Background
Mitral annular displacement (MAD) is a simple marker of left ventricular (LV) systolic function. The aim of this study was to test the hypothesis that MAD can distinguish patients with non–ST-segment elevation myocardial infarctions (NSTEMIs) from those with significant coronary artery disease without infarctions, identify coronary occlusion, and predict mortality in patients with NSTEMIs. MAD was compared with established indices of LV function.
Methods
In this retrospective study, 167 patients with confirmed NSTEMIs were included at two Scandinavian centers. Forty patients with significant coronary artery disease but without myocardial infarctions were included as controls. Doppler tissue imaging was performed at the mitral level of the left ventricle in the three apical planes, and velocities were integrated over time to acquire MAD. LV ejection fraction, global longitudinal strain (GLS), and wall motion score index were assessed according to guidelines.
Results
MAD and GLS could accurately distinguish patients with NSTEMIs from controls. During 48.6 ± 12.1 months of follow-up, 22 of 167 died (13%). MAD, LV ejection fraction, and GLS were reduced and wall motion score index was increased among those who died compared with those who survived ( P < .001, P < .001, P < .001, and P = .02, respectively). Multivariate Cox proportional-hazards analyses revealed that MAD was an independent predictor of death (hazard ratio, 1.36; 95% confidence interval, 1.07–1.73; P = .01). MAD and GLS were reduced and wall motion score index was increased in patients with coronary artery occlusion compared with those without occlusion ( P = .006, P = .001, and P = .02), while LV ejection fraction did not differ ( P = .20).
Conclusions
MAD accurately identified patients with NSTEMIs, predicted mortality, and identified coronary occlusion in patients with NSTEMIs.
Coronary disease is highly prevalent in the Western world and is the main cause of mortality. Prompt diagnosis and treatment are essential. Acute myocardial infarction damages myocardial tissue and leads to impaired systolic function, and the extent of the damage correlates with clinical outcomes. Patients with ST-segment elevation myocardial infarctions (STEMIs) are treated urgently with acute reperfusion therapy to reduce infarct size and mortality. Many patients with non–ST-segment elevation myocardial infarctions (NSTEMIs) have occluded coronary arteries and probably share many of the pathophysiologic features of patients with STEMIs, but they do not receive acute revascularization therapy, because the occlusion is not readily identified. Occluded arteries in patients with NSTEMIs result in larger infarcts and impaired left ventricular (LV) function, and instant identification and quicker treatment of these patients could lead to a reduction of infarct size and increase survival. Electrocardiography has suboptimal sensitivity for the detection of acute myocardial infarction, identifying acute coronary occlusion, and has a modest correlation with infarct size. There is therefore a need for better diagnostic tools.
Systolic LV long-axis deformation decreases with increasing myocardial damage. In patients with NSTEMIs, occluded coronary arteries result in larger infarcts than in patients without occlusion, and increasing infarct size results in decreasing systolic function. Doppler tissue imaging depicts myocardial motion by measuring tissue velocity at specific myocardial regions. The integration of tissue velocity over time produces the absolute distance moved by that particular region. The position of the apex is relatively stationary throughout the cardiac cycle, and the longitudinal mitral annular displacement (MAD) reflects the global shortening deformation of the heart. Global longitudinal strain (GLS) by two-dimensional (2D) speckle-tracking echocardiography is an accurate measure of myocardial deformation, but the technique requires good visualization of the entire LV curvature and a cardiologist, and it is time consuming. The LV ejection fraction (LVEF) and wall motion score index (WMSI) are widely used but are also experience dependent and time consuming. In this study, we assessed whether a simple method such as MAD assessment could identify patients with NSTEMIs and, among these patients, identify coronary occlusion and predict mortality. The performance of MAD was compared with that of other indices of LV function.
Methods
In this retrospective study, a total of 167 patients with confirmed NSTEMI diagnoses, selected for angiography, were included. Oslo University Hospital is a tertiary coronary care center at which patients with non–ST-segment elevation acute coronary syndromes (NSTE-ACS) are referred for coronary angiography according to current guidelines. In the study period, 150 consecutive patients with NSTE-ACS were referred. Twenty-nine of these had unstable angina pectoris and were excluded. Of the remaining 121 patients with NSTEMIs, magnetic resonance imaging (MRI) was performed in 61 patients at follow-up 9 ± 3 months after NSTEMI. These 61 patients were ultimately included in our study from Oslo University Hospital. Karolinska Institute is a secondary coronary care center. Among patients admitted for NSTE-ACS, selection for angiography is done according to current guidelines. In the study period, 117 consecutive patients with NSTE-ACS were selected for angiography. Eleven patients with unstable angina pectoris were excluded, leaving a total of 106 patients with NSTEMIs to be included in our study from Karolinska Institute. At both centers, patients admitted for NSTE-ACS receive standard medical therapy (double-antiplatelet treatment, β-blockers, low–molecular weight heparin, and statins) if tolerable, according to guidelines. Forty patients with stable angina pectoris and significant coronary disease (>50% stenosis) and no myocardial infarction, as verified by cardiac MRI, were included as controls. Patient data, angiography results, echocardiographic films, and MRI results were acquired from the medical records. The diagnosis of NSTEMI was made according to guidelines. Major exclusion criteria were prior myocardial infarction (assessed by history, electrocardiographic findings, and angiographic signs of old coronary occlusions), evidence of STEMI during the admission, and any condition interfering with the patient’s ability to comply. The study was approved by the regional ethics committees, and all patients gave written informed consent.
Echocardiography
Seventy-five patients were examined immediately before angiography (on average 2.3 ± 0.9 days after the initial admission for NSTE-ACS), and 92 were examined shortly after (1.7 ± 1.5 days) angiography, which was performed within 72 hours of the initial hospital admission. Echocardiography was performed using Vivid 7 machines (GE Vingmed Ultrasound AS, Horten, Norway). Tissue Doppler velocity measurements were obtained from color 2D Doppler images and obtained at the basal part of the left ventricle in the three apical planes (four-chamber [anteroseptal, inferolateral], two-chamber [anterior, inferior], and long-axis [anterolateral, inferoseptal]) in EchoPAC (GE Vingmed Ultrasound AS). The traces were then exported as text files and imported to customized software (GHLab; Gripping Heart AB, Stockholm, Sweden), which calculated the displacement of each of the aforementioned points of the mitral annulus by integrating the velocity curves over time. MAD was defined as the part of the displacement curve corresponding to the interval from the onset of the QRS complex to the end of the T wave on the electrocardiogram. The mean value of these displacements represents the average MAD ( Figure 1 ).

Peak s′ and e′ were also acquired at the six basal parts of the left ventricle and averaged. E was acquired from mitral flow readings from the four-chamber apical views. Longitudinal strain was measured using speckle-tracking echocardiography in a 16-segment LV model.
Peak negative systolic strain, which represents the maximum systolic longitudinal strain, was noted for each segment. As a measure of global systolic function, values of all segments were averaged to obtain GLS.
WMSI was calculated from a 16-segment model. Each segment was analyzed individually and scored on the basis of its motion and systolic thickening. Each segment’s function was confirmed in multiple views. Segments were scored as follows: 1 = normal or hyperkinetic, 2 = hypokinetic, 3 = akinetic, and 4 = dyskinetic (or aneurysmatic). WMSI was derived as the sum of all scores divided by the number of segments visualized. LVEF was calculated using Simpson’s method.
Coronary Angiography
Coronary angiography was performed using standard techniques with digital image acquisition and storage. Significant stenosis was defined as a coronary stenosis >50%. Significant stenosis was found in 139 patients, of whom 56 (34% of 167) had coronary occlusions. Twenty-eight patients had no disease, 88 had single-vessel disease (24 with occlusions), 38 had two-vessel disease (22 with occlusions), and 13 had three-vessel disease (10 with occlusions). Revascularization was performed in all patients with significant stenosis according to current clinical indications (30% with coronary artery bypass grafting, 70% with percutaneous coronary intervention). Among patients with coronary occlusion ( n = 56), echocardiography was performed before revascularization in 44 and after revascularization in 12 patients. In all patients who underwent coronary artery bypass grafting, echocardiography was performed before surgery.
MRI
In the 61 patients from Oslo University Hospital, final infarct size was quantified by MRI 9 ± 3 months after inclusion, using a 1.5-T unit (Magnetom Sonata; Siemens Healthcare, Erlangen, Germany) in 29 patients and a 3-T unit (Philips Medical Systems, Andover, MA) in 32 patients. Late-enhancement images were obtained 10 to 20 min after the intravenous injection of 0.1 to 0.2 mmol/kg gadopentetate dimeglumine (Magnevist; Schering AG, Berlin, Germany) in multiple short-axis slices covering the entire left ventricle. Typical image parameters were as follows: slice thickness, 8 mm; gap, 2 mm; and inversion time, 270 ms. On each short-axis image, total myocardial area as well as the area of infarcted myocardium was manually drawn in the picture archiving and communication system (Sectra AG, Linköping, Sweden). Final infarct size was calculated as infarct volume as a percentage of total myocardial volume. In the MRI subpopulation, echocardiography was performed before angiography, thus representing the prerevascularization state. The patients included in our study had no previously known coronary disease and had not undergone coronary interventions before inclusion. Fifty-four of the 61 patients had significant stenosis and underwent revascularization. Between revascularization and follow-up, one patient had a reinfarction with minimal enzyme release (troponin T, 0.18 μg/L) in the same vessel as was initially treated. Both short-term and long-term mortality rates have been demonstrated to be increased in patients with infarct sizes ≥12%. Therefore, patients were also dichotomized by infarct size using 12% as a cutoff. MRI was performed depending on lab availability at follow-up and only in the patients at Oslo University Hospital. Although the patients were not strictly randomized to MRI, there was no systematic bias in giving priority to MRI referral.
Follow-Up
Data on all-cause mortality were acquired from patients’ medical records. The mean follow-up time was 48.6 ± 12.1 months from the date of the myocardial infarction.
Statistical Analysis
Continuous variables are presented as mean ± SD or as median (interquartile range). Differences between groups were analyzed using Student’s t test. Categorical variables are presented as number (percentage), and differences between groups were analyzed using χ 2 tests. Bivariate correlations are presented as Pearson’s coefficients. When performing receiver operating characteristic (ROC) curve analyses, we split the patient populations in half (automatically by PASW; IBM, Armonk, NY). We used half of the cohort to derive optimal cutoff values from ROC curves and then applied these values in the second half of the cohort, in which standard reference methods allowed us to determine true-negative or false-negative and positive cases and calculate levels of sensitivity, specificity, positive predictive value, and negative predictive value. The optimal cutoffs were defined as the values of the ROC curves that were closest to the upper left corner. The reliability of the optimal cutoff values was validated using bootstrap resampling (1,000 iterations), and 95% confidence intervals based on bootstrap percentiles are presented. Areas under the curve (AUCs) are presented with 95% confidence intervals. Univariate and multivariate Cox proportional-hazards regression analyses were performed to identify predictors of mortality (hazard ratios are given with 95% confidence intervals). Adjusted hazard ratios were obtained after adjustment for potential confounders. Event-free survival was analyzed using Kaplan-Meier curves. Differences in event rates over time were evaluated using log-rank tests. All statistical analyses were performed with PASW version 18, except for differences between ROC curves and bootstrapping, which were analyzed using MedCalc (MedCalc Software, Mariakerke, Belgium).
Results
Patient characteristics, medications, and risk factors are listed in Table 1 , and the different echocardiographic parameters are summarized in Table 2 .
Characteristic | Patients ( n = 167) | Controls ( n = 40) | P |
---|---|---|---|
Age (yrs) | 63.3 ± 11.8 | 59.8 ± 9.8 | .09 |
Body mass index (kg/m 2 ) | 27.1 ± 3.8 | 26.0 ± 3.7 | .11 |
Diabetes | 36 (22%) | 2 (5%) | .015 |
Hypertension ∗ | 81 (48%) | 20 (50%) | .87 |
Current smokers | 45 (31%) | 8 (20%) | .28 |
Hypercholesterolemia † | 155 (93%) | 30 (75%) | <.001 |
Men | 126 (75%) | 14 (35%) | <.001 |
β-blockers | 144 (86%) | 26 (65%) | .001 |
Statins | 155 (93%) | 30 (75%) | .001 |
Angiotensin-converting enzyme inhibitors/angiotensin receptor blockers | 92 (55%) | 12 (30%) | .004 |
Aspirin | 160 (96%) | 32 (80%) | <.001 |
∗ Use of antihypertensive medication.
† Use of statins or cholesterol >240 mg/dL at the time of angiography.
Variable | Coronary occlusion | No occlusion | Survival | Death | Correlation with infarct size by MRI ( r ) | Infarct size <12% | Infarct size ≥12% |
---|---|---|---|---|---|---|---|
MAD (mm) | 8.8 ± 2.4 | 10.3 ± 2.1 ∗ | 9.8 ± 2.1 | 7.9 ± 2.0 † | −0.46 † | 10.3 ± 2.2 | 8.6 ± 1.8 ∗ |
GLS (%) | −13.8 ± 3.3 | 16.4 ± 3.0 ∗ | −15.0 ± 3.1 | −10.9 ± 3.7 † | 0.68 † | −16.9 ± 2.2 | −12.6 ± 1.7 † |
LVEF (%) | 55 ± 8 | 55 ± 12 § | 53 ± 10 | 41 ± 12 † | −0.53 † | 58 ± 8 | 48 ± 9 ∗ |
WMSI | 1.2 ± 0.2 | 1.1 ± 0.2 ‡ | 1.1 ± 0.2 | 1.3 ± 0.3 ‡ | 0.74 † | 1.1 ± 0.1 | 1.4 ± 0.1 † |
Peak s′ (cm/sec) | 4.4 ± 1.0 | 5.2 ± 1.4 § | 5.0 ± 1.1 | 4.4 ± 1.0 ‡ | −0.36 ∗ | 5.1 ± 1.3 | 4.4 ± 1.1 § |
E/e′ ratio | 13.7 ± 7.7 | 12.4 ± 5.9 § | 12.7 ± 5.4 | 16.9 ± 7.9 ‡ | 0.30 ‡ | 10.5 ± 3.5 | 16.1 ± 9.8 § |
Identification of Patients with NSTEMIs
MAD, GLS, and LVEF were significantly reduced in patients with NSTEMIs compared with those with stable angina with significant coronary artery disease without signs of myocardial infarction (MAD, 9.6 ± 2.1 vs 12.5 ± 1.3 mm; GLS, 14.5 ± 3.5% vs 20.4 ± 2.3%; LVEF, 51.6 ± 11.0% vs 57.6 ± 7.0%; P < .001 for all). ROC analyses showed equal ( P = .12) and good AUCs for GLS and MAD, while LVEF did not reach statistical significance ( Figure 2 ). A cutoff value of 10.9 mm for MAD identified 67 of the 88 patients with NSTEMIs and falsely suggested NSTEMI in only two of the 16 patients with significant coronary disease without infarctions. The corresponding numbers for GLS were 73 and one patient ( Table 3 ).
Variable | AUC (95% CI) | P | Cutoff (95% CI) | Sensitivity | Specificity | PPV | NPV |
---|---|---|---|---|---|---|---|
MAD (mm) | 0.88 (0.81 to 0.95) | <.001 | 10.9 (9.5 to 11.2) | 0.76 | 0.87 | 0.97 | 0.40 |
GLS (%) | 0.94 (0.90 to 0.98) | <.001 | −18.1 (−16.4 to −18.7) | 0.83 | 0.94 | 0.99 | 0.50 |
LVEF (%) | 0.59 (0.48 to 0.72) | .17 |
Mortality
During a mean follow-up period of 48.6 ± 12.1 months, 22 patients (13%) died. Fourteen of 22 deaths were of cardiovascular causes, and the causes of death were unclear regarding the remaining eight patients. MAD, GLS, LVEF, and peak s′ were significantly lower and WMSI and E/e′ ratio higher in those who died than in those who survived ( Table 2 ). ROC curves for mortality are shown in Figure 3 , and AUCs and optimal cutoffs with sensitivity, specificity, positive predictive value, and negative predictive value are shown in Table 4 . Comparison of AUCs showed no significant differences between any of the indices for myocardial function. Age, diabetes, and hypertension rates were higher and the proportions of patients using aspirin and statins slightly lower among those who died than among survivors. In univariate Cox proportional-hazards analysis, age, diabetes, hypertension, LVEF, MAD, GLS, WMSI, peak s′, and E/e′ ratio were predictors of death. We restricted multivariate analysis to four variables because of few events. LVEF, GLS, WMSI, peak s′, and E/e′ ratio all correlated with MAD and were therefore not included in a multivariate regression analysis together with MAD. In Cox proportional-hazards multivariate analysis including age, diabetes, MAD, and hypertension, MAD, diabetes, and age remained independent predictors of death ( Table 5 ). However, in separate multivariate analyses, in which MAD was replaced by either LVEF, GLS, or WMSI, these indices could also predict mortality when analyzed along with age, diabetes, and hypertension ( Table 5 ). Thrombolysis In Myocardial Infarction risk score, N-terminal pro–brain natriuretic peptide, and left atrial volume were significantly higher in patients who died ( P = .002, P < .001, and P = .001, respectively) while there were no differences in LV deceleration time ( P = .26) and peak troponin I ( P = .45). The differences in MAD, GLS, LVEF, E/e′ ratio, Thrombolysis In Myocardial Infarction risk score, N-terminal pro–brain natriuretic peptide, and left atrial volume between those who died from definite cardiovascular causes ( n = 14) and those who survived remained significant ( P < .05 for all). Kaplan-Meier analysis showed that patients with MAD values in the first quartile (<8.0 mm) had significantly lower survival probability compared with patients with MAD values in the fourth quartile (≥11.0 mm) (log-rank P = .004; Figure 4 ).
Variable | AUC (95% CI) | P | Cutoff (95% CI) | Sensitivity | Specificity | PPV | NPV |
---|---|---|---|---|---|---|---|
MAD (mm) | 0.79 (0.67–0.88) | .004 | 9.1 (8.0–10.0) | 0.58 | 0.63 | 0.20 | 0.91 |
GLS (%) | 0.85 (0.75–0.93) | <.001 | 11.0 (8.4–13.8) | 0.42 | 0.88 | 0.35 | 0.91 |
LVEF (%) | 0.80 (0.68–0.89) | .003 | 44 (44–62) | 0.50 | 0.88 | 0.40 | 0.92 |
WMSI | 0.76 (0.65–0.86) | .008 | 1.3 (1.0–1.3) | 0.33 | 0.79 | 0.20 | 0.88 |
Peak s′ (cm/sec) | 0.69 (0.56–0.79) | .05 | 4.1 (2.6–5.2) | 0.33 | 0.86 | 0.27 | 0.89 |
E/e′ ratio | 0.76 (0.65–0.86) | .008 | 13.4 (8.3–16.9) | 0.50 | 0.72 | 0.22 | 0.90 |
Variable | Unadjusted | Adjusted | ||||
---|---|---|---|---|---|---|
HR | CI | P | HR | CI | P | |
MAD | 1.55 | 1.24–1.93 | <.001 | 1.34 § | 1.06–1.73 | .01 |
GLS | 1.38 | 1.22–1.56 | <.001 | 1.23 § | 1.08–1.40 | .002 |
LVEF ∗ | 1.47 | 1.24–1.74 | <.001 | 1.34 § | 1.12–1.60 | .002 |
WMSI † | 1.24 | 1.08–1.42 | .003 | 1.16 § | 1.02–1.33 | .02 |
Peak s′ | 1.64 | 1.08–2.50 | .02 | |||
E/e′ ratio | 1.08 | 1.03–1.13 | .003 | |||
Age ‡ | 1.55 | 1.27–1.90 | <.001 | 1.49 || | 1.20–1.85 | <.001 |
Diabetes | 3.34 | 1.44–7.73 | .005 | 2.37 || | 1.01–5.57 | .05 |
Hypertension | 3.91 | 1.44–10.59 | .007 | 2.42 || | 0.88–6.68 | .09 |
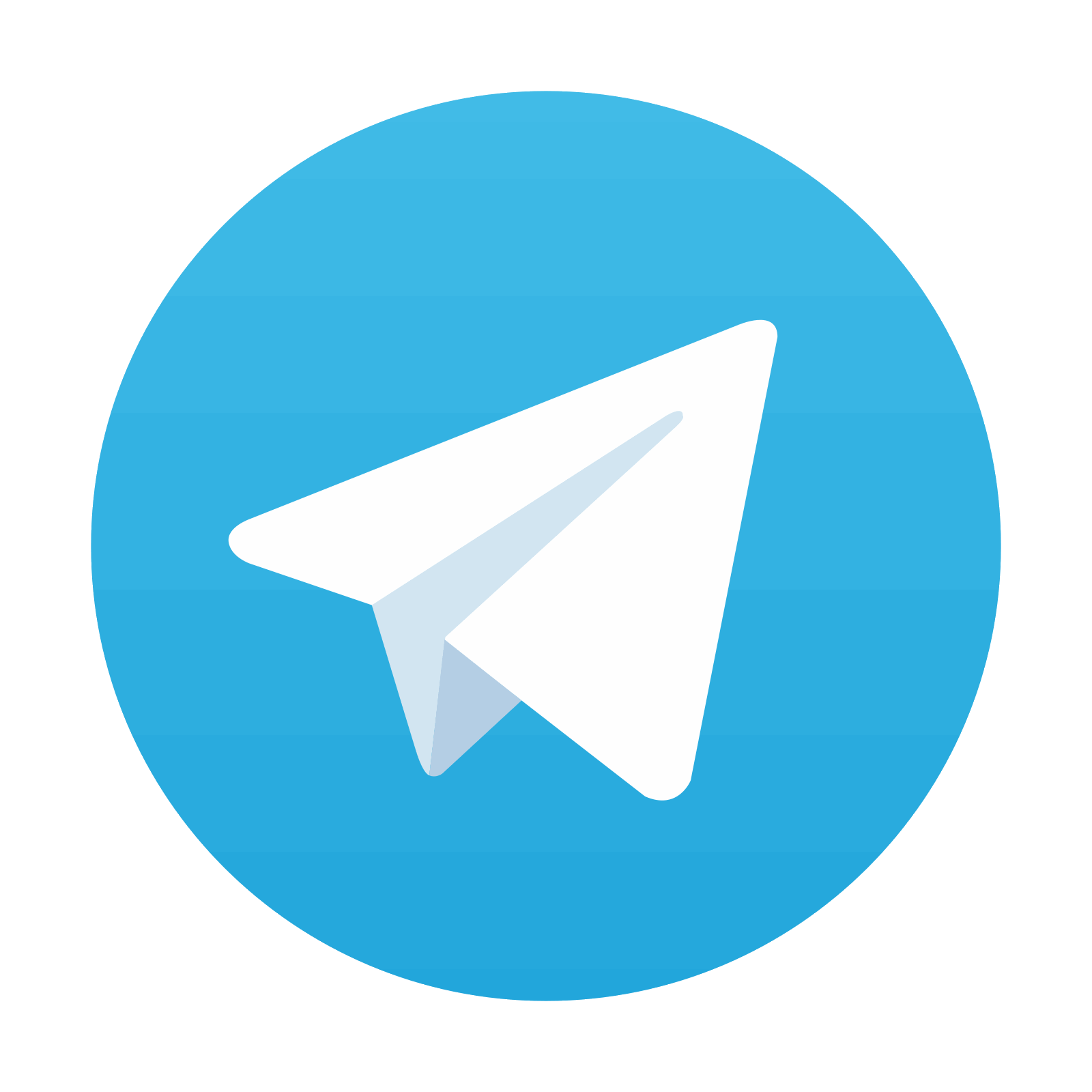
Stay updated, free articles. Join our Telegram channel

Full access? Get Clinical Tree
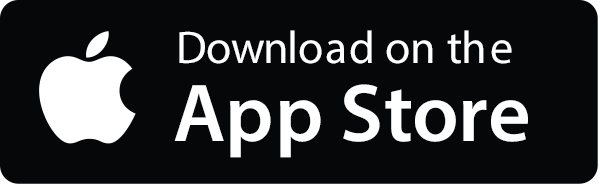

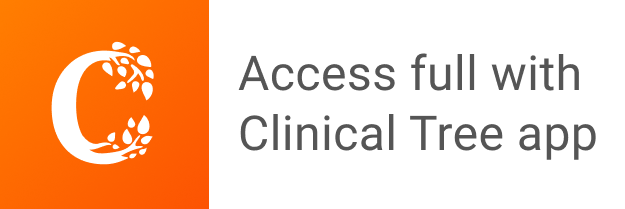