrasts with the methodology employed by the TQT study in which attention falls on a specific set of time points for two specific doses, the proposed therapeutic dose and the chosen supratherapeutic dose. The intersection–union test treats dose and time points as independent categorical variables, ignoring the influence of drug concentration per se. This is an anomaly from the perspective of biological plausibility since most drugs prolong QTc as a function of plasma concentration. While the issues of multiplicity arising from evaluating QTc at every time point for both doses are appropriately addressed by the methodology underpinning the intersection–union test (as discussed in Chap. 5), it nonetheless can yield a bias towards false-positive results since it ignores reductions in QTc: in contrast, exposure–response modeling avoids such bias (Bloomfield 2015).
Exposure–response modeling also has additional advantages. Early-phase single ascending dose (SAD) and multiple-ascending dose (MAD) studies often explore the highest concentrations ever tested in humans. Over the last few years, some large pharmaceutical companies have started collecting robust QTc data in SAD and MAD studies by incorporating the elements of rigorous ECG collection and analysis utilized in TQT studies. To date, for the companies that have done so, this strategy has facilitated early but somewhat idiosyncratic internal decision-making regarding the viability of continuing with a drug’s development program: some sponsors have likely terminated what could have been useful drugs with acceptable benefit–risk balances at the first hint of QTc liability. A formalized approach to rigorous early QTc assessment via the collection of robust QTc data and the employment of prespecified exposure–response models detailed in statistical analysis plans has the potential to provide useful information as early in a clinical development program as possible and hence facilitate a more structured, rational, and consistent approach to early go/no-go decision-making (Turner et al. 2015). While this approach requires collecting high-quality ECGs alongside pharmacokinetic sampling in one or more Phase I studies, the ability to extract ECGs from digital 24-h ECG recordings makes this feasible in most Phase I studies (Bloomfield 2015).
8.3 Discussions of Exposure–Response Modeling in the Second Revision of the ICH E14 “Questions & Answers” Document
Now that ICH E14 Q&A R3 has been released, the previous versions of this document are not posted on the ICH web site per se. However, the respective questions asked and answered in the original document and each of the three revisions can be identified within ICH E14 Q&A R3 by looking at the “Date of Approval” column for each question and associated answer:
Questions and answers in the original document are identified by the date June 2008.
Those in the first revision are identified by the date April 2012.
Those in the second revision (ICH E14 Q&A R2) are identified by the date March 2014.
Those in ICH E14 Q&A R3 are identified by the date December 2015.
One of the questions in ICH E14 Q&A R2 addressed the topic of exposure–response modeling. The relevant questions can be paraphrased as follows:
The answer presented can be paraphrased as follows:
The 2005 ICH E14 Guideline states that analysis of the relationship between drug concentration and QT/QTc interval changes is under active investigation. Has this investigation yielded a reasonable approach to concentration-response modeling during drug development? How can exposure–response modeling guide the interpretation of QTc data?
Exposure-response modeling can be an important component of a ‘totality of evidence’ assessment of the risk of QTc prolongation. It can be evaluated in early-phase studies and as part of the conventional study of QTc prolongation, and may help inform further evaluation. There are many different types of models for the analysis of concentration-response data, including descriptive pharmacodynamic (PD) models and empirical models that link pharmacokinetic (PK) models (dose-concentration-response) with PD models.
An understanding of the exposure-response relationship can help predict the QTc effects of doses, dosing regimens, routes of administration, or formulations that were not studied directly. Interpolation within the range of concentrations studied is considered more reliable than extrapolation above the range.
If the results for the study drug are ambiguous (e.g., QTc prolongation at lower dose but no prolongation at higher dose, or QTc prolongation at a single isolated time point), exposure-response analysis can help interpret the data.
Careful replicate ECG collection with concurrent plasma drug concentrations measured in SAD and MAD studies provide a unique opportunity to evaluate potential drug-induced QTc effects over a broad range of doses and concentrations that might not be evaluated again during the drug development process. Pooling QTc and drug concentration data across doses, time, participants, and studies can yield large data sets to which PK-PD models can be applied to predict concentrations at which QTc prolongation could be clinically relevant. Exposure-response modeling applied on early clinical QTc data from healthy volunteers seems promising in terms of enhancing our confidence to characterize QTc prolongation.
Bloomfield (2015) commented upon the language used there, specifically that exposure–response modeling “seems promising.” Using the abbreviation ER for exposure–response modeling, he noted that “the ICH E14 working group was unable to agree on specific guidance on how ER modeling could be used for regulatory decision making in lieu of a TQT study. A key gap identified was the lack of data available to the working group to inform the relationship between the traditional endpoints used in a TQT study and ER modeling.”
See Shah and colleagues (2015) for additional commentary on ICH E14 Q&A R2.
8.4 An Illuminating Retrospective Study of QTc Exposure–Response Modeling
Zhang and colleagues provided insights regarding the numbers and types of studies evaluating QTc prolongation that were reported to the FDA between July 2006 and December 31, 2012 (Zhang et al. 2015). These studies were reviewed by their QT Interdisciplinary Review Team (QT-IRT), which was formed in 2006. The QT-IRT is responsible for reviewing study protocols and completed study reports addressing QTc liability assessment, including both TQT studies and non-TQT studies addressing this issue in the most feasible manner possible. Membership of the QT-IRT includes statisticians, clinical pharmacologists, medical officers, projects managers, and data managers. According to the intended indication for their candidate drug, sponsors submit QTc-related proposals and study reports to the appropriate therapeutic review division within FDA. The review division then forwards the document to the QT-IRT. Members review each document and give what is considered nonbinding advice to the review division. The division takes the QT-IRT’s comments and recommendations into consideration when forming its response to the sponsor and communicates the response directly to the sponsor (Turner and Durham 2009).
During the six-and-a-half-year time window reported in Zhang and colleagues’ paper, the QT-IRT reviewed nearly 1,500 QT-related documents, including protocols, meeting packages, and study reports. The total number of study reports was 277. Of these, 229 were TQT studies and 48 were non-TQT studies, studies that did not conform precisely to TQT study requirements but were nonetheless good faith attempts to assess QTc prolongation liability to the greatest degree possible in their particular circumstances.
The authors also presented the rationale for, and the results of, a retrospective investigation comparing TQT-like “negative” and “positive” designations for a large subset of these studies with the results of QTc-concentration relationship analysis using data from each respective study. From the 277 unique studies identified, 53 were excluded since pharmacokinetic information was missing, and one was excluded because of a clear case of hysteresis. Of the 223 studies therefore included in their analysis, 204 were TQT studies and 19 were non-TQT studies. Of the TQT studies, 141 employed a crossover design, and 63 employed a parallel design. The two overall findings were as follows (Zhang et al. 2015):
In 86 % of the cases where exposure–response analysis showed that the slope was not positive, i.e., there was no evidence of a relationship between drug concentration and QTc, the corresponding QT study had been designated as negative. This equates to a sensitivity of 86 %.
In 92 % of the cases where exposure–response analysis revealed a positive slope, indicating a relationship between drug concentration and QTc, the corresponding QT study had been designated as positive. This equates to a specificity of 92 %.
In the present context, sensitivity measures the proportion of actual negative studies that are correctly identified as not demonstrating a QT-concentration relationship, and specificity measures the proportion of actual positive studies that are correctly identified as demonstrating a QT-concentration relationship. For completeness, Table 8.1 presents results for analyses conducted by type of study.
Table 8.1
Results from the Zhang et al. analysis by study type
Study type | Sensitivity and specificity |
---|---|
All studies (N = 223) | 86 % and 92 % |
All TQT studies (204) | 86 % and 95 % |
Crossover design TQT studies (N = 141) | 88 % and 96 % |
Parallel design TQT studies (N = 63) | 82 % and 93 % |
Non-TQT studies (N = 19) | 86 % and 83 % |
Following appropriate discussion of the study’s limitations, the authors concluded that results from a QTc-concentration relationship analysis and those from the traditional ECH E14 analysis appear to be “strongly concordant” as long as the data collected and used in the QTc-concentration relationship analyses are of the same quality as those collected and used in ICH E14-guided analyses.
8.5 Prospective Evaluation of Exposure–Response Modeling: The IQ/CSRC Study
While retrospective analyses can certainly be very informative, prospective studies are typically awarded more gravitas. In 2013, a collaboration between the International Consortium for Innovation and Quality in Pharmaceutical Development (IQ) (IQ web site 2015) and the CSRC was initiated to design and conduct a clinical trial in healthy participants to investigate in a prospective manner whether or not it might be possible to replace the TQT study by rigorous ECG monitoring and exposure–response modeling of data generated from SAD studies or at least to provide an alternate to the TQT study. The design of the IQ/CSRC study was published in 2014 (Darpo et al. 2014a) and the results were published in 2015 (Darpo et al. 2015a).
8.5.1 Design of the IQ/CSRC Prospective Study
The IQ/CSRC Prospective Clinical Phase 1 Study was a three-period, blinded, randomized, placebo-controlled study in 20 healthy participants conducted in a design similar to a Phase I SAD study, with the primary objective being to estimate the effect of the drugs on the QTc interval using exposure–response analysis. Six marketed drugs with well-characterized QT effects were selected for the evaluation, including five “QT-positive” drugs and one “QT-negative” drug. The QT-positive drugs, known to prolong the QT interval, were ondansetron, quinine, dolasetron, moxifloxacin, and dofetilide: the QT-negative drug, which is not associated with QT interval prolongation, was levocetirizine.
The QT-positive drugs were chosen after discussions with FDA. Selection criteria included a drug’s toxicity profile (i.e., did it allow ethical administration of the drug to healthy participants?), lack of a substantial heart rate effect, and its degree of QTc prolongation. A lower dose administered on Day 1 was recommended by the FDA and was meant to achieve a mean placebo-corrected, change-from-baseline QTc (ΔΔQTc) of 9–12 msec. A higher dose, expected to result in ΔΔQTc of around 15–20 msec, was administered on Day 2. The higher dose was chosen to mimic a typical SAD study. In addition to similarity with a SAD design, the higher dose was intended to increase the precision of the slope of the estimated exposure–response model when data from the two dose levels were pooled. ECG recording, processing, and analysis were performed using rigorous methods as traditionally employed in TQT studies.
It was agreed ahead of time that if the results of the study were to show a positive QT-prolonging effect (upper bound of QTc change from baseline ≥10 ms at mean C max) by exposure–response modeling for all five QT-positive drugs, and additionally excluded a QTc effect for the negative control drug levocetirizine, it would be deemed to have met its objective successfully (Darpo et al. 2014a, b).
The 20 healthy participants were randomized to a three-period crossover study design, where they received three of the six study drugs or placebo in an incomplete block design that resulted in each study drug being administered to nine participants and placebo being administered to six participants in separate periods.
8.5.2 Results of the IQ/CSRC Prospective Study
Results from the study showed that the upper bound of the 90 % confidence interval (CI) of the mean predicted placebo-adjusted QTc change from baseline at geometric C max with all five QT-positive drugs exceeded 10 msec and that the slope of the exposure–response model was positive for all of these five drugs. In contrast, the upper bound for levocetirizine was less than 10 msec even when a single dose comprising six times the therapeutic dose was administered.
Using data from nine participants in each group treated with the study drug and six participants receiving placebo, the means (90 % CI) of the predicted ∆∆QTcF at geometric Cmax were as follows: 9.5 msec (7.2, 13.5) for ondansetron, 9.8 msec (6.7, 17.3) for quinine, 6.8 msec (3.4, 11.6) for dolasetron, 11.7 msec (10.6, 17.9) for moxifloxacin, 11.3 msec (6.1, 14.6) for dofetilide, and 2.0 msec (−2.6, 6.0) for levocetirizine.
While two participants received placebo in a crossover design in this study, first-in-human studies usually do not involve a crossover placebo period. After excluding these two participants, results for seven participants who had received the study drug or placebo in a parallel design were similar.
8.6 Concerns Expressed Following the Publication of the Results of the IQ/CSRC Study
While the IQ/CSRC Prospective Study certainly served as proof of concept, it had limitations and left some questions unaddressed (Turner et al. 2015). Some of these were discussed by Strnadova at the November 2014 DIA China Drug-Induced Cardiovascular Toxicity Workshop (Strnadova 2014):
- 1.
Clinically relevant plasma concentrations of the drug and its metabolites are usually not known in early-phase clinical development.
- 2.
Choice of ECG time points is limited by the lack of knowledge of the pharmacokinetics of the parent drug and metabolites.
- 3.
SAD studies may be too short to detect delayed effects.
- 4.
There is a need to demonstrate retrospectively that relevant concentrations and time points were studied.
- 5.
The absence of a positive control to verify assay sensitivity in the proposed early-phase QT studies raises concerns about the risk of false negatives, i.e., the study excludes a QT effect for a drug that truly has one.
- 6.
Exposure–response modeling is not standardized and the results can be operator and model dependent.
- 7.
The utility of this approach using challenging compounds remains to be evaluated. This includes drugs with prominent effects on heart rate, slow elimination, poor tolerability, and drugs that affect QT by mechanisms other than hERG channel blockade.Stay updated, free articles. Join our Telegram channel
Full access? Get Clinical Tree
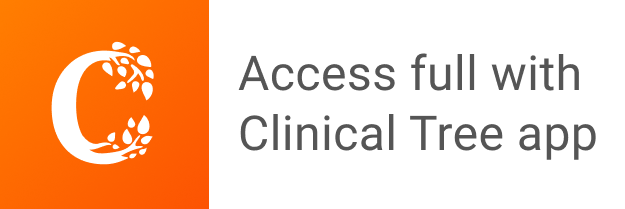