Disease management programs that target patients with the highest risk of subsequent costs may help payers and providers control health care costs, but identifying these patients prospectively is challenging. We hypothesized that medical history and clinical data from a heart failure registry could be used to prospectively identify patients with heart failure most likely to incur high costs. We linked Medicare inpatient claims to clinical registry data for patients with heart failure and calculated total Medicare costs during the year after the index heart failure hospitalization. We defined patients as having high costs if they were in the upper 5% (>$76,500) of the distribution. We used logistic regression models to identify patient and clinical characteristics associated with high costs. Costs for 40,317 patients in the study varied widely. Patients in the upper 5% of the cost distribution incurred mean costs of $117,193 ± 55,550 during 1 year of follow-up compared to $17,086 ± 17,792 for the lower-cost group. Demographic and clinical characteristics associated with high costs included younger age and black race; history of anemia, chronic obstructive pulmonary disease, ischemic heart disease, diabetes mellitus, or peripheral vascular disease; serum creatinine level; and systolic blood pressure at admission. Mean 1-year Medicare costs for patients whom the model predicted would exceed the high-cost threshold were >2 times the costs for patients below the threshold. In conclusion, a model based on variables from clinical registries can identify a group of patients with heart failure who on average will incur higher costs in the first year after hospitalization.
Disease management programs that target patients with the highest risk of subsequent costs may help payers and providers control health care costs. An inpatient episode of heart failure provides a unique opportunity to evaluate a patient’s eligibility for postdischarge disease management, but the value of using inpatient medical history and clinical data to identify high-cost patients is unclear. Therefore, we used a nationwide sample of Medicare beneficiaries with heart failure from the Organized Program to Initiate Lifesaving Treatment in Hospitalized Patients with Heart Failure (OPTIMIZE-HF) registry and the Get With the Guidelines–Heart Failure (GWTG-HF) registry to examine the value of clinical registry data to prospectively identify patients who are most likely to incur the highest costs.
Methods
We linked Medicare inpatient claims data from January 1, 2003, through December 31, 2006, with data from the OPTIMIZE-HF and GWTG-HF registries ( http://www.Clinicaltrials.gov , trial identifier NCT00344513 ). In 2005 OPTIMIZE-HF transitioned to GWTG-HF under sponsorship of the American Heart Association. The registries had the same design, inclusion criteria, and data-collection methods. Outcome Sciences, Inc. (Cambridge, Massachusetts), serves as the data-collection and coordination center for GWTG. The Duke Clinical Research Institute (Durham, North Carlina) serves as the data analysis center and has an agreement to analyze aggregate de-identified data for research purposes. Patients were eligible for inclusion in the registries if they were admitted for an episode of worsening heart failure or had developed significant heart failure symptoms during a hospitalization for which heart failure was the primary discharge diagnosis. Hospital teams used heart failure case-ascertainment methods similar to those used by the Joint Commission and submitted data on medical history, signs and symptoms, medications, contraindications for or intolerance to medications, and diagnostic test results by a Web-based registry. The representativeness and validity of the OPTIMIZE-HF registry have been described previously.
For each patient in this study we obtained Medicare inpatient, outpatient, carrier, and denominator files from 2003 through 2007. These files include institutional claims for facility costs covered under Medicare Parts A and B and noninstitutional claims for physician services covered under Medicare Part B. Denominator files include beneficiary demographic characteristics, dates of death, program eligibility, and enrollment information.
We used indirect identifiers to link data for patients ≥65 years old from the OPTIMIZE-HF and GWTG-HF registries with inpatient Medicare claims files, a method described previously by Hammill et al. Using this method we linked 62,311 (78%) of the 79,837 eligible OPTIMIZE-HF and GWTG-HF hospitalizations to Medicare inpatient claims. We included only patients living in the United States enrolled in fee-for-service Medicare who were discharged alive from a hospital participating fully in the OPTIMIZE-HF or GWTG-HF program. If a patient had multiple linked registry hospitalizations, we selected the first as the index hospitalization. The institutional review board of the Duke University Health System approved the study.
Patient-level information for risk adjustment was obtained from registry data and included patient demographic characteristics, medical history, and results of admission laboratory tests and examinations. Race was recorded on the case-report form as “Asian/Pacific Islander,” “black or African-American,” “Caucasian,” “Native-American,” “unknown,” or “other.” Consistent with previous analyses we collapsed the categories into “black” and “other.” Variables in this analysis had low rates of missing data (i.e., <5% of records) with the exception of evaluation of left ventricular function (13.3%). Because the risk of biased parameter estimates is minimal with missing rates <5%, we adopted an approach for missing variables used in the development of 30-day readmission and mortality risk models for patients with heart failure. For continuous variables and the variable for evaluation of left ventricular function we created categorical variables that included a category for missing values. For the dichotomous smoking history variable we imputed missing values to “no.”
We followed patients for 1 year after discharge from the index hospitalization. We calculated total costs to Medicare for subsequent hospitalizations by summing payment amounts and per-diem adjustments for inpatient stays within 1 year of the index discharge date. Similarly, we calculated total outpatient facility costs by summing payment amounts from outpatient claims and total physician services costs by summing payment amounts from carrier claims. We adjusted all costs to 2007 US dollars based on the Consumer Price Index. We calculated total costs to Medicare as the sum of inpatient, outpatient, and carrier costs and created a binary indicator of “high costs” as those in the top 5% of total Medicare costs (>$76,500) during 1 year of follow-up. We defined patients in this top 5% group as high cost and patients in the bottom 95% as lower cost.
We were also interested in all-cause mortality and readmission. We obtained mortality information from the Centers for Medicare and Medicaid Services denominator files. Using the inpatient claims files, we identified the first hospital readmission within 1 year after the index discharge date. Transfers to or from another hospital and admissions for rehabilitation (diagnosis-related group 462 or an admission diagnosis code of V57.xx) did not count as readmissions.
For patient characteristics we present categorical variables as frequency and continuous variables as mean ± SD and/or median with interquartile range. We used Kaplan–Meier methods to calculate unadjusted 30-day, 90-day, 180-day, and 1-year mortality and log-rank tests to compare differences between the high-cost and lower-cost groups. To account for the competing risk of death we used the cumulative incidence function to calculate unadjusted 30-day, 90-day, 180-day, and 1-year readmission at the patient level. We used Gray tests to assess differences in readmission rates between the high-cost and lower-cost groups.
We used logistic regression models with hospital-level random effects to examine unadjusted and multivariable relations between study variables and high costs. Covariates included age, female sex, and black race; medical history; clinical measurements at admission including systolic function, serum creatinine, systolic blood pressure, serum sodium, and hemoglobin; year of index hospitalization; and a variable indicating whether length of stay for the index hospitalization was >7 days. Hospital-level random effects account for the variance in cost among hospitals so the independent association between patient factors and cost can be estimated more accurately. In a sensitivity analysis we excluded patients who were discharged from the index hospitalization to a skilled nursing facility (n = 7,538) or hospice care (n = 637).
Using simple random selection we constructed a derivation cohort composed of 75% of the study population and a validation cohort composed of the remaining 25% of the study population. We initially developed logistic regression models in the derivation cohort and then used the results from these models to score records in the validation cohort. We evaluated the calibration and discrimination of all models in the 2 cohorts and refit the models for the entire study population. Based on the refitted model we generated predicted probabilities of incurring high costs in the year after the index hospitalization. We considered patients who were in the top 5%, top 10%, or top 25% of the predicted probabilities to be those whom the model predicted would incur high costs. At each threshold we calculated the model’s sensitivity (i.e., number of correctly predicted high-cost cases divided by total number of actual high-cost cases), false-positive rate (i.e., 1 minus the number of correctly predicted low-cost cases divided by the total number of actual low-cost cases), and positive predictive value (i.e., the number of correctly predicted high-cost cases divided by the total number of cases predicted as high-cost), and the mean observed total costs. We used SAS 9.2 (SAS Institute, Cary, North Carolina) for all analyses.
Results
After linking hospitalizations from OPTIMIZE-HF and GWTG-HF to Medicare inpatient claims and applying the exclusion criteria, the study population included 40,317 index cases ( Table 1 ). Ischemic heart disease, diabetes, and hypertension were the most common comorbid conditions. The 75% derivation cohort and the 25% validation cohort were comparable to the full cohort in patient characteristics (data not shown).
Age (years), mean ± SD | 79.7 ± 7.9 |
Women | 22,492 (55.8%) |
Race | |
Black | 4,096 (10.2%) |
Other | 36,221 (89.8%) |
Medical history | |
Anemia based on standardized laboratory assessments | 7,301 (18.1%) |
Atrial arrhythmia | 14,650 (36.3%) |
Chronic obstructive pulmonary disease | 11,259 (27.9%) |
Chronic renal insufficiency | 7,476 (18.5%) |
Coronary artery disease/ischemic heart disease | 21,041 (52.2%) |
Depression | 4,131 (10.2%) |
Diabetes mellitus | 15,844 (39.3%) |
Current or previous treatment for hyperlipidemia | 13,943 (34.6%) |
Current or previous treatment for hypertension | 28,745 (71.3%) |
Peripheral vascular disease | 5,684 (14.1%) |
Previous cerebrovascular accident/transient ischemic attack | 6,832 (16.9%) |
Smoker within previous year | 3,632 (9.0%) |
Clinical measurements at admission | |
Systolic function | |
Preserved systolic function | 20,128 (49.9%) |
Left ventricular systolic dysfunction | 14,841 (36.8%) |
Missing | 5,348 (13.3%) |
Serum creatinine (mg/dl), mean ± SD | 1.7 ± 2.2 |
Systolic blood pressure (mm Hg), mean ± SD | 141.7 ± 30.6 |
Serum sodium (mEq/L), mean ± SD | 137.3 ± 8.2 |
Hemoglobin (g/dl), mean ± SD | 12.1 ± 5.8 |
Length of stay for index hospitalization (days) | |
Mean ± SD | 5.5 ± 4.7 |
Median (interquartile range) | 4.0 (3.0–7.0) |
Index year | |
2003 | 9,319 (23.1%) |
2004 | 14,551 (36.1%) |
2005 | 6,445 (16.0%) |
2006 | 10,002 (24.8%) |
As presented in Table 2 , unadjusted 1-year mortality was 34.4% and 1-year readmission rate was 65.4%. Unadjusted 30-, 90-, and 180-day mortality rates were lower in patients who incurred the highest costs and unadjusted 1-year mortality was slightly higher in patients with high costs. Rate of readmission at 30 days was 2 times as high in the high-cost group compared to the lower-cost group. Nearly all patients in the high-cost group were readmitted within 1 year compared to 64% in the lower-cost group. Patients in the high-cost group incurred mean total 1-year costs of $117,193 and accounted for 27% of total Medicare costs in the study population. Overall the distribution of 1-year costs was right skewed and 30% of patients incurred <$5,000 in total costs.
Outcome | All Patients (n = 40,317) | Patients in Upper 5% of Medicare Costs (n = 2,029) | Patients in Lower 95% of Medicare Costs (n = 38,288) | p Value |
---|---|---|---|---|
Mortality | ||||
30 days | 3,106 (7.7%) | — ⁎ | 3,101 (8.1%) | |
90 days | 6,391 (15.9%) | 56 (2.8%) | 6,335 (16.6%) | <0.001 |
180 days | 9,460 (23.6%) | 212 (10.5%) | 9,248 (24.3%) | <0.001 |
1 year | 13,698 (34.4%) | 728 (36.5%) | 12,970 (34.3%) | 0.15 |
All-cause readmission, no. (cumulative incidence) | ||||
30 days | 8,693 (21.6) | 866 (42.7) | 7,827 (20.5) | <0.001 |
90 days | 15,765 (39.2) | 1,524 (75.1) | 14,241 (37.3) | <0.001 |
180 days | 20,924 (52.2) | 1,877 (92.6) | 19,047 (50.1) | <0.001 |
1 year | 26,100 (65.4) | 2,011 (99.3) | 24,089 (63.6) | <0.001 |
Costs to Medicare at 1 year | ||||
Inpatient | <0.001 | |||
Mean ± SD | 14,619 ± 23,866 | 86,400 ± 48,707 | 10,815 ± 13,660 | |
Median (interquartile range) | 6,400 (0−19,130) | 74,749 (59,976–98,803) | 5,780 (0–16,317) | |
Outpatient | <0.001 | |||
Mean ± SD | 2,572 ± 6,336 | 11,124 ± 14,447 | 2,119 ± 5,209 | |
Median (interquartile range) | 599 (78–1,838) | 3,438 (859–19,776) | 557 (67–1,675) | |
Carrier | <0.001 | |||
Mean ± SD | 4,932 ± 8,643 | 19,669 ± 30,347 | 4,151 ± 4,215 | |
Median (interquartile range) | 3,235 (1,412–6,316) | 16,134 (12,162–21,674) | 3,006 (1,327–5,695) | |
Total Medicare cost | <0.001 | |||
Mean ± SD | 22,124 ± 30,575 | 117,193 ± 55,550 | 17,086 ± 17,792 | |
Median (interquartile range) | 11,849 (3,190–29,324) | 100,779 (86,145–127,658) | 10,721 (2,861–25,445) |
Regression models were well calibrated for predicting which patients would have high costs. Predicted probability distributions for the derivation and validation cohorts were highly consistent and percentages of predicted and observed high-cost patients were similar within deciles ( Figure 1 ) . Table 3 presents results of the refitted unadjusted and multivariable models examining relations between patient characteristics and high-cost status. After adjustment, black race and a history of anemia, chronic obstructive pulmonary disease, ischemic heart disease, diabetes mellitus, and peripheral vascular disease were positively associated with greater odds of high costs in the year after the index hospitalization. Older age, because of its association with a higher risk of mortality, was associated with lower costs during 1-year follow-up. The odds of incurring high costs were >2 times as high in patients with serum creatinine levels ≥2 mg/dl compared to patients with serum creatinine levels <1.5 mg/dl. Systolic blood pressure >120 mm Hg was also associated with higher costs.
Variable | Unadjusted OR (95% CI) | p Value | Adjusted OR (95% CI) ⁎ | p Value |
---|---|---|---|---|
Age (years) | ||||
65–69 | 1.00 (reference) | 1.00 (reference) | ||
70–74 | 0.77 (0.68–0.88) | <0.001 | 0.79 (0.69–0.91) | <0.001 |
75–79 | 0.59 (0.51–0.67) | <0.001 | 0.62 (0.54–0.71) | <0.001 |
≥80 | 0.25 (0.22–0.29) | <0.001 | 0.28 (0.25–0.33) | <0.001 |
Women | 0.80 (0.73–0.88) | <0.001 | 0.96 (0.87–1.06) | 0.45 |
Race | ||||
Black | 2.15 (1.88–2.45) | <0.001 | 1.70 (1.48–1.96) | <0.001 |
Other | 1.00 (reference) | 1.00 (reference) | ||
Medical history | ||||
Anemia | 1.45 (1.29–1.62) | <0.001 | 1.18 (1.05–1.34) | 0.006 |
Atrial arrhythmia | 0.75 (0.68–0.83) | <0.001 | 0.91 (0.82–1.01) | 0.07 |
Chronic obstructive pulmonary disease | 1.22 (1.11–1.35) | <0.001 | 1.17 (1.05–1.30) | 0.003 |
Chronic renal insufficiency | 2.12 (1.92–2.35) | <0.001 | 1.10 (0.96–1.25) | 0.16 |
Coronary artery disease/ischemic heart disease | 1.27 (1.16–1.39) | <0.001 | 1.14 (1.04–1.26) | 0.007 |
Depression | 1.05 (0.90–1.23) | 0.50 | 1.05 (0.90–1.24) | 0.52 |
Diabetes mellitus | 1.68 (1.53–1.84) | <0.001 | 1.16 (1.05–1.28) | 0.003 |
Hyperlipidemia | 1.23 (1.12–1.35) | <0.001 | 1.04 (0.94–1.15) | 0.47 |
Hypertension | 1.17 (1.05–1.30) | 0.004 | 1.01 (0.91–1.14) | 0.80 |
Peripheral vascular disease | 1.41 (1.24–1.59) | <0.001 | 1.14 (1.00–1.29) | 0.05 |
Previous cerebrovascular accident/transient ischemic attack | 0.97 (0.85–1.09) | 0.57 | 0.94 (0.83–1.06) | 0.32 |
Smoker within previous year | 1.21 (1.04–1.41) | 0.01 | 0.90 (0.77–1.06) | 0.22 |
Findings on admission | ||||
Systolic function | ||||
Preserved systolic function | 1.00 (reference) | 1.00 (reference) | ||
Left ventricular systolic dysfunction | 1.07 (0.97–1.18) | 0.18 | 0.99 (0.89–1.10) | 0.82 |
Missing | 1.13 (0.98–1.30) | 0.09 | 1.12 (0.97–1.29) | 0.13 |
Serum creatinine (mg/dl) | ||||
<1.5 | 1.00 (reference) | 1.00 (reference) | ||
1.5–<2.0 | 1.32 (1.16–1.50) | <0.001 | 1.26 (1.10–1.44) | <0.001 |
≥2.0 | 2.93 (2.64–3.25) | <0.001 | 2.34 (2.05–2.67) | <0.001 |
Missing | 1.15 (0.57–2.35) | 0.70 | 1.20 (0.57–2.55) | 0.63 |
Systolic blood pressure (mm Hg) | ||||
<120 | 1.00 (reference) | 1.00 (reference) | ||
≥120–<140 | 1.10 (0.96–1.25) | 0.17 | 1.18 (1.03–1.35) | 0.02 |
≥140–<160 | 1.04 (0.90–1.19) | 0.61 | 1.10 (0.96–1.27) | 0.19 |
≥160 | 1.17 (1.03–1.34) | 0.02 | 1.18 (1.02–1.35) | 0.02 |
Missing | 0.99 (0.48–2.07) | 0.99 | 1.30 (0.63–2.68) | 0.48 |
Serum sodium (mEq/L) | ||||
<135 | 1.00 (reference) | 1.00 (reference) | ||
≥135–<145 | 1.15 (1.02–1.30) | 0.02 | 1.13 (1.00–1.29) | 0.05 |
≥145 | 1.05 (0.82–1.34) | 0.71 | 1.00 (0.78–1.30) | 0.97 |
Missing | 0.78 (0.46–1.32) | 0.35 | 0.90 (0.50–1.63) | 0.73 |
Hemoglobin (g/dl) | ||||
<9 | 1.00 (reference) | 1.00 (reference) | ||
≥9–<12 | 0.98 (0.81–1.17) | 0.79 | 1.14 (0.94–1.38) | 0.18 |
≥12 | 0.67 (0.56–0.81) | <0.001 | 0.92 (0.75–1.13) | 0.43 |
Missing | 0.64 (0.45–0.89) | 0.009 | 0.81 (0.56–1.17) | 0.26 |
Length of index stay >7 days | 1.24 (1.12–1.38) | <0.001 | 1.10 (0.99–1.23) | 0.08 |
Index year | ||||
2003 | 1.00 (reference) | 1.00 (reference) | ||
2004 | 1.13 (1.00–1.29) | 0.06 | 1.17 (1.03–1.34) | 0.02 |
2005 | 1.09 (0.90–1.32) | 0.36 | 1.15 (0.95–1.39) | 0.16 |
2006 | 1.04 (0.86–1.24) | 0.70 | 1.17 (0.97–1.40) | 0.11 |
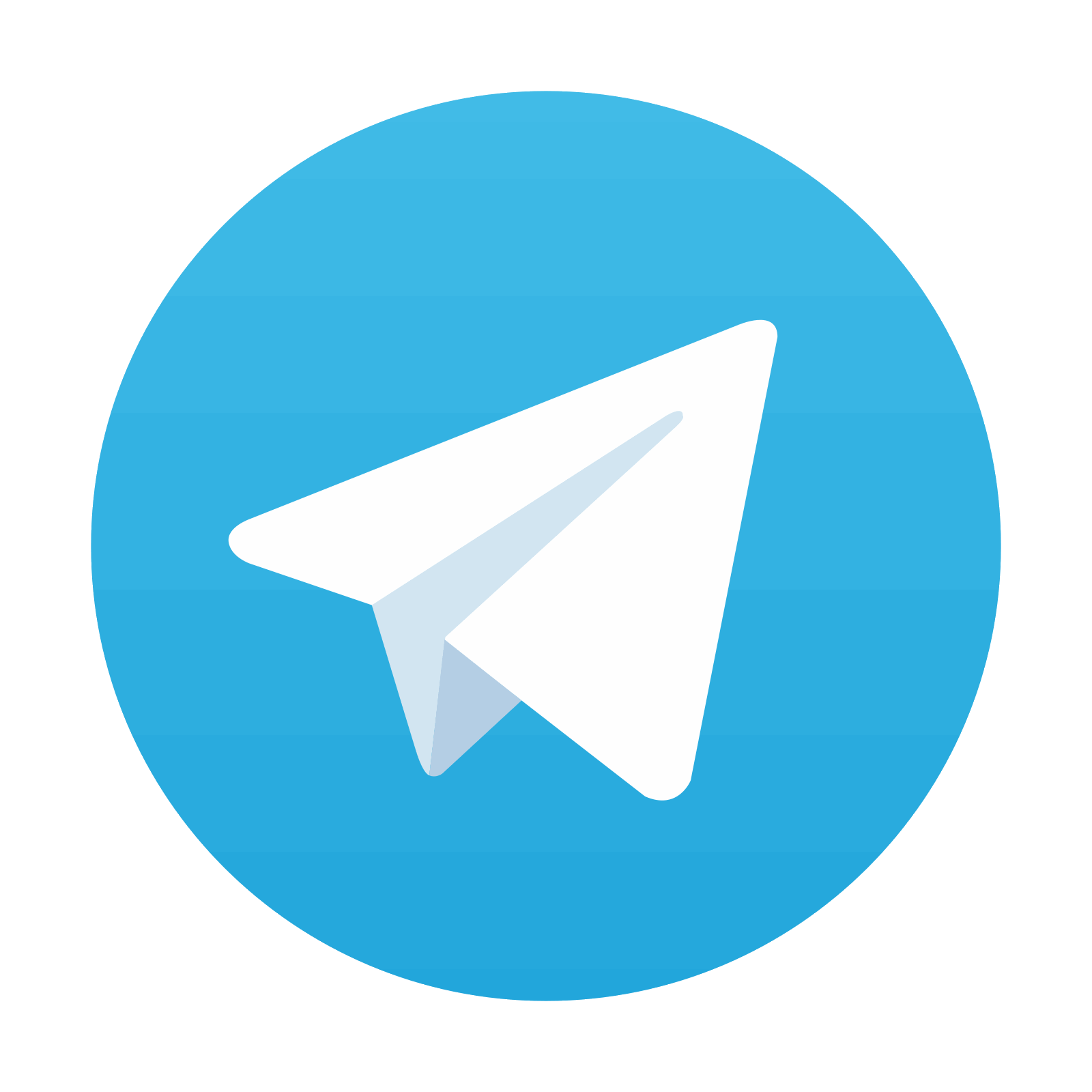
Stay updated, free articles. Join our Telegram channel

Full access? Get Clinical Tree
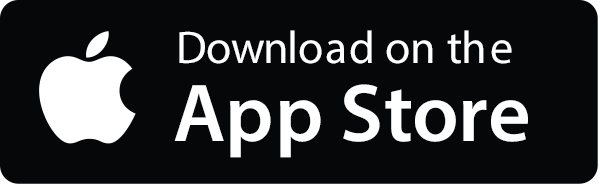

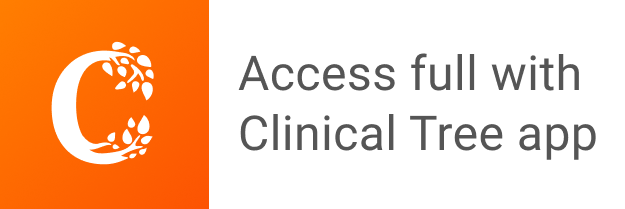