A shared understanding of medical conditions between patients and their health care providers may improve self-care and outcomes. In this study, the concordance between responses to a medical history self-report (MHSR) form and the corresponding provider documentation in electronic health records (EHRs) of 19 select co-morbidities and habits in 230 patients with heart failure were evaluated. Overall concordance was assessed using the κ statistic, and crude, positive, and negative agreement were determined for each condition. Concordance between MHSR and EHR varied widely for cardiovascular conditions (κ = 0.37 to 0.96), noncardiovascular conditions (κ = 0.06 to 1.00), and habits (κ = 0.26 to 0.69). Less than 80% crude agreement was seen for history of arrhythmias (72%), dyslipidemia (74%), and hypertension (79%) among cardiovascular conditions and lung disease (70%) and peripheral arterial disease (78%) for noncardiovascular conditions. Perfect agreement was observed for only 1 of the 19 conditions (human immunodeficiency virus status). Negative agreement >80% was more frequent than >80% positive agreement for a condition (15 of 19 [79%] vs 8 of 19 [42%], respectively, p = 0.02). Only 20% of patients had concordant MSHRs and EHRs for all 7 cardiovascular conditions; in 40% of patients, concordance was observed for ≤5 conditions. For noncardiovascular conditions, only 28% of MSHR-EHR pairs agreed for all 9 conditions; 37% agreed for ≤7 conditions. Cumulatively, 39% of the pairs matched for ≤15 of 19 conditions. In conclusion, there is significant variation in the perceptions of patients with heart failure compared to providers’ records of co-morbidities and habits. The root causes of this variation and its impact on outcomes need further study.
Heart failure (HF) prevalence is growing and primarily affects the elderly. The complex array of physiologic, psychological, social, and health care delivery issues that accompany HF make it a difficult chronic disease to manage. Optimal self-care behavior is important for achieving the best outcomes for chronic diseases such as HF. For patients to actively participate in their care, however, it is important for them to have a clear understanding of their health-related problems. This is particularly critical for patients with HF, as they tend to be older, have a higher co-morbidity burden, and often require complex treatment plans. From a provider perspective, medical record documentation of disease states is an essential part of care provision. This is especially true in the current era of increasing use of electronic health records (EHRs), as many providers communicate information exclusively through this medium. It may be assumed that what is documented in EHRs is the same as patients’ understanding and reporting. However, if this is not true, this discordance may lend itself to poor patient self-care behavior (related to not understanding or not reporting their conditions) or to insufficient medical care (due to misunderstanding by providers). In the current era, whether EHR entries are congruent with patients’ reporting of health-related conditions, and to what extent, is not known. In this study, we sought to assess and compare patient self-report versus EHR documentation of cardiovascular and noncardiovascular conditions and behavioral habits in patients with HF.
Methods
The data for this study were derived from patients enrolled in the Atlanta Cardiomyopathy Consortium. This prospective cohort study is enrolling patients from the Emory University Hospital, Emory University Hospital Midtown, and the Grady Memorial Hospital in Atlanta, Georgia. All patients undergo detailed medical history surveys, electrocardiography, 6-minute walk tests, standardized questionnaires, and collection of blood and urine samples at baseline. Every 6 months, patients are contacted to assess outcomes, including interim medication changes, procedures, new disease diagnoses, and hospitalizations. Mortality data are collected through medical record review, information obtained from family members, and Social Security Death Index query. The institutional review board has approved the study. At the time of this analysis, a total of 238 patients were enrolled; we included 230 of these patients (96.6%), excluding 8 patients who did not complete medical history surveys.
Research nurses abstracted data from the EHRs independently without discussion with the patients or their survey documentation. The main source of EHR data (n = 222 [96.5%]) was Emory Healthcare’s electronic medical record system, which is based on the Cerner Millennium platform (Cerner Corporation, Kansas City, Missouri). The system provides a comprehensive view of clinical data collected across hospitals and clinics. Data on 8 patients (3.5%) were collected from the EHR system at the Grady Memorial Hospital, which is based on the Siemens Medical Solutions (Malvern, Pennsylvania) Health Services platform.
All patients completed a medical history self-report (MHSR) form, which included questions regarding cardiovascular conditions (history of heart attack or myocardial infarction, high blood pressure or hypertension, high cholesterol, heart rhythm problems or arrhythmias, coronary artery bypass graft surgery, coronary stent placement, and implantable cardioverter defibrillator or pacemaker implantation), and noncardiovascular conditions (diabetes mellitus, peripheral arterial disease, pulmonary disease, liver disease, peptic ulcer disease, thyroid disease, cancer, osteoarthritis, and human immunodeficiency virus (HIV) infection). The pulmonary disease question was open ended, allowing patients to manually enter specific diagnoses. Data on history of tobacco, alcohol, and cocaine use were also obtained.
To assess the reliability of EHR data abstraction, data on 10% of the total charts, selected using a random number generator ( http://www.random.org ), were independently abstracted. Cumulative agreement between the 2 independent EHR data abstractions for all study variables was 93.1%.
EHR data for each condition (yes or no) were compared with the data from MHSR forms (yes or no), and concordance was assessed using the κ statistic. Crude, positive, and negative agreement were calculated to facilitate interpretation of κ values. Crude agreement is equal to the number of pairs that agree divided by the number of pairs available for analysis. The number of pairs available differed for each condition because of missing values in patient responses. Positive and negative agreement measures were calculated. The positive agreement measure is the ratio of total concordant positive responses over the average positive responses of patients and EHRs. The negative agreement is the ratio of total concordant negative responses over the average negative responses of patients and EHRs. Kappa statistics were interpreted as follows : values of 0.93 to 1.00 denote almost perfect agreement, 0.81 to 0.92 very good agreement, 0.61 to 0.80 substantial agreement, 0.41 to 0.60 moderate agreement, 0.21 to 0.40 fair agreement, 0.01 to 0.20 slight agreement, and 0 no agreement. Finally, to summarize agreement by patient, the sum of the number of concordant conditions per participant was calculated. There were 7 cardiovascular and 9 noncardiovascular conditions and 3 habits included in the summary measure. McNemar’s statistic was calculated for paired comparisons. Finally, patients’ responses as “don’t know” to select conditions were captured and compared with EHR data. All analyses were performed using SAS version 9.2 (SAS Institute Inc., Cary, North Carolina).
Results
The baseline patient characteristics and treatment pattern are listed in Table 1 . The mean age of patients was 56.6 ± 11.9 years; 64.5% were men, and 55.2% were white. The mean left ventricular ejection fraction was 39.3 ± 14.6%.
Characteristic | Value |
---|---|
Age (years) | 56.6 ± 11.9 |
Male | 149 (64.8%) |
White | 127 (55.2%) |
Education (years) | 14.1 ± 3.1 |
Living alone | 41 (17.9%) |
Insured | 212 (92.2%) |
Married | 143 (62.2%) |
Ischemic cause of HF | 69 (31.3%) |
Left ventricular ejection fraction (%) | 39.3 ± 14.6 |
Systolic blood pressure (mm Hg) | 112 ± 18 |
Diastolic blood pressure (mm Hg) | 71 ± 11 |
Heart rate (beats/min) | 72 ± 11 |
Creatinine (mg/dl) | 1.4 ± 1.1 |
Sodium (mEq/L) | 138 ± 3 |
Hemoglobin (g/dl) | 13.3 ± 1.8 |
Brain natriuretic peptide (ng/L) | 202 (73–664) |
β-blocker use | 219 (94.8%) |
Angiotensin-converting enzyme inhibitor or angiotensin receptor blocker use | 197 (85.6%) |
Defibrillator/pacemaker | 145 (64.5%) |
Table 2 lists the agreement data. There was fair agreement for arrhythmia history and moderate agreement for dyslipidemia and hypertension. The strongest agreement was noted for procedural care, including coronary artery bypass grafting and implantable cardioverter-defibrillator and/or pacemaker implantation. For noncardiovascular conditions, there was only fair agreement for pulmonary disease; of the 47 of 82 patients (57%) who entered specific diagnoses, there was poor agreement for chronic obstructive pulmonary disease, asthma, and sleep apnea (not listed in Table 2 ). There was very good agreement for cancer and diabetes mellitus and perfect agreement for HIV. For alcohol use, there was fair agreement. In 80% of the patients (12 of 15) in whom there was disagreement, the patients did not report alcohol use when the EHRs suggested histories. There was moderate agreement for cocaine and tobacco use. “Don’t know” responses were uncommon, including 13 for myocardial infarction (12 had no EHR documentation), 6 for stents and 1 for coronary bypass surgery (all with no EHR entries), and 2 for diabetes mellitus (1 had EHR documentation).
Co-Morbidity | Number | Yes/Yes | No/No | Yes/No | No/Yes | Crude Agreement | Positive Agreement | Negative Agreement | κ |
---|---|---|---|---|---|---|---|---|---|
Cardiovascular | |||||||||
Arrhythmia | 191 | 103 | 35 | 36 | 17 | 72% | 80% | 57% | 0.37 |
Dyslipidemia ⁎ | 223 | 94 | 71 | 34 | 24 | 74% | 76% | 71% | 0.48 |
Hypertension | 224 | 127 | 49 | 26 | 22 | 79% | 84% | 67% | 0.51 |
Stent | 221 | 15 | 185 | 21 | 0 | 90% | 59% | 95% | 0.54 |
Myocardial infarction | 196 | 50 | 123 | 16 | 7 | 88% | 81% | 91% | 0.73 |
Coronary bypass surgery | 225 | 39 | 183 | 3 | 0 | 99% | 97% | 99% | 0.95 |
Defibrillator/pacemaker | 221 | 140 | 77 | 3 | 1 | 98% | 99% | 98% | 0.96 |
Noncardiovascular | |||||||||
Peptic ulcer disease | 218 | 1 | 198 | 4 | 15 | 91% | 10% | 95% | 0.06 |
Peripheral arterial disease | 230 | 4 | 176 | 0 | 50 | 78% | 14% | 88% | 0.11 |
Lung disease | 203 | 29 | 113 | 39 | 22 | 70% | 49% | 79% | 0.28 |
Osteoarthritis | 215 | 10 | 174 | 23 | 8 | 86% | 39% | 92% | 0.32 |
Liver disease | 225 | 3 | 212 | 2 | 8 | 96% | 38% | 98% | 0.36 |
Thyroid | 214 | 26 | 174 | 7 | 7 | 93% | 79% | 96% | 0.75 |
Cancer | 229 | 34 | 185 | 8 | 2 | 96% | 87% | 97% | 0.85 |
Diabetes mellitus | 228 | 69 | 144 | 6 | 9 | 93% | 90% | 95% | 0.85 |
HIV infection | 182 | 1 | 181 | 0 | 0 | 100% | 100% | 100% | 1.00 |
Behavioral | |||||||||
Excess alcohol use | 218 | 3 | 200 | 3 | 12 | 93% | 29% | 96% | 0.26 |
Cocaine abuse | 224 | 11 | 198 | 14 | 1 | 93% | 59% | 96% | 0.56 |
Tobacco | 203 | 64 | 108 | 31 | 0 | 85% | 81% | 87% | 0.69 |
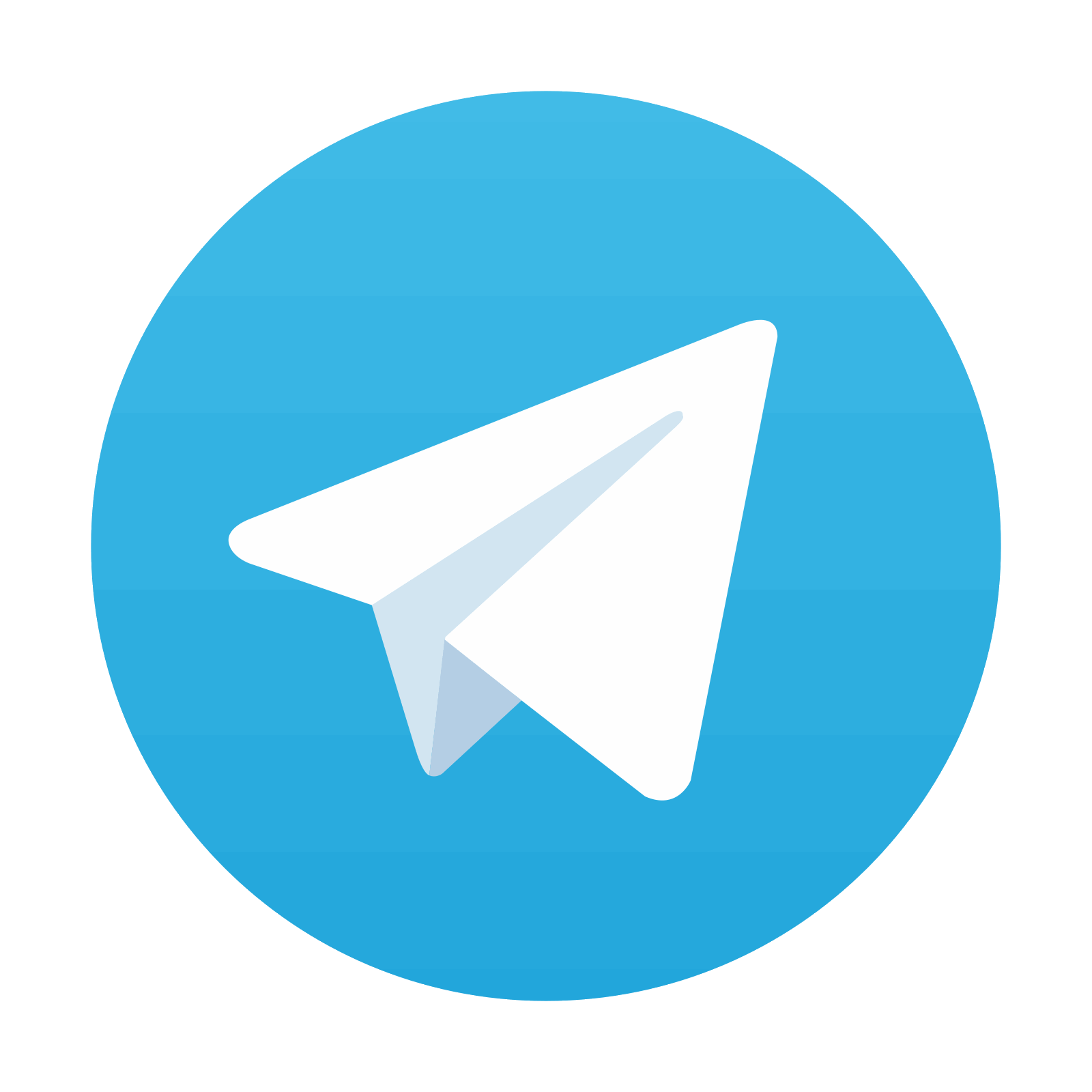
Stay updated, free articles. Join our Telegram channel

Full access? Get Clinical Tree
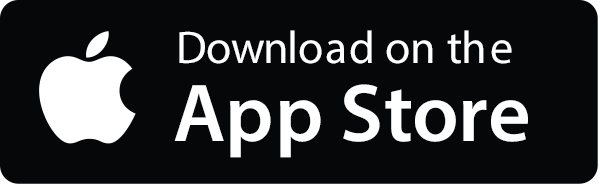

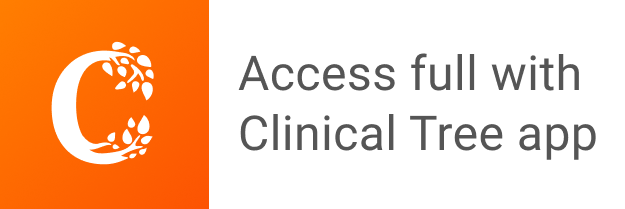