Outcomes in Echocardiography: Statistical Considerations
Colleen Gorman Koch
To guess is cheap.
To guess wrong is expensive.
Old Chinese Proverb
OUTCOMES RESEARCH
Outcomes research has achieved considerable stature over the last two decades. An increased emphasis on outcomes and assessment of effectiveness has come about in response to a number of factors. Among these factors is the observation of unexplained variation in medical care across geographic areas (1). Variation in practice patterns can, in part, be explained by patient preferences and/or differences in demographics (2). However, variation may also reflect suboptimal care in the low-use areas or needless cost in the high-use areas (3). Considerable variations in practice patterns are likely influenced by physicians’ approaches to clinical decision making. Physicians may approach similar medical problems with different theoretical assumptions. Eckman described it in Bayesian terms: Physicians bring differing prior probabilities to the decision making process, which leads to variations in their medical decisions (4). Difficulty incorporating an understanding of disease probabilities into decisions is potentially magnified further by variability in the perceptions of outcomes (2,4). The economics of health care have further driven the increased emphasis on outcomes. The need for cost containment in response to escalating health-care costs (2,3,5), competition within the healthcare sector (3), limitations of randomized control trials (6), findings of inappropriate use of medical services (1), and the need for greater accountability have all driven the increased interest in outcomes.
The field of outcomes research distinguishes itself from traditional research in that it can embrace a broader range of endpoints. It may focus on a number of patientcentered outcomes such as measurements of patient satisfaction and quality of life, daily functioning activities, and economics (3,7,8). Another distinguishing feature of outcomes research is its emphasis on effectiveness rather than efficacy. Efficacy denotes “usefulness of a medical intervention tested under optimal conditions” (9). Effectiveness can be thought of as the “utility” of a health-care intervention in routine clinical practice (9). An additional factor determining the usefulness of a medical intervention is its efficiency, that is, the value of the intervention to the individual patient (9). Johanson summarized these in a practical way: “efficacy addresses the question of whether an intervention can work, effectiveness answers the question of whether it works in a routine practice setting and efficiency determines whether it is worth doing” (9). These concepts figure prominently into the development of practice guidelines from the results of outcomes research. Similar to other research methods, outcomes research focuses on two things: choosing the right variable to study and selecting the data source to make inferences about that specific variable and its relationship to other independent variables (10). Perrin and Mitchell state that “outcomes research gets its name from the choice of the dependent variable.” It is the choice of, the definition of, and the development of measures for the dependent variable that has been the focus of research for the last decade. He stressed that current emphasis should be on the inference process: “defining the independent variables and thinking about study design and data sets that allow inferences to be made to set the tone for outcomes research for the future” (10).
A number of goals can be achieved by focusing on outcomes: increased understanding of the effectiveness of various treatment strategies; identifying the most efficient and effective use of limited resources and integrating these into the development of medical standards
and practice guidelines; and finally, optimizing the use of resources by third-party payers (3,9). The creation of medical practice guidelines by consensus panels assists physicians in the clinical application of specific diagnostic tests. The American College of Cardiology (ACC) and the American Heart Association (AHA) have developed guidelines for the clinical application of echocardiography. A panel of experts in the field of echocardiography developed the guidelines based on expert opinion and extensive review of the literature. The guidelines serve as a summary of current knowledge on the effectiveness of the imaging test, specifically classifying the clinical utility of echocardiography for specific cardiovascular diseases and common cardiovascular symptoms. Class I reflects evidence or general agreement that the given procedure is effective. Class II reflects conditions where there is conflicting evidence or opinion with regard to the usefulness of echocardiography. Class III reflects conditions for which there is evidence or general agreement that the procedure is not effective or useful (11,12). Recommendations for the use of transesophageal echocardiography (TEE) follow a similar guideline structure, provided by the American Society of Anesthesiologists and the Society of Cardiovascular Anesthesiologists Task Force on Transesophageal Echocardiography (13).
and practice guidelines; and finally, optimizing the use of resources by third-party payers (3,9). The creation of medical practice guidelines by consensus panels assists physicians in the clinical application of specific diagnostic tests. The American College of Cardiology (ACC) and the American Heart Association (AHA) have developed guidelines for the clinical application of echocardiography. A panel of experts in the field of echocardiography developed the guidelines based on expert opinion and extensive review of the literature. The guidelines serve as a summary of current knowledge on the effectiveness of the imaging test, specifically classifying the clinical utility of echocardiography for specific cardiovascular diseases and common cardiovascular symptoms. Class I reflects evidence or general agreement that the given procedure is effective. Class II reflects conditions where there is conflicting evidence or opinion with regard to the usefulness of echocardiography. Class III reflects conditions for which there is evidence or general agreement that the procedure is not effective or useful (11,12). Recommendations for the use of transesophageal echocardiography (TEE) follow a similar guideline structure, provided by the American Society of Anesthesiologists and the Society of Cardiovascular Anesthesiologists Task Force on Transesophageal Echocardiography (13).
DATA SOURCES AND METHODS FOR ANALYZING OUTCOMES RESEARCH
Outcomes research may incorporate a variety of methodologies and approaches to collecting, examining, and analyzing data. Data sources include results from randomized clinical trials, quasiexperimental designs, and effectiveness trials (9), cohort or case-control designs, or other retrospective analyses from databases and routine observational data from hospital discharge summaries (3,7,8,14). Data synthesis can be performed in multiple ways, such as metaanalysis, decision analysis, and crossdesign synthesis. It is useful to consider the strength of the evidence produced by a study as it relates specifically to a study design; data sources can be listed in descending order of the strength of evidence they produce: randomized controlled trial, nonrandomized trial with contemporaneous controls, nonrandomized trial with historical controls, cohort study, case-control study, cross-sectional study, surveillance (database), descriptive study, and case report (15,16). All of these methods have strengths and weaknesses and can provide valuable information for outcomes research.
Randomized Controlled Trials
From a design perspective, randomized controlled trials are the strongest for internal consistency (10). The goal of the randomized controlled trial is to reduce variability and bias. Pocock defined variability as “that which deals with imprecision in estimates caused by sampling from non-homogeneous populations” and bias as “any influence which acts to make the observed results non-representative of the true effects of therapy” (17). By the randomization process, randomized controlled trials control for known and unknown confounding variables (3), the end result being comparable groups with regard to comorbid conditions (17). These key design elements are intended to “isolate” or clarify differences in outcome due to treatment assignment rather than specific differences in the patient population (18). Randomization also reduces conscious, as well as unconscious biases, such as those that may occur with patient selection (17). Another strength of randomized controlled trials is their ability to assess efficacy; that is, whether an intervention will produce a desired effect under the best possible circumstances (3). Because of highly specific inclusion and exclusion criteria, randomized controlled trials may not be universally applicable, nor reveal how effective the intervention is for many patients in every day clinical practice (3,18). Finally, not all questions are suitable nor can they all be answered by randomized controlled trials.
Quasiexperimental Designs
Quasiexperimental designs are studies that manipulate treatment without randomization. Within the control group there is some control over treatment assignment, however, the control group is not randomized. A disadvantage of this experimental design can be a systematic difference between the groups being compared, which can influence outcomes beyond the assigned treatment (19). Quasiexperimental designs can use statistical techniques, such as stratification, matching, and structural modeling to control for known confounding variables (3).
Effectiveness Trials
Effectiveness trials differ in methodology from randomized controlled trials in that they are population based, often retrospective (1), and are significantly less restrictive. There may be no prescribed protocol, no random assignment of treatment or health-care provider, and there are less stringent exclusion criteria. These trials may include patients with multiple comorbid conditions, occur in office settings and community hospitals, and include an extensive mix of investigators (9). Effectiveness trials can provide practical information about patients seen in routine clinical practice (9). Because of the lack of randomization, risk adjustment strategies are used to control for influential variables in assessing the effectiveness of the health-care intervention (1).
Cohort Studies
Cohort, or prospective, studies involve two or more groups of “exposed” and “nonexposed” individuals in which groups are followed up to compare the incidence of disease over a period of time. These studies work well when exposure is rare and disease is frequent among the exposed. Cohort studies do not involve a randomization process, in that patients are not randomized to the exposure; therefore, the observed associations between exposure and disease outcome may be influenced by known or unknown variables. A number of potential biases are associated with conducting cohort studies: bias in the assessment of the outcome, information, nonresponse and analytic biases, and patients who are lost to follow-up (20,21).
Case-Control Studies
Case-control, or retrospective, studies identify groups of patients with a disease—“cases” and comparison groups without disease termed “controls.” A determination may then be made as to the proportion of cases and controls that were exposed or not exposed. These studies are optimal when a disease is rare and when exposure is frequent among the population. Since case-control studies require information from past events or exposures, there are potential sources of error with incomplete information about exposure, limitations of recall, and recall bias. Control selection may also be problematic. Matching cases and controls on important variables known to be associated with the disease can help avoid the differences in exposure between the cases and controls being attributable to factors other than exposure status (21,22).
Databases
Observational data from large databases from which effectiveness outcome studies are reported are primarily compiled for administrative and billing purposes rather than research purposes (18,23). Data acquired for large databases are collected prospectively and therefore not biased by any selection process (9). Database information is obtained from routine clinical practice and therefore may be more reflective of the breadth of medical practice than the randomized trial (64). Observational databases can serve as useful guides to the design of new controlled trials. They can also be useful adjuncts to randomized controlled trials to assess whether efficacy can be translated into effective treatments in routine clinical practice (24). Concato and colleagues reported that well-designed observational studies do not systematically overestimate the magnitude of effects of treatment as compared to randomized controlled trials on the same topic. The popular belief that observational studies are inherently misleading is dispelled by this work (16).
However, methodologies used for large effectiveness outcome studies from databases can lead to erroneous conclusions (9). Data collected for observational studies are uncontrolled observations and, because each person’s treatment is chosen rather than randomly assigned, they are prone to selection biases that would be eliminated by the randomization process (9,24). Among other concerns are that there is often a lack of standardized definitions used, medical records may be incomplete, physicians’ decisions regarding treatment assignments are not at random, and there may be issues with chart abstraction of data (18,23). Without proper controls, these data sources cannot address specific differences in clinical effectiveness of treatment strategies and therefore, specific treatment comparisons should be interpreted cautiously. Because these large databases rarely contain detailed information on clinical severity, comorbid conditions can be unaccounted for, thus creating potentially important differences in the patient populations (18,23,25). Statistical models can be used to adjust for influential variables between the patient groups being compared that could impact on patient outcome (6,9). However, it is difficult to assume that statisticians know which variables are influential, measure those variables on each patient, and use those measurements to make the appropriate adjustments. Certainly, unknown variables can have important influences on outcome (15). Adjusting for severity may also introduce bias caused by timely changes in severity assessments or by the validity of the adjustment scheme (6).
Propensity modeling represents an advance in statistical techniques as applied to nonrandomized study designs to control for differences in background characteristics among groups under investigation (26). Prior to propensity modeling, multivariable risk factor analysis was the primary methodology used to adjust for baseline differences when examining specific outcomes (29). The propensity score is the conditional probability of assignment to a group given a number of observed covariates (27). It is calculated by predicting group membership from a number of confounding covariates with logistic regression or discriminant analysis (26). The propensity score can be seen as a single confounding covariate representing a collection of confounding covariates. At each given value of propensity score, the distribution of covariates is typically balanced between groups allowing for equivalent comparisons to be made between groups under investigation (28). Particular applications of the propensity score include matching, stratification, or multivariable adjustment on propensity score (26,27,28,29).
Difficulties with large databases can be averted by avoiding the use of databases designed for billing or administrative purposes and instead, collecting clinical data according to a specific protocol with a quality assurance program (15).
DATA SYNTHESIS METHODS
Metaanalysis
The term metaanalysis was first coined by GV Glass in 1976 (30,31) for a quantitative technique that combines the results of multiple studies investigating the same question with roughly the same experimental design or quasiexperimental design (30). Metaanalysis seeks to explore reasons for disparate findings among clinical studies. Many studies are inconclusive, often because of insufficient sample size (30,32,33). By combining the results from a number of studies, metaanalysis can detect patterns across studies, as well as, in some cases, give more precise estimates of treatment effects (30,32). Bangert-Drowns described metaanalysis as each individual study being represented by a data point with its own probabilistic distribution (30). The process of metaanalysis includes: formulating a specific research question, collecting studies through a literature search, and analyzing the quality of the studies. Design characteristics and quality scores for each study are used to develop inclusion and exclusion criteria or for weighing individual study results in a theoretically or mathematically more powerful pooled analysis. The individual study characteristics and outcomes are coded and translated into a common metric and relations between study features and outcomes are statistically tested (30,32,34,35).
Decision Analysis
Decision analysis, a derivative of operations research and game theory, is a structured step-wise process of combining data to compare treatment strategies by simulation. Two or more treatment strategies are compared in quantitative terms along with potential outcomes of each strategy. These are represented in the form of a decision tree (32,36,37). The concept of decision analysis involves structuring a problem as a decision model, such that alternative therapeutic strategies are specified and the important outcomes selected. Estimates of the probabilities for the outcomes are based upon a systematic search of the literature focusing on those studies with good methodology (20,32). Quantitative values for the outcomes can be expressed in a number of ways: life years, quality-adjusted life years (QALYs), cases of disease, complications prevented, or utilities (20). The product of outcome values and their probabilities of occurrence is the expected value of each clinical strategy (32). A sensitivity analysis is performed to evaluate the degree of uncertainty of the estimates, which is similar to a confidence interval (36). Sensitivity analysis can focus attention on probability estimates that need to be defined more precisely and can provide insight into the “robustness” of the baseline analysis (36).
Economic Analysis
There are a number of economic analyses: cost-identification, cost-benefit, cost-utility, and cost-effectiveness (38). Cost-effectiveness analysis requires that an intervention be compared to alternatives; implicit with this type of research is the acknowledgement that resources are finite. Economics defines natural units (dollars) that are applicable across all strategies and whose nature is to reflect value. The objective of the analysis may not be so much to limit care or expense, but to limit waste and promote efficiency with the greatest overall benefit (38). The defining principles of cost-effectiveness research include the following: explicitly state the perspective of the analysis; define what benefits are being studied and how they will be measured; define the costs being studied; discount costs and benefits to the extent that costs and benefits occur at different times; and provide a summary statement—a ratio of costs to benefits (39,40). Costs, like risks, may not drive the medical decision-making process, but lend perspective to the pursuit of the best strategy. The importance of the economic assessment and, in particular, the relationship of decision analytical modeling and cost-effectiveness is stressed by Mushlin (41). Decision analytic models can assist in determining those diagnostic procedures that are or are not cost-effective (41). One of the problems with decision analysis is that it may oversimplify medical problems (36). Furthermore, there are particular medical problems that cannot be broken down into a finite set of discrete events with well-defined probabilities (18) or there may not be available data to support the analysis, and there have been methodological problems with measurements such as quality of life (36).
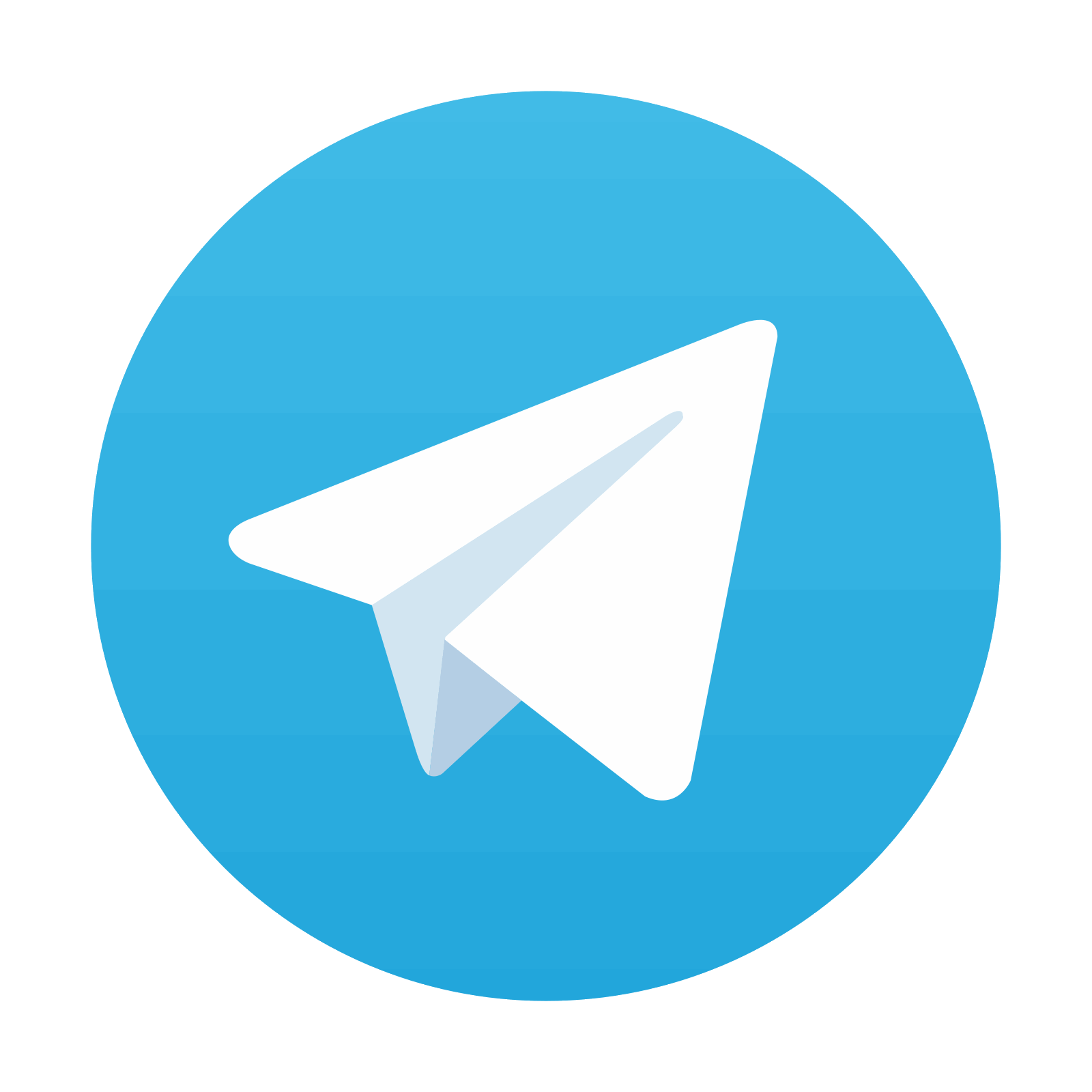
Stay updated, free articles. Join our Telegram channel

Full access? Get Clinical Tree
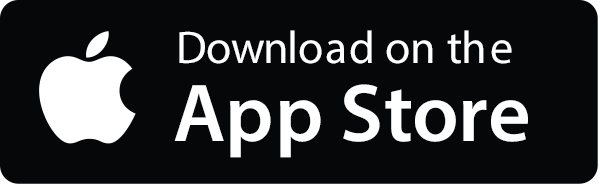
