Although fractional flow reserve (FFR) and myocardial perfusion imaging (MPI) findings fundamentally differ, several cohort studies have revealed that these findings correlate. Here, we investigated whether flow-limiting FFR could be predicted from adenosine stress thallium-201 MPI with single-photon emission computed tomography (SPECT) findings derived from 84 consecutive, prospectively identified patients with stable coronary artery disease and 212 diseased vessels. Among them, FFR was measured in 136 diseased vessels (64%). The findings were compared with regional perfusion abnormalities including stress total perfusion defect (TPD) − rest TPD determined using quantitative perfusion single-photon emission computed tomography software. The FFR inversely correlated the most accurately with stress TPD − rest TPD ( r = −0.552, p <0.001). Predictors of major vessels of interest comprising FFR <0.80, included stress TPD − rest TPD, the transient ischemic dilation ratio, left ventricular ejection fraction at rest and beta blockers for left anterior descending artery (LAD) regions, and stress TPD − rest TPD, left ventricular mass, left ventricular ejection fraction at rest, right coronary artery lesions, the transient ischemic dilation ratio, and age for non-LAD regions. The diagnostic accuracy of formulas to predict major vessels of interest with FFR <0.80 was high (sensitivity, specificity and accuracy for LAD and non-LAD: 84%, 87% and 86%, and 75%, 93% and 87%, respectively). In conclusion, although somewhat limited by a sample size and a single-center design, flow-limiting FFR could be predicted from MPI findings with a defined probability. A cohort study might validate our results and provide a novel adjunctive tool with which to diagnose functionally significant coronary artery disease from MPI findings.
Fractional flow reserve (FFR) and perfusion abnormalities assessed by vasodilator stress myocardial perfusion imaging (MPI) with single-photon emission computed tomography (SPECT) fundamentally differ; the former represents the ratio of mean pressure at the distal epicardial coronary conduit of an atheromatous coronary lesion to that of the aorta in pharmacologically induced hyperemia, whereas the latter represents a change in myocardial blood flow (MBF) at hyperemia to MBF at rest which is affected not only by epicardial coronary perfusion but also by microcirculatory and myocardial properties. Some mismatches between FFR and findings of invasive coronary flow reserve measurements have been described, especially in patients with diffuse disease of the epicardial conduit vessels or disease of the coronary microvasculature. However, several group evaluations have found that FFR and perfusion abnormalities assessed by MPI with SPECT significantly correlate. Based on these lines of evidences, we postulated that flow-limiting FFR could be predicted from MPI findings. Recent advances in technology have allowed the introduction of promising tools for absolute MBF quantitative assessment using SPECT using tracer kinetics. However, applying such new technologies seems limited by the standard gamma camera for SPECT that is commonly encountered in clinical inventories. We aimed to predict flow-limiting FFR using quantitative perfusion SPECT (QPS) software after clarifying the relation between FFR and quantitative MPI findings.
Methods
We enrolled 84 consecutive, prospectively identified patients with stable coronary artery disease who underwent adenosine stress thallium-201 MPI with SPECT equipped with IQ-SPECT followed by elective FFR assessment at the Hiroshima Red Cross Hospital and Atomic-bomb Survivors Hospital (Hiroshima, Japan) from April 2013 to March 2014. A subset of this study total comprised 212 diseased vessels with angiographically suspected flow limitation. To incorporate all potentially flow-limiting coronary lesions, coronary lesions with a percent diameter stenosis ≥50% determined by quantitative coronary angiography (QCA, n = 178) were included along with lesions that were <50% stenosed, with visually interpreted morphologic abnormalities that might cause flow limitations such as in-stent or stent marginal (n = 8), hazy (n = 9), ulcerated (n = 5), and diffuse (>20 mm, n = 11) lesions as well as one lesion with kinked motion (n = 1). Among them, FFR was measured in 136 diseased vessels (64%). The guidewire could not pass through lesions in the remaining 76 vessels because of being located in branches (n = 26), chronic total obstruction (n = 22), distal and imposed technical difficulties (n = 20), being diffuse (n = 5) or tortuous (n = 2), or having an anomalous coronary origin (n = 1). Patients with acute coronary syndrome, previous coronary artery bypass surgery, and contraindications against adenosine were excluded. The Ethics Review Board of Hiroshima Red Cross Hospital and Atomic-bomb Survivors Hospital approved the study protocol (Approval No. 204), and all patients provided written informed consent to participate.
We measured FFR in patients within 4 weeks after MPI. All medications were discontinued 24 hours before, and the patients refrained from consuming foods or beverages containing caffeine for at least 12 hours before starting the study. We measured FFR using a standard 5Fr diagnostic or 6Fr guiding catheter without a side hole. A pressure transducer was standardized at zero, 5 cm below the sternum. The severity of coronary lesions was determined using QCA and CAAS5 software (Pie Medical Imaging, Maastricht, Belgium), and FFR was measured using a PressureWire Certus intracoronary pressure sensor-tipped guidewire (St. Jude Medical, Uppsala, Sweden). An intracoronary bolus of isosorbide dinitrate (0.5 mg) was administered; then, the pressure-monitoring wire was calibrated and advanced to the tip of the catheter to equalize pressure and introduced into the far distal part of identified coronary lesions. The FFR was derived online within a Radi Analyzer Unit (St. Jude Medical) from the ratio of a 5-beat average of mean distal coronary pressure to proximal aortic pressure at maximal steady state hyperemia. Maximal hyperemia was achieved through a continuous infusion of adenosine triphosphate through the brachial, femoral, or internal jugular vein from a weight-adjusted initial dose of 120 μg/kg/min and titrated to 210 μg/kg/min in 30 μg/kg/min increments for 3 minutes each until FFR reached a steady state. Uptitration proceeded in the event of anginal chest pain, extensive electrocardiographic change, severe hypotension (systolic blood pressure <70 mm Hg), or severe bradycardia (<40 beats/min). The lowest FFR was defined as representative of a diseased vessel.
Coexistent valvular heart disease and left ventricular mass were assessed in all patients by transthoracic echocardiography one day before FFR was assessed.
All patients enrolled in this study were assessed by MPI using a one-day stress/rest SPECT protocol with thallium-201. Stress was induced by a continuous 6-minute infusion of adenosine (140 μg/kg/min) through a brachial vein, and 111 MBq (3 mCi) of thallium-201 was injected 3 minutes after starting the adenosine infusion. Rest images were acquired 4 hours after acquiring stress images, and the patients remained supine throughout all image acquisitions. Gated SPECT images were acquired using a Symbia T6 SPECT/CT (Siemens Healthcare, Erlangen, Germany) equipped with an IQ-SPECT system (SPECT/CT-IQ-SPECT; Siemens Healthcare). Images were acquired over 208° in step mode using dual-head Anger cameras with 34 views (17 seconds/view). Acquisitions were gated for 12 frames per cardiac cycle. A 20% acceptance window was located only around the 70-keV photon peak. The raw gated SPECT data were ungated and reconstructed using iterative algorithms. The images were processed using QPS software (Cedars-Sinai Medical Center, Los Angeles, California). Left ventricular end-diastolic volume, left ventricular end-systolic volume, and left ventricular ejection fraction (LVEF) under stress and rest conditions were analyzed using quantitative gated SPECT software (Cedars-Sinai Medical Center). Transient ischemic dilation (TID) ratios were calculated as ratios of the left ventricular end-diastolic volume at stress to that at rest assessed by quantitative gated SPECT. None of the CT-based attenuation–corrected images was analyzed.
We quantitatively interpreted MPI images using the total perfusion defect (TPD) and the defect extent (Ext). The TPD is automatically derived by QPS, and it is designed to be equivalent to visual summed scores representing combined extent and severity on a continuous pixel-by-pixel basis. A score of 0.0 was assigned to pixels within normal limits, and a maximum score of 4.0 was assigned to all pixels with values >70% below the normal limit. The Ext was also automatically derived by QPS, and it represents Ext when a pixel value reduces 2.5 SDs below the normal limit. Coronary perfusion abnormalities were calculated by subtracting the rest, from the regional stress TPD (stress TPD − rest TPD) or the regional stress Ext (stress Ext − rest Ext). We compared averaged values from adenosine stress thallium-201 myocardial perfusion SPECT images of 72 patients (men, n = 36; women, n = 36) with angiographically proved normal coronary arteries and selected patients according to our inclusion criteria as institutional gender-independent reference databases for quantitation. Areas with perfusion abnormalities were assigned a coronary territory according to a 3-segment model (left anterior descending artery [LAD], left circumflex artery [LCX], and right coronary artery [RCA]) basically by the QPS software. The territory of a dominant LCX or dominant RCA was considered to represent the region, LCX + RCA. When lesions were identified in both the LAD and a diagonal branch where FFR was measurable only in the LAD, the LAD territory represented the septal + apex region according to a 5-segment (anterior, septal, inferior, lateral, and apical) model in the QPS software.
We developed the following formulas to predict diseased vessels in LAD and non-LAD regions with FFR <0.80 based on the results of multivariate logistic regression analyses:
- 1.
A LAD = −6.750 + 0.909 × [stress TPD − rest TPD, %] + 1.343 × [TID ratio] × 10 − 0.117 × [LVEF at rest, %] − 2.392 × [beta blockers],
- 2.
A non-LAD = 25.123 + 1.275 × [stress TPD − rest TPD, %] − 0.036 × [left ventricular mass, g] − 0.105 × [LVEF at rest, %] + 4.188 × [RCA lesions] − 0.967 × [TID ratio] × 10 − 0.112 × [age, years],
- 3.
Predicted value = 1/{1 + EXP (-A)},
Continuous and categorical variables are expressed as means ± SD and as ratios (%), respectively. Continuous variables between 2 groups were compared using unpaired t tests or nonparametric Mann–Whitney tests, and categorical variables were compared using chi-square tests. Receiver operating characteristic (ROC) curves were developed according to univariate or multivariate logistic regression models, and pairs of ROC curves were assessed by comparing the area under the curves (AUCs) and diagnostic accuracy at a predicted value of 0.50. Two logistic regression models were also compared by net reclassification analysis. To identify independent predictive factors of FFR <0.80, quantitative myocardial perfusion abnormalities, parameters obtained by MPI, and patients’ backgrounds ( Tables 1 and 2 ) were compared using unpaired t tests, Mann–Whitney tests, or chi-square tests between groups with FFR <0.80 and ≥0.80, and parameters with p >0.25 were excluded. Among the multicollinear candidate parameters, we applied that with the highest coefficient of determination (R 2 ) to FFR <0.80 as representative. Candidate parameters were analyzed using multivariate stepwise logistic regression analysis with a threshold of p ≤0.10. The statistical significance was p <0.001 in both multivariate analyses of the LAD and non-LAD. We otherwise considered p <0.05 as indicating a significant difference. Comparisons of AUCs between 2 ROC curves and net reclassifications were statistically assessed using the R statistical software packaged with pROC and PredictABEL (R 3.0.2, The R Foundation for Statistical Computing, http://www.r-project.org/ ), and other data were statistically analyzed using SPSS 21.0 for Windows software (SPSS Inc., Chicago, Illinois).
Men | 52 (62%) |
Age (years) | 73.5 ± 9.1 |
Height (cm) | 156.0 ± 10.4 |
Body weight (kg) | 60.5 ± 14.0 |
Body mass index (kg/m 2 ) | 24.6 ± 3.8 |
Body surface area (m 2 ) | 1.59 ± 0.22 |
Diabetes mellitus | 44 (52%) |
Hemoglobin A1c (%) | 6.4 ± 1.3 |
Hypertension | 77 (92%) |
Dyslipidemia | 70 (83%) |
Familial history of coronary artery disease | 29 (35%) |
Smoker | 46 (55%) |
Creatinine (mg/dL) | 0.97 ± 0.33 |
Estimated glomerular filtration rate (mL/min/1.73 m 2 ) | 58 ± 18 |
Hemodialysis | 9 (11%) |
Obesity (body mass index ≥ 25.0 kg/m 2 ) | 36 (43%) |
Atrial fibrillation | 3 (3.6%) |
Chronic heart failure | 29 (35%) |
Brain natriuretic peptide (pg/mL) | 251.0 ± 765.4 |
Prior myocardial infarction | 24 (29%) |
Prior percutaneous coronary intervention | 36 (43%) |
Echocardiography findings | |
Valvular heart disease | 6 (7.1%) |
Left ventricular mass (g) | 160 ± 46 |
Left ventricular mass index (g/m 2 ) | 101 ± 29 |
Coronary narrowings (%diameter stenosis ≥ 50%) determined by quantitative coronary angiography | |
Left main | 6 (7.1%) |
Left anterior descending | 63 (75%) |
Left circumflex | 39 (46%) |
Right | 52 (62%) |
Left main | 1 (1.2%) |
Left main + one-vessel disease | 1 (1.2%) |
Left main + two-vessel disease | 1 (1.2%) |
Left main + three-vessel disease | 3 (3.6%) |
No vessel disease | 5 (6.0%) |
One-vessel disease | 22 (26%) |
Two-vessel disease | 33 (39%) |
Three-vessel disease | 18 (21%) |
Co-existing chronic totally obstructive lesion | 21 (25%) |
Parameters determined by quantitative gated single-photon emission computed tomography | |
Left ventricular end-diastolic volume at stress (mL) | 80 ± 35 |
Left ventricular end-systolic volume at stress (mL) | 37 ± 27 |
Left ventricular ejection fraction at stress (%) | 58 ± 14 |
Heart rate at stress image (bpm) | 65.0 ± 12.5 |
Left ventricular end-diastolic volume at rest (mL) | 75 ± 34 |
Left ventricular end-systolic volume at rest (mL) | 33 ± 25 |
Left ventricular ejection fraction at rest (%) | 61 ± 15 |
Heart rate at rest image (bpm) | 67.0 ± 13.5 |
Transient ischemic dilation ratio | 1.10 ± 0.13 |
Quantitative analysis of perfusion abnormality of total heart using quantitative perfusion single-photon emission computed tomography | |
Stress defect extent (%) | 10.3 ± 12.1 |
Rest defect extent (%) | 6.4 ± 9.0 |
Stress – rest defect extent (%) | 3.9 ± 6.5 |
Stress total perfusion defect (%) | 9.4 ± 10.3 |
Rest total perfusion defect (%) | 6.2 ± 7.9 |
Stress – rest total perfusion defect (%) | 3.2 ± 5.3 |
Medications | |
β-Blockers | 21 (25%) |
Angiotensin converting enzyme inhibitors/Angiotensin receptor blockers | 57 (68%) |
Calcium-channel blockers | 48 (57%) |
Nitrates | 4 (4.8%) |
α-Blockers | 13 (16%) |
Statins | 61 (73%) |
Total | Fractional flow reserve < 0.80 | Fractional flow reserve ≥ 0.80 | |
---|---|---|---|
Number of vessels | 136 | 62 | 74 |
Fractional flow reserve findings | |||
Measured coronary arteries | |||
Left anterior descending | 69 (51%) | 38 (61%) ∗ | 31 (42%) |
Left circumflex | 32 (24%) | 11 (18%) | 21 (28%) |
Right | 35 (26%) | 13 (21%) | 22 (30%) |
Previous myocardial infarcted artery | 16 (12%) | 10 (16%) | 6 (8.1%) |
Adenosine triphosphate (μg/kg/min) | 175 ± 18 | 168 ± 18 ∗ | 181 ± 16 |
Aortic mean pressure at control (mmHg) | 92 ± 14 | 92 ± 13 | 93 ± 15 |
Distal coronary mean pressure at control (mmHg) | 80 ± 19 | 71 ± 18 ∗ | 88 ± 15 |
Aortic mean pressure at hyperemia (mmHg) | 76 ± 13 | 77 ± 12 | 76 ± 14 |
Distal coronary mean pressure at hyperemia (mmHg) | 59 ± 16 | 49 ± 14 ∗ | 67 ± 13 |
Fractional flow reserve | 0.77 ± 0.16 | 0.63 ± 0.13 ∗ | 0.89 ± 0.06 |
Parameters of perfusion abnormalities in vessel territories measured using quantitative perfusion single-photon emission computed tomography | |||
Stress defect extent (%) | 3.8 ± 7.2 | 6.3 ± 9.2 ∗ | 1.7 ± 4.1 |
Rest defect extent (%) | 2.4 ± 5.5 | 3.2 ± 6.5 ∗ | 1.7 ± 4.5 |
Stress – rest defect extent (%) | 1.4 ± 4.0 | 3.1 ± 5.2 ∗ | 0.0 ± 1.8 |
Stress total perfusion defect (%) | 3.2 ± 5.6 | 5.2 ± 7.0 ∗ | 1.4 ± 3.0 |
Rest total perfusion defect (%) | 2.2 ± 4.4 | 2.9 ± 5.3 ∗ | 1.6 ± 3.4 |
Stress – rest total perfusion defect (%) | 1.0 ± 2.8 | 2.4 ± 3.5 ∗ | 0.0 ± 1.4 |
Results
Tables 1 and 2 list the characteristics of patients and of 136 vessels in which FFR was measured, respectively. Lesions with FFR <0.80 had a smaller minimum lumen diameter and more percent diameter stenosis in lesions of the LAD, LCX, and RCA compared with those with FFR ≥0.80 assessed by QCA ( Supplementary Table 1 ). Table 3 summarizes correlations between quantitative parameters for myocardial perfusion abnormalities assessed by QPS software and FFR. The FFR inversely correlated the most accurately with stress TPD − rest TPD that were considered statistically moderate. Univariate logistic regression analyses showed that the diagnostic accuracy of stress TPD − rest TPD was highest at FFR <0.80 (sensitivity, 60%; specificity, 91%; accuracy, 77%; Figure 1 ). At FFR <0.80, The AUC and the diagnostic accuracy of stress TPD − rest TPD was larger (p = 0.019) and higher than those of stress Ext − rest Ext ( Figure 2 ), respectively. Thus, subsequent multivariate analysis included stress TPD − rest TPD at an FFR cutoff of <0.80.
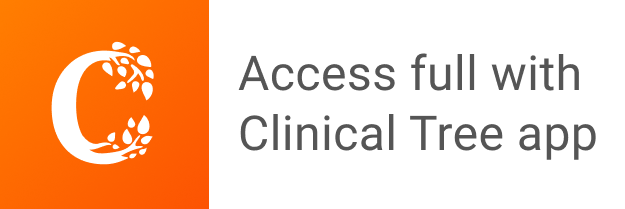