Background
The aim of this study was to find the best model to obtain valid and normally distributed Z scores for coronary artery (CA) diameters in a large, heterogeneous population of healthy children.
Methods
Echocardiography was performed on 1,033 healthy children. Several regression models were tested with height, weight, body surface area, and aortic valve diameter. The computed Z scores were tested for normal distribution and stability.
Results
CA diameter was best predicted using regression with the square root of body surface area. The weighted least squares method yielded normally distributed and very stable Z -score estimates for all CA segments. In prepubertal children, aortic valve diameter was also a valid predictor of CA diameter.
Conclusions
This study shows two valid methods to estimate Z scores for CA size in children of all ages. Such Z scores are important for risk stratification in patients with Kawasaki disease.
Kawasaki disease (KD) is the most common acquired heart disease in the developed world and the primary etiology of acquired coronary artery (CA) disease during childhood. The occurrence of a CA aneurysm is indeed the most severe outcome in KD, but even the transitory dilatation of the CAs remains essential in the diagnosis, management, and follow-up of children with KD. In fact, the identification of CA dilatation upon the onset of KD has become central to support the diagnosis and guide therapy. The initial recommendations for the case definition of CA dilatation were issued by the Japan Ministry of Health. However, their use was limited because of the lack of a correlation with body habitus and the nondifferentiation between the right and the left CAs. The categorization of aneurysm size seemed to represent a prognostic value, but equations predicting CA growth were needed. Since then, equations have been published, and both linear and exponential functions with body surface area (BSA) were proposed. Heteroscedasticity—the increasing dispersion of standard deviation with the independent variable—was considered only in the later two studies. Normal distribution and stability of a Z score with growth are crucial for its validity, but such information is lacking in all previously published studies. There are also concerns regarding the simple coronary/aortic valve ratio equations proposed by Tan et al. because of the small sample size and the restriction of the study sample to children aged 2 months to 8 years. In this study, we sought to redefine Z -score equations from a large pediatric cohort with appropriate statistical validations. The aim was to propose accurate and validated Z -score equations by testing several different models to ensure not only appropriate goodness of fit but also adequate distribution of the Z scores obtained. We also sought to investigate the accuracy of coronary/aortic valve ratios as an alternative way of assessing CA dilatation.
Methods
Population
All children referred to the pediatric cardiology outpatient clinic of Sainte-Justine Hospital (Montreal, Quebec, Canada) between 2001 and 2008 for systolic murmur, syncope, or chest pain who underwent complete echocardiographic studies were considered for inclusion. Patients were included if the syncope or chest pain was considered benign, they had normal physical examination results (including physiologic murmur), and their echocardiograms were interpreted as normal by their attending cardiologists. Patients were excluded if they had concurrent unexplained fever; evidence of any structural anomaly, ventricular dilatation, or hypertrophy; or suspicion or history of KD. Patients’ height and weight were recorded.
Echocardiography and Coronary Measurements
Echocardiographic studies were performed on either a GE Vivid echocardiograph (GE Healthcare, Milwaukee, WI) or a Philips iE33 echocardiograph (Philips Medical Systems, Bothell, WA). All echocardiographic data used for this analysis were digitally recorded, allowing offline measurements with automatic calibration. Coronary measurements for all segments are routinely done for all new echocardiograms at our institution. For studies done between February 2001 and December 2002, CAs were measured “live,” while subsequent data were remeasured offline by a single experienced senior sonographer. In preliminary analysis, there was no statistically significant difference in Z scores between these two periods. The intraluminal diameters of CA segments were measured from inner edge to inner edge. The left main CA (LMCA) was measured midway between the ostium and the bifurcation of the circumflex artery and the left anterior descending CA in the parasternal short-axis view. The proximal right CA (RCA), circumflex artery, and left anterior descending CA measurements were obtained 3 to 5 mm distal to their origin in the parasternal short-axis view. The RCA segments measured beyond the proximal portion were in the subcostal view at the lateral aspect of the atrioventricular sulcus for the mid segment and in the apical view with caudal inclination at the posterior aspect of the atrioventricular sulcus for the distal segment. The aortic valve measurement was performed in the parasternal long-axis view at the anterior and posterior hinge points during early systole. CA and aortic valve diameters are expressed in millimeters, weight in kilograms, height in centimeters, and BSA in square meters.
Statistical Analyses
Regression was used to model the relation of CA diameter with four different independent variables: weight, height, BSA, and aortic valve diameter. Models using linear ( y = a + β 1 x ), logarithmic ( y = a + β 1 ln[ x ]), power ( y = a + β 1 x 2 + β 2 x ), exponential (ln[ y ] = a + β 1 ln[ x ]), and square root ( y = a + β 1 √ x ) relations were tested. Visual inspection and the R 2 score were used to determine which model best fitted the data.
Heteroscedasticity was considered present if the residual absolute values modeled with the independent variable displayed a statistically significant positive trend, or if either the White test or the Breusch-Pagan test was significant at the α = 0.05 level. When significant heteroscedasticity was detected, it was approached using the weighted least squares method. We first calculated the residual for each observation (the observed value minus the modeled predicted value). We then modeled the absolute value of that residual using linear regression and used the inverse of the result of this model to attribute a weight to each value. The aim of this operation was to redistribute the weight in the model so that standard error would remain constant as the value of the independent variable increased. Outliers were identified visually and using the Dffits method. Two observations were omitted in the final analysis because they significantly deviated from the models.
Although the original BSA estimation by DuBois and DuBois is still widely used, other enhanced formulas better suited to estimate BSA for infants and children have been developed. We used Haycock et al. ’s equation to compute BSA. For comparison, we also modeled CA diameter with BSA estimated with Du Bois and Du Bois’s and Mosteller’s formulas.
The Z score is a standardized score that indicates by how many standard deviations a value is above or below the mean in a normally distributed population. By definition, Z scores must also be normally distributed with a mean of zero and a standard deviation of one. In each model, we computed CA Z score by dividing the residual value by the modeled standard error of the residual value. To assess Z -score validity in our population, we used the Anderson-Darling test to measure the goodness of fit of our computed Z score for a normal distribution with a mean of zero and a standard deviation of one. A significant P value indicates a statistically significant departure from such a distribution. To evaluate Z scores’ stability with the independent variable, we also modeled computed Z scores with that independent variable. A statistically significant positive slope indicates an increasing overestimation of Z score as the value of the independent variable increases. Finally, we calculated CA Z scores from our cohort’s raw data using four previously published CA Z -score equations. The validity of these scores with our observations was also tested as described above.
We used SAS for Windows version 9.1 (SAS Institute Inc., Cary, NC) for all analyses. All regressions were performed using the REG procedure. The standard least squares method was used for R 2 score. Weighted least squares regressions were performed with the “weight” statement in the REG procedure. We used the MODEL procedure to detect heteroscedasticity. The t score was used to compute P value in linear regression. The t test was used to compare Z scores between groups. A P value < .05 was considered statistically significant.
Results
Population Description
A total of 1,036 echocardiographic studies were available in our database. Of them, three studies were excluded (two were considered major outliers and one had missing data for height and weight). There were 49.3% male patients. Weight ranged from 0.8 to 135.0 kg (median, 21.5 kg; interquartile range, 33 kg); 5.8% and 2.1% of subjects weighted <5 kg and <3 kg, respectively. There were 15.3% and 43.0% of participants between 5 and 10 kg and between 10 and 30 kg, respectively. Height ranged from 27.8 to 191.0 cm (median, 115 cm; interquartile range, 68.5 cm). Final analyses included measurements for 996 LMCAs, 869 left anterior descending CAs, 841 circumflex arteries, 982 proximal RCAs, 750 mid RCAs, and 711 distal RCAs.
Preliminary Models
CA diameter was first modeled with weight, height, and BSA. Linear, logarithmic, exponential, power, and square root models were fitted. Parameter estimates, R 2 scores, and statistical tests for heteroscedasticity are presented in Tables 1 and 2 for the LMCA and proximal RCA, respectively. According to R 2 scores, fit with weight, height, and BSA was overall similar although slightly better with BSA. The best fit for LMCA was obtained using the square root model with BSA ( R 2 = 0.796) and the exponential model with BSA ( R 2 = 0.794). R 2 scores were >0.7 for all models. Results for the proximal RCA were comparable, and the best fit was obtained using the square root model with BSA.
Model characteristics ∗ | Model estimates ∗ | Heteroscedasticity | |||||
---|---|---|---|---|---|---|---|
Relation | Independent variable | Intercept | β 1 | β 2 | R 2 | White test P value | Breusch-Pagan test P value |
Linear | Weight | 1.498 | 0.036 | 0.703 | <.0001 | <.0001 | |
Height | 0.232 | 0.020 | 0.763 | <.0001 | <.0001 | ||
BSA | 1.058 | 1.578 | 0.764 | <.0001 | <.0001 | ||
Logarithmic | Weight | 0.073 | 0.815 | 0.763 | <.0001 | <.0001 | |
Height | −7.394 | 1.906 | 0.748 | <.0001 | <.0001 | ||
BSA | 2.811 | 1.160 | 0.764 | <.0001 | <.0001 | ||
Exponential | Weight | −0.289 | 0.381 | 0.793 | .005 | .085 | |
Height | −3.321 | 0.893 | 0.768 | <.0001 | .009 | ||
BSA | 0.992 | 0.544 | 0.794 | .002 | .046 | ||
Power | Weight | 1.198 | −0.0003 | 0.062 | 0.757 | <.0001 | <.0001 |
Height | 0.469 | 0.00002 | 0.015 | 0.763 | <.0001 | <.0001 | |
BSA | 0.763 | −0.384 | 2.362 | 0.779 | <.0001 | <.0001 | |
Square root | Weight | 0.557 | 0.399 | 0.782 | <.0001 | <.0001 | |
Height | −1.679 | 0.398 | 0.762 | <.0001 | <.0001 | ||
BSA | −0.182 | 2.924 | 0.796 | <.0001 | <.0001 |
∗ Model characteristics: linear ( y = a + β 1 x ), logarithmic ( y = a + β 1 ln[ x ]), power ( y = a + β 1 x 2 + β 2 x ), exponential (ln[ y ] = a + β 1 ln[ x ]), and square root ( y = a + β 1 √ x ), where y is the CA and x is the independent variable.
Model characteristics ∗ | Model estimates ∗ | Heteroscedasticity | |||||
---|---|---|---|---|---|---|---|
Relation | Independent variable | Intercept | β 1 | β 2 | R 2 | White test P value | Breusch-Pagan test P value |
Linear | Weight | 1.239 | 0.035 | 0.702 | <.0001 | <.0001 | |
Height | 0.085 | 0.019 | 0.740 | <.0001 | <.0001 | ||
BSA | 0.827 | 1.522 | 0.751 | <.0001 | <.0001 | ||
Logarithmic | Weight | −0.035 | 0.758 | 0.720 | <.0001 | <.0001 | |
Height | −5.961 | 1.754 | 0.708 | <.0001 | <.0001 | ||
BSA | 2.509 | 1.070 | 0.723 | <.0001 | <.0001 | ||
Exponential | Weight | −0.491 | 0.405 | 0.761 | .002 | .281 | |
Height | −3.657 | 0.945 | 0.737 | .002 | .853 | ||
BSA | 0.869 | 0.577 | 0.762 | .001 | .336 | ||
Power | Weight | 0.997 | −0.0003 | 0.057 | 0.738 | <.0001 | <.0001 |
Height | 0.502 | 0.00004 | 0.001 | 0.745 | <.0001 | <.0001 | |
BSA | 0.660 | −0.228 | 1.979 | 0.757 | <.0001 | <.0001 | |
Square root | Weight | 0.368 | 0.380 | 0.761 | <.0001 | <.0001 | |
Height | −1.672 | 0.371 | 0.733 | <.0001 | <.0001 | ||
BSA | −0.304 | 2.752 | 0.768 | <.0001 | <.0001 |
∗ Model characteristics: linear ( y = a + β 1 x ), logarithmic ( y = a + β 1 ln[ x ]), power ( y = a + β 1 x 2 + β 2 x ), exponential (ln[ y ] = a + β 1 ln[ x ]), and square root ( y = a + β 1 √ x ), where y is the CA and x is the independent variable.
Analyses of Z -score distribution and trend are shown in Tables 3 and 4 for the LMCA and proximal RCA, respectively. Many models yielded Z scores with significant departures from a normal distribution, including models that were well fitted, such as exponential models with BSA or weight. Overall, Z scores obtained with BSA as the independent variable showed less departure from a normal distribution than those derived from models fitted with weight and height. For LMCA, the “best” normal distribution (the lowest A score) was obtained with the square root and the power models with BSA. For proximal RCA, the square root model also yielded the Z scores closest to a normal distribution. However, in contrast to the LMCA, the exponential model of the proximal RCA with BSA rendered Z scores with significant departures from a normal distribution ( P = .041). Because the regression fit and the distribution tended to be better with BSA as the independent variable for both the LMCA and the proximal RCA, further analyses were computed only with BSA.
Model characteristics | Departure from normal distribution ∗ | Trend of Z score † | ||||
---|---|---|---|---|---|---|
Relation | Independent variable | A 2 | P | Skewness | β | P |
Linear | Weight | 1.323 | 0.229 | −0.177 | −0.003 | .049 |
Height | 1.394 | 0.210 | 0.467 | 0.000 | .768 | |
BSA | 0.606 | >0.25 | −0.036 | −0.061 | .283 | |
Logarithmic | Weight | 2.806 | 0.036 | 0.547 | 0.009 | <.0001 |
Height | 4.630 | 0.005 | 0.687 | 0.003 | <.0001 | |
BSA | 3.017 | 0.027 | 0.053 | 0.369 | <.0001 | |
Exponential | Weight | 3.389 | 0.019 | −0.444 | −0.001 | .386 |
Height | 1.186 | >0.25 | −0.162 | 0.001 | .778 | |
BSA | 2.816 | 0.036 | −0.405 | −0.012 | .841 | |
Power | Weight | 0.546 | >0.25 | 0.003 | 0.000 | .956 |
Height | 1.051 | >0.25 | 0.408 | 0.000 | .957 | |
BSA | 0.263 | >0.25 | 0.093 | −0.003 | .969 | |
Square root | Weight | 0.399 | >0.25 | 0.001 | −0.003 | .046 |
Height | 2.589 | 0.046 | 0.576 | 0.001 | .087 | |
BSA | 0.297 | >0.25 | 0.125 | 0.020 | .745 |
∗ Anderson-Darling test for normal distribution with a mean of zero and a standard deviation of one. Higher A values and lower P values indicate a higher probability of departure from normality.
† Beta estimate (slope) from linear regression of Z scores against the dependent variable.
Model characteristics | Departure from normal distribution ∗ | Trend of Z score † | ||||
---|---|---|---|---|---|---|
Relation | Independent variable | A 2 | P | Skewness | β | P |
Linear | Weight | 0.362 | >0.25 | −0.013 | −0.002 | .093 |
Height | 1.039 | >0.25 | 0.400 | 0.001 | .418 | |
BSA | 0.479 | >0.25 | −0.008 | −0.047 | .445 | |
Logarithmic | Weight | 3.287 | 0.021 | 0.563 | 0.011 | <.0001 |
Height | 5.865 | 0.001 | 0.630 | 0.004 | <.0001 | |
BSA | 3.764 | 0.012 | 0.577 | 0.454 | <.0001 | |
Exponential | Weight | 2.822 | 0.036 | −0.400 | 0.001 | .617 |
Height | 1.196 | >0.25 | −0.260 | 0.001 | .487 | |
BSA | 2.689 | 0.041 | −0.394 | 0.047 | .447 | |
Power | Weight | 0.436 | >0.25 | −0.008 | 0.000 | .960 |
Height | 0.514 | >0.25 | 0.306 | 0.000 | .948 | |
BSA | 0.435 | >0.25 | 0.025 | −0.002 | .979 | |
Square root | Weight | 0.442 | >0.25 | 0.027 | 0.001 | .561 |
Height | 2.674 | 0.042 | 0.513 | 0.002 | .011 | |
BSA | 0.391 | >0.25 | 0.131 | 0.097 | .112 |
∗ Anderson-Darling test for normal distribution with a mean of zero and a standard deviation of one. Higher A values and lower P values indicate a higher probability of departure from normality.
† Beta estimate (slope) from linear regression of Z scores against the dependent variable.
Final Models
Figures 1 A to 1 E present regression results for the LMCA for all five models with Z = 0, Z = 2, and Z = −2 boundaries. Visual inspection clearly showed a nonlinear relationship with significant heteroscedasticity. All models offered good fit for higher BSA, but only the square root and exponential models fitted the steeper slope of smaller children (BSA < 0.3 m 2 ). Figures 1 F to 1 J present plots of Z scores against BSA for all five models. These plots are useful for visual inspection because valid Z scores must be symmetrically distributed around zero, and their scattering must be constant across the entire range of BSA. Visually, only the square root and exponential model yielded Z scores evenly distributed around zero for small children. The departure from a normal distribution of Z scores observed with the exponential model was most likely explained by the overall asymmetric distribution around zero (greater dispersion for negative Z scores with a negative skewness score of −0.362) or by the artificial compression of higher values caused by the logarithmic transformation, possibly both. Again, results for the RCA were equivalent (data not shown).

With similar overall fit, the square root model with BSA offered a better fit to the CAs of smaller children and, most important, yielded Z scores with a distribution that did not fail the normality test. Such a normal distribution is critical because the principal use of a Z score is that it allows one to estimate the proportion of observations that lie above a given value of Z in a population, but these proportions are valid only if the Z score is normally distributed. Accordingly, the square root model with BSA was used to compute the final regression estimates for all six CA segments ( Table 5 ). Departure from a normal distribution was observed for the distal RCA mainly because of positive skew of 0.795. Tables with Z scores boundaries for all CA segments are presented in the Appendix . Z scores can be calculated using the results of Table 5 in the following equation:
Z = CA obs − [ a + ( b × BSA ) ] a SE + ( b SE × BSA ) ,
Model estimates | Standard error | Departure from normal distribution ∗ | ||||||
---|---|---|---|---|---|---|---|---|
Coronary artery segment | n | R 2 | Intercept | β | Intercept | β | A 2 | P |
LMCA | 996 | 0.797 | −0.1817 | 2.9238 | 0.1801 | 0.2530 | 0.298 | >.25 |
LAD | 869 | 0.727 | −0.1502 | 2.2672 | 0.1709 | 0.2293 | 1.051 | >.25 |
Cx | 841 | 0.709 | −0.2716 | 2.3458 | 0.1142 | 0.3423 | 0.952 | >.25 |
RCA | ||||||||
Proximal | 982 | 0.768 | −0.3039 | 2.7521 | 0.1626 | 0.2881 | 0.391 | >.25 |
Medial | 750 | 0.720 | −0.3060 | 2.4078 | 0.1324 | 0.3259 | 1.314 | .232 |
Distal | 711 | 0.707 | −0.3185 | 2.3295 | 0.1099 | 0.3198 | 3.271 | .021 |
∗ Anderson-Darling test for normal distribution with a mean of zero and a standard deviation of one. Higher A values and lower P values indicate a higher probability of departure from normality.
Gender
When the influence of subjects’ gender was studied, we found no difference between predicted LMCA diameter (0.04-mm difference, P = .429) and predicted Z = 2 boundary (0.02-mm difference, P = .757) according to gender. However, a slight but statistically significant difference was observed for Z scores according to the gender of the subjects ( Z -score mean difference, 0.25; P < .001). When parameters were recalculated separately for both genders, the gender-specific Z = 2 boundaries differed significantly but at most by 0.15 mm, often <0.1 mm. We felt that such a difference was not clinically relevant. Consequently, gender-specific models were not further developed to avoid a decrease in statistical power.
Effect of BSA Estimation
Regression results from the LMCA were compared when different BSA estimation methods were used. When the square root model was computed using BSA estimated with DuBois and DuBois’s method, the Z scores obtained were almost identical to those obtained with BSA estimated with Haycock et al. ’s equation ( P = .951). Similar results were obtained when comparing Mosteller’s equation and Haycock et al. ’s equation ( P = .928). Z -score differences were almost always <0.1. When BSAs estimated with DuBois and DuBois’s formula were put in the Z -score equation computed with a different BSA estimation (such as the one in Table 5 ), the Z scores obtained were very similar, and misclassification was observed for only 0.3% of subjects (classified as abnormal [ Z > 2] with BSA from DuBois and DuBois but normal with BSA from Haycock et al. ).
Model With Aortic Valve Annulus
Similar to previously published findings, our preliminary analyses showed that the ratio of CA over aortic valve diameter was only very slightly influenced by height, weight, or BSA ( R 2 = 0.037, 0.016, and 0.030, respectively). A second model was consequently developed using aortic valve diameter as the independent variable. Visually, an inflexion point was apparent and seemed to coincide with the beginning of puberty ( Figure 2 ). When subjects with heights > 140 cm were excluded, the relation was mostly linear. Heteroscedasticity was not obvious by visual inspection but was nonetheless present ( P = .0041). When weighted least squares linear models were computed, it was found that although the variation of the standard error with BSA was statistically significant, its increase with BSA was clinically irrelevant (0.48% increase of the standard error for each millimeter increase of aortic annular diameter). The difference between Z scores from weighted and nonweighted least squares models was not significantly different from zero ( P = .943), with <1% of subject with Z -score differences > 0.1.

Linear regression models for the LMCA and proximal RCA with aortic valve diameter had R 2 scores of 0.578 and 0.549, respectively, and Z scores were normally distributed ( A scores of 0.379 and 0.531, P > .25 and P > .25, for the LMCA and proximal RCA, respectively). Table 6 shows the regression results for all coronary segments for subjects <140 cm and >3.0 kg. Similar to models with BSA, distal RCA Z scores departed from normal distribution. The statistical power to estimate the equivalent regression in postpubertal subjects was insufficient. Z scores can be calculated using the results of Table 6 in the following equation:
Z = CA obs − [ a + ( b × Ao ) ] SE ,
Model estimates | Departure from normal distribution ∗ | ||||||
---|---|---|---|---|---|---|---|
Coronary artery segment | n | Intercept | β | Standard error | R 2 | A 2 | P |
LMCA | 649 | 0.3593 | 0.1398 | 0.3507 | 0.578 | 0.379 | >.25 |
LAD | 577 | 0.2364 | 0.1109 | 0.3409 | 0.491 | 1.137 | >.25 |
Cx | 596 | 0.1940 | 0.1081 | 0.3673 | 0.436 | 0.940 | >.25 |
RCA | |||||||
Proximal | 636 | 0.1812 | 0.1320 | 0.3579 | 0.549 | 0.531 | >.25 |
Medial | 504 | 0.1827 | 0.1104 | 0.3748 | 0.443 | 1.041 | >.25 |
Distal | 511 | 0.2191 | 0.1003 | 0.3627 | 0.404 | 5.388 | .003 |
∗ Anderson-Darling test for normal distribution with a mean of zero and a standard deviation of one. Higher A values and lower P values indicate a higher probability of departure from normality.
We compared LMCA Z scores obtained with the square root model with BSA and the linear model with aortic valve. They were highly correlated ( R 2 = 0.729), and their means were not significantly different (mean difference, 0.0002; P = .990). However, the linear model with aortic valve tended to slightly underestimate Z scores for extreme values compared with the square root model with BSA. However, disagreement between the two methods ( Z scores > 2.0 with one method but not with the other) was observed in <2% of subjects.
Comparison With Previous Equations
We analyzed Z scores obtained with our cohort using four previously published equations. Figure 3 presents the LMCA cutoff of Z = –2 and Z = 2 for the current equation and previous equations. Only equations proposed by McCrindle et al. and Olivieri et al. accounted for the nonlinear relation of CA and BSA ( Figure 3 B). Visually, the upper boundaries of these two latter equations seemed adequate, but the steeper slope observed for children at low BSA value was less well fitted. Table 7 shows the results of the statistical test for normality and of regressions of Z values against BSA. All methods yielded statistically significant departure from normality (Anderson-Darling test) and a statistically significant positive trend of Z scores with BSA. Regarding CA/aorta ratios, the mean Z score computed with the equation published by Tan et al. was high (1.7618), and >25% of LMCA measurements had Z scores > 2. Similarly, for the LMCA and proximal RCA, respectively, 25% and 20% of our measurements were above the cutoff ratios of 19% and 17% suggested by the same authors.
Departure from normal distribution ∗ | Trend † | ||||||
---|---|---|---|---|---|---|---|
Equation source | Mean | Standard deviation | A 2 | P | Intercept | β | β P value |
Expected from theoretical Z -score distribution | 0 | 1 | 0 | 1 | 0 | 0 | 1 |
McCrindle et al. | −0.434 | 1.012 | 1.459 | <.005 | −1.183 | 0.803 | <.001 |
Tan et al. (with BSA) | 1.789 | 2.581 | 1.438 | <.005 | 1.494 | 0.316 | .047 |
Tan et al. (with aortic valve) | 1.761 | 2.641 | 11.802 | <.005 | −1.648 | 0.234 | <.001 |
Olivieri et al. | −0.208 | 1.196 | 3.214 | <.005 | −1.271 | 1.140 | <.001 |
De Zorzi et al. | −0.116 | 1.286 | 4.262 | <.005 | −1.384 | 1.364 | <.001 |
∗ Anderson-Darling test for normal distribution. Higher A values and lower P values indicate a higher probability of departure from normality.
† Beta estimate (slope) from linear regression of Z scores against the dependent variable.
Results
Population Description
A total of 1,036 echocardiographic studies were available in our database. Of them, three studies were excluded (two were considered major outliers and one had missing data for height and weight). There were 49.3% male patients. Weight ranged from 0.8 to 135.0 kg (median, 21.5 kg; interquartile range, 33 kg); 5.8% and 2.1% of subjects weighted <5 kg and <3 kg, respectively. There were 15.3% and 43.0% of participants between 5 and 10 kg and between 10 and 30 kg, respectively. Height ranged from 27.8 to 191.0 cm (median, 115 cm; interquartile range, 68.5 cm). Final analyses included measurements for 996 LMCAs, 869 left anterior descending CAs, 841 circumflex arteries, 982 proximal RCAs, 750 mid RCAs, and 711 distal RCAs.
Preliminary Models
CA diameter was first modeled with weight, height, and BSA. Linear, logarithmic, exponential, power, and square root models were fitted. Parameter estimates, R 2 scores, and statistical tests for heteroscedasticity are presented in Tables 1 and 2 for the LMCA and proximal RCA, respectively. According to R 2 scores, fit with weight, height, and BSA was overall similar although slightly better with BSA. The best fit for LMCA was obtained using the square root model with BSA ( R 2 = 0.796) and the exponential model with BSA ( R 2 = 0.794). R 2 scores were >0.7 for all models. Results for the proximal RCA were comparable, and the best fit was obtained using the square root model with BSA.
Model characteristics ∗ | Model estimates ∗ | Heteroscedasticity | |||||
---|---|---|---|---|---|---|---|
Relation | Independent variable | Intercept | β 1 | β 2 | R 2 | White test P value | Breusch-Pagan test P value |
Linear | Weight | 1.498 | 0.036 | 0.703 | <.0001 | <.0001 | |
Height | 0.232 | 0.020 | 0.763 | <.0001 | <.0001 | ||
BSA | 1.058 | 1.578 | 0.764 | <.0001 | <.0001 | ||
Logarithmic | Weight | 0.073 | 0.815 | 0.763 | <.0001 | <.0001 | |
Height | −7.394 | 1.906 | 0.748 | <.0001 | <.0001 | ||
BSA | 2.811 | 1.160 | 0.764 | <.0001 | <.0001 | ||
Exponential | Weight | −0.289 | 0.381 | 0.793 | .005 | .085 | |
Height | −3.321 | 0.893 | 0.768 | <.0001 | .009 | ||
BSA | 0.992 | 0.544 | 0.794 | .002 | .046 | ||
Power | Weight | 1.198 | −0.0003 | 0.062 | 0.757 | <.0001 | <.0001 |
Height | 0.469 | 0.00002 | 0.015 | 0.763 | <.0001 | <.0001 | |
BSA | 0.763 | −0.384 | 2.362 | 0.779 | <.0001 | <.0001 | |
Square root | Weight | 0.557 | 0.399 | 0.782 | <.0001 | <.0001 | |
Height | −1.679 | 0.398 | 0.762 | <.0001 | <.0001 | ||
BSA | −0.182 | 2.924 | 0.796 | <.0001 | <.0001 |
∗ Model characteristics: linear ( y = a + β 1 x ), logarithmic ( y = a + β 1 ln[ x ]), power ( y = a + β 1 x 2 + β 2 x ), exponential (ln[ y ] = a + β 1 ln[ x ]), and square root ( y = a + β 1 √ x ), where y is the CA and x is the independent variable.
Model characteristics ∗ | Model estimates ∗ | Heteroscedasticity | |||||
---|---|---|---|---|---|---|---|
Relation | Independent variable | Intercept | β 1 | β 2 | R 2 | White test P value | Breusch-Pagan test P value |
Linear | Weight | 1.239 | 0.035 | 0.702 | <.0001 | <.0001 | |
Height | 0.085 | 0.019 | 0.740 | <.0001 | <.0001 | ||
BSA | 0.827 | 1.522 | 0.751 | <.0001 | <.0001 | ||
Logarithmic | Weight | −0.035 | 0.758 | 0.720 | <.0001 | <.0001 | |
Height | −5.961 | 1.754 | 0.708 | <.0001 | <.0001 | ||
BSA | 2.509 | 1.070 | 0.723 | <.0001 | <.0001 | ||
Exponential | Weight | −0.491 | 0.405 | 0.761 | .002 | .281 | |
Height | −3.657 | 0.945 | 0.737 | .002 | .853 | ||
BSA | 0.869 | 0.577 | 0.762 | .001 | .336 | ||
Power | Weight | 0.997 | −0.0003 | 0.057 | 0.738 | <.0001 | <.0001 |
Height | 0.502 | 0.00004 | 0.001 | 0.745 | <.0001 | <.0001 | |
BSA | 0.660 | −0.228 | 1.979 | 0.757 | <.0001 | <.0001 | |
Square root | Weight | 0.368 | 0.380 | 0.761 | <.0001 | <.0001 | |
Height | −1.672 | 0.371 | 0.733 | <.0001 | <.0001 | ||
BSA | −0.304 | 2.752 | 0.768 | <.0001 | <.0001 |
∗ Model characteristics: linear ( y = a + β 1 x ), logarithmic ( y = a + β 1 ln[ x ]), power ( y = a + β 1 x 2 + β 2 x ), exponential (ln[ y ] = a + β 1 ln[ x ]), and square root ( y = a + β 1 √ x ), where y is the CA and x is the independent variable.
Analyses of Z -score distribution and trend are shown in Tables 3 and 4 for the LMCA and proximal RCA, respectively. Many models yielded Z scores with significant departures from a normal distribution, including models that were well fitted, such as exponential models with BSA or weight. Overall, Z scores obtained with BSA as the independent variable showed less departure from a normal distribution than those derived from models fitted with weight and height. For LMCA, the “best” normal distribution (the lowest A score) was obtained with the square root and the power models with BSA. For proximal RCA, the square root model also yielded the Z scores closest to a normal distribution. However, in contrast to the LMCA, the exponential model of the proximal RCA with BSA rendered Z scores with significant departures from a normal distribution ( P = .041). Because the regression fit and the distribution tended to be better with BSA as the independent variable for both the LMCA and the proximal RCA, further analyses were computed only with BSA.
Model characteristics | Departure from normal distribution ∗ | Trend of Z score † | ||||
---|---|---|---|---|---|---|
Relation | Independent variable | A 2 | P | Skewness | β | P |
Linear | Weight | 1.323 | 0.229 | −0.177 | −0.003 | .049 |
Height | 1.394 | 0.210 | 0.467 | 0.000 | .768 | |
BSA | 0.606 | >0.25 | −0.036 | −0.061 | .283 | |
Logarithmic | Weight | 2.806 | 0.036 | 0.547 | 0.009 | <.0001 |
Height | 4.630 | 0.005 | 0.687 | 0.003 | <.0001 | |
BSA | 3.017 | 0.027 | 0.053 | 0.369 | <.0001 | |
Exponential | Weight | 3.389 | 0.019 | −0.444 | −0.001 | .386 |
Height | 1.186 | >0.25 | −0.162 | 0.001 | .778 | |
BSA | 2.816 | 0.036 | −0.405 | −0.012 | .841 | |
Power | Weight | 0.546 | >0.25 | 0.003 | 0.000 | .956 |
Height | 1.051 | >0.25 | 0.408 | 0.000 | .957 | |
BSA | 0.263 | >0.25 | 0.093 | −0.003 | .969 | |
Square root | Weight | 0.399 | >0.25 | 0.001 | −0.003 | .046 |
Height | 2.589 | 0.046 | 0.576 | 0.001 | .087 | |
BSA | 0.297 | >0.25 | 0.125 | 0.020 | .745 |
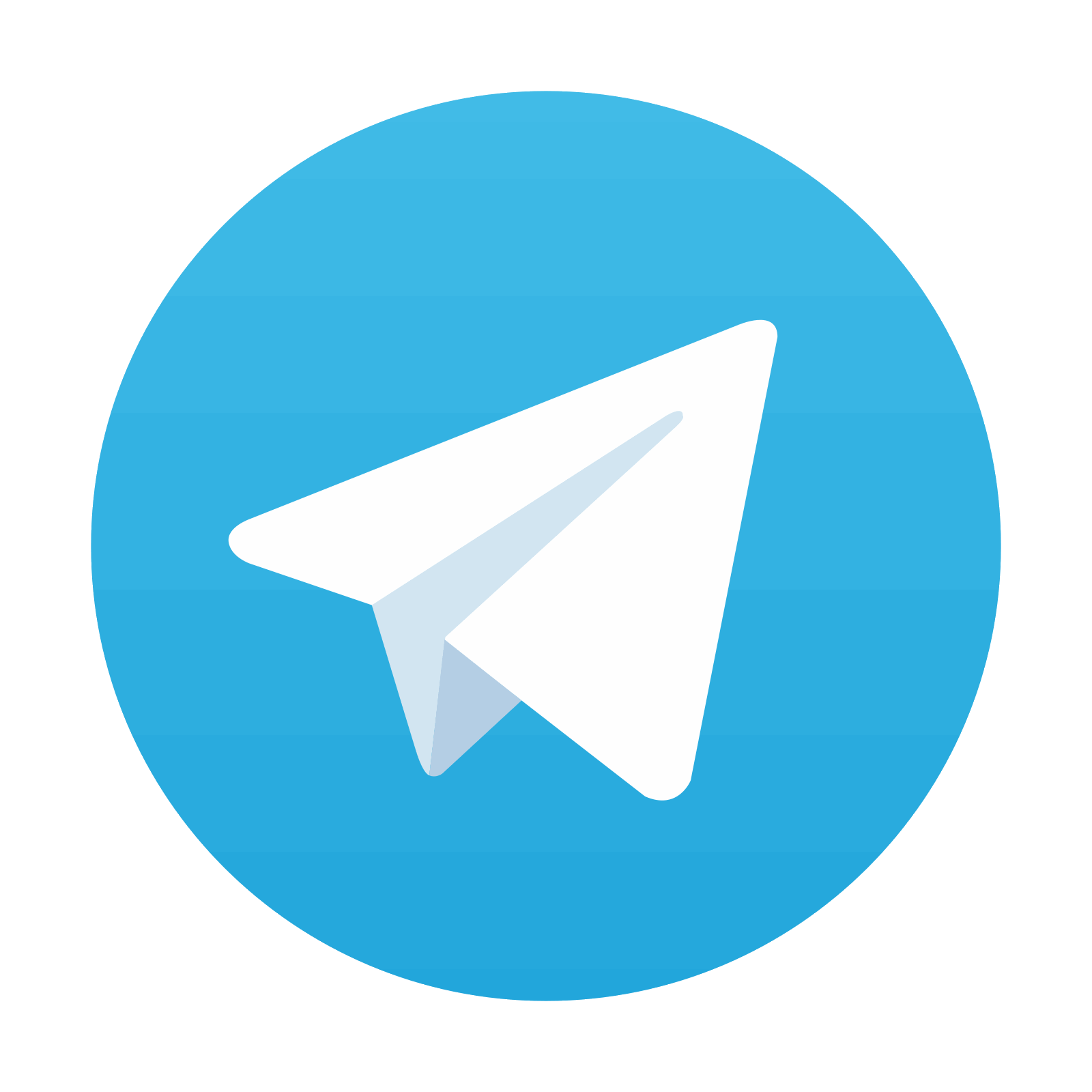
Stay updated, free articles. Join our Telegram channel

Full access? Get Clinical Tree
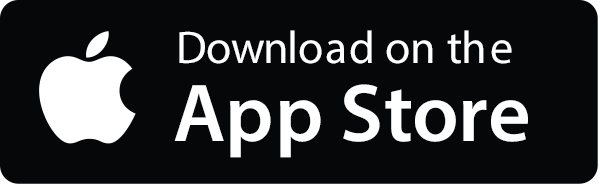

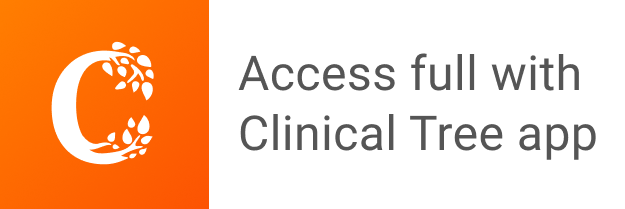