Introduction
In recent years the volume and variety of pediatric cardiovascular data captured across various sources have continued to expand. These datasets are increasingly being integrated and used for a variety of research and quality improvement purposes. Regardless of the data source, there are several important points to consider in analyzing data or utilizing the literature to guide evidence-based care in this population. In this chapter, we highlight key aspects related to pediatric cardiovascular data, analysis, and critical appraisal of the literature.
Pediatric Cardiovascular Data
Current Data Environment
The present decade has ushered in an era of “big data” during which the volume, velocity, and variety of data captured across numerous sources and many different fields have increased exponentially. Along with this, new techniques and capabilities have been developed to better collect, manage, analyze, and provide feedback regarding these data, with the goal of optimizing performance and outcomes across numerous industries. For example, the automotive industry captures data generated by sensors on electric cars to better understand people’s driving habits. These data are merged and analyzed with information on the frequency and location of battery charging to aid in better design of the next generation of vehicles and charging infrastructure. In the hotel industry, certain chains merge and analyze weather and airline flight cancellation data, along with information on the geographic location of their hotels. These data are used to target mobile ads to stranded passengers to promote easy booking of nearby hotels.
Health Care Data
Historically in health care and in the hierarchy of medical research, the value of databases, registries, and other data sources in the cycle of scientific discovery and in patient care has not always been recognized. “Mining datasets” and “database research” have been characterized as lesser pursuits compared with basic science research or clinical trials.
However, several recent developments have begun to change the way we view data and their potential value. First, similar to other industries, the volume and granularity of health care data have increased exponentially, including data captured in the electronic health record, clinical registries, research datasets, at the bedside, and from mobile monitors as well as other sources. It has become increasingly recognized that the analysis and integration of these datasets may expand the range of questions that can be answered. For example, early results suggest that the integration of continuous data streams generated by various bedside monitoring systems with data on clinical outcomes may enable better prediction and treatment of adverse events in intensive care settings.
Second, along with this trend toward the increasing availability of data, there has been a simultaneous trend toward declining federal funding to support biomedical research. This has led to further interest in improving our understanding of how to leverage available data sources to power research more efficiently. For example, the use of existing registries or the electronic health record as platforms to support clinical trials has been proposed with the goal of reducing the time and costs associated with data collection.
Finally, the current emphasis in the United States on both improving the quality of health care and lowering costs has necessitated the analysis and integration of both quality and resource utilization data across numerous sources in order to elucidate the landscape of care delivery and outcomes, to investigate relationships between quality and cost, and to develop and investigate strategies for improvement. These and other recent trends have led to a greater recognition in the health care field of the value of leveraging the increasing volume of available data, and numerous recent initiatives have been launched with the goal of further integrating information across sources to conduct novel research and improve care. These sources include the National Institutes of Health Big Data to Knowledge and Precision Medicine Initiatives, among others.
Pediatric Cardiovascular Data
In 2015, the National Heart, Lung, and Blood Institute of the US National Institutes of Health convened a working group to characterize the current data environment in the field and to offer recommendations for further development and integration. The working group described several strengths and weaknesses related to the existing data environment as detailed in the following sections.
Data Sources
Numerous clinical and quality improvement registries, administrative/billing databases, public health databases, research datasets, and other sources now exist in the field and contain detailed information that is used for pediatric cardiovascular research, surveillance, and quality improvement purposes. Comprehensive listings of available data sources, both in the United States and worldwide ( Table 24.1 ), have recently been published. In addition, data are being increasingly captured via a variety of newer techniques and modalities, including the electronic health record, medical monitors and devices, and genetic and biomarker data. Some centers are also now capturing standardized longer-term outcomes data, such as quality-of-life and neurodevelopmental outcomes data.



Infrastructure and Collaboration
Many programs focusing on congenital heart disease across the United States have developed local infrastructure and personnel to support data collection for various registries and other datasets and to support the management and analyses of their local data for administrative, quality improvement, and research purposes. Several centers and research organizations also function as data-coordinating centers, aggregating and analyzing various multicenter datasets in the field.
There is also an environment of collaboration across many programs focusing on congenital heart disease and investigators related to participation in various multicenter research and quality improvement efforts. This has also extended in many cases to collaboration with patient and parent advocacy groups. Examples include the National Pediatric Cardiology Quality Improvement Collaborative, Pediatric Heart Network, Congenital Heart Surgeons Society, Pediatric Cardiac Critical Care Consortium, and many others. Annual meetings of the Multi-societal Database Committee for Pediatric and Congenital Heart Disease have aided in facilitating the sharing of ideas and collaboration across the many different registries and databases.
Standardized Nomenclature
Another important aspect of the current pediatric cardiovascular data landscape has been the major effort over the past two decades to develop a standardized nomenclature system. In the 1990s both the European Association for Cardio-Thoracic Surgery (EACTS) and the Society of Thoracic Surgeons (STS) created databases to assess congenital heart surgery outcomes and established the International Congenital Heart Surgery Nomenclature and Database Project. Subsequently, the International Society for Nomenclature of Pediatric and Congenital Heart Disease was formed; it cross-mapped the nomenclature developed by the surgical societies with that of the Association for European Pediatric Cardiology, creating the International Pediatric and Congenital Cardiac Code (IPCCC, http://www.IPCCC.net ). The IPCCC is now used by multiple databases spanning pediatric cardiovascular disease, and a recent National Heart, Lung, and Blood Institute working group recommended that the IPCCC nomenclature should be used across all datasets in the field when possible.
Current Data Limitations
Although a great deal of progress has been made over the past several years to better capture important pediatric cardiovascular data, many limitations remain. First, there are several limitations related to data collection. Many registries and databases contain duplicate fields, some with nonstandard definitions, leading to redundant data entry, high personnel costs, and duplication of efforts. There is also wide variability in missing data, data errors, mechanisms for data audits and validation, and overall data quality across different datasets. Second, there are limitations related to data integration. Most datasets remain housed in isolated silos, without the ability to easily integrate or share information across datasets. This limits the types of scientific questions that may be answered and adds to high costs and redundancies related to separate data coordinating and analytic centers. Finally, there are limitations related to organizational structure—generally there is a separate governance and organizational structure for each database or registry effort, which adds to the inefficiencies and lack of integration. Some are a relatively small part of larger organizations focused primarily on adult cardiovascular disease, with limited input or leadership from the pediatric cardiac population. This can lead to further challenges in driving change.
Pediatric Cardiovascular Data Sharing and Integration
To address the limitations outlined in the preceding sections, recent work has focused on developing better mechanisms to foster data sharing and integration. These efforts hold the potential to drive efficiencies by minimizing redundancies in data collection, management, and analysis. In turn, this work could save both time and costs. In addition, data integration efforts can support novel investigation not otherwise possible with the use of isolated datasets alone. Data linkages expand the pool of available data for analysis and also capitalize on the strengths and mitigate the weaknesses of different data sources. These data sharing and collaboration activities may take place through several mechanisms and can involve partnerships or data linkage activities on either the “front end” (at or before the time of data collection) or the “back end” (once data have already been entered).
Partnerships Across Databases: Shared Data Fields and Infrastructure
Partnerships between new and/or existing registries and organizations can drive efficiencies in several ways. For example, the Pediatric Acute Care Cardiology Collaborative (PAC3) recently collaborated with the Pediatric Cardiac Critical Care Consortium (PC 4 ) to add data from cardiac step-down units to the intensive care unit data collected by PC 4 . Data will be collected and submitted together, allowing for integrated feedback, analysis, and improvement activities. This approach is more time- and cost-efficient than creating a separate step-down registry, in which many of the fields regarding patient characteristics, operative data, and clinical course prior to transfer would have been duplicated. Similar efforts have integrated anesthesia data with the STS Congenital Heart Surgery Database and electrophysiology data within the American College of Cardiology Improving Pediatric and Adult Congenital Treatments (IMPACT) registry, which collects cardiac catheterization data. These approaches have involved varying organizational structures governing data access and analysis.
A related method involves a more distributed approach with sharing of common data fields and definitions between organizations, information technology solutions allowing single entry of shared data at the local level, and subsequent submission and distribution of both shared and unique data variables to the appropriate data coordinating centers for each organization/registry. An example of this is the shared variables and definitions for certain fields across the STS, PC, and IMPACT registries.
Linking Existing Datasets
Linking existing data that have already been collected can be accomplished through a variety of mechanisms. Linkage of patient records can be accomplished through the use of unique identifiers such as medical record number, social security number, or combinations of “indirect” identifiers (such as date of birth, date of admission or discharge, sex, and center where hospitalized) when unique identifiers are not available.
These linked datasets have been used to conduct a number of analyses that would not have been possible within individual datasets alone—several examples are highlighted below:
- ■
Academic outcomes: Clinical data from a state birth defects registry have been linked with state education records to understand academic outcomes in children with congenital heart defects.
- ■
Comparative effectiveness and cost analyses: Clinical data from the STS Congenital Heart Surgery Database have been linked with resource utilization data from the Children’s Hospital Association to perform comparative effectiveness and cost-quality analyses. This linked dataset now spans more than 60,000 records and more than 30 children’s hospitals. Similar methods have also been used to link clinical trial data from the Pediatric Heart Network with administrative datasets to clarify the impact of therapies on not only clinical outcomes but also costs of care.
- ■
Long-term survival and other outcomes: Clinical information from the Pediatric Cardiac Care Consortium (PCCC) registry has been linked with the National Death Index and United Network for Organ Sharing dataset in order to elucidate longer-term outcomes (mortality and transplant status) in patients with congenital heart disease undergoing surgical or catheter-based intervention.
- ■
Care models: Center-level clinical data from the STS Congenital Heart Surgery Database have been linked with various survey data to clarify the association of clinical outcomes with certain hospital care models and nursing variables.
Data Modules
Methods have also been developed to create data modules enabling efficient collection of supplemental data points to an existing registry or database. The modules can be quickly created and deployed to allow timely collection of additional data needed to answer research questions that may arise. For example, this methodology has been recently used by PC 4 to study the relationship between Vasoactive-Inotropic Score and outcome after infant cardiac surgery. A module allowing for capture of additional data related to inotrope use was created, deployed, and linked to the main registry. This facilitated efficient data collection with 391 infants prospectively enrolled across four centers in just 5 months.
Trial Within a Registry
It has become increasingly recognized that many variables of interest for prospective investigation, including clinical trials, are being captured within clinical registries on a routine basis. It has been proposed that leveraging these existing registry data may be a more efficient way to power prospective research, avoiding duplicate data collection and reducing study costs. These methods have been successfully used to support clinical trials in adult cardiovascular medicine.
In the pediatric cardiovascular realm, the Pediatric Heart Network recently conducted a study to evaluate the completeness and accuracy of a site’s local surgical registry data (collected for submission to the STS Database). Results were supportive of the use of these data for a portion of the data collection required for a prospective study (e.g., the Residual Lesion Study), which is ongoing and the first example of the use of registry data for this purpose in the field.
Pediatric Cardiovascular Data: Future Directions
While there are now a number of pediatric cardiovascular data sources available for research, quality improvement, and other purposes, important limitations remain. Although several initiatives have supported greater integration and efficiencies across data sources, as described in the preceding sections, most have involved 1 : 1 data linkages to answer a specific question. More comprehensive approaches are needed to better streamline data collection; integrate information across existing and newer data sources; develop organizational models for more efficient data management, governance, and analysis; and reduce duplicative efforts, personnel, and costs. In addition to supporting more efficient research, these efforts also hold the potential to allow us to answer broader questions rather than those confined to a specific hospitalization, episode of care, or intervention, as is the focus of our current individual registries. Newer analytic approaches such as machine learning techniques are also being further investigated and may allow us to uncover important patterns in the data that would otherwise not be apparent using traditional techniques.
To begin to address these remaining challenges, a series of meetings across multiple stakeholder groups was held over the course of 2017. As a result of these meetings, five initial networks/registries agreed to collaborate and align efforts, forming Cardiac Networks United. These initial five organizations include the PC 4 , PAC3, National Pediatric Cardiology Quality Improvement Collaborative, Cardiac Neurodevelopmental Outcomes Collaborative, and Advanced Cardiac Therapies Improving Outcomes Network. Efforts are ongoing to align attempts to foster novel science not possible in individual silos, accelerate the translation of discovery to improvements in care, and reduce infrastructure and personnel costs through the sharing of data and resources.
Measurement and Description of Data
Regardless of the source of data, there are several important considerations to keep in mind when describing and analyzing pediatric cardiovascular data.
Data and Variables
Data are specific pieces of information defined by their level of measurement and their relationship to other data. They are often referred to as variables , since they may take on different values. The type of values that a variable may assume determines the level of measurement, which in turn determines how the values for a given variable should be described and how associations between variables should be assessed.
Categorical variables are those for which the values fall into discrete and mutually exclusive categories. The relationship between the different categories reflects a qualitative difference. For example, for the variable indicating type of atrial septal defect, the possible values could be ostium primum, secundum, or sinus venosus. Variables with only two possible values are referred to as being dichotomous or binary. Examples of dichotomous variables include yes versus no and right versus left.
A specific type of dichotomous categorical variable is the occurrence of a discrete event, such as receiving an intervention, or death. Events are almost always associated with a period of time at risk, which is an important aspect of that particular variable. This can be presented as the number of patients experiencing a particular event during a specified period expressed as a proportion of the total patients at risk for that event. For example, “There were 5 (13%) deaths within 30 days of surgery in 38 patients undergoing Fontan palliation.” When analyzing this type of data in more complex datasets that include varying lengths of time for which each patient is followed and patients are lost to follow-up, specific analyses that can account for these issues, called censoring , must be used. Kaplan-Meier time to event analyses are the most common seen in the medical literature (see later).
Ordinal variables reflect a specific type of categorical level of measurement in which the values can be ordered in a quantitative manner. An example would be the subjective grading of valvar regurgitation from echocardiography—trivial is less than mild, which is less than moderate. The categories are discrete and ordered, and the values would be presented in a manner similar to other categorical variables—as frequencies, proportions, and percentages. A specific quantitative value is not assigned to differences between the groups; we merely know that one category is more or less than another.
Quantitative or continuous variables are those where the difference between two values reflects a quantifiable amount. Examples include height, weight, age, ventricular ejection fraction, and blood pressure. When measured repeatedly, continuous variables tend to take on a distribution. A distribution is a description of the relative likelihood of any particular value occurring.
In describing the distribution of a continuous variable, the standard is to present some measure of the center of the values along with the magnitude and spread of their variation. The first step is to look at a frequency plot of the distribution of values. If the distribution is equal on each side of center, or bell shaped, we refer to this is as being normally distributed. In a normal distribution, the center and variation of the spread (or distance of the variables from the center) have specific definable properties or parameters. The measure of the center would be the mean or average value, and exactly half of the individual’s measures fall above or below the mean. The typical measure of variation in a normal distribution is the standard deviation. This is calculated as the sum of the square of each of the differences between the values and the mean divided by the number of values. The standard deviation details the shape of the normal curve and thereby the relationship of the all the variables’ values to the mean. In total, 66% of all values of a variable are within 1 standard deviation of the mean, 95% are within 2, and 99.7% are within 3 standard deviations.
Not all distributions are normal. If the tails or the sides of the distribution are unequal (i.e., lop-sided), it is referred to as a skewed distribution. Kurtosis refers to a distribution that is either peaked or flattened. Important skewness or kurtosis can cause the distribution to become nonnormal; the standard parameters and characteristics of mean and standard deviation then no longer apply. In this case, measures of the center should be chosen that reflect the ranking of values and not their interval magnitude. In ranking all of the values, the median value would be that measured value at the 50th percentile. For nonnormal data, the greater the amount of skewness, the greater the difference between the median value and the calculated mean. Measures of spread in a nonnormal distribution include values at specific percentiles, such as the quartile values, presented as the measured values at the 25th and 75th percentiles, with the interquartile range presented as the difference between these two values. Alternatively, the measured values at the 5th and 95th percentiles or the minimum and maximum values might be presented. Since these values are not dependent on the distribution being normal, they are often referred to as nonparametric measures.
Validity, Accuracy, and Reliability
Variables have properties reflecting the impact of how the measurements were determined. These properties include validity, accuracy, and reliability.
Validity
Validity assesses whether the measurement used is a true reflection of the desired concept. It answers the question, “Am I really measuring what I think I am measuring?” Validity can be challenging to achieve, particularly when the phenomenon being measured is qualitative and subjective.
If we take aortic valve regurgitation as an example, a subjective grading is often applied when performing echocardiographic assessment, characterized by ordinal categories of none, trivial, or trace or mild, moderate, and severe. The subjective and qualitative grade is meant to reflect the overall impression of the observer, who takes into account many aspects related to aortic valve regurgitation, such as the width of the jet, the function of the ventricle, pressure-halftime measurement, and diastolic flow reversal in the aorta. In using all of this information, we may give more weight to some over others in assigning the final grade of aortic valve regurgitation. If we wished to validate our subjective system of grading, we might start by convening a panel of expert echocardiographers and asking them first to define the concept of aortic valve regurgitation. After discussion, they may agree that no single indirect measure will suffice, and that multiple items may need to be considered simultaneously. The individual items and measures are chosen because they have content validity , meaning that they are judged to be related to specific aspects reflecting aortic valve regurgitation, and construct validity , meaning that they are judged to have a plausible causal or physiologic reason for having a relationship to aortic valve regurgitation. Alternatively, the panel may seek to measure aortic valve regurgitation using other methods, such as with magnetic resonance imaging or cardiac catheterization. This process is aimed at criterion-related validity , or the degree to which the proposed measure relates to accepted existing measures. They may also seek to assess how the subjective grade relates to clinical or outcome measures, known as predictive validity .
Accuracy
Once a measure is deemed to be valid, its accuracy and precision should be assessed. Accuracy is a reflection of validity in that it assesses how close a measure comes to the truth, but it also includes any systematic error or bias in making the measurement. Systematic error refers to variations in the measurements that might always occur predominately in one direction. In other words, the deviation of a measurement from the truth tends to be consistent. Regarding aortic valve regurgitation, this might reflect technical differences in echocardiographic assessment, as in the settings of gain or frequency of the probe that was used. This may also occur at the level of the observer, whereby the observer has a consistent bias in making the interpretation of aortic valve regurgitation, such as grading all physiologic aortic valve regurgitation as mild instead of trace. Alternatively, some observers may place more weight on a specific aspect when assigning a specific grade that tends to shift their grade assignment in one direction.
Reliability
Reliability or precision refers to the reproducibility of the measurement under a variety of circumstances and relates to random error or bias. It is the degree to which the same value is obtained when the measurement is made under the same conditions. Some of the random variation in measurements may be attributed to the instruments, such as obtaining the echocardiogram using two different machines. Some of the random variation may also relate to the subject, such as variations in physiologic state when the echocardiograms were obtained.
The reliability and accuracy of a measurement can be optimized via measurement standardization. Training sessions for observers on assessment and interpretation of a measure can be designed so that criteria for judgment are applied in a uniform manner. Limiting the number of observers, having independent adjudications, and defining and standardizing all aspects of assessment also improve reliability. In our case, this could be achieved by having the same readers assess aortic valve regurgitation using the same echocardiography machine with the same settings in patients of similar fluid status under similar resting conditions.
Analysis of Data
Analysis is the method by which data or measurements are used to answer questions, and then to assess the confidence in inferring those findings beyond the subjects that were studied. The plan for analysis of the data is an integral part of the study design and protocol. The appropriate planning, strategy, execution, and interpretation are essential elements to the critical appraisal of any research report.
Research Question
Every study must begin with a well-defined question, and the drafting of this question is the first step toward creating a research protocol. The research question often suggests the design of the study, the population to be studied, the measurements to be made, and the plan for analysis of the data. It also determines whether the study is descriptive or comparative. The process of constructing a research question is often iterative. For example, in considering the topic of hypertrophic cardiomyopathy, a descriptive research question might be “What are the outcomes of hypertrophic cardiomyopathy?” This question is nonspecific, but steps can subsequently be taken to refine and focus the question. The first step would be to determine what answers are already known regarding this question and what areas of controversy warrant further study. After a background review, an investigator may further clarify the question by asking the following: “What outcomes do I wish to study?”, “How will I define hypertrophic cardiomyopathy and in what subjects?”, and “At what time point or over what time do I wish to examine these outcomes?” In answering these questions, the research question is revised and further specified to “What is the subsequent risk of sudden death for children with familial hypertrophic cardiomyopathy presenting to a specialized clinic?” This refined question now defines the cohort to be studied—children with familial hypertrophic cardiomyopathy in a specialized clinic and the outcome of interest, sudden death—and it suggests that the study will have some type of observational design. Thus a well-defined and focused research question is essential to considering other aspects of the proposed study or report.
Using Variables to Answer Questions
Once the research question is established, the next step in generating an analysis plan is to select and define variables. Specifically, the researcher must establish the information needed to answer the question. This process should include setting definitions, determining the source(s) of data, and considering issues of measurement validity and reliability.
Types of Variables
Variables can be classified for statistical purposes as either dependent or independent variables. Dependent variables are generally the outcomes of interest, and either change in response to an intervention or are influenced by associated factors. Independent variables are those that may affect the dependent variable. The research question should define the primary independent variable, which is commonly a specific treatment or a key subject characteristic. A detailed consideration of the question should clearly identify the key or primary dependent and independent variables.
In any study there are usually one or two primary outcomes of interest, but there are often additional secondary outcomes. Analysis of secondary outcomes is used for supporting the primary outcome or exploring or generating additional hypotheses. It should be recognized that the greater the number of outcomes examined in a study, called multiple comparisons , the more likely it is that one of them will be statistically significant purely by chance. When assessing multiple comparisons, the level of certainty required to reach significance must increase.
Composite outcomes are a different but also important concept. A composite outcome results when several different outcomes are grouped together into one catchall outcome. As an example, a study of the effect of digoxin on adolescent patients with advanced heart failure might have a composite outcome of admission to the intensive care unit, listing for transplantation, and death. Having a composite outcome raises the likelihood that the study has a high enough number of outcomes to support an analysis. However, the appropriateness of composite outcomes is questionable, and issues have been raised about their validity. First, not all possible outcomes that might be included in a composite outcome have the same importance for subjects. In our example, admission to the intensive care unit and death, while both serious, would likely be deemed equivalent by very few people. Second, the creation of a composite outcome might obscure differences between the individual outcomes. Third, the risk for the component outcomes may be different with different associations. In our example, we would not be able to detect if any variables were associated specifically with intensive care unit admission. We would only be able to assess association with the composite outcome. Thus specific outcomes should be favored over composite outcomes when feasible and relevant.
Data Description and Planning the Analysis
A clear understanding of the basic characteristics of the study data is necessary to plan subsequent steps in analysis. Description of the data is important in detailing the characteristics of the subjects to be studied, usually at baseline, and it allows the researcher to determine what next steps in analysis are feasible or valid. Description is also used to determine issues that might have an impact on statistical testing, such as extreme values or outliers, missing values, categories with too few values, and skewed distributions. These issues are very important in selecting appropriate statistical testing to help answer the research question.
Types of Relationships Between Variables
An important defining feature of variables is the relationship between them. The aim of many studies is to determine relationships that are cause leading to effect; this concept is termed causality . The nature of associations and features of study designs that help to give confidence that a discovered association is cause and effect will be described later in this chapter.
Confounding occurs when the independent variable is associated with the dependent variable primarily through its relationship with a further independent variable that is more directly related to the dependent variable. Confounding is most likely to occur when independent variables are highly related or correlated with one another, which is referred to as collinearity .
For example, a hypothetical study shows an association between increased use of systemic anticoagulation and increased risk of death after the Fontan procedure. Consideration is given to recommending against the use of routine anticoagulation. Further analysis, however, reveals that the use of systemic anticoagulation was predominately in those patients with poor ventricular function. Poor ventricular function is then found to be causally and strongly related to mortality, and the association of anticoagulation with mortality is felt to be indirect and confounded because of its increased use in patients with poor ventricular function. To combat confounding, stratified, or multivariable analyses are often used to explore, detect, and adjust for confounding and to determine relationships between variables that are most likely to be independent of other variables.
Interaction is a particular type of relationship between two or more independent variables and a dependent variable in which relationship between one independent variable and the dependent variable is influenced or modified by an additional independent variable. For example, in our hypothetical study, further analysis shows that the relationship between systemic anticoagulation and mortality is more complex. For patients with poor ventricular function who are treated with systemic anticoagulation, mortality is less than for those not treated. For patients without poor ventricular function, there is no difference in mortality between those treated versus not treated with systemic anticoagulation. Thus there is an important interaction present between systemic anticoagulation and poor ventricular function as demonstrated by the differential association of anticoagulation with mortality in the presence of poor ventricular function, but anticoagulation on its own does not influence mortality.
Principles of Probability and Probabilistic Distribution: the Science of Statistics
Statistics is the science of how we make and test predictions about the true nature of the world based on measurements. The distribution of our measured data has significant implications for how well we can predict an outcome, and these implications and how statistics accounts for them is the subject of this section.
While conducting a census of every citizen of a given country, you find that the proportion of women in the population is exactly 52%, and that their average systolic blood pressure is 100 mm Hg. You select a random sample of 100 people from this same population and, to your surprise, 55% of your sample is composed of women, and their average blood pressure is 95 mm Hg. Subsequently, you decide to select a second random sample of 100 people. This time, 47% of your sample is women, and their average blood pressure is 106 mm Hg. Why do these measures differ from one another and from the census (true) values in the population?
The phenomenon at play here is called random error . Each individual sample taken randomly from a larger population will have an uncertain distribution in terms of characteristics. The distribution of characteristics in each sample is a description of the probability of each value for a given characteristic in the sample. This distribution can be plotted into a probability curve. The shape of each curve and the probability it implies have specific properties about the variation that allow us to be able to predict how frequently a given outcome will be observed in an infinite number of random samples. This is the basis for statistical inference.
Inference Based on Samples From Random Distributions
When we measure something in a research study, we may find that the values from our study subjects are different from what we might note in a normal or an alternative population. We want to know if our findings represent a true deviation from normal or whether they were just due to chance or random effect. Inferential statistics use probability distributions based on characteristics of the overall population to tell us what the likelihood might be for our observation in our subjects given that our subjects come from the overall population. We can never know for certain if our subjects truly deviate from the norm, but we can infer the probability of our observation from the probability distribution. In general, we assume that if we can be 95% certain and accept a 5% chance that our observation is really not different from normal, or the center of the probability distribution, then we state that our results are significant. The probability that the observed result may be due to chance alone represents the P value of inferential statistics.
When a random sample is selected from a population, differences between the sample and population are due to the random effect, or random error. Although the entire population in our census had an average systolic blood pressure of 100 mm Hg, this does not mean that everyone in this population had a blood pressure of 100 mm Hg. Some had 90 mm Hg and others had 120 mm Hg. Hence, if you select a random sample of 100 people, some will have higher or lower blood pressure. By chance alone, it might be that a specific sample of the population will have more people with higher blood pressure. As long as the sample was randomly chosen, the mean of your sample should be close to the mean of the population. The larger your sample, the more precise the measurement and the closer you will be to the true mean. This is because based on the actual distribution of blood pressures in the population, more individuals have a value near 100 mm Hg, and with increased samples, each individual value contributes less to the total, so extreme values have less effect on the mean.
How do we tell whether measurements are different from each other by chance or truly different? Consider this situation: a researcher polls a random sample of 100 pediatric cardiologists regarding their preferred initial therapy for heart failure, finding that 72% of the sampled physicians prefer using ACE inhibitors (ACEIs) over β-blockers. Since the sample was chosen at random, the researcher decides that it is a reasonable assumption that this group is representative of all pediatric cardiologists. A report is published titled, “ACEIs Are Preferred Over β-Blockers for the Treatment of Heart Failure in Children.” Had all pediatric cardiologists been polled, would 72% of them have chosen ACEIs? If another researcher had selected a second random sample of 100 pediatric cardiologists, would 72% of them also have chosen ACEIs over β-blockers? The answer in both cases is probably not, but if both the samples came from the same population and were chosen randomly, the results should be close. Next, suppose that a new study is subsequently published reporting that β-blockers are actually better at improving ventricular function than ACEIs. You subsequently poll a new sample of 100 pediatric cardiologists and find that only 44% now prefer ACEIs. Is the difference between your original sample and your new sample due to random error, or did the publication of the new study have an effect on preference in regard to therapy for children in heart failure? The key to answering this question is to estimate the probability by chance alone of obtaining a sample in which 44% of respondents prefer ACEIs when, in fact, 72% of the population from which the sample is drawn actually prefer ACEIs. In such a situation, inferential statistics can be used to assess the difference between the distribution in the sample as opposed to the population, and the likelihood or probability that the difference is due to chance or random error.
Relationship Between Probability and Inference
Statistical testing comparing two groups starts with the hypothesis that both groups are equivalent, also called the null hypothesis . A two-tailed test tests the probability that group A is different than group B, either higher or lower, whereas a one-tailed test tests the probability that group A is either specifically higher or lower than group B but not both. Two-tailed tests are generally used in medical research statistics (with a common exception being noninferiority trials). Statistical significance is reached when the P value obtained from the tests is under 0.05, meaning that the probability that both groups are equivalent is lower than 5%. The P value is an expression of the confidence we might have that the findings are true and not the result of random error. Using our previous example of preferred treatment for heart failure, suppose the P value was <.001 for 44% being different from 72%. This means that the chance that our sample measure of 44% was different from 72% due to random error was 1 in 1000. We can confidently conclude, therefore, that the second sample is truly different from the original sample and that the opinions of the pediatric cardiologists have changed.
Relevance of P Values
Limitations.
P < .05 is the standard value for defining statistical significance, meaning that we typically accept a result as significant if the chance of its occurrence by random error alone was less than 5%. However, there is nothing particularly unique about the specific value P < .05. If the measured P value for a statistic is .06, is the 6% probability that a result was due to chance really nonsignificant, whereas the 4% chance implied by a P value of .04 is significant? Yet many assume that if the P value is <.05, then the results are important and meaningful. This is not the case, since a P value is only a measure of confidence. Thus it is important to understand the implications and meaning of a P value. The results must always be considered in light of the magnitude of the observed difference or association, the variation in the data, the number of subjects or observations, and the clinical importance of the observed results.
Clinical Relevance Versus Statistical Significance.
A primary consideration regarding statistical analysis in medical research is the difference between statistical significance and clinical relevance. There is no real value to an association that is highly statistically significant but clinically or biologically implausible, which is a more common finding for studies with very large populations. Likewise, studies with results that are clinically important but not statistically significant are of uncertain value, which is a more common finding for studies with very small populations. Statistical significance does not necessarily equate to clinical relevance and, similarly, a result that is not statistically significant might be very clinically important.
Confidence Intervals.
Confidence intervals are important tools for assessing clinical relevance because they are intrinsically linked to a statistical P value, but they give much more information. The confidence interval is a representation of the underlying probability distribution of the observed result based on the sample size and variation in the data. A 95% confidence interval would encompass 95% of the results from similar samples with the same sample size and variation properties and thus would represent a wider range of values within which we might be confident that the true result might lie.
The confidence interval asks two questions: first, what is the likely difference between groups and, second, what are the possible values this difference might have? For example, suppose that a randomized clinical trial testing the effect a new drug called “pumpamine,” for children with low cardiac output, found a reduction in mortality with pumpamine compared with placebo, but the P value was >.05; hence the result did not achieve statistical significance. Before we conclude that pumpamine conferred no benefit, we must examine the result and the confidence interval. The trial randomized 30 patients to pumpamine and 30 to placebo. The mortality in the intensive care unit was 40% with pumpamine and 20% with placebo, giving a 20% absolute difference in mortality for pumpamine. When assessing the significance of the results, the 95% confidence interval ranges from −41% to +3%, and the P value is .09. Based on the P value, we might conclude that there is no benefit for pumpamine. But we also know that we are 95% confident that the truth lies somewhere between a 41% reduction in mortality and a 3% increase in mortality. Since the interval includes the value 0%, we cannot confidently conclude that there is a difference between the two drugs. However, we also cannot confidently conclude that there was not an important difference between the two drugs, including a benefit potentially as great as a 41% absolute reduction in mortality for pumpamine. It would be difficult to know what to conclude from this study. This situation most commonly arises when we have an insufficient number of study subjects or observations, or the study lacks adequate power .
Type I Error, Type II Error, and Power
Results drawn from samples are susceptible to two types of error: type I error and type II error. A type I error is one in which we conclude that a difference exists when in truth it does not (the observed difference was due to chance). From probability distributions, we can estimate the probability of making this type of error, which is referred to as alpha. This is also the P value—the probability that we have made a type I error. Such errors are evident when a P value is statistically significant but there is no true difference or association. In a given study, we may conduct many tests of comparison and association, and each time we are willing to accept a 5% chance of a type I error. The more tests that we do, the more likely we are to make a type I error, since by definition 5% of our tests may reach the threshold of a P value <.05 by chance or random error alone. This is the challenge of doing multiple tests or comparisons. To avoid making this error, we could lower our threshold for defining statistical significance, or we can perform adjustments that take into account the number of tests or comparisons being made. That said, for many observational studies, it is appropriate to take advantage of the opportunity to examine data in multiple ways, and interesting findings may still be of importance and necessarily rejected simply because multiple comparisons were made.
In a type II error, we conclude from the results in our sample that there is no difference or association, when in truth one actually exists. We can also determine the probability of making this type of error, called beta , from the probability distribution. Beta is most strongly influenced by the number of subjects or observations in the study, with a greater number of subjects giving a lower beta. We can use beta to calculate power, which is 1-beta. Power is the probability of concluding from the results in our sample that a difference or association exists when in truth it does exist. It is a useful calculation to make when the P value of a result is nonsignificant and we are not confident that the observed result is due to chance or random error. Before we conclude that there is no difference or association, we must be sure that we had sufficient power to detect an important difference or association reliably. As a general rule, a study reporting a negative result must have a power of at least 80%. This means that we are 80% sure that the negative results from this study are not due to a failure to reject a difference when one truly exists. We are taking a 20% chance of making a type II error. Power is affected by several factors, including the beta (or chance of a type II error) that we set, the alpha we set (a higher acceptable alpha raises power), the sample size (a bigger sample increases power), and the effect size being measured (a larger effect to detect increases power).
Applying Statistical Testing
Comparing Two or More Groups or Categories
Comparing two or more groups defined by a particular characteristic or treatment is a very common application of statistics. Using the concept of independent and dependent variables, the group assignment represents the independent variable, and we seek to determine differences in outcomes, which are the dependent variables. The type of variable being measured dictates the test used for a comparison.
If we are comparing a categorical variable (dichotomous or otherwise) between two or more groups, a chi-square test is commonly used. This test can be applied if there are more than two categories for either the dependent or independent variable or both. Chi-square testing uses the distance between the observed frequency of a variable and the frequency that would be expected under the null hypothesis to determine significance. A related test, called Fisher’s exact test, is used when the number of subjects being compared is small. If the categories of the dependent variable are ordinal in nature, then a special type of chi-square called the Mantel-Haentzel test can be an indicator of trend.
When the dependent variable is continuous and has a relatively normal distribution and the independent categorical variable has only two categories or groups, then Student’s t -test is applied. The probability of the observed difference relative to a hypothesis that there is no difference is derived from a unique probability distribution called a t-distribution. When there are more than two groups, an analysis of variance, or ANOVA, is applied, with use of the F-distribution. Importantly, if the P value for an ANOVA is significant, there is not a clear way to tell where the difference between multiple groups occurred. This is a case in which making multiple two-group comparisons is appropriate and useful.
If the dependent variable is a skewed continuous variable, then a nonparametric analysis may be needed that utilizes ranks rather than actual values. A rank is the ordinal number value of a dataset arranged in some particular order (typically from low absolute value to high absolute value). The Wilcoxon rank-sum test, also called the Mann-Whitney U test, compares two groups using the rank of a value in the set measures rather than the magnitude of the actual measure itself. When there are more than two groups to compare, Kruskal-Wallis testing can be used.
Correlations
Sometimes a statistical test is aimed not at comparing two groups but at characterizing the extent to which two variables are associated with each other. Correlations estimate the extent to which change in one variable is associated with change in a second variable. Correlations are unable to assess any cause-and-effect relationship, only associations. The strength in the association is represented by the correlation coefficient r , which can range from −1 to 1. An r value of −1 represents a perfect inverse correlation, where an increase of 1 unit in a variable is exactly associated with a decrease of 1 unit in the other. Conversely, an r value of 1 is a perfect correlation, where an increase of 1 unit in a variable is exactly associated with an increase of 1 unit in the other. An r value of zero indicates that a change in one variable is not at all associated with a change in the other.
There are many types of measures of correlation. For two ordinal variables, Spearman rank correlation is used. For two continuous variables, Pearson correlation is used. For instance, if we were studying the association of body mass index with maximal VO 2 , we might find a Pearson correlation of −0.5, meaning that for every 1 unit increase in body mass index, there was a 0.5 unit decrease in VO 2 .
Matched Pairs and Measures of Agreement
When measurements are made in two groups of subjects composed of separate individuals that bear no relationship to one another, we use nonmatched or unpaired statistics. Alternatively, the two groups may not be independent but have an individual-level relationship, such as a group of subjects and a group of their siblings or the systolic blood pressure measurement in an individual before and after antihypertensive medication. When this is the case, we must use statistical testing that takes into account the fact that the two groups are not independent. If the independent variable was categorical, we would use a McNemar chi-square test. If the independent variable was ordinal, we would use an appropriate nonparametric type of test, such as the Wilcoxon signed rank test. If the independent variable was continuous, we would use a paired t -test.
Linear and Logistic Regression
Often the relationship between two variables can be represented by a line graphed on a set of axes. In these cases, regression analyses are powerful and useful tools. For continuous dependent variables, that line can be straight, in which case the appropriate analysis would be linear regression. Sometimes the relationship is more complex over the range of values of the dependent variable and may not be linear. In this case, transformations of dependent and independent variables may be used, or nonlinear regression techniques can be applied. If the dependent variable is dichotomous, then the relationship is between the probability of a value of the dependent variable as a function of the independent variable. In this case a logistic regression is used. These different regression techniques can be applied to incorporate the relationship between the dependent variable and multiple independent variables. Regression equations are very useful in determining the independent effect of specific variables of interest on a given dependent variable by allowing the investigator to account for bias resulting from potential confounding factors. It should be noted that although this reduces bias, the adjustments are always incomplete, as they account only for factors that have been included or measured. Bias or confounding can (and usually are) always present from unmeasured factors. Statistical testing can be applied to the whole regression equation and to the individual variables that were included. Interaction can also be explored within a regression analysis.
Survival or Time-to-Event Analysis
In a survival analysis, the dependent variable is time-to-event, and we assess how independent variables influence this time. Since times-to-events are usually not normally distributed and there are incomplete data regarding individuals who do not reach an observed event, standard multivariable regression models that ignore the time element are not ideal. Additionally, we must make sure that all of the surviving subjects at risk are accounted for. One of the challenges of following cohorts over time is that subjects may be lost to follow-up or be lost to further observation before they achieve the event of interest, which is known as censoring . They may also have other events, called competing events , that preclude the event of interest, as in an analysis of time to heart transplantation, where some subjects die. To depict these phenomena accurately over time, we must continuously account for these changes to the cohort both for people who reach the outcome and for those who are no longer available to study or at risk for the event of interest.
The most common form of time-to-event analysis is the Kaplan-Meier approach. Here the proportion of individuals surviving out of all individuals still available to be measured (at risk) is plotted in series of time intervals. Kaplan-Meier takes into account that subjects are dropping out of both the numerator (having events) and the denominator (ending their period of observation) over time. Typically, the proportion surviving (or not reaching the specified event) is plotted on the y -axis and time is plotted on the x -axis, which gives a visual representation of temporal survival trends. Further, after creating the plot, we can use statistical tests to determine if independent variables have an association with time-to-event using Wilcoxon and log rank tests. We can also use a particular type of multivariable regression analysis that handles time-related events as the dependent variable by using Cox’s proportional hazard regression modeling. This allows us to explore the relationship and independence of multiple variables with time to event.
Longitudinal or Serial/Repeated Measures Analysis
The value of some variables can change over time if we measure them repeatedly, and trends can be noted. An example might be left ventricular ejection fraction in an individual. If we measure something repeatedly in a subject, then the measures for that individual are not independent—they are clearly related to one another and more related to each other than to the measurement of left ventricular ejection fraction in a different individual. We need to account for this in an analysis. We may also wish to determine if independent variables are associated with the measurements’ change over time. Specific types of regression analyses have been developed and applied to handle this type of complex data. If the serial measurements are of a continuous variable, then mixed linear or nonlinear regression analysis can be used. If the variable is categorical or ordinal, a general estimating equation type of regression analysis can be used.
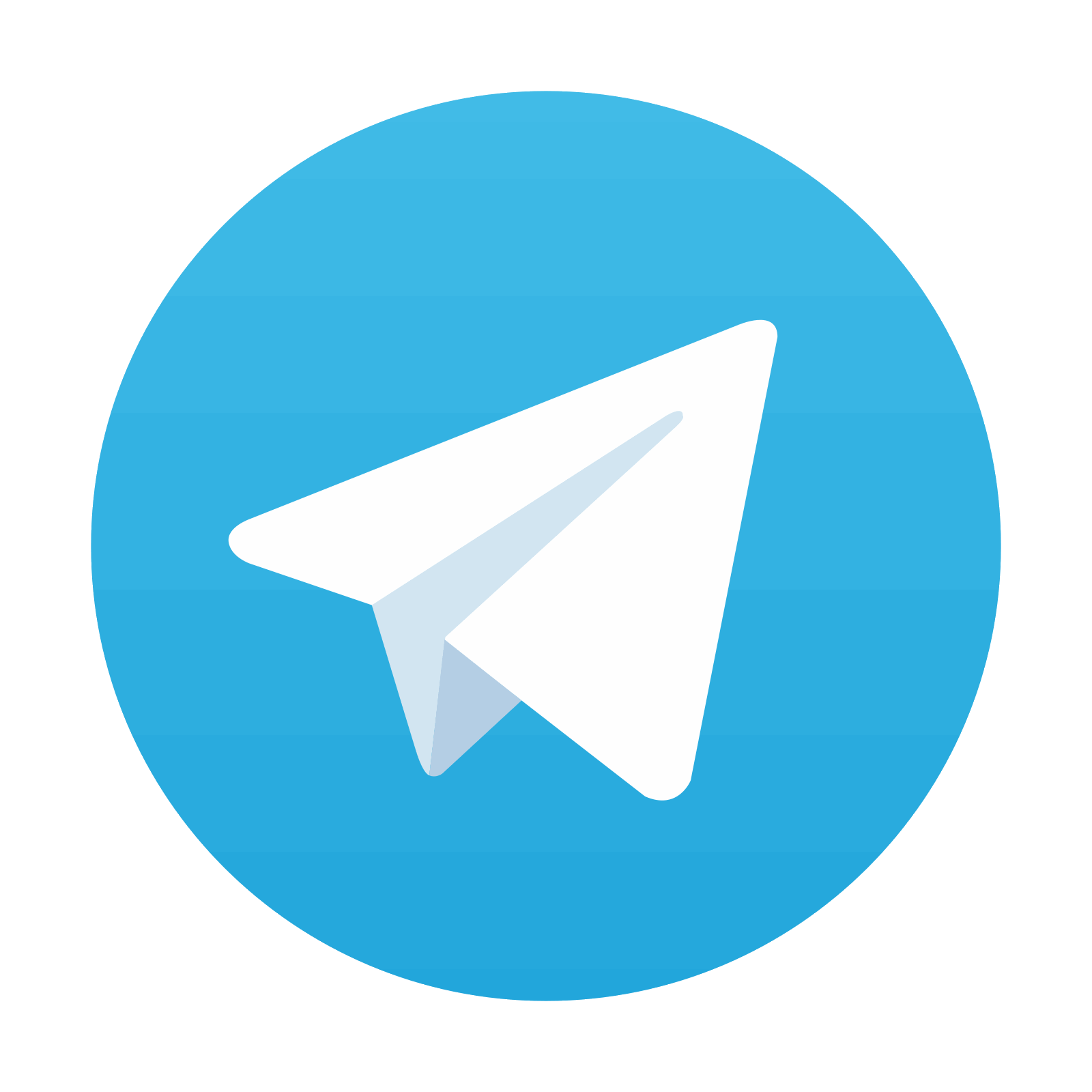
Stay updated, free articles. Join our Telegram channel

Full access? Get Clinical Tree
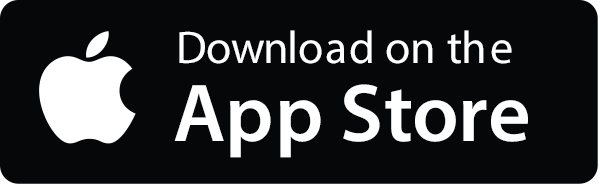
