Abstract
Background
Congenital heart disease (CHD) is the main cause of perinatal morbidity and mortality. Nuchal Translucency (NT), Ductus Venosus (DV), and Tricuspid Regurgitation (TR) have shown potential in CHD detection.
Aim of review
We evaluated the pooled diagnostic test accuracy of these markers during the first-trimester screening.
Key scientific concepts of review
PubMed, Scopus, Web of Science, and Embase were searched. A bivariate random effects model created Summary Receiver Operating Characteristic (SROC) curves and the pooled sensitivities and specificities. Forty-two studies were included. For major CHDs, the pooled sensitivities and specificities were 43.1 % (95 % CI: 35.0 %–51.6 %) and 95.5 % (95 % CI: 93.5 %–96.9 %) for A/R DV a wave, 57.8 % (95 % CI: 43.3 %–71.0 %) and 88.8 % (95 % CI: 77.7 %–94.7 %) for abnormal DV-PIV, 37.0 % (95 % CI: 26.6 %–48.6 %) and 97.7 % (95 % CI: 94.6 %–99.1 %) for TR, 41.4 % (95 % CI: 23.2 %–62.2 %) and 93.7 % (95 % CI: 92.7 %–94.6 %) for NT > 95th percentile, and 26.6 % (95 % CI: 11.0 %–51.7 %) and 98.3 % (95 % CI: 97.5 %–98.9 %) for NT > 99th percentile. For the combined models in detecting major CHDs, the highest specificity of 97.8 % (95 % CI: 93.9 %–99.2 %) belonged to NT > 95th percentile and A/R DV a wave. The most sensitive tests were the combination of NT > 95th percentile or A/R DV a wave or TR 61.4 % (95 % CI: 49.7 %–71.9 %). Combining increased NT with the presence of A/R a-wave can help diagnose CHD, while normal NT, A/R DV a wave, and TR indicate lower CHD risk.
Highlights
- •
This meta-analysis assessed first-trimester ultrasound markers for heart defect screening.
- •
Most sensitive test combined NT > 95th percentile, DV a wave, or tricuspid regurgitation.
- •
NT > 95th percentile alone showed the highest specificity for detecting heart defects.
- •
Combining ultrasonography markers improves early detection of fetal heart defects.
1
Introduction
Congenital Heart Defects (CHD) is the leading type of congenital malformations [ ]. Prenatal diagnosis of CHD can significantly decrease perinatal mortality and morbidity [ ]. Early diagnosis has numerous advantages [ ]. Moreover, since there is a correlation between the incidence of CHD and chromosomal and other malformations, the detection of CHD in the first trimester gives physicians much more information about the risk of 2nd and 3rd-trimester gestational risks [ ]. In addition, the complexity of some types of CHD increases the risk of termination of pregnancy. By detecting CHDs in the first trimester, an earlier elective abortion can reduce the psychological and physical burden of pregnancy termination [ ].
Nuchal Translucency (NT) measures the temporary fluid collection in the back of the fetus’s neck. The risk of CHD for those with an NT > 99th percentile is six times higher than for those with normal NT [ ]. NT can also decrease the false-positive rate of other ultrasonographic features and improve their performance in the detection of CHD [ ].
Another ultrasonographic marker is the Doppler evaluation of blood flow across the ductus venosus (DV), a representative of the fetal central venous system [ ]. Any abnormality occurring on the right side of the heart affects the blood flow through DV [ ]. DV is assessed qualitatively as the absence or reversal of DV a-wave (A/R a wave) or quantitatively as DV Pulsatility Index for Veins (DV-PIV) [ ].
Tricuspid regurgitation (TR) is a marker that can detect CHD. Although the link between abnormal TR and CHD prevalence has been established in high-risk pregnancies like positive NT, no significant correlation has been found in the normal pregnant population. Therefore, performing this screening test for all pregnant women is not considered to be cost-effective [ ]. However, an individualized decision-making pattern should be designed based on the various parameters to determine which characteristics make the woman eligible for first-trimester TR measurement.
In this systematic review and meta-analysis, we assessed the diagnostic performance of these three ultrasonographic markers, independently or jointly, for detecting CHD during the first trimester.
2
Methods
The investigation was carried out per the preferred reporting guidelines for systematic reviews and meta-analyses (PRISMA) 2020 declaration [ ] (Supplementary file, PRISMA checklist). The research protocol was registered in PROSPERO (42023401007).
2.1
Search strategy and eligibility criteria
We systematically searched PubMed, Scopus, Web of Science, and Embase databases until March 8, 2024. The search used the following keywords: prenatal diagnosis, prenatal ultrasonography, first trimester, nuchal translucency, tricuspid regurgitation, ductus venosus, and congenital heart defect. Language and research type limitations were not imposed. Furthermore, we searched the references for the papers included and Google Scholar. The detailed search query is provided in Supplementary material Table S1.
2.2
Study selection
PE and NE. DJG evaluated half of the studies on Rayyan [ ], based on the titles and the abstracts, independently rechecked by PE and NE. DJG resolved disagreements. Studies were included if they reported data on the diagnostic performance of ultrasonography screening of DV A/R a wave, DV-PIV, TR, NT, or their combinations. We excluded all reviews, systematic reviews, conference papers and abstracts, editorials, case reports and series, notes, letters, books, and comments. Also, non-observational studies, pregnancies after the 14th week of gestation, or non-English papers were excluded.
GMT and MT conducted separate evaluations of the full texts, and PV resolved disagreements. If the study did not report true positive (TP), false positive (FP), true negative (TN), and false negative (FN), or sensitivity and specificity, it was not considered for inclusion.
2.3
Data collection
Relevant data from eligible studies, such as characteristics of CHD patients, maternal and gestational age, NT and DV-PIV cutoff, sensitivity, specificity, TP, TN, FP, FN, and Area Under the Curve (AUC), was extracted. PS and NE extracted data and DJG re-checked it.
2.4
Risk of bias assessment
PE and PV used the quality assessment of diagnostic accuracy studies-2 (QUADAS-2) appraisal tool to assess the potential for bias [ ]. The visualization was facilitated by the template for graphical display released with the questionnaire [ ]. QUADAS-2 encompasses four domains of patient selection: index test, reference standard, and flow and time. All are domains of bias, and the first three are for applicability concerns, too. When all were rated as “low,” the study was regarded as having a “low risk of bias.” Conversely, if one or more categories were deemed to have an “unclear risk” or a “high risk of bias,” the study was considered biased.
2.5
Data synthesis
The screening tests analyzed included DV A/R a wave, DV-PIV, TR, increased NT, and their combinations. The primary focus was on predicting major CHDs, though some studies also considered any CHD (major or minor). As the nature of evaluated CHDs can significantly impact diagnostic outcomes, analyses for major and any CHDs were conducted separately. We complied with the definition of major CHDs that each study provided and used their data accordingly.
For each screening criterion, except for increased NT, a diagnostic test accuracy (DTA) meta-analysis was conducted using a bivariate random effects model by Reitsma [ ].
This model jointly estimates sensitivity and specificity while accounting for study-level variability and the correlation between these metrics. It is widely used in diagnostic meta-analyses because it retains the natural trade-off between sensitivity and specificity. Subgroup analyses were conducted to compare diagnostic accuracy across different screening tools. If a specific combination of screening criteria was evaluated in at least three studies, we conducted a separate meta-analysis for that combination.
Summary Receiver Operating Characteristic (SROC) curves were generated from the bivariate model to visualize the relationship between sensitivity and specificity across studies. We also used a random effects univariate Diagnostic Odds Ratio (DOR) model to summarize the diagnostic performance of each test as a single index.
Heterogeneity among study results was evaluated using the I 2 metric [ ]. An I 2 confidence interval (CI) above 50 % indicated significant heterogeneity. When heterogeneity was observed, we explored potential sources through sensitivity analyses and subgroup assessments, particularly focusing on the influence of including high-risk populations.
Because NT thresholds varied widely across studies, we employed a multiple cutoffs DTA meta-analysis approach for NT, using the method proposed by Steinhauser et al. [ ]. This approach models the cumulative distribution functions of the biomarker (NT) among both diseased and non-diseased individuals. Specifically, it assumes that NT follows a normal distribution in each group. Reported sensitivities and specificities at different thresholds from individual studies are treated as empirical estimates of the cumulative distribution function. These values are transformed using standard statistical functions (logit) to make them suitable for linear modeling. The transformed data were then analyzed using linear mixed effects models, which incorporate random variation across studies to account for differences in study populations and methodologies. The model allows the estimation of pooled sensitivity and specificity for any NT threshold, generates an SROC curve, and identifies an optimal threshold by maximizing the Youden index [ ].
To evaluate the clinical applicability of the diagnostic tests, we constructed Fagan’s nomograms and likelihood ratio scattergrams. These visual tools help estimate how the probability of CHD changes with a positive or negative test result. Positive and negative likelihood ratios (pLR and nLR) and their 95 % confidence intervals were calculated using Zwinderman’s method, which is specifically suited to the bivariate structure of the Reitsma model [ ].
For tests involving multiple NT thresholds, pLR and nLR were directly calculated from the pooled sensitivity and specificity. However, because this method does not allow for the calculation of confidence intervals for the likelihood ratios, we did not generate likelihood ratio scattergrams for these cases.
The post-test probability of CHD was derived using Fagan’s nomogram. We assumed a pre-test probability of 1 % for any CHD and 0.5 % for major CHDs, reflecting estimates from previous literature in general population settings (as reported by Liu et al. [ ]). This means that before performing any screening test, the assumed probability of CHD was 1 in 100 births (or 1 in 200 for major CHDs). Using these baseline values, we aimed to calculate the post-test probability of CHD in the event of either a positive or negative test result, allowing interpretation of how test outcomes alter the likelihood of CHD presence.
Publication bias was assessed using a modified version of Egger’s regression test specific to the DTA meta-analysis [ ]. This involved analyzing funnel plot asymmetry through 2000 sample bootstrapping.
All analyses were conducted using R (version 4.2.1), employing packages “Mada” [ ], “MVPBT” [ ], “diagmeta” [ ], “Metafor” [ ], and “meta” [ ]. Fagan’s nomograms and likelihood ratio scattergrams were inspired by the STATA module Midas’s “fagan” and “lrmat” functions, with plotting Fagan’s nomograms being facilitated by the ‘nomogrammer’ GitHub repository ( https://github.com/achekroud/nomogrammer ).
3
Results
3.1
Study selection
The initial search obtained 3088 records. After removing duplicate entries and irrelevant studies, we selected 42 studies that met the eligibility criteria ( Fig. 1 ). Table S2 of the Supplementary file represents the reasons for excluding 130 articles during the full-text review.

3.2
Study characteristics
The analysis included 745,580 participants from Asia, Europe, and the Americas. Of whom, 2755 had congenital heart disease. Most studies focused on fetuses with normal karyotypes, excluding any aneuploidies. However, 11 studies did not exclude abnormal karyotypes [ ]. Most studies used a retrospective or prospective cohort design, with two studies using a case-control design [ , ]. The maternal ages ranged from 14 to 53 years. All participants underwent sonography within a gestational age range of 9 to 14 weeks. The primary ground truth was clinical follow-up and echocardiography ( Table 1 ).
Author | Country | R or P | Sample size | Only normal karyotype? | Did the study exclude high-risk pregnancies? | Did the study only include singleton pregnancies? | Number of CHD | Only major? | Maternal age (years) | Maternal age range (years) | Gestational age range (weeks) | Reference standard |
---|---|---|---|---|---|---|---|---|---|---|---|---|
Abu-Rustum 2010, [ ] | Lebanon | R | 1370 | No | No | No | 8 | Yes | 27 (median) | 15-43 | 11-13 | CFU ± Echo |
Alanen 2017, [ ] | Finland | R | S841 | No | No | No | 79 | Yes | severe CHD: 31.2 (median), 29.7 (mean) | – | 9-13 | CFU/autopsy |
Baran 2020, [ ] | Turkey | R | 556 | Yes | Unclear | Yes | 4 | Yes | 30.64 (mean) | 18-47 | 11-14 | CFU ± Echo |
Becker 2006, [ ] | Germany | P | 3094 | No | No | No | 38 | Yes | 35 (median) | 15-46 | 11-13 | CFU |
Bilardo 1998, [ ] | Netherlands | P | 1631 | Yes | No | Yes | 4 | Yes | 37.1 (mean) | 20-46 | – | CFU |
Borelli 2017, [ ] | USA | R | 118,194 | Yes | Yes | Yes | 284 | Yes | Most 18-34 | – | CFU | |
Borrell 2013, [ ] | Spain | P | 12,402 | Yes | Yes | Yes | 37 | Yes | – | 11-14 | CFU ± Echo | |
Bruns 2006, [ ] | Brazil | R | 3664 | Yes | Yes | Yes | 20 | No | 32 (mean) | 14-53 | 11-13 | CFU ± Echo |
ÇaliŞkan 2009, [ ] | Turkey | P | 956 | No | Yes | Yes | 7 | No | 34 (median) | 18-43 | 11.2-14.1 | CFU ± Echo |
Chelemen 2011, [ ] | UK | R | 40,990 | Yes | Yes | Yes | 85 | Yes | 31 (median) | 14-51 | 11-13 | CFU ± Echo |
Faiolia 2005, [ ] | UK | R | 458 | Yes | Yes | Yes | 32 | No | – | 11-13 | CFU ± Echo | |
Favre 2003, [ ] | France | P | 998 | Yes | Yes | Yes | 10 | Yes | 31.9 (mean) | 16-46 | 11-14 | CFU ± Echo/autopsy |
Hafner 2003, [ ] | Austria | R | 12,978 | Yes | Yes | No | 27 | Yes | 28 (mean) | 14-48 | – | CFU ± Echo |
Hyett 1999, [ ] | UK | R | 29,154 | Yes | Yes | Yes | 50 | Yes | 34 (mean) | 15-48 | 10-14 | CFU ± Echo |
Ji 2021, [ ] | China | P | 3356 | No | No | No | 66 | No | 29.2 (mean) | – | 11-14 | CFU ± Echo/autopsy |
Josefsson 1998, [ ] | Sweden | P | 1460 | Yes | No | No | 13 | Yes | 28 (mean) | – | CFU | |
Maiz 2008, [ ] | UK | P | 10,490 | No | No | Yes | 20 | Yes | 32 (median) | 16-49 | 11-13 | CFU ± Echo |
Mavrides 2001, [ ] | UK | P | 7339 | Yes | Yes | No | 26 | Yes | 27 (mean) | 15-44 | 10-14 | CFU ± Echo |
Michailidis 2001, [ ] | UK | R | 6606 | Yes | Yes | No | 11 | Yes | 30.6 (mean) | 13-47 | 12-13 | CFU ± Echo |
Minnella 2020, [ ] | UK | R | 93,209 | Yes | Yes | Yes | 211 | Yes | 31 (median) | – | 11-13 | CFU ± Echo |
Muller 2007, [ ] | Netherlands | P | 4144 | Yes | Yes | No | 24 | No | 31 (median) | 16-45 | 10-14 | CFU ± Echo |
Orlandi 2014, [ ] | Italy | P | 4030 | No | Yes | Yes | 20 | Yes | 33 (mean) | 16-43 | 11-14 | CFU ± Echo |
Orlic 2019, [ ] | Serbia | P | 20,010 | Yes | Yes | Yes | 92 | Yes | 31.2 (mean) | – | 11-13 | CFU ± Echo/autopsy |
Orvos 2002, [ ] | Hungary | R | 4309 | Yes | Yes | No | 39 | Yes | – | 10-13 | CFU ± Echo/autopsy | |
Pawlowski 2015, [ ] | USA | R | 76,089 | Yes | Yes | Yes | 190 | Yes | 18-34 | 11-14 | Hospital records | |
Pereira 2011, [ ] | UK | R | 40,990 | Yes | Yes | Yes | 85 | Yes | 31 (median) | 14-51 | 11-13 | CFU ± Echo |
Shamshirsaz 2014, [ ] | USA | R | 8541 | Yes | Yes | Yes | 33 | No | 33.3 (mean) | – | 11-13 | CFU ± Echo |
Simpson 2007, [ ] | USA | P | 34,266 | Yes | Yes | Yes | 224 | No | 30.2 (mean) | – | 10-13 | CFU ± Echo |
Singh 2005, [ ] | USA | P | 8167 | Yes | Yes | Yes | 21 | Yes | 34.5 (mean) | – | 10-13 | CFU ± Echo |
Snanaes 2010, [ ] | France | R | 12,910 | Yes | Yes | No | 44 | Yes | 32.3 (mean) | 15-51 | 10-14 | CFU ± Echo/autopsy |
Syngelaki 2011, [ ] | UK | P | 40,949 | Yes | Yes | Yes | 106 | No | 31 (median) | 14-51 | 11-13 | CFU ± Echo |
Syngelaki 2019, [ ] | UK | R | 100,997 | Yes | Yes | Yes | 389 | 31 (median) | – | 11-13 | CFU | |
Timmerman 2010, [ ] | Netherlands | R | 792 | Yes | Yes | Yes | 26 | Yes | 35 (mean) | 19-46 | 11-13 | CFU |
Toyama 2004, [ ] | Brazil | P | 1060 | Yes | No | Yes | 7 | Yes | 32.1 (mean) | 14-47 | 11-14 | CFU ± Echo/autopsy |
Traisrisilp 2021, [ ] | Thailand | P | 7126 | No | No | Yes | 76 | Yes | 29.8 (mean) | – | 11-13 | CFU ± Echo |
Volpe 2011, [ ] | Italy | P | 4425 | Yes | Yes | No | 18 | Yes | 29 (median) | 17-44 | 11-14 | CFU ± Echo/autopsy |
Wagner 2019, [ ] | Germany | R | 528 | Yes | Yes | Yes | 48 | Yes | CHD: 33 HC: 33.8 (median) | CHD: 30 HC: 30.7- CHD: 36 HC: 36.4 | Both: 12.4-CHD: 13.6 | CFU/autopsy |
Westin 2006, [ ] | Sweden | P | 16,383 | Yes | Yes | Yes | 127 | No | – | 12-14 | CFU ± Echo/autopsy | |
Wiechec 2015, [ ] | Poland | P | 1075 | No | No | Yes | 37 | No | 30.5 (mean) | – | 11-13 | Medical records autopsy |
Wiechec 2016, [ ] | Poland | R | 5673 | Yes | Yes | Yes | 28 | No | – | 11-13 | CFU/autopsy | |
Wiechec and Knafel 2015, [ ] | Poland | P | 1084 | No | No | No | 35 | No | 32.3 (median) | 27-40 | 11-13 | CFU ± Echo/autopsy |
Zheng 2019, [ ] | China | P | 1568 | No | No | Yes | 54 | Yes | 30.8 (median) | 20-47 | – | CFU ± Echo/autopsy |
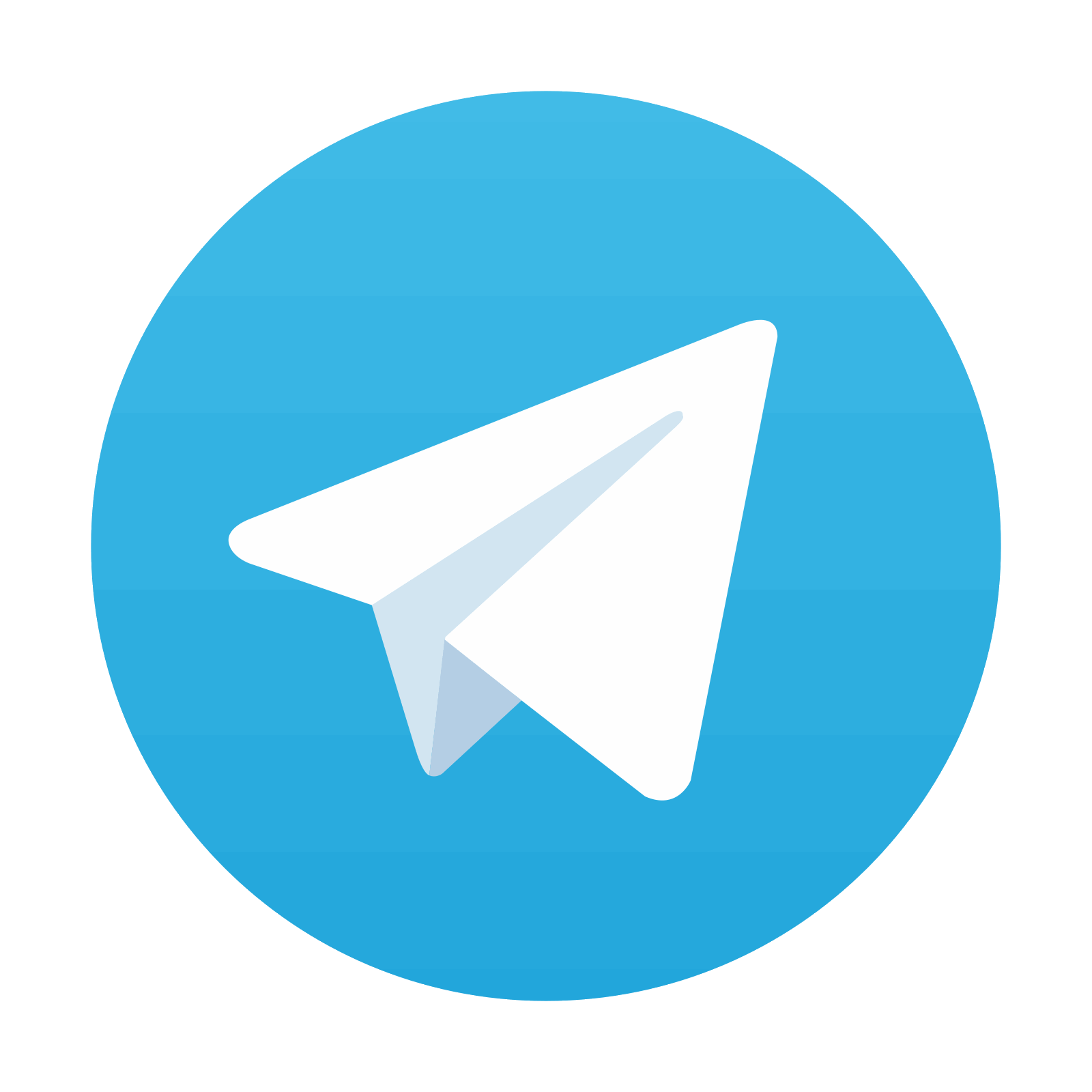
Stay updated, free articles. Join our Telegram channel

Full access? Get Clinical Tree
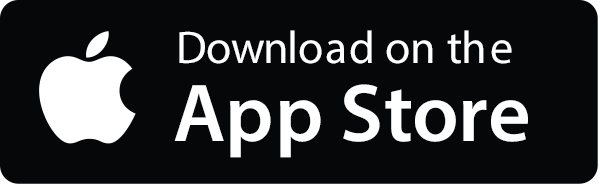

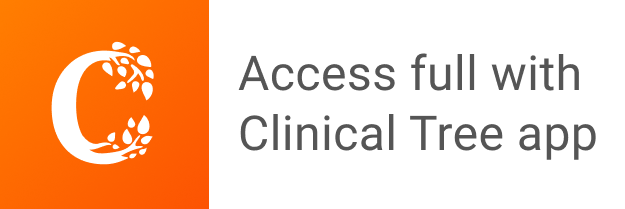