Patients with continuous flow destination therapy (DT) left ventricular assist devices (LVAD) comprise a heterogeneous population. We hypothesized that phenotypic clustering of individuals with DT LVADs by their implantation characteristics will be associated with different long-term risk profiles. We analyzed 5,999 patients with continuous flow DT LVADs in Interagency Registry for Mechanically Assisted Circulatory Support using 18 continuous variable baseline characteristics. We Z-transformed the variables and applied a Gaussian finite mixture model to perform unsupervised clustering resulting in identification of 4 phenogroups. Survival analyses considered the competing risk for cumulative incidence of transplant or the composite end point of death or heart transplant where appropriate. Phenogroup 1 (n = 1,163, 19%) was older (71 years) and primarily white (81%). Phenogroups 2 (n = 648, 11%) and 3 (n = 3,671, 61%) were of intermediate age (70 and 62 years), weight (85 and 87 kg), and ventricular size. Phenogroup 4 (n = 517, 9%) was younger (40 years), heavier (108 kg), and more racially diverse. The cumulative incidence of death, heart transplant, bleeding, LVAD malfunction, and LVAD thrombosis differed among phenogroups. The highest incidence of death and the lowest rate of heart transplant was seen in phenogroup 1 (p <0.001). For adverse outcomes, phenogroup 4 had the lowest incidence of bleeding, whereas LVAD device thrombosis and malfunction were lowest in phenogroup 1 (p <0.001 for all). Finally, the incidence of stroke, infection, and renal dysfunction were not statistically different. In conclusion, the present unsupervised machine learning analysis identified 4 phenogroups with different rates of adverse outcomes and these findings underscore the influence of phenotypic heterogeneity on post-LVAD implantation outcomes.
The use of left ventricular assist devices (LVADs) in patients with end-stage heart failure is well-established and most devices are implanted with a bridge to transplant (BTT) or destination therapy (DT) strategy. DT LVADs currently comprise the most common indication for LVAD implantation and these individuals are phenotypically diverse, likely linked to a spectrum of reasons for heart transplant ineligibility (e.g., age, weight, and so on). As such, patients with DT LVADs are an inherently different population compared with patients implanted with a BTT strategy. Despite efforts to develop prediction models for LVAD postimplant complications, performance characteristics of these models remain suboptimal. Therefore, novel methods for phenotypic classification may be useful for identifying these unique risk associations and identifying personalized management strategies for patients with DT LVADs. We sought to determine phenotypic clusters of patients with continuous flow DT LVADs through an unsupervised machine learning algorithm and establish whether these clusters associate with clinical outcomes.
Data were obtained from 22,142 patients enrolled into the Interagency Registry for Mechanically Assisted Circulatory Support (INTERMACS) registry from the National Institutes of Heart, Lung, and Blood Institute’s Biologic Specimen and Data Repository Information Coordinating Center through an approved proposal and a local institutional review board approval. All patient data extraction and protocols were approved by the institutional review board at each respective site and the study was in compliance with the Declaration of Helsinki. The present version of the INTERMACS registry includes all adults (≥18 years) who received an approved mechanical circulatory support device between January 1, 2008 and December 31, 2017 at 170 active centers throughout the United States and Canada. Of the initial 22,142 patients, we excluded 2,766 participants with a previous LVAD, use of a pulsatile device, or use of temporary device; 124 patients were excluded due to the presence of a right ventricular assist device; and 11,007 patients were excluded due to a device strategy other than DT ( Figure 1 ).

We a priori included 25 continuous patient characteristics present at device implantation that had the potential to influence outcomes based on clinical knowledge. These included demographic, echocardiographic, hemodynamic, and laboratory data ( Table 1 ). Categoric variables were not included due to the analytical technique. We excluded pulmonary artery systolic and diastolic pressure, mean right atrial pressure, pulmonary artery wedge pressure and right ventricular ejection fraction due to missingness >10%. Due to the potential association between left ventricle chamber size and LVAD-related outcomes, left ventricular end diastolic diameter (LVEDD) was the only variable with >10% missingness that was retained for clustering. The distribution of LVEDD was approximately normal, and linear regression imputation was performed for the 25% of patients with missing values. Because correlated variables can affect clustering performance, we calculated the spearman’s correlation coefficient for each pair of characteristics and excluded covariates with | r | >0.6. Among these covariate pairs, blood urea nitrogen and creatinine and aspartate aminotransferase and alanine aminotransferase were highly correlated. Thus, we excluded creatinine and aspartate aminotransferase resulting in 18 remaining covariates. Our primary outcome was the cumulative incidence of death with heart transplantation as a competing risk after DT LVAD implantation. Secondary outcomes included the cumulative incidence of stroke, LVAD malfunction, LVAD thrombus, bleeding, infection, and worsening renal function as defined by the INTERMACS registry.
Patient Characteristics at Left Ventricular Assist Device Implantation | |
---|---|
Age | Diuretic dose |
New York Heart Association class | albumin |
Weight | alanine aminotransferase |
Heart rate | aspartate aminotransferase |
Systolic blood pressure | blood urea nitrogen |
Diastolic blood pressure | creatinine |
Pulmonary artery systolic pressure | hemoglobin |
Pulmonary artery diastolic pressure | platelets |
Mean right atrial pressure | serum potassium |
Pulmonary capillary wedge pressure | serum sodium |
Left ventricular end-diastolic diameter | total bilirubin |
Left ventricular ejection fraction | white blood cell count |
From the 8,245 patients with continuous flow LVADs implanted with a DT strategy, we excluded 2,246 patients with any missing values for any of the 18 covariates (other than LVEDD) resulting in a final dataset with 5,999 patients. We performed Z-transformation on the 18 continuous variables followed by principal component analysis on this Z-transformed matrix and the first 2 components were selected as the input for the downstream clustering analysis. We applied a gaussian finite mixture model to perform unsupervised clustering and the optimal number of clusters was assessed by minimization of the Bayesian Information Criterion. Baseline characteristics across phenogroups with continuous variables were presented as median and interquartile range and categorical baseline variables were presented as number and percentage and compared by the Kruskal-Wallis and chi-square tests, respectively. A radar plot comparing the 18 continuous baseline characteristics was created by calculating Z scores for phenogroups based on the group continuous variable mean. Survival analyses for long-term events considered competing risk for cumulative incidence of heart transplantation or the composite end point of death or heart transplantation when appropriate. Cumulative incidence curves for each time-to-event outcome were plotted. Two-sided p values <0.05 were considered statistically significant. Statistical analyses were completed by using R 3.6.2.
Among 5,999 patients with continuous flow LVADs implanted with a destination strategy, we identified 4 phenogroups. Baseline characteristics stratified by phenogroups obtained at the time of LVAD implantation are shown in Table 2 . Phenogroup 1 (n = 1,163, 19%) was primarily white (81%) with the oldest median age (71 years). These participants also had the lowest weight, highest prevalence of frailty (9%), and the least dilated ventricles. Phenogroup 2 (n = 648, 11%) comprised of patients with intermediate age and weight, and had the highest prevalence of an implantable cardioverter-defibrillator and/or cardiac resynchronization therapy. Phenogroup 3 (n = 3,671, 61%) encompassed patients of intermediate age, weight, and ventricular size with intermediate prevalence of smoking, frailty, and peripheral vascular disease. Finally, phenogroup 4 (n = 517, 9%) was the youngest (median age 40 years), heaviest, and the most racially diverse (white 46%). There were no statistically significant differences among phenogroups for sex or hemoglobin A1c. However, there were small but statistically significant differences in New York Heart Association class and creatinine. A comparison of the 18 baseline continuous characteristics for the clustering analysis by phenogroup are shown in a radar plot in Figure 2 and by heatmap in Figure 3 .
Phenogroup | |||||
---|---|---|---|---|---|
Variable | 1(n = 1,163) | 2(n = 648) | 3(n = 3,671) | 4(n = 517) | p Value |
Age (years) | 71 (67–75) | 70 (65–74) | 62 (54–69) | 40 (31–49) | <0.01 |
Weight (kg) | 76 (66–87) | 85 (75–98) | 87 (74–103) | 108 (84–130) | <0.01 |
Men | 931 (80%) | 538 (83%) | 2,914 (80%) | 420 (81%) | 0.19 |
White | 939 (81%) | 515 (80%) | 2,455 (67%) | 236 (46%) | <0.01 |
Centrifugal LVAD | 47 (4.0%) | 17 (2.6%) | 125 (3.4%) | 28 (5.4%) | 0.05 |
NYHA | 4 (4–4) | 4 (3–4) | 4 (4–4) | 4 (4–4) | <0.01 |
LVEDD (cm) | 6.3 (5.8–6.9) | 6.5 (6.0–7.1) | 6.9 (6.2–7.6) | 7.5 (6.9–8.2) | <0.01 |
Frailty | 102 (9%) | 43 (7%) | 215 (6%) | 16 (3%) | <0.01 |
Inotrope use | 439 (38%) | 229 (36%) | 1,402 (39%) | 208 (41%) | 0.27 |
CRT | 299 (34%) | 209 (39%) | 932 (32%) | 98 (22%) | <0.01 |
ICD | 991 (86%) | 576 (89%) | 3,068 (84%) | 387 (75%) | <0.01 |
Cerebrovascular disease | 14 (1.5%) | 1 (0.2%) | 15 (0.5%) | 4 (0.9%) | 0.01 |
COPD | 70 (6%) | 30 (5%) | 219 (6%) | 18 (4%) | 0.08 |
Current smoker | 40 (4%) | 32 (5%) | 246 (7%) | 56 (11%) | <0.01 |
Pulmonary hypertension | 141 (13%) | 80 (13%) | 587 (16%) | 57 (11%) | <0.01 |
PVD | 63 (5.5%) | 32 (5%) | 145 (4%) | 8 (1.5%) | 0<0.01 |
Severe diabetes | 64 (6%) | 42 (7%) | 237 (7%) | 27 (5%) | 0.52 |
Albumin (g/100 ml) | 3.4 (3.0–3.7) | 3.8 (3.4–4.0) | 3.3 (3.0–3.7) | 3.3 (2.9–3.7) | <0.01 |
BNP (pg/ml) | 837 (426–1,702) | 536 (250–1,150) | 842 (417–1,614) | 884 (449–1,601) | <0.01 |
BUN (mg/100 ml) | 29 (21–41) | 25 (19–33) | 26 (18–38) | 25 (17–39) | <0.01 |
Creatinine (mg/100 ml) | 1.4 (1.1–1.7) | 1.3 (1.1–1.6) | 1.3 (1.0–1.7) | 1.3 (1.0–1.7) | 0.01 |
Hemoglobin A1c (%) | 6.2 (5.8–6.8) | 6.0 (5.5–7.2) | 6.3 (5.8–7.1) | 6.1 (5.6–7.0) | 0.19 |
Hemoglobin (g/100 ml) | 10.9 (9.8–12.1) | 12.4 (11.3–13.6) | 11.1 (9.7–12.6) | 11.2 (9.7–12.5) | <0.01 |
Sodium (mmol/L) | 137 (135–139) | 139 (137–141) | 135 (132–137) | 132 (128–135) | <0.01 |
Total bilirubin (mg/100 ml) | 0.9 (0.6–1.3) | 0.7 (0.5–1.0) | 1.0 (0.7–1.6) | 1.5 (1.0–2.6) | <0.01 |
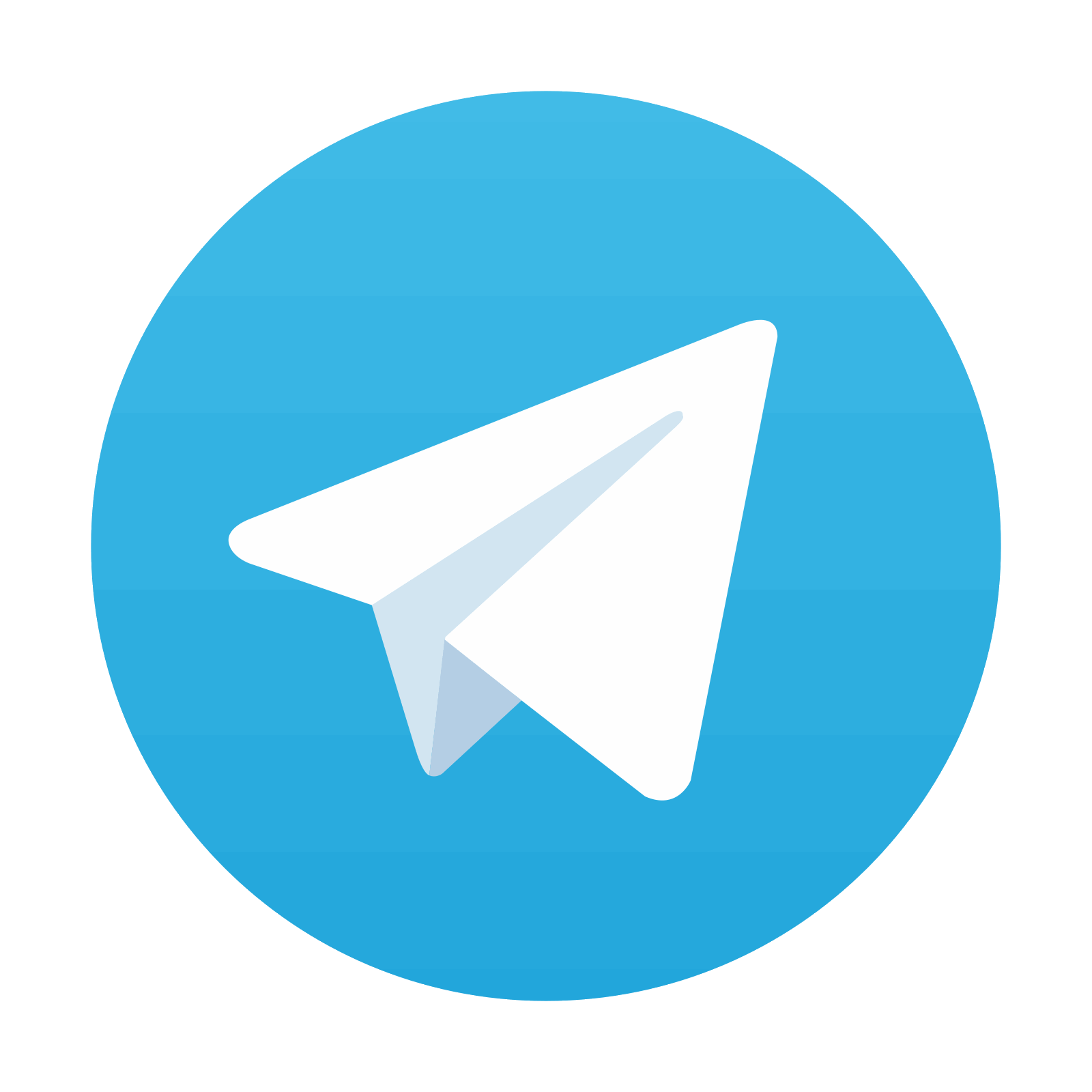
Stay updated, free articles. Join our Telegram channel

Full access? Get Clinical Tree
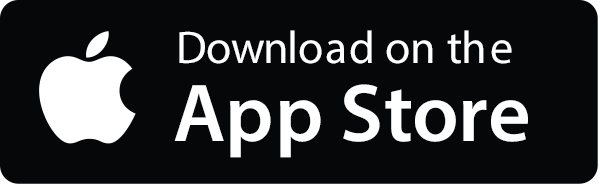
